数据库
- 开放数据标准:Postgres,OTel,与Iceberg
- 数据库老司机:文章导航
- 小数据的失落十年:分布式分析的错付
- OpenAI:将PostgreSQL伸缩至新阶段
- Etcd 坑了多少公司?
- AI时代,软件从数据库开始
- MySQL vs PostgreSQL @ 2025
- 数据库火星撞地球:当PG爱上DuckDB
- 对比Oracle与PostgreSQL事务系统
- 数据库即业务架构
- 七周七数据库(2025年)
- 自建Supabase:创业出海的首选数据库
- 面向未来数据库的现代硬件
- PZ:MySQL还有机会赶上PostgreSQL的势头吗?
- 开源“暴君”Linus清洗整风
- 先优化碳基BIO核,再优化硅基CPU核
- MongoDB没有未来:好营销救不了烂芒果
- MongoDB: 现在由PostgreSQL强力驱动?
- 瑞士强制政府软件开源
- MySQL 已死,PostgreSQL 当立
- CVE-2024-6387 SSH 漏洞修复
- Oracle 还能挽救 MySQL 吗?
- Oracle最终还是杀死了MySQL
- MySQL性能越来越差,Sakila将何去何从?
- 国产数据库到底能不能打?
- 20刀好兄弟PolarDB:论数据库该卖什么价?
- Redis不开源是“开源”之耻,更是公有云之耻
- MySQL正确性竟如此垃圾?
- 数据库应该放入K8S里吗?
- 专用向量数据库凉了吗?
- 数据库真被卡脖子了吗?
- EL系操作系统发行版哪家强?
- 基础软件需要什么样的自主可控?
- 正本清源:技术反思录
- 数据库需求层次金字塔
- 分布式数据库是不是伪需求?
- 微服务是不是个蠢主意?
- 是时候和GPL说再见了
- 容器化数据库是个好主意吗?
- 理解时间:闰年闰秒,时间与时区
- 理解字符编码原理
- 并发异常那些事
- 区块链与分布式数据库
- 一致性:过载的术语
- 为什么要学习数据库原理
开放数据标准:Postgres,OTel,与Iceberg
作者:Paul Copplestone,Supabase CEO 译者:冯若航,Pigsty Founder,数据库老司机
数据世界正在浮出水面的三大新标准:Postgres、Open Telemetry,以及 Iceberg。
Postgres 基本已经是事实标准;OTel 和 Iceberg 尚在成长, 但它们具备当年让 Postgres 走红的同样配方。常有人问我:“为什么最后是 Postgres 赢了?” 标准答案是“可扩展性” —— 对,但不完整。
除了产品本身优秀,Postgres 还踩中了开源生态爆点 —— 关键在于“开源的姿势”本身。
开源的三个信条
我逐渐悟到,开发者判断一个项目“开源味”浓不浓,大致看三点:
- 许可证:是否为 OSI 核准 的开源协议。
- 自托管:能否把完整产品端到端地自己部署。
- 商业化:有没有商业中立、无厂商绑架;更妙的是,有 多家 公司背书而非一家独大。
第三点我领悟得最慢 —— 是的,Postgres 赢在产品力,但更赢在 “谁也控不住” 。 治理结构与社区文化决定了它不可能被任何公司收编。它就像国际空间站,多家公司只能合作,因为谁都没本事说 “这就是我的”。
Postgres 点满了 “开源” 技能点,但它也并非在所有数据场景里都是银弹。
三类数据角色
数据领域里主要有三种 “操盘手” 及其趁手工具:
- OLTP 数据库:开发者 写应用用。
- 遥测 / 观测:SRE 运维基建、调优应用用。
- OLAP / 数仓:数据工程师 / 科学家 挖掘洞见用。
数据生命周期通常是 1 → 2 → 3
:先有应用,再加点基础遥测(很多时候直接塞进 OLTP 系统),等表长到塞不下,就得上数仓了。
三类角色各玩各的,但行业正整体“左移”:工具越发友好,观测与数仓也慢慢被开发者收编。SRE 和数据岗并非故意让贤,只是数据库本身越来越能打,创业团队能撑更久再招专家。
三大开放数据标准
围绕以上三大场景,正冒出三套满足同样开源三信条的开放标准:
- OLTP: PostgreSQL
- 遥测: Open Telemetry
- OLAP: Iceberg
后两者更像“标准”而非“工具”,类似 HTML 与浏览器:大家约好格式,其他工具要么跟进要么淘汰。
标准往往草根起家,商业公司则陷入经典的 颠覆式创新 两难:
- 不跟?潮流跑了,错过增长趋势。
- 跟了?自家产品锁定度变低。
对开发者而言,这简直不能更香了 —— 我们坚信:可迁移性会逼着厂商拼体验。
下面逐一展开深入探讨。
Postgres:开放式 OLTP 标准
Postgres 虽是一款数据库,却已成 “标准接口”。
几乎所有新数据库都宣称“兼容 Postgres wire 协议”。
因为谁也管不了 Postgres,各大云厂商要么主动,要么被用户倒逼着上架 Postgres —— 连 Oracle Cloud 都供着。
体验差?一句 pg_dump
走人。Postgres 用 PostgreSQL License —— 功能上和 MIT 相当。
OTel:开放式遥测标准
“open telemetry” 的名字是字面含义:开放遥测。OTel 仍年轻且颇为复杂,但契合开源三信条:Apache 2.0,厂商中立。 正如云厂商拥抱 Postgres,主流观测平台也在集体投 OTel,包括 Datadog、Honeycomb、Grafana Labs 与 Elastic。 想自托管?可选 SigNoz、OpenObserve,再不济用官方 OTel 工具集。
Iceberg:开放式 OLAP 标准
开放表格式 算是新赛道:大家约定目录+元数据格式,任何计算引擎都能查询。 虽有 DeltaLake、Hudi 等对手,但目前 Iceberg 已然领跑。
各大数仓陆续“投靠” Iceberg:包括 Databricks、Snowflake 和 ClickHouse。 最关键的商业推手是 AWS —— 2024 年底官宣 S3 Tables,在 S3 上提供开箱即用的 Iceberg。
S3:终极数据基础设施
对象存储很便宜,已成三大标准的基石。今天凡是数据工具,不是原生 S3 就是兼容 S3。
AWS S3 团队连环上新,把 “S3 当数据库” 的幻想推向现实。诸如 Conditional Writes 和 S3 Express —— 速度比普通 S3 快 10 倍,最近还 逆天降价 85%。
不同场景对 S3 的姿势略有差异:
- OLTP:性能要命,S3 与 NVMe 永远隔着物理网线。因此重点是 Zero ETL & 分层存储:冷热数据自由搬迁。Postgres 现有多种读 Iceberg 的方式,如 pg_mooncake、pg_duckdb 及 Iceberg FDW。
- 遥测 / 数仓:关键字是“基数”。S3 越便宜,大家越把海量数据往里倒,催生“存算分离”的架构。于是出现一堆以计算层自居的嵌入式数据库:如 DuckDB(OLAP)、SQLite 的云后端存储、turbopuffer(向量)、SlateDB(KV)、Tonbo(Arrow)。它们既可嵌入应用,也能单飞。
Supabase 的数据蓝图
大家知道 Supabase 是 Postgres 服务商,我们花了 5 年打造让开发者舒爽的数据库平台,这仍是主航道。
不同的是,我们不止做 Postgres(虽然梗图挺火)。我们还提供 Supabase Storage,一套兼容 S3 的对象存储。未来,Supabase 聚焦的不是“一个数据库”,而是“所有数据”:
- 给我们维护的所有开源工具加上 OTel。
- 在 Supabase Storage 引入 Iceberg。
- 在 Supabase ETL 里打通 Postgres ↔ Iceberg 零 ETL。
- 通过扩展和 FDW,让 Postgres 能读能写 Iceberg。
接下来,我们押注三大开放数据标准:Postgres、OTel、Iceberg。敬请期待。
老冯点评
Supabase 是我最欣赏的数据库创业公司,他们的创始人认知水平非常在线。 例如在三年前 OpenAI 插件带火向量数据库赛道之前,Supabase 就已经发掘出 pgvector 进行 RAG 的玩法了。
YC S20 的项目走过五年发展到今天,已经是估值 2B 的独角兽了。目前 YC 80% 的初创公司都在用 Supabase 起步。 目前有小道消息称 OpenAI 即将收购 Supabase,如果是真的,那他们也算功德圆满,实至名归。
关于 Postgres
老冯非常认同 Paul 的观点,Postgres 已经成为 OLTP 世界的事实标准。 但至少在当下,还有几件事是 PostgreSQL “不擅长” (不是做不到)的:
- 遥测
- 海量分析
- 对象存储
所以如果你想要提供一个真正 “完全覆盖” 的数据基础设施,那么光有 PostgreSQL 是不行的。
我的意思是,你可以使用 TimescaleDB 扩展存储遥测数据,但体验与表现是比不上 Prometheus,VictoriaMetrics 的等专用 APM 组件的。 你确实可以用原生 PG,TimescaleDB,Citus,以及好几个 DuckDB 缝合扩展做数仓 —— 尽管我认为 DuckDB PG 缝合有潜力解决这个问题,但至少在当下,当数据量超过几十个 TB 时,专用数仓的性能依然还是压着 PG 打的。 有一些 “邪路” 可以将 PG 作为文件系统,例如 JuiceFS,但这仅适用于小规模的数据存储(也许几十GB?),海量 PB 级对象存储依然是原生 PG 所望尘莫及的。
至于其他的细分领域,比如向量数据库,文档数据库,地理空间数据库,时序数据库,消息队列,全文检索引擎,乃至是图数据库,PostgreSQL 都已经 “足够好” 了。 留给其他产品的只剩下一个极端场景专用组件的 Niche,不会再有其他这种体量的玩家出现了。
因此,在我做 Pigsty 的时候,也是用相同的思路构建的,以 PostgreSQL 为核心,以可观测性作为这个发行版的基石(Postgres in Grafana Style:这是最初的缩写),以同心圆的方式对外摊大饼。 用 MinIO 补足对象存储,用 DuckDB / Greenplum 补足数仓分析能力,最后用数量惊人的扩展插件来覆盖其他细分领域。
关于开源
Paul 说关于开源的三点精髓,第三点他领悟的是最慢的:
有没有商业中立、无厂商绑架;更妙的是,有 多家 公司背书而非一家独大。
其实我非常理解 Paul 的感受,在前两年,Supabase 的想法可能是 —— “我要占领开源道德高地,但是也要用 PG 扩展构建自己的商业壁垒。”
虽然 Supabase 提供了 Docker Compose 自建模板,但那个数据库容器镜像充其量就是个玩具,而且里面包含着隐藏的壁垒。 主要是他们自己用 Rust 写了几个扩展插件,这几个扩展插件虽然是开源的,但打包构建的知识并没有在社区普及 —— 你无法指望让用户自己去编译这些东西。
老冯就干了件 “缺德” 或者说 “有德” 的事(取决于厂家还是用户视角),把他们的扩展插件全都编译打包成了 10 大 Linux 主流系统下的 RPM/DEB 包, 这样你就可以真的在自己的 PostgreSQL 上自建 Supabase 了。我们还提供了一个模板,可以在一台裸服务器上自建 Supabase,目前是 Supabase 官方推荐的三个三方教程之一。
Supabase 还在想其他方法构建壁垒,例如他们去年收购了 OrioleDB,一个云原生,无膨胀的 PostgreSQL 存储引擎扩展(需要Patch内核)。 还没等正式 GA 上线,老冯就也已经打好了 OrioleDB 的 RPM/DEB 包,供用户自建使用了。
我估计 Paul 的心情是复杂的,一方面他想要将用户锁定在 Supabase 云服务上,看到别人真的用开源来拆台,心里肯定不爽。 但另一方面正是这些三方社区厂商的努力,反而让 Supabase 开枝散叶,不是一个 “只有我提供” 的东西,有了开源的醍醐味。 所以最后也释然了,坦然接受了这种现状。
但这件事也对老冯有所触动,我也开始思考,Pigsty 作为一个开源项目,是否也有类似的 “开源三信条”?
老实说,老冯很怀念全职创业前的那种状态,完全不考虑商业化,为了兴趣,热情,公益而开源,所以使用的是 Apache 2.0 协议。 后来因为拿投资人钱要有一个交代,所以把协议修改为更严格的 AGPLv3 ,目标是为了阻止云厂商与同行白嫖。 但既然现在我又成了数据库个体户,其实也是可以回到 Supabase 的这种状态 —— 用就用吧,反正我也不指望靠这个赚钱。
数据库老司机:文章导航
国产信创篇
- 2025-02-28 国产数据库里有能打的吗?
- 2024-11-18 这么吹国产数据库,听的尴尬癌都要犯了
- 2024-10-25 开源皇帝Linus清洗整风
- 2024-10-01 第二批数据库国测名单:国产化来了怎么办?
- 2024-07-15 机场出租车恶性循环与国产数据库怪圈
- 2024-04-25 国产数据库到底能不能打?
- 2024-01-11 国产数据库是大炼钢铁吗?
- 2024-01-08 中国对PostgreSQL的贡献约等于零吗?
- 2023-11-02 数据库真被卡脖子了吗?
- 2023-10-09 EL系操作系统发行版哪家强?
- 2023-08-31 基础软件到底需要什么样的自主可控?
行业洞察篇
- 2025-05-27 开放数据标准:Postgres,OTel,与Iceberg Paul
- 2025-05-23 小数据的失落十年:分布式分析的错付
- 2025-04-27 SaaS已死?AI时代,软件从数据库开始
- 2025-04-28 分布式数据库是伪需求
- 2025-04-14 Claude Code泄密:MCP 爆火的隐藏真相
- 2025-03-13 数据库火星撞地球:当PG爱上DuckDB
- 2025-03-10 HTAP数据库,一场无人鼓掌的演出
- 2024-12-03 七周七数据库 @ 2025
- 2024-11-21 面向未来数据库的现代硬件
- 2024-11-17 20刀好兄弟PolarDB:论数据库该卖什么价?
- 2024-08-13 谁整合好DuckDB,谁赢得OLAP数据库世界
- 2023-11-22 向量数据库凉了吗?
- 2023-11-17 重新拿回计算机硬件的红利
- 2023-04-17 分布式数据库是伪需求吗?
- 2023-03-29 数据库需求层次金字塔
DBA/RDS篇
- 2025-05-18 不缺好数据库内核,缺能用好数据库的DBA
- 2025-05-09 这次数据库真爆炸了,但摇人也没用了
- 2025-04-28 数据库爆炸了怎么摇人?
- 2024-09-07 先优化碳基BIO核,再优化硅基CPU核
- 2023-12-05 数据库应该放入K8S里吗?
- 2023-12-04 把数据库放入Docker是一个好主意吗?
- 2023-01-30 云数据库是不是智商税
- 2022-05-16 云RDS:从删库到跑路
- 2024-02-02 DBA会被云淘汰吗?
- 2023-03-01 驳《再论为什么你不应该招DBA》
- 2023-01-31 你怎么还在招聘DBA? 马工
- 2022-05-10 DBA还是一份好工作吗?
PG生态篇
- 2025-05-16 PGCon.dev闪电演讲,硬控PG大佬5分钟
- 2025-04-09 PG扩展峰会日程出炉,蒙特利尔见
- 2025-04-06 OrioleDB 奥利奥数据库来了!
- 2025-04-03 带有MySQL兼容的PG内核现已加入Pigsty
- 2025-03-01 PostgreSQL取得对MySQL的压倒性优势
- 2025-01-01 Andy Pavlo: 2024年度数据库回顾
- 2024-12-18 PostgreSQL 2024 社区现状调查报告
- 2024-07-25 StackOverflow 2024调研:PostgreSQL已经超神了
- 2024-03-24 PostgreSQL会修改开源许可证吗?
- 2024-05-16 为什么PostgreSQL是未来数据的基石?
- 2024-03-16 PostgreSQL is eating the database world
- 2024-03-04 PostgreSQL正在吞噬数据库世界
- 2024-02-19 技术极简主义:一切皆用Postgres
- 2024-01-03 2023年度数据库:PostgreSQL (DB-Engine)
- 2022-08-22 PostgreSQL 到底有多强?
- 2022-07-12 为什么PostgreSQL是最成功的数据库?
- 2022-06-24 StackOverflow 2022数据库年度调查
- 2021-05-08 为什么说PostgreSQL前途无量?
最佳实践篇
- 2025-05-19 OpenAI:将PostgreSQL伸缩至新阶段
- 2025-05-03 影视飓风达芬奇千万级数据库演化及实践
- 2025-03-21 PGFS:将数据库作为文件系统
- 2024-11-30 你为什么不用连接池?
- 2024-01-13 令人惊叹的PostgreSQL可伸缩性
PG发布篇
- 2024-11-15 不要更新!发布当日叫停:PG也躲不过大翻车
- 2024-11-14 PostgreSQL 12 过保,PG 17 上位
- 2024-11-02 PostgreSQL神功大成!最全扩展仓库
- 2024-09-27 PostgreSQL 17 发布:摊牌了,我不装了!
- 2024-09-05 PostgreSQL 17 RC1 发布!与近期PG新闻
- 2024-08-09 PostgreSQL小版本更新,17beta3,12将EOL
- 2024-05-24 PostgreSQL 17 Beta1 发布!牙膏管挤爆了!
- 2024-01-14 快速掌握PostgreSQL版本新特性
- 2024-01-05 展望PostgreSQL的2024 (Jonathan Katz)
创业融资篇
- 2025-05-12 数据库茶水间:OpenAI拟收购Supabase ?
- 2025-05-06 PG生态赢得资本市场青睐:Databricks收购Neon,Supabase融资两亿美元,微软财报点名PG
- 2024-09-26 PG系创业公司Supabase:$80M C轮融资
- 2024-07-31 ClickHouse收购PeerDB:这浓眉大眼的也要来搞 PG 了?
- 2024-02-18 PG生态新玩家ParadeDB
- 2023-10-08 FerretDB:假扮成MongoDB的PostgreSQL?
MySQL杀手篇
- 2024-11-05 PZ:MySQL还有机会赶上PostgreSQL的势头吗?
- 2024-07-12 MySQL新版恶性Bug,表太多就崩给你看!
- 2024-07-09 MySQL安魂九霄,PostgreSQL驶向云外
- 2024-06-26 用PG的开发者,年薪比MySQL多赚四成?
- 2024-06-20 Oracle最终还是杀死了MySQL!
- 2024-06-19 MySQL性能越来越差,Sakila将何去何从?
- 2023-12-30 MySQL的正确性为何如此拉垮?
- 2023-08-11 如何看待 MySQL vs PGSQL 直播闹剧
- 2023-08-09 驳《MySQL:这个星球最成功的数据库》
其他DB篇
- 2024-09-04 MongoDB没有未来:“好营销”救不了烂芒果
- 2024-09-03 《黑历史:Mongo》:现由PostgreSQL驱动
- 2024-09-02 PostgreSQL可以替换微软SQL Server吗?
- 2024-08-30 ElasticSearch又重新开源了???
- 2024-03-26 Redis不开源是“开源”之耻,更是公有云之耻
- 2023-10-08 FerretDB:假扮成MongoDB的PostgreSQL?
司机本人篇
- 2024-08-22 GOTC 2024 BTW采访冯若航:Pigsty作者,简化PG管理,推动PG开源社区的中国参与
- 2023-12-31 2023总结:三十而立
- 2023-09-08 冯若航:不想当段子手的技术狂,不是一位好的开源创始人
- 2022-07-07 90后,辞职创业,说要卷死云数据库
数据库老司机专栏
- 2025-05-27 开放数据标准:Postgres,OTel,与Iceberg
- 2025-05-23 小数据的失落十年:分布式分析的错付
- 2025-05-19 OpenAI:将PostgreSQL伸缩至新阶段
- 2025-05-18 不缺好数据库内核,缺能用好数据库的DBA
- 2025-05-16 PGCon.dev闪电演讲,硬控PG大佬5分钟
- 2025-05-12 数据库茶水间:OpenAI拟收购Supabase ?
- 2025-05-09 这次数据库真爆炸了,但摇人也没用了
- 2025-04-28 数据库爆炸了怎么摇人?
- 2025-05-06 PG生态赢得资本市场青睐:Databricks收购Neon,Supabase融资两亿美元,微软财报点名PG
- 2025-05-03 影视飓风达芬奇千万级数据库演化及实践
- 2025-04-27 SaaS已死?AI时代,软件从数据库开始
- 2025-04-28 分布式数据库是伪需求
- 2025-04-19 兼容Oracle的开源 PostgreSQL?
- 2025-04-17 2025年:MySQL vs PostgreSQL
- 2025-04-16 PG被黑慢MySQL 360倍,这次我真忍不了
- 2025-04-14 Claude Code泄密:MCP 爆火的隐藏真相
- 2025-04-09 PG扩展峰会日程出炉,蒙特利尔见
- 2025-04-06 OrioleDB 奥利奥数据库来了!
- 2025-04-03 带有MySQL兼容的PG内核现已加入Pigsty
- 2025-03-21 PGFS:将数据库作为文件系统
- 2025-03-13 数据库火星撞地球:当PG爱上DuckDB
- 2025-03-10 HTAP数据库,一场无人鼓掌的演出
- 2025-03-06 阿里云rds_duckdb:致敬还是抄袭?
- 2025-03-01 PostgreSQL取得对MySQL的压倒性优势
- 2025-02-28 国产数据库里有能打的吗?
- 2025-02-27 对比Oracle与PostgreSQL事务系统
- 2025-02-11 如何在数据库中直接检索PDF
- 2025-03-12 Wiz: DeepSeek数据库暴露
- 2025-01-24 PostgreSQL 生态前沿进展
- 2025-01-22 数据库即架构
- 2025-01-16 网传江西教育厅高考查分网站删库跑路
- 2025-01-14 PostgreSQL荣获2024年度数据库(5冠王)
- 2025-01-11 PII数据安全合规与PG Anonymizer最佳实践
- 2025-01-07 第七届PG生态大会:一些感想
- 2025-01-01 Andy Pavlo: 2024年度数据库回顾
- 2024-12-31 Pigsty@2024:今年没啥财运,但事儿整的还不赖
- 2024-12-23 小猪骑大象:PG内核与扩展包管理神器
- 2024-12-18 PostgreSQL 2024 社区现状调查报告
- 2024-12-03 七周七数据库 @ 2025
- 2024-11-30 你为什么不用连接池?
- 2024-11-26 创业出海神器 Supabase 自建指南
- 2024-11-21 面向未来数据库的现代硬件
- 2024-11-18 这么吹国产数据库,听的尴尬癌都要犯了
- 2024-11-17 20刀好兄弟PolarDB:论数据库该卖什么价?
- 2024-11-15 不要更新!发布当日叫停:PG也躲不过大翻车
- 2024-11-14 PostgreSQL 12 过保,PG 17 上位
- 2024-11-05 PZ:MySQL还有机会赶上PostgreSQL的势头吗?
- 2024-11-02 PostgreSQL神功大成!最全扩展仓库
- 2024-10-28 YC教父Paul Graham:写作者与非写作者
- 2024-10-25 开源皇帝Linus清洗整风
- 2024-10-01 第二批数据库国测名单:国产化来了怎么办?
- 2024-09-27 PostgreSQL 17 发布:摊牌了,我不装了!
- 2024-09-26 PG系创业公司Supabase:$80M C轮融资
- 2024-09-07 先优化碳基BIO核,再优化硅基CPU核
- 2024-09-05 PostgreSQL 17 RC1 发布!与近期PG新闻
- 2024-09-04 MongoDB没有未来:“好营销”救不了烂芒果
- 2024-09-03 《黑历史:Mongo》:现由PostgreSQL驱动
- 2024-09-02 PostgreSQL可以替换微软SQL Server吗?
- 2024-08-30 ElasticSearch又重新开源了???
- 2024-08-22 GOTC 2024 BTW采访冯若航:Pigsty作者,简化PG管理,推动PG开源社区的中国参与
- 2024-08-13 谁整合好DuckDB,谁赢得OLAP数据库世界
- 2024-08-09 PostgreSQL小版本更新,17beta3,12将EOL
- 2024-08-06 PG隆中对,一个PG三个核,一个好汉三百个帮
- 2024-08-03 最近在憋大招,数据库全能王真的要来了
- 2024-07-31 ClickHouse收购PeerDB:这浓眉大眼的也要来搞 PG 了?
- 2024-07-25 StackOverflow 2024调研:PostgreSQL已经超神了
- 2024-07-15 机场出租车恶性循环与国产数据库怪圈
- 2024-07-12 MySQL新版恶性Bug,表太多就崩给你看!
- 2024-07-09 MySQL安魂九霄,PostgreSQL驶向云外
- 2024-06-28 CentOS 7过保了,换什么OS发行版更好?
- 2024-06-26 用PG的开发者,年薪比MySQL多赚四成?
- 2024-06-20 Oracle最终还是杀死了MySQL!
- 2024-06-19 MySQL性能越来越差,Sakila将何去何从?
- 2024-06-18 让PG停摆一周的大会:PGCon.Dev参会记
- 2024-05-29 PGCon.Dev 2024 温哥华扩展生态峰会小记
- 2024-05-24 PostgreSQL 17 Beta1 发布!牙膏管挤爆了!
- 2024-05-16 为什么PostgreSQL是未来数据的基石?
- 2024-04-25 国产数据库到底能不能打?
- 2024-03-28 PostgreSQL 主要贡献者 Simon Riggs 因坠机去世
- 2024-03-26 Redis不开源是“开源”之耻,更是公有云之耻
- 2024-03-24 PostgreSQL会修改开源许可证吗?
- 2024-03-16 PostgreSQL is eating the database world
- 2024-03-14 RDS阉掉了PostgreSQL的灵魂
- 2024-03-04 PostgreSQL正在吞噬数据库世界
- 2024-02-19 技术极简主义:一切皆用Postgres
- 2024-02-18 PG生态新玩家ParadeDB
- 2024-02-02 DBA会被云淘汰吗?
- 2024-01-14 快速掌握PostgreSQL版本新特性
- 2024-01-13 令人惊叹的PostgreSQL可伸缩性
- 2024-01-11 国产数据库是大炼钢铁吗?
- 2024-01-08 中国对PostgreSQL的贡献约等于零吗?
- 2024-01-05 展望PostgreSQL的2024 (Jonathan Katz)
- 2024-01-03 2023年度数据库:PostgreSQL (DB-Engine)
- 2023-12-31 2023总结:三十而立
- 2023-12-30 MySQL的正确性为何如此拉垮?
- 2023-12-05 数据库应该放入K8S里吗?
- 2023-12-04 把数据库放入Docker是一个好主意吗?
- 2023-11-22 向量数据库凉了吗?
- 2023-11-17 重新拿回计算机硬件的红利
- 2023-11-02 数据库真被卡脖子了吗?
- 2023-10-26 PG查询优化:观宏之道
- 2023-10-09 EL系操作系统发行版哪家强?
- 2023-10-08 FerretDB:假扮成MongoDB的PostgreSQL?
- 2023-09-27 如何用 pg_filedump 抢救数据?
- 2023-09-26 PGSQL x Pigsty: 数据库全能王来了
- 2023-09-10 PG先写脏页还是先写WAL?
- 2023-09-08 冯若航:不想当段子手的技术狂,不是一位好的开源创始人
- 2023-08-31 基础软件到底需要什么样的自主可控?
- 2023-08-11 如何看待 MySQL vs PGSQL 直播闹剧
- 2023-08-09 驳《MySQL:这个星球最成功的数据库》
- 2023-08-06 向量是新的JSON
- 2023-06-27 ISD数据集:分析全球120年气候变化
- 2023-05-10 AI大模型与向量数据库 PGVECTOR
- 2023-05-09 技术反思录:正本清源 之 序章
- 2023-05-07 微服务是不是个蠢主意?
- 2023-04-17 分布式数据库是伪需求吗?
- 2023-04-10 AI 会有自我意识吗?
- 2023-03-29 数据库需求层次金字塔
- 2023-03-01 驳《再论为什么你不应该招DBA》
- 2023-01-31 云数据库是不是杀猪盘
- 2023-01-31 你怎么还在招聘DBA? 马工
- 2023-01-30 云数据库是不是智商税
- 2022-08-22 PostgreSQL 到底有多强?
- 2022-07-12 为什么PostgreSQL是最成功的数据库?
- 2022-07-07 90后,辞职创业,说要卷死云数据库
- 2022-06-24 StackOverflow 2022数据库年度调查
- 2022-05-16 云RDS:从删库到跑路
- 2022-05-10 DBA还是一份好工作吗?
- 2021-05-08 为什么说PostgreSQL前途无量?
小数据的失落十年:分布式分析的错付
如果 2012 年 DuckDB 问世,也许那场数据分析向分布式架构的大迁移根本就不会发生。通过在2012年的Macbook笔记本上运行 TPC-H 评测,我们发现数据分析确实在分布式架构上走了十年弯路。
作者: Hannes Mühleisen,发布于 2025 年 5 月 19 日,英文
译评:冯若航,数据库老司机,云数据库泥石流
太长不看:我们在一台 2012 年款 MacBook Pro 上对 DuckDB 进行了基准测试,想要弄清楚在过去十年里,我们是否在追逐分布式数据分析架构的过程中迷失了方向?
包括我们自己在内,很多人都反复提到过这一点:数据其实没那么大 。 而且硬件进步的速度已经超越了有用数据集规模的增长速度。我们甚至预测预测过 在不久的将来会出现“数据奇点” ——届时 99% 的有用数据集都能在单节点上轻松查询。最近的研究数据显示 , Amazon Redshift 和 Snowflake 上的查询中位扫描数据量仅约 100 MB,而 99.9 百分位点也不到 300 GB。由此看来,“奇点”也许比我们想象的更近。
但是我们开始好奇,这一趋势究竟是从什么时候开始的?像随处可见、通常只用来跑 Chrome 浏览器的 MacBook Pro 这样的个人电脑,是什么时候摇身一变成为了如今的数据处理大师?
让我们把目光投向 2012 年的 Retina MacBook Pro。许多人(包括我自己)当年购买这款电脑是为了它那块华丽的 “Retina”(视网膜) 显示屏 —— 销量以百万计。我当时虽没工作,但还是咬牙加钱把内存升级到了 16 GB。不过,这台机器上还有一个常被遗忘的革命性变化:它是第一款内置固态硬盘(SSD)并配备性能强劲的 4核 2.6 GHz Core i7 CPU 的 MacBook。重看一遍当年的 发布会视频 仍然颇为有趣 —— 他们 确实 也强调了这种 “全闪存架构” 的性能优势。
题外话:实际上早在 2008 年 MacBook Air 就已经是第一款可选配内置 SSD 的 MacBook,只可惜它没有 Pro 版那样强劲的 CPU 火力。
巧的是,我现在手头仍有这样一台笔记本放在 DuckDB Labs 办公室,我的孩子们平时来玩时,会用它来刷 Youtube 看动画片。那么,这台老古董还跑得动现代版本的 DuckDB 吗?它的性能和现代的 MacBook 相比如何? 我们可以在 2012 年就迎来当今的数据革命吗?让我们一探究竟!
软件
首先来说说操作系统。为了让这次跨年代的对比更公平,我们特地把 Retina 本的系统 降级 到 OS X 10.8.5 “Mountain Lion”——这正是该笔记本上市几周后的 2012 年 7 月发布的操作系统版本。虽然这台 Retina 笔记本实际上可以运行 10.15 (Catalina),但我们觉得要做真正的 2012 年对比,就该使用那个年代的操作系统。下面这张截图展示了当年的系统界面,我们这些上了年纪的人看了不禁有点感慨。
再来说 DuckDB 本身。在 DuckDB 团队,我们对可移植性和依赖有着近乎宗教般的坚持(更准确的说是 “零依赖”)。正因如此,要让 DuckDB 在古老的 Mountain Lion 上跑起来几乎不费吹灰之力:DuckDB 的预编译二进制默认兼容到 OS X 11.0 (Big Sur),我们只需调整一个编译标志重新编译,就使 DuckDB 1.2.2 顺利运行在了 Mountain Lion 上。我们本想尝试用 2012 年的老旧编译器来构建 DuckDB,无奈 C++11 在当年还太新,编译器对它的支持根本跟不上。话虽如此,生成的二进制运行良好——实际上,只要费些功夫绕过编译器的几个 bug,当年也是可以把它编译出来的。或者,我们大可以像 其他人那样 干脆直接手写汇编。
基准测试
但我们感兴趣的可不是什么 CPU 综合跑分,我们关注的是 SQL综合跑分!为了检验这台老机器在严肃的数据处理任务下的表现,我们使用了如今已经有些老掉牙但依然常用的 TPC-H 基准测试,规模因子设为 1000。这意味着其中两张主要表 lineitem
和 orders
分别包含约 60 亿和 15 亿行数据。将数据生成 DuckDB 数据库文件后,大约有 265 GB 大小。
根据 TPC 官网的审计结果 ,可以看出在单机上跑如此规模的基准测试,似乎需要价值数十万美元的硬件设备。
我们将 22 个基准查询各跑了五遍,取中位数运行时间来降噪。(由于内存只有 16 GB,而数据库大小达到 256 GB,缓冲区几乎无法缓存多少输入数据,因此这些严格来说都算不上大家口中的 “热运行“。)
下面列出了每个查询的耗时(单位:秒):
查询 | 耗时 |
---|---|
1 | 142.2 |
2 | 23.2 |
3 | 262.7 |
4 | 167.5 |
5 | 185.9 |
6 | 127.7 |
7 | 278.3 |
8 | 248.4 |
9 | 675.0 |
10 | 1266.1 |
11 | 33.4 |
12 | 161.7 |
13 | 384.7 |
14 | 215.9 |
15 | 197.6 |
16 | 100.7 |
17 | 243.7 |
18 | 2076.1 |
19 | 283.9 |
20 | 200.1 |
21 | 1011.9 |
22 | 57.7 |
但是,这些冰冷的数字实际上意味着什么呢?令人窃喜的是,这台老电脑居然真的用 DuckDB 跑完了所有基准查询!如果仔细看看那些耗时,每个查询大致在几分钟到半小时之间。这种数据量下跑分析型查询,这样的等待时间一点也不离谱。老天,要是在 2012 年,你光等 Hadoop YARN 去调度你的作业就得更久,最后很可能它只会朝你吐出一堆错误堆栈。
2023 年的改进
那么这些结果与一台当代 MacBook 比又如何呢?作为比较,我们使用了一台现代 ARM 架构 M3 Max MacBook Pro(碰巧就在同一张桌子上)。这两台 MacBook 之间代表了超过十年的硬件发展差距。
从 GeekBench 5 基准测试分数 来看,全核性能提升了约 7 倍,单核性能提升约 3 倍。当然,RAM 和 SSD 速度的差距也非常明显。有趣的是,屏幕尺寸和分辨率几乎没有变化。
下面将两台机器的结果并排列出:
查询 | 旧耗时 | 新耗时 | 加速比 |
---|---|---|---|
1 | 142.2 | 19.6 | 7.26 |
2 | 23.2 | 2.0 | 11.60 |
3 | 262.7 | 21.8 | 12.05 |
4 | 167.5 | 11.1 | 15.09 |
5 | 185.9 | 15.5 | 11.99 |
6 | 127.7 | 6.6 | 19.35 |
7 | 278.3 | 14.9 | 18.68 |
8 | 248.4 | 14.5 | 17.13 |
9 | 675.0 | 33.3 | 20.27 |
10 | 1266.1 | 23.6 | 53.65 |
11 | 33.4 | 2.2 | 15.18 |
12 | 161.7 | 10.1 | 16.01 |
13 | 384.7 | 24.4 | 15.77 |
14 | 215.9 | 9.2 | 23.47 |
15 | 197.6 | 8.2 | 24.10 |
16 | 100.7 | 4.1 | 24.56 |
17 | 243.7 | 15.3 | 15.93 |
18 | 2076.1 | 47.6 | 43.62 |
19 | 283.9 | 23.1 | 12.29 |
20 | 200.1 | 10.9 | 18.36 |
21 | 1011.9 | 47.8 | 21.17 |
22 | 57.7 | 4.3 | 13.42 |
显而易见,我们获得了可观的加速效果,最低约 7 倍,最高超过 50 倍。运行时间的几何平均数 从 218 秒降低到了 12 秒,整体提升了约 20 倍。
可复现性
所有二进制文件、脚本、查询和结果都已发布在 GitHub 上供大家查阅。我们还提供了 TPC-H SF1000 数据库文件 下载,这样你就不用自己生成。不过请注意,文件非常大。
讨论
我们看到,这台已有十年历史的 Retina MacBook Pro 成功完成了复杂的分析型基准测试,而更新的笔记本则显著缩短了运行时间。但对于用户而言,那些绝对的加速倍数其实意义不大—— 这里的差别纯粹是 量变 而非什么 质变。
从用户的角度来看,更重要的是这些查询能够在相当合理的时间内完成,而不是纠结于到底用了 10 秒还是 100 秒。用这两台笔记本,我们几乎可以解决同样规模的数据问题,只不过旧机器需要我们多等待一会儿而已。尤其是 DuckDB 能够处理超出内存大小的数据集——必要时可以将查询中间结果溢出到磁盘,这让单机处理大数据成为可能。
更有意思的是,早在 2012 年,像 DuckDB 这样单机 SQL 引擎完全有能力在可接受的时间内跑完对一个包含 60 亿行数据的数据库的复杂分析查询——而这一次我们甚至不需要 把机器泡在干冰里。
历史不乏各种 **“假如当初……” **的假设。如果 2012 年就出现了 DuckDB,会发生什么呢?主要的条件那时其实都已具备—— 矢量化查询处理技术早在2005年就已经问世。如今回头再看那场数据分析向分布式架构的大迁移显得有些傻气,如果那时候就有 DuckDB,也许那场运动根本不会发生。
我们这次使用的基准数据集规模,非常接近 2024 年分析查询输入数据量的 99.9 百分位点。而 Retina MacBook Pro 虽然在 2012 年属于高端机型,但到了 2014 年,许多厂商提供的笔记本电脑也都配备了内置 SSD,且更大容量的内存逐渐变得司空见惯。
所以,没错,我们的确整整浪费了十年。
老冯评论
老冯一直认为在当代硬件条件下,分布式数据库是一个伪需求。在《分布式数据库是伪需求吗?》那篇文章中,我比较保守的将 “OLAP 分析” 从中排除 —— 因为我确实在阿里处理过单机没法搞的数据量级 —— 每天 70 TB 的全网 PV 日志。
但我必须承认,那种情况真的属于极端特例,实际上绝大多数的分析场景并不会有那么多的数据。毕竟根据各种数据泄漏案例来看,全国人口数据,GA 全量结构数据,也就两百多个 GB 而已。许多所谓的“大数据场景” 其实并没有那么多数据,每次查询的时候实际读取处理的数据就更少了。(请看《DuckDB宣言:大数据已死》)
DuckDB 的这篇文章无疑撕开了整个数据分析,分布式数据库与大数据行业的遮羞布。是的,早在十年前,像几百 GB 的全量分析,就已经可以在一台 Macbook 笔记本上进行了!我们确实整整浪费了十年的时间,在错误的道路上蹉跎了岁月。
我的意思是,TPC-H 1000 仓的分析,可以在一台普通笔记本上用 6 分钟(370s)跑完,在十年前的笔记本上用 6 小时(8344s)跑出来,这是一个惊人的成绩。如果我们把现在的各路分布式数据库,OLAP,HTAP,MPP 各种 P 拉出来对比一下的话,就不难发现这是多么惊人的一个成绩了。
例如国产数据库标杆 TiDB 主打 HTAP 概念,并提供了 TiFlash 用于分析加速。然而其官网公布的 TPC-H 评测结果,用 92C 478G 处理 50 仓的数据,耗时几乎和一台 10C64GB 笔记本处理 300 仓的接近,在相同的时间里用十倍的资源却只处理了 1/6 的数据。这不禁让人怀疑,在这里用分布式真的有意义吗?
有人说 OLTP 也许会有超出单机吞吐的情况必须要用到分布式数据库,可是拥有五亿活跃用户的 OpenAI 竟然只用了一套 1主40从的 PostgreSQL 集群,在未分片的情况下直接支撑起了整个业务(《OpenAI:将PostgreSQL伸缩至新阶段》)。如果 OpenAI 能用集中式架构做到这一点,我相信你的业务也一定可以。
DuckDB 的例子进一步证明了在当代,分布式数据库已经成为了伪需求 —— 不仅仅是 OLTP,甚至是 OLAP。实际上如果我们关注 DB-Engine 上的热度就不难发现,分布式数据库作为一个 Niche(NewSQL),甚至都还没有像产生像 NoSQL 这样的影响力,就已经过气了。而我相信,重新融合 OLTP 和 OLAP 的新物种,将由 PostgreSQL 和 DuckDB 杂交而出。
OpenAI:将PostgreSQL伸缩至新阶段
在 PGConf.Dev 2025 全球 PG 开发者大会上, 来自 OpenAI 的 Bohan Zhang 分享了 OpenAI 在 PostgreSQL 上的最佳实践, 让我们得以一窥最牛独角兽内部的数据库使用情况。
“在 OpenAI,我们在使用一写多读的未分片架构,证明了 PostgreSQL 在海量读负载下也可以伸缩自如”
—— PGConf.Dev 2025 Bohan Zhang from OpenAI
Bohan Zhang 是 OpenAI Infra 组成员,师从 CMU 网红教授 Andy Pavlo ,并与其共同创办了 OtterTune 。本文为 Bohan 在大会上的演讲。 中文翻译/点评 by 冯若航:Pigsty 作者,PostgreSQL 老司机
Hacker News Discussion: OpenAI: Scaling Postgres to the Next Level
背景
PostgreSQL 是 OpenAI 绝大多数关键系统的核心支撑数据库,如果 Postgres 挂了,OpenAI 的很多关键服务就直接宕掉了 —— 而这是有不少先例的,PostgreSQL 相关的故障曾经在过去导致多次 ChatGPT 的故障。
OpenAI 使用 Azure 上的托管 PostgreSQL 数据库,没有使用分片与Sharding, 而是一个主库 + 四十多个从库的经典 PostgreSQL 主从复制架构。 对于像 OpenAI 这样拥有五亿活跃用户的服务而言,可伸缩性是一个重要的问题。
挑战
在 OpenAI 一主多从的 PostgreSQL 架构中,PG 的的读伸缩性表现极好,“写请求” 成为了一个主要的瓶颈。 OpenAI 已经在这上面进行了许多优化,例如将能移走的写负载尽可能移走,避免把新业务放进主数据库中。
PostgreSQL 的 MVCC 设计存在一些已知的问题,例如表膨胀与索引膨胀,自动垃圾回收调优较为复杂,每次写入都会产生一个完整新版本, 索引访问也可能需要额外的回表可见性检查。这些设计会带来一些 “扩容读副本” 的挑战: 例如更多 WAL 通常会导致复制延迟变大,而且当从库数量疯狂增长时,网络带宽可能成为新的瓶颈。
措施
为了解决这些问题,我们进行了多个层面上的努力:
控制主库负载
第一项优化是抹平主库上的写尖峰,尽可能的减少主库上的负载,例如:
- 把能移走的写入统统移走
- 在应用层面尽可能避免不必要的写入
- 使用惰性写入来尽可能抹平写入毛刺
- 回填数据的时候控制频次
此外,OpenAI 还尽最大可能把读请求都卸载到从库上去,一些因为放在读写事务中,无法从主库上移除的读请求则要求尽可能高效。
查询优化
第二项是在查询层面进行优化。因为长事务会阻止垃圾回收并消耗资源,因此他们使用 timeout 配置来避免 Idle in Transaction 长事务,并设置会话,语句,客户端层面的超时。 同时,还把一些多路 JOIN 的查询(比如一次 Join 12 个表)给优化掉了。分享中还特别提到使用 ORM 容易导致低效的查询,应当慎用。
治理单点问题
主库是一个单点,如果挂了就没法写入了。与之对应,我们有许多只读从库,一个挂了应用还可以读其他的。 实际上许多关键请求是只读的,所以即使主库挂了,它们也可以继续从主库上读取。
此外,我们低优先级请求与高优先级请求也进行了区分,对于那些高优先级的请求,OpenAI 分配了专用的只读从库,避免它们被低优先级的请求影响
模式管理
第四项是只允许在此集群上进行轻量的模式变更。这意味着:
- 新建表,或者把新的负载丢上来是不允许的
- 可以新增或移除列(设置5秒超时),任何需要重写全表的操作都是不允许的。
- 可以创建/移除索引,但必须使用
CONTURRENTLY
。
另一个提及的问题是运行中持续出现的长查询(>1s)会一直阻塞模式变更,最终导致模式变更失败。解决这个问题的措施是让应用把这些慢查询优化掉或者移到只读从库上去。
结果
- 将 Azure 上的 PostgreSQL 伸缩至百万 QPS,支撑了 OpenAI 的关键服务
- 在不增加复制延迟的前提下新增了几十个从库(不到 50)
- 将只读从库部署至不同的地理区域并保持低延迟
- 过去9个月内只有一起与 PostgreSQL 有关的零级事故•仍然为未来增长保留了足够的空间
“在 OpenAI,我们在使用一写多读的未分片架构,证明了 PostgreSQL 在海量读负载下也可以伸缩自如”
故障案例
此外,OpenAI 还分享了几个问题案例研究,第一个案例是缓存故障导致的雪崩。
第二个故障比较有趣,是极高 CPU 使用率下触发了一个 BUG。导致即使 CPU 水位恢复,WALSender 也一直在自旋循环而不是干正事发送 WAL 日志给从库,从而导致复制延迟增大。
功能需求
最后,Bohan 也向 PostgreSQL 开发者社区提出了一些问题与特性建议:
第一个是关于禁用索引问题的,不用打索引会导致写放大与额外的维护开销,他们希望移除没用的索引,然而为了最小化风险, 他们希望有一个 “Disable” 索引的特性,并监控性能指标确保没问题后再真正移除索引。
第二个是关于可观测性的,目前的 pg_stat_statement
只提供每类查询的平均响应时间,
而没法直接获得 (p95, p99)延迟指标。他们希望拥有更多类似 histogram 与 percentile 延迟的指标。
第三项是关于模式变更的,他们希望 PostgreSQL 可以记录模式变更事件的历史,例如新增/移除列,以及其他 DDL操作。
第四个 Case 是关于监控视图语义的。他们发现了一条会话 State = Active,WaitEvent = ClientRead 持续了两个多小时。 也就是有一条链接 QueryStart 之后一直 Active 了很长时间,而这样的链接就没有办法被 idle in transaction 超时给杀掉,希望了解这是一个 Bug 吗,以及如何解决。
最后是关于 PostgreSQL 默认参数的优化建议,PostgreSQL 默认参数值过于保守了。 是否可以使用一些更好的默认值,或者使用启发式的设置规则?
老冯评论
尽管 PGConf.Dev 2025 主要关注的是开发,但也经常可以看到一些用户侧的 Use Case 分享。 比如这次 OpenAI 的 PostgreSQL 伸缩实践。其实这类主题对于内核开发者来说还是很有趣的, 因为很多内核开发者确实对极端场景下的 PostgreSQL 用例没有概念,而这类分享会很有帮助。
老冯从 2017 年底在探探管理着几十套 PostgreSQL 集群,算是国内互联网场景下最大最复杂的部署之一: 几十套 PG,250 万左右的 QPS。那时候我们最大的核心主库一主 33 从,一套集群承载了 40万左右的 QPS。 瓶颈也卡在了单库写入上,最后进行了分库分表,使用类 Instagram 的应用侧 Sharding 解决了这个问题。
可以说,OpenAI 在这次分享中遇到的问题,以及采取的解决手段,我都曾经遇到过。 当然不同的是当下的顶级硬件可要比八年前牛逼太多了,让 OpenAI 这样的创业公司可以用一套 PostgreSQL 集群,在不分片,不Sharding 的情况下直接服务整个业务。 这无疑为《分布式数据库是个伪需求》提供了又一记强有力的例证。
在聊天提问的时候,老冯了解到 OpenAI 使用的是 Azure 上的托管 PostgreSQL,使用最高可用规格的服务器硬件,从库数量达到 40+,包括一些异地副本, 这套巨无霸集群总的读写 QPS 为 100 万左右。监控使用 Datadog,业务从 Kubernetes 中通过业务侧的 pgbouncer 链接池化之后访问 RDS 集群。
因为是战略级甲方,Azure PostgreSQL Team 提供贴心服务。但显然,即使是使用了顶级的云数据库服务,也需要客户在应用/运维侧有足够的认知与水平 —— 即使有 OpenAI 这样的智力储备,也依然会在 PostgreSQL 的一些实践驾驶案例中翻车。
会议结束后晚上的 Social 环节,老冯和 Bohan 还有两位数据库 Founder 一起唠嗑唠到了凌晨,相谈甚欢。非公开的讨论十分精彩,不过老冯无法就此透露更多,哈哈。
老冯答疑
关于 Bohan 提出的几个问题与特性建议,老冯倒是可以在这里做一个解答。
其实大部分 OpenAI 想要的功能特性需求 PostgreSQL 生态中已经有了,只不过不一定在原生 PG 内核与云数据库环境中可用。
关于禁用索引
PostgreSQL 其实是有禁用索引的“功能”,只需要更新 pg_index
系统表中的 indisvalid
字段为 false
,
这个索引就不会被 Planner 使用,但仍然会在 DML 中被继续维护。从原理上讲这没什么毛病,因为并发创建索引就是利用这两个标记位(isready
, isvalid
)来实现的,并不算什么黑魔法。
但 OpenAI 无法使用这种方式,我可以理解这里的原因:这是一个未被文档记录的 “内部细节” 而非正式特性,但更重要的原因是云数据库通常不提供 Superuser 权限,所以没办法这样更新系统目录。
但回到最原始的需求 —— 害怕误删索引,这个问题有更简单的解决办法,直接从监控视图中确认索引在主从上都没有访问即可。你只要知道了很长时间都没人用这个索引,就可以放心删除它。
使用 Pigsty 监控系统 PGSQL TABLES 查阅在线切换索引的过程
-- 创建一个新索引
CREATE UNIQUE INDEX CONCURRENTLY pgbench_accounts_pkey2 ON pgbench_accounts USING BTREE(aid);
-- 标记原索引为无效(不使用),但继续维护,Planner 将自动使用其他索引替代
UPDATE pg_index SET indisvalid = false WHERE indexrelid = 'pgbench_accounts_pkey'::regclass;
关于可观测性
其实 pg_stat_statements
提供了均值与标准差,可以使用正态分布的性质来估算出分位点指标。
但这只能作为模糊的参考,而且需要定时重置计数器,否则全量历史统计值的效果会越来越差。
PGSS 在短期内可能并不会提供 P95, P99 RT 这样的百分位点指标,因为这会导致这个扩展所需的内存翻个几十倍 —— 对于现代服务器来说这倒也不算什么,但对于一些极端保守的场景就会有问题。我在 Unconference 上问了 PGSS 的维护者这个问题,短期内可能并不会发生。 我也问了 Pgbouncer 的维护者 Jelte 是否可能在链接池层面解决这个问题,短期内也不会有这样的特性出现。
然而这个问题其实也是有其他解法的,首先 pg_stat_monitor
这个扩展就明确提供了详细的分位点 RT 指标,可以解决这个问题,但也要考虑分位点指标采集对集群性能造成的影响。
通用,无侵入,且无数据库性能损耗的办法,是在应用层面 DAL 直接添加查询 RT 监控,但这需要应用端的配合与努力。
此外,使用 ebpf 旁路采集 RT 指标是一个很棒的想法,不过考虑到他们用的 Azure 托管 PostgreSQL,不会给服务器权限,所以这条路可能被堵死了。
关于模式变更记录
其实 PostgreSQL 的日志已经提供这个选项了,只要把 log_statement
设置为 ddl
(或更高级的 mod
, all
),所有 DDL 日志就会保留下来。扩展插件 pgaudit
也提供了类似的功能。
但我猜他们想要的不是这种 DDL 日志,而是类似提供一个可以通过 SQL 查询的系统视图。所以另一种选项是 CREATE EVENT TRIGGER
,
使用事件触发器直接在数据表中记录 DDL 事件即可。扩展 pg_ddl_historization
提供了更简便的记录方式,我也编译打包了这个扩展。
创建事件触发器也需要 superuser 权限,AWS RDS 有一些特殊处理可以使用这个功能,不过 Azure 上的 PostgreSQL 似乎就不支持了。
关于监控视图语义
在 OpenAI 的这个例子中,pg_stat_activity.state
= Active
意味着后端进程依然在同一条 SQL 语句的生命周期里,WaitEvent
= ClientWait
意味着进程在CPU上等客户端的数据过来。
两者同时出现,典型的例子就是 COPY FROM STDIN 空等,但也可能是 TCP 阻塞,或者卡在 BIND / EXECUTE 中间。所以也不好说就是 BUG,还是要看链接具体在做什么。
有人认为,等待 Client I/O ,这从 CPU 角度来看这不应该是 “空闲” (Idle) 状态吗?但 State 关注的是语句本身的执行状态,而不是 CPU 的忙闲与否。
State = Active 意味着 PostgreSQL 后端进程认为 “这条语句尚未结束”。行锁、buffer pin、快照、文件句柄等资源就被视为“正在使用”,这并不代表它正在 CPU 上运行,
当该进程在 CPU 上运行,在 For 循环中等待客户端数据的到来时,等待事件为 ClientRead
,而当它让出 CPU 在后台等待时,等待事件为 NULL
。
当然回到这个问题本身,其实是有别的解决办法的。例如在 Pigsty 中,当通过 HAProxy 访问 PostgreSQL 时, 我们会在 LB 层面为 Primary 服务设置一个 链接超时,默认为 24h ,更高标准的环境会更短,比如 1h。 那么就意味着超过1小时的链接就会被挂断。当然,这个也需要在应用侧的链接池相应配置最大生命周期,尽可能主动挂断而不是被挂断。 对于离线只读服务则可以不设置这个参数,来允许那种跑两三天的超长查询。这样就可以为这种 Active 但等待 I/O 的情况提供兜底。
但我也怀疑在 Azure PostgreSQL 是否提供了这种控制的可能性。
关于默认参数
PostgreSQL 的默认参数相当保守,例如 默认使用 128 MB 内存(最小可以设置 128 KB !) 从好的方面讲这让他的默认配置能在几乎所有环境中都能跑起来。从坏的方面讲我真的见过 1TB 物理内存使用 128 MB 默认配置运行的案例……(因为双缓冲,竟然还真跑了很久生产业务)。
但总体来说,我觉得默认参数保守点不是坏事,这个问题可以在更灵活的动态配置过程中解决。
RDS 和 Pigsty 都提供了足够好的 初始参数启发式配置规则,充分解决这个问题了。
但这个特性确实可以加入到 PG 命令行工具中,比如在 initdb
时自动检测 CPU/内存数量,磁盘大小与介质并相应设置优化的参数值。
自建 PostgreSQL ?
OpenAI 提出的几个问题,挑战其实并不是来自 PostgreSQL 本身,而是来自托管云服务的额外限制。 一种解决办法就是利用 Azure 或其他资源云的 IaaS 层,使用本地 NVMe SSD 实例存储自建 PostgreSQL 集群以绕开限制。
实际上,老冯的 Pigsty 就是为了解决类似规模下 PostgreSQL 挑战而给自己做的云数据库解决方案。 It scales well ,支撑起了探探 25K vCPU 的 PostgreSQL 集群与 2.5 M QPS。 包括上面这些问题,甚至是许多 OpenAI 还没有遇到的问题也都有了解决方案,并做到了 Pigsty 中,并开源免费,开箱即用。
如果 OpenAI 感兴趣,我当然乐意提供一些支持,不过我觉得狂飙增长的时候,折腾数据库 Infra 可能并非高优先级的事项。 好在,他们还是有着非常优秀的 PostgreSQL DBA,能够继续探索出这些道路来。
Etcd 坑了多少公司?
前几天 影视飓风分享的 Pigsty / PostgreSQL 高可用案例 里面踩的一个雷在 X 上引起网友热议。 “因为 etcd 未开启自动压实功能,且默认仅为 2GB 容量”,ayanamist 评论到:“我倒要看看 etcd 这个傻逼 2G 的设计可以坑多少公司”。
etcd 的 slogan 是:“一个分布式的、可靠的键-值存储,用于存放系统中最为关键的配置数据”。 目前最常见的场景是用于存储 K8S 的元数据。当然类似 Patroni 这样的 PostgreSQL 高可用方案也可能会用到 etcd 作为 DCS。
在 Ayanamist 这条推的评论下,可以看到许多人都踩过这个雷。大部分是 K8S 用户,也有在 PG 高可用场景上翻车的例子。
etcd 的缺陷
ETCD 有一个非常傻逼的设计,就是在默认配置下,写满 2GB 数据就挂了。
具体来说,etcd 每次写入都会创建一个新的版本,当这些数据/版本超过 2GB 之后,etcd 就会进入维护模式(挂了)。 想象一个没有启用 GC 垃圾回收的 Java JDK,就可以理解 etcd 有多坑了。
当然,其实是有参数配置项可以解决这个问题的,例如使用以下配置项,可以让 etcd 只保留最近 24 小时的版本,从而避免了存储空间的无限增长。
auto-compaction-retention: "24h"
但坑就坑在,这并不是一个默认配置,这个参数的默认值是 0
,也就是保留所有历史版本。
更坑的是,在 etcd “维护” 部分的文档里的语句很有误导性,例如在 Maintenance 部分是这么说的:
为了保持稳定性,etcd 集群需要定期维护。根据 etcd 应用的需求,这些维护通常可以自动化执行,且不会导致停机或显著性能下降。
而且在下面的 “Auto Compaction” 部分,一眼看过去,给人的感觉就是 auto compact 设置了很好的默认值嘛: 每小时垃圾回收一次,保留10个小时,这不是挺好的?应该不需要我操心了。
但如果你没去看那个 Configuration Options 参考文档,那完犊子了
最后,这个问题还不会在 etcd 开始服务时立即暴露,而是会在使用几个月之后突然爆雷。
PostgreSQL 的例子
其实在很早的时候(8.0, 2005 年前) PostgreSQL 也有这个问题。 PostgreSQL 使用与 etcd 类似的 MVCC 逻辑,每次写入也都是创建新版本/标记删除,所以会留下许多历史版本。 当垃圾版本积累的太多而没有清理时,数据库就炸了,而这个操作是需要管理员手工执行的,所以成为了 PG 广为诟病的一个问题。
不过后来 PostgreSQL 引入了 AutoVacuum 机制,也就是自动垃圾回收,会有守护进程不断扫描清理回收,免去了人工操作的烦恼。 因此在现代硬件上,默认参数下的 PostgreSQL 基本不会再因为这个问题而头疼了。
然而,并非所有数据库都像 PostgreSQL 这样 “靠谱贴心”,给用户设置了足够好的 “默认值”,可以开箱即用。etcd 就是一个非常典型的例子。
Pigsty 的例子
Pigsty 也在这个问题上翻过车。从 2023-02-28 发布的 v2.0.0 首次引入 etcd 作为 DCS 开始,到 2024-02-13 v2.6.0 修复 etcd 的这个问题, 整整一年的版本都受到 etcd 这个问题的影响。我们在文档的 漏洞缺陷 与 ETCD FAQ 中多次强调过这个问题。
在这里还是要特别提醒一下使用 Pigsty v2.0 ~ v2.5 的用户,请尽快更新升级一下 Etcd 的配置。
AI时代,软件从数据库开始
未来的软件形态是 Agent + 数据库。没有前后端中间商,Agent直接CRUD。数据库技能相当保值,而 PostgreSQL 会成为 Agent 时代的数据库。
SaaS已死?软件从数据库开始
AI行业近年大爆发,生成式模型席卷各个领域,仿佛不谈AI就落伍了。然而软件的世界终究是从数据库开始的。微软 CEO 纳德拉在公开访谈中表示:在 Agent 时代,SaaS is Dead,而未来的软件形式将是 Agent + Database 。
“…I think, the notion that SaaS business applications exist, that’s probably where they’ll all collapse, right in the Agent era…”
— Satya Nadella https://medium.com/@iamdavidchan/did-satya-nadelle-really-say-saas-is-dead-fa064f3d65d1
“对于 SaaS 应用,也就是各类企业软件,这确实是个至关重要的问题。进入 Agent 时代之后,传统‘业务应用’这一层大概率会被压缩乃至消解。 为什么?本质上,这些应用就是一张 CRUD 数据库外加一堆业务逻辑。 而这些业务逻辑今后都会迁移到智能 Agent 中 —— 这些 Agent 能跨多库 CRUD,不会在意底层是什么后台,想改哪张表就改哪张表。 逻辑一旦全部上移到 AI 层,人们自然就会开始替掉后端,对吧?”
这个观点一出,业界一片哗然:难道几十年来我们习以为常的软件中间层要被挤掉,只剩智能 Agent 直接对话数据库了吗?如果你使用过一些 Vibe Coding,或者 MCP 桌面端,就能理解纳德拉想说什么。 你可以在 Claude / Cursor 中直接通过对话来访问像 PostgreSQL 这样的数据库。让 AI 根据你的问题,生成查询,处理并整合结果。在一些比较简单分析场景中,它的表现已经超出我的预期了。
在未来,当所有应用的逻辑都移动到 AI Agent 层后,能坚挺地留在后端撑场子的,也就是数据库了。 今天,老冯就来和大家聊聊,为什么在AI浪潮下数据库依然是那个“定海神针”,同时也探讨AI时代软件开发哪些技能会贬值、哪些依然保值,并展望 Agent 时代,哪些数据库能够笑到最后。
Agent + DB:AI时代中间商消失
曾几何时,我们习惯了在应用里点点点,然后后台服务器去查询数据库、执行逻辑,再把结果返回前端给我们看。在这个过程中,应用本身其实是用户和数据库之间的“中间商”。但在AI时代,这个中间商正面临失业危机——智能代理可以直接跟数据库对话,把中间那层壳子拿掉。
想象一个生动的例子:订机票。传统流程是用户打开订票网站或App,填写日期地点,前端把请求发给后端,后端调用数据库或第三方API查航班,再把结果包装成网页返回给你。在整个过程中,浏览器或者 APP充当了中介。然而在Agent+Database的新形态下,你也许只需要对着你的AI助手说:“帮我订下周一去东京的最便宜的直飞航班,靠窗座,谢谢!”
接下来像魔法的事情发生了:这个AI Agent 会自动去几个航空公司的数据库或接口抓取航班数据,比价筛选,直接在数据库里下单锁座,然后给你回复:“搞定啦,电子机票已发邮箱。” 整个过程几乎没有传统意义上的前端界面—— AI Agent 本身就是界面,它替你完成了以前由多个软件系统串联才能完成的工作。
当然,符合大部分用户想像的 AI Agent 可能是那种 RPA Computer Use Agent,就是 AI 替你去移动鼠标敲键盘,通过网站或者 API 去请求服务。但是如果我们考虑理想的终局状态 —— 那些网页界面都是给人用的,机器其实不需要这么麻烦,完全可以绕过所有的前后端,直接操作最核心的东西 —— 数据库。
当然,现实中完全去掉中间层还需要解决安全、权限等诸多问题。不过大方向是高度确定的。应用逻辑越来越多由AI Agent 驱动,数据库则成为这些AI的“原料仓库”和“工作台”。在这个新范式下,数据库不但没被边缘化,反而因为直接面对AI请求而地位特殊。
纳德拉说的正是这种 Agent 直连数据库的终局状态。在 AI 时代,“没有中间商赚差价”不再只是段子,而是可能成为现实:中间应用层被压缩甚至消失,AI直接对接数据库完成任务。Agent 就像全能的小秘书,各家数据库成了它手里的工具箱。听起来很科幻?其实趋势已经很明显了:最近 MCP 爆火不过是 Agent 时代的前奏。
我听陆奇博士说过,比尔·盖茨其实几十年前就已经判断出软件领域的最终形态是 PDA (个人数字助理),当然几十年前受技术条件限制,手持好记星文曲星式的 PDA 肯定离着 AI 数字秘书差着十万八千里。但现在,这个愿景已经完全具备实现的技术条件了。当下爆火的 MCP 不过是 Agent 时代的前夜,而 Google 发布的 ADK,A2A 则可能才刚刚拉开 Agent 时代的序幕。
数据库为什么不会过时?
有人或许担心:AI都这么智能了,会不会有一天不需要传统数据库,让 AI 直接生成就行?这个想法听上去很酷,但实际情况是模糊智能系统无法取代精确的数据库系统,原因有很多:
1. 模糊系统 vs 精确系统: 大型语言模型本质上是概率模型,擅长处理不确定性和模糊问题,但让它们记住精确事实可不容易。在这一点上,它跟人类有着一样的缺陷 —— 都是模糊系统。人脑再好使,问题复杂了也需要求诸于计算器,Excel,数据库等 精确工具。无论AI多聪明,它要给出靠谱的结果,背后还是得有准确的数据支持,而数据库擅长的正是精确存储和检索。AI生成一段文本可以有点小误差无伤大雅,但要是涉及订单数量、财务报表这些严肃数据,一丝偏差都可能酿成大祸,这时候还是得靠传统数据库的精确性来保障。模糊AI负责智能推理,精确数据库负责事实基石,两者是互补而非互斥关系。
2. 没有数据库,AI就是无源之水: AI需要海量数据作为原始生产资料,这是显而易见的道理。计算机领域有句老话:“Garbage In, Garbage Out(垃圾进,垃圾出)”,在AI时代依然是真理。如果喂给AI模型的数据源本身质量堪忧,那AI只会加速吐出垃圾结果。这意味着企业越依赖AI决策,就越需要高质量的数据来训练和提供给AI调用。数据从哪儿来?还不是从各种数据库和数据仓库里来!正如业内所强调的:AI系统只能达到其所用数据的水准。因此,数据库作为数据的载体和提供者地位不降反升。哪怕未来最先进的AI代理,上线第一天也得先连接上你的数据库取数,否则就是个巧妇难为无米之炊的空架子。
3. 信息的可靠存储与可信度: 人类社会早就明白保存信息的价值。尽管人类可以“口耳相传”,但记忆容量有限,传承会模糊走样。从商周铸鼎刻字、甲骨铭文,到史官笔录春秋,再到印刷术和现代的图书馆档案室,我们一直在追求更可靠、更长久的存储方式。数据库正是信息社会的“鼎”和“史册”。它承担着记录和保管的重要职责,是当代的“数字档案库”。LLM 可以用有损压缩的方式模糊记住主要的知识,但精确保留的原始实时记录依然还需要外部存储。只要人类还重视信息的可靠存储和可信度,数据库的重要性就不会消失。
说到底,问题的关键在于 LLM Agent 替代的是“人”本身,而非人使用的工具。当然肯定有人会问前后端开发也是工具,为什么就数据库这个工具不一样呢?那是因为要解决存储与记忆的问题,无论是人类还是大模型 Agent,都需要使用数据库,这是解决问题的需要面对的本质复杂度。然而本质上来说 LLM/Agent 并不需要这些 给人使用的中间工具 来操作数据库,它本身就拥有这样的能力,这些对 Agent 来说属于无谓的额外复杂度。
当然我们不排除以后会出现一种将数据库直接融入大模型中的新物种(比如沙丘中的人肉计算机“门泰特”,用模糊系统来仿真精确系统),可以直接将数据存储在模型权重中并实时更新,动态调整,让现在的数据库“过时”。但起码对于最近十几年来说,依然是遥远的设想而已。
IT技能:哪些贬值,哪些保值?
我最近重新做了 Pigsty 的官网。整个过程就我一个人,全是我靠嘴来说,Cursor 来脑补,最后摇个 Gemini 2.5 来画图。我想找个前端大手子帮我美化一下,大手子说,我也只能做到这个水平了。他要价 1000 ¥/页面,而我耗时十几分钟靠嘴实现了这样的效果。另一个例子是文档翻译,在三年前,我花了五千块找了个计算机研究生,帮我把三十多份文档从中文翻译成英文。而现在一个翻译工作流,半个小时就能信达雅的将中文内容翻译成各种语言。
AI 带来的生产力提高是非常惊人的,但反过来说,AI 消也灭掉了很多工作岗位,比如翻译,插画师,前端UX 等。你可以说,细分领域 1% 的头部专家永远有饭吃,但 99% 的工作岗位被铲平了,基本上也就意味着这个行业被 AI “替代”掉了。
让许多人震惊的是,AI 最先替代的并不是传统意义上很多人设想的体力劳动或一般性脑力劳动,而是高创造性的领域。例如,创造 AI 的软件工程师/程序员本身就在 AI 时代面临着巨大的冲击。
随着 Anthropic 的 Claude Code Agent 代码泄漏,各种代码 Agent 已经出现了百花齐放。中低级程序员目前已经接近团灭状态,UI / UX 哭晕在厕所,前后端岌岌可危。很多技能在 Vibe Coding 面前快速贬值 —— 几年经验的程序员,被 20 $/月的 AI Agent 打翻在地。不过总的来说,尽管程序员本身受到 Code Agent 的巨大冲击,但好在其他行业还在补数字化的课,程序员作为平均最熟悉 AI 的群体,起码不至于像翻译/插画那样跳崖式塌方。
那么什么 IT 技能最保值呢?在这个剧烈变化的时代中,有一项技能依然稳如老狗,那就是数据库。 AI 行业现状发展瞬息万变,跟之前前端领域有一拼,刚学的新花样很可能过两个月就过时了。反而是几十年前的数据库设计,建模,SQL 等知识在几十年后依然硬朗坚挺。并且按目前的发展势头,还会在 AI 时代继续坚挺下去。未来掌握数据库和数据工程技能的程序员,会更加抢手。而纯粹只会切图写页面、不懂业务数据的人,饭碗可能就危险了。
AI 时代,哪些数据库有机会
那么,哪些数据库值得学习呢?
展望未来数据库江湖,在AI和新应用需求的冲击下,胜出的将是那些满足 Agent 需求的数据库 —— 当然老冯懒得说一些正确而无用的冠冕堂皇屁话 —— 没其他数据库什么事儿了,基本上就是 PostgreSQL 了。
首先,OpenAI 用的是什么?PostgreSQL。然后,Cursor 用的是什么?PostgreSQL,此外,还有 Dify,Notion,Cohere,Replit,Perplexity,你会发现,新一代 AI 公司的数据库选型惊人的一致。Anthropic 虽然没有公开说他们用什么,但是 MCP 的例子里大剌剌摆着 PostgreSQL 作为除了文件系统之外的第二个例子,就很能说明问题了。
而 Cursor 的 CTO Sualeh Asif 在斯坦福 153 Infra @ Scale 上的演讲说的更直接(https://www.youtube.com/watch?v=4jDQi9P9UIw )
用 Postgres 就行了,别整那些花里胡哨的东西。
大家选择 PostgreSQL 原因很简单, PostgreSQL 可以在一个数据库里解决所有问题:关系型数据,向量数据,JSON文档数据,GIS 地理空间数据,全文检索词向量与倒排索引数据,图数据,甚至还有 OLAP 分析性能比肩 CK 的 DuckDB 列式存储引擎。而这种多模态的能力正是 Agent 复杂多面需求所需要的终极 All-In-One 解决方案:如果你能通过 PG 扩展用一行 SQL 解决千行代码才能解决的问题,那么就可以极大降低 LLM 的心智负担,智力要求与 Token 消耗。
PostgreSQL 生态 + pgvector 俨然成为 LLM-驱动产品的“默认安全牌”。pgvector 在很多人印象里只是 PG 生态中的一个扩展,实际上直到 OpenAI Retrivial Plugin 带火向量数据库赛道之前,这还都属于个人兴趣项目。然而在 PG 社区生态的合力下,例如 AWS ,Neon,Supabase 都砸入了大量资源改进它,让他从六七款 PG 向量扩展中脱颖而出,在一年时间内实现了 150 倍的性能改进,将整个专用向量数据库赛道变成了一个笑话。 —— 即使是专用向量数据库中最能打的 Milvus,也无法撼动这一点:它不是在跟某个 PG 社区爱好者打擂台,而是跟 AWS RDS 团队和好几个精英团队在拼资源 … )
当然,我认为 SQLite 也会在 Agent 时代进一步大放光彩,当然 PG 生态也有 PGLite/DuckDB 来这个领域竞争。
当然最后是老冯的广告时 间。PostgreSQL 是个好数据库,但想用好这个数据库其实并不容易。无论是天价的 RDS 还是稀缺的 DBA,对许多用户都不是一个可选项。但比起手工土法自建来说,我们提供了一个开源免费的更好选择:开箱即用的 PostgreSQL 发行版 Pigsty,打包了 PG 生态所有能打的扩展插件(当然 pgvector 必须默认安装),让你一分钟,几条命令就从一台裸服务器上拉起生产级的 PostgreSQL RDS 服务。如果你需要专家的服务支持,我们提供可以摇人兜底的付费订阅选项~:但软件本身是开源免费不要钱的,纯属做做公益,交个朋友,希望能够帮助大家用好 PostgreSQL。
MySQL vs PostgreSQL @ 2025
在 2025 年的当下,MySQL 无论是在功能特性集,质量正确性,性能表现,还是生态与社区上都被 PostgreSQL 拉开了差距,而且这个差距还在进一步扩大中。
今天我们就来对 MySQL 与 PostgreSQL 进行一个全方位的对比,从功能,性能,质量,生态来全方位反映这几年的生态变化。
功能
让我们先从开发者最关注的东西 —— 功能特性开始说起。
新版本
昨天,MySQL 发布了 “创新版本” 9.3 但是看上去和先前的 9.x 一样,都是些修修补补,看不到什么创新的东西。 搜索尚未发布的 PostgreSQL 18,你能看到无数特性预览的介绍文章;而搜索 MySQL 9.3,能看到的是社区对此的抱怨与失望。
MySQL 老司机丁奇看完 ReleaseNote 之后表示,《MySQL创新版正在逐渐失去它的意义》,德哥看后写了 《MySQL将保持平庸》。 对于 MySQL 的 “创新版本”,Percona CEO, Peter Zaitsev 也发三篇《MySQL将何去何从》,《Oracle最终还是杀死了MySQL》,《Oracle还能挽救MySQL吗》,公开表达了对 MySQL 的失望与沮丧。
在最近几年,MySQL 在新功能上乏善可陈,与突飞猛进的 PostgreSQL 形成了鲜明的对比。
新功能
以数据库领域最近两年最为火爆的增量场景 —— 向量数据库为例。在前两年的 向量数据库热潮中,PostgreSQL 生态里就涌现出了至少六七款向量数据库扩展( pgvector
,pgvector.rs
,pg_embedding
,latern
,pase
,pgvectorscale
, vchord
),并在你追我赶的赛马中卷出了新高度。最终在 AWS 的资源投入下,pgvector
在一年内实现了 150x 的性能飞跃,将整个专用向量数据库市场都给轰平了。
在当下,PostgreSQL 生态正在进行着 如火如荼的 DuckDB 缝合大赛 ,pg_duckdb
与 pg_mooncake
等扩展甚至已经进 ClickBench 分析性能榜单 T0 梯队,
也开始进入 Thoughtworks 技术雷达评估 Radar,正在攻克真正的 OLTP 与 OLAP 融合问题,旨在替代 OLAP 大数据全家桶。
与此同时进行的还有用于原地替代 ElasticSearch Tantivy/BM25 缝合大赛。
而 MySQL 在这段时间里更新了什么功能呢?一个不支持计算距离与索引的羞辱性 VECTOR 实现;还有一个企业版专属的 JS 存储过程支持(开源版没有!),而这是 PG 15 年前就可以通过 plv8
扩展实现的功能了。当 MySQL 还局限在 “关系型 OLTP 数据库” 的定位时, PostgreSQL 早已经放飞自我,从一个关系型数据库发展成了一个多模态的数据库,成为了一个数据管理的抽象框架与开发平台。
扩展性
来自 CMU 的 Abigale Kim 对主流数据库的可扩展性进行了研究:PostgreSQL 有着所有 DBMS 中最好的 可扩展性(Extensibility),以及其他数据库生态难望其项背的扩展插件数量 —— 375+,这还只是 PGXN 注册在案的实用插件,实际生态扩展总数已经破千。至少在开源的 PostgreSQL RDS 发行版 Pigsty 中,就已经开箱即用的提供 405 个扩展的 DEB/RPM 包了。
PostgreSQL 有着一个繁荣的扩展生态 —— 地理空间,时间序列,向量检索,机器学习,OLAP分析,全文检索,图数据库;这些扩展让 PostgreSQL 真正成为一专多长的全栈数据库 —— 单一数据库选型便可替代各式各样的专用组件: MySQL,MongoDB,Kafka,Redis,ElasticSearch,Neo4j,甚至是专用分析数仓与数据湖。
PostgreSQL正在吞噬数据库世界 —— 它正在通过插件的方式,将整个数据库世界内化其中。“一切皆用 Postgres” 也已经不再是少数精英团队的前沿探索,而是成为了一种进入主流视野的最佳实践。
而在新功能支持上,MySQL 却显得十分消极 —— 一个应该有大量 Breaking Change 的“创新大版本更新”,不是糊弄人的摆烂特性,就是企业级的特供鸡肋,一个大版本就连鸡零狗碎的小修小补都凑不够数。
兼容性
除了海量扩展外,PostgreSQL 生态还有更离谱的:兼容功能:你还可以使用扩展或者分支,实现对其他数据库的兼容。
内核 | 特色 | 交付形态 | 公司 |
---|---|---|---|
Citus | 分布式 HTAP / 多租户 | 扩展 | 微软 |
DocumentDB | MongoDB 功能特性兼容 | 扩展 | 微软 |
Babelfish | MSSQL 线缆协议兼容 | 内核分支 | AWS |
FerretDB | MongoDB 线缆协议兼容 | 中间件 | Ferret |
OrioleDB | 云原生Undo存储引擎 | 扩展+补丁内核 | Supabase |
OpenHalo | MySQL 线缆协议 兼容 | 内核分支 | 易景 |
IvorySQL | Oracle 语法特性兼容 | 内核分支 | 瀚高 |
PolarDB | Aurora/RAC 特性兼容 | 内核分支 | 阿里云 |
PolarDB O | Oracle 语法特性兼容 | 内核分支 | 阿里云 |
其中,openHalo 对 MySQL 生态可谓釜底抽薪 —— 在 PG 上直接兼容 MySQL 的线缆协议,这意味着 MySQL 应用可以在不改驱动/代码的情况下迁移到 PostgreSQL 上来。 另外,OrioleDB 在原生 PostgreSQL 的基础上,增加了云原生 Undo 存储引擎,支持高并发的分布式事务处理,号称实现了 4x 的吞吐量性能。
这并非纸面上的 PR 稿,这些内核/扩展都已经全部在 PostgreSQL 发行版 Pigsty 中作为开箱即用的 RDS 服务直接可用。
在这种性能怪兽面前,MySQL 将何去何从?
性能
缺少功能也许并不是一个无法克服的问题 —— 对于一个数据库来说,只要它能将自己的本职工作做得足够出彩,那么架构师总是可以多费些神,用各种其他的数据积木一起拼凑出所需的功能。
性能劣化的MYSQL
MySQL 曾引以为傲的核心特点便是 性能 —— 至少对于互联网场景下的简单 OLTP CURD 来说,它的性能是非常不错的。然而不幸地是,这一点也正在遭受挑战:Percona 的博文《Sakila:你将何去何从》中提出了一个令人震惊的结论:
MySQL 的版本越新,性能反而越差。
根据 Percona 的测试,在 sysbench 与 TPC-C 测试下,最新 MySQL 8.4 版本的性能相比 MySQL 5.7 出现了平均高达 20% 的下降。而 MySQL 专家 Mark Callaghan 进一步进行了 详细的性能回归测试,确认了这一现象:
MySQL 8.0.36 相比 5.6 ,QPS 吞吐量性能下降了 25% ~ 40% !
鸡肋的分析性能
尽管 MySQL 的优化器在 8.x 有一些改进,一些复杂查询场景下的性能有所改善,但分析与复杂查询本来就不是 MySQL 的长处与适用场景,只能说聊胜于无。相反,如果作为基本盘的 OLTP CRUD 性能出了这么大的折损,那确实是完全说不过去的。
ClickBench:MySQL 打这个榜确实有些不明智
Peter Zaitsev 在博文《Oracle最终还是杀死了MySQL》中评论:“与 MySQL 5.6 相比,MySQL 8.x 单线程简单工作负载上的性能出现了大幅下滑。你可能会说增加功能难免会以牺牲性能为代价,但 MariaDB 的性能退化要轻微得多,而 PostgreSQL 甚至能在 新增功能的同时显著提升性能”。
稳步提升的PostgreSQL性能
MySQL的性能随版本更新而逐步衰减,但在同样的性能回归测试中,PostgreSQL 性能却可以随版本更新有着稳步提升。特别是在最关键的写入吞吐性能上,最新的 PostgreSQL 17beta1 相比六年前的 PG 10 甚至有了 30% ~ 70% 的提升。
在 Mark Callaghan 的 性能横向对比 (sysbench 吞吐场景) 中,我们可以看到五年前 PG 11 与 MySQL 5.6 的性能比值(蓝),与当下 PG 16 与 MySQL 8.0.34 的性能比值(红)。PostgreSQL 和 MySQL 的性能差距在这五年间拉的越来越大。
几年前的业界共识是 PostgreSQL 与 MySQL 在 简单 OLTP CRUD 场景 下的性能基本相同。然而此消彼长之下,现在 PostgreSQL 的性能已经远远甩开 MySQL 了。 PostgreSQL 的各种读吞吐量相比 MySQL 高 25% ~ 100% 不等,在一些写场景下的吞吐量更是达到了 200% 甚至 500% 的恐怖水平。
在真实场景中的对比
一个有趣的佐证是知名开源项目 JuiceFS 对不同数据库作为元数据引擎的性能测试。
在这个例子中,我们可以很清晰的看出 MySQL 和 PostgreSQL 在一个真实三方评测中的性能差距。
MySQL 赖以安身立命的性能优势,已经不复存在了。
关于 PostgreSQL 与 MySQL 与 PostgreSQL 的性能评测,我建议各位参考 Mark Callaghan 发表在 Small Datum 上的文章。这是前 Google / Meta 的 MySQL Tech Lead 。 尽管他的主要职业生涯在与 MySQL,Oracle,MongoDB 打交道,并非 PostgreSQL 专家,但他严谨的测试方法与结果分析为读者带来了许多数据库性能方面的洞见。
- Postgres 17.4 与大型服务器上的 sysbench
- https://smalldatum.blogspot.com/2025/03/at-what-level-of-concurrency-do-mysql.html
质量
如果新版本只是性能不好,总归还有办法来优化修补。但如果是质量出了问题,那真就是无可救药了。
正确性
例如,Percona 最近刚刚在 MySQL 8.0.38 以上的版本(8.4.x, 9.0.0)中发现了一个 严重Bug —— 如果数据库里表超过 1万张,那么重启的时候 MYSQL 服务器会直接崩溃! 一个数据库里有1万张表并不常见,但也并不罕见 —— 特别是当用户使用了一些分表方案,或者应用会动态创建表的时候。而直接崩溃显然是可用性故障中最严重的一类情形。
但 MySQL 的问题不仅仅是几个软件 Bug,而是根本性的问题 —— 《MySQL 糟糕的 ACID 正确性》指出,在正确性这个体面数据库产品必须的基本属性上,MySQL 的表现一塌糊涂。
权威的分布式事务测试组织 JEPSEN 研究发现,MySQL 文档声称实现的 可重复读/RR 隔离等级,实际提供的正确性保证要弱得多 —— MySQL 8.0.34 默认使用的 RR 隔离等级实际上并不可重复读,甚至既不原子也不单调,连 单调原子视图/MAV 的基本水平都不满足。这意味着 MySQL 的 RR 隔离等级实际上还不如绝大多数 DBMS 的 RC 隔离等级(实际 MAV)。
MySQL 的 ACID 存在缺陷,且与文档承诺不符 —— 而轻信这一虚假承诺可能会导致严重的正确性问题,例如数据错漏与对账不平。对于一些数据完整性很关键的场景 —— 例如金融,这一点是无法容忍的。
此外,能“避免”这些异常的 MySQL 可串行化/SR 隔离等级难以生产实用,也非官方文档与社区认可的最佳实践;尽管专家开发者可以通过在查询中显式加锁来规避此类问题,但这样的行为极其影响性能,而且容易出现死锁。
与此同时,PostgreSQL 在 9.1 引入的 可串行化快照隔离(SSI) 算法可以用极小的性能代价提供完整可串行化隔离等级 —— 而且 PostgreSQL 的 SR 在正确性实现上毫无瑕疵 —— 这一点即使是 Oracle 也难以企及。
李海翔教授在《一致性八仙图》论文中,系统性地评估了主流 DBMS 隔离等级的正确性,图中蓝/绿色代表正确用规则/回滚避免异常;黄A代表异常,越多则正确性问题就越多;红“D”指使用了影响性能的死锁检测来处理异常,红D越多性能问题就越严重;
不难看出,这里正确性最好(无黄A)的实现是 PostgreSQL SR,与基于PG的 CockroachDB SR,其次是略有缺陷 Oracle SR;主要都是通过机制与规则避免并发异常;而 MySQL 出现了大面积的黄A与红D,正确性水平与实现手法糙地不忍直视。
做正确的事很重要,而正确性是不应该拿来做利弊权衡的。在这一点上,开源关系型数据库两巨头 MySQL 和 PostgreSQL 在早期实现上就选择了两条截然相反的道路: MySQL 追求性能而牺牲正确性;而学院派的 PostgreSQL 追求正确性而牺牲了性能。
在互联网风口上半场中,MySQL 因为性能优势占据先机乘风而起。但当性能不再是核心考量时,正确性就成为了 MySQL 的致命出血点。 更为可悲的是,MySQL 连牺牲正确性换来的性能,都已经不再占优了,这着实让人唏嘘不已。
完备性
SQL 特性与标准支持:PostgreSQL 一直以高度符合 SQL 标准著称,支持复杂查询、窗口函数、公共表表达式(CTE)、递归查询、完整的外键约束等功能,并且实现了丰富的 SQL/JSON 标准和自定义函数。 MySQL 过去在标准支持上相对落后,但自 8.0 版本起补齐了一些短板:如支持窗口函数和 CTE(包括递归 CTE)等,使其在查询特性上拉近了距离。 但是魔鬼在细节中,许多看上去 “你有我也有” 的功能,内在的实现水准是完全不一样的。
以 Ecoding 字符编码与 Collation 排序规则为例,这是很典型的企业级应用需要的多语言关键特性。PostgreSQL 在 ICU 支持下提供了 42 种字符集编码与 815 种排序规则支持,覆盖了几乎你能想象到的一切排序方法。 而 MySQL 在基本上就只有 五种字符集和几十个基于此的排序规则。这是一个很好的微观细节样本,体现出 PostgreSQL 与 MySQL 在细节上的用心程度与差异。
生态
对一项技术而言,用户的规模直接决定了生态的繁荣程度。瘦死的骆驼比马大,烂船也有三斤钉。 MySQL 曾经搭乘互联网东风扶摇而起,攒下了丰厚的家底,它的 Slogan 就很能说明问题 —— “世界上最流行的开源关系型数据库”。
开发者
MySQL 的 Slogan 是 “世界上最流行的开源关系型数据库”,但似乎现在并没有多少权威数据能支持这个说法。
相反的是,在 StackOverflow 过去八年的全球开发者调研中,我们可以观察到 PostgreSQL 在开发者中的使用率节节攀升,并于 2023 年第一次超过 MySQL ,成为最流行的数据库。
从各个角度上来看,MySQL “最流行” 的称号已经名不副实了。而 PostgreSQL 已经成为这几年最流行的数据库,并且不需要不需要开源/关系型等定语修饰。
在最为活跃的前端开发者生态中,PostgreSQL 已经凭借丰富的功能特性,以压倒性优势成为最受欢迎的的数据库。
在 Vercel 支持的 7 款存储服务上,四个是 Postgres 衍生(Neon,Supabase,Nile,Gel),两个 Redis 衍生,一个 DuckDB ,完全不见 MySQL 的踪影。
而根据 DBDB.io 的统计数据,派生自 PostgreSQL 的数据库项目也显著超过了 MySQL。
厂商
最直观的数据: AWS RDS 上 PostgreSQL 实例的数量与 MySQL 实例的数量已经达到了 6:4 ,也就是 PG 实例的数量已经比 MySQL 要多 50% 了。 详情参考:《PostgreSQL取得对MYSQL的压倒性优势》
即使是在 MYSQL 曾经占据压倒性优势的中国大陆,来自阿里云 RDS 实例数的样本也说明 MYSQL:PG 从前几年的 10:1 快速缩小到 5:1,并且增量上 PG 也已经超过 MYSQL 了。
从商业角度看,云厂商已经将重注下在了 PostgreSQL,而非 MySQL 上。 例如 AWS RDS (MySQL+PG)产品经理是 PostgreSQL 社区核心组成员 Jonathan Katz,也是最近 pg/pgvector 在向量数据库领域崛起关键推手之一。
最近的 Aurora 新品分布式 DSQL 只有 PostgreSQL 兼容,没搞 MySQL 的,而以前这种事从来都是 MySQL 优先,这次似乎直接放弃 MYSQL 支持了。 Google 的 OLTP 数据库 AlloyDB 也选择完全兼容 PostgreSQL ,并且也在 Spanner 中提供 PostgreSQL 了。 国内云厂商例如阿里云也选择押宝 PostgreSQL 分支路线,例如获得信创资质认证的 PolarDB 2.0 (Oracle)兼容其实就是基于 PolarDB PG 二次分枝的版本。
资本市场
最近的 大额融资纪录,也基本发生在 PostgreSQL 生态中。
而 MySQL 生态屈指可数,基本只有 SingleStore,TiDB ,而原本生态中全村的希望 MariaDB 则一路跌的干脆直接要退市私有化了。
大型用例
对于制造业,金融,非互联网场景,PostgreSQL 凭借其强大的功能特性与正确性,已经成为了许多大型企业的首选数据库。
例如在我任职 Apple 期间,我们部门使用 PostgreSQL 存储所有工厂的工业互联网数据并进行数据分析。包括我们部门在内的许多项目都在使用 PostgreSQL,甚至有一个内部的社区与兴趣小组。
大型互联网公司受制于历史路径依赖于惯性仍然保留有大量 MySQL,但在新兴创业公司中,PostgreSQL 已经取得显著优势。 例如,Cursor、 Dify、Notion 这样的 AI 新宠都默认使用 PostgreSQL 作为元数据存储。支付明星企业 Strip 也在一些系统中使用 PostgreSQL 进行分析。
Cloudflare 与 Vercel 的内部系统大量使用了 PostgreSQL, Node.js 社区项目也明显对 PostgreSQL 有偏好(例如 Prisma ORM 对PG 支持更完善)
MYSQL 到底怎么了?
究竟是谁杀死了 MySQL,难道是 PostgreSQL 吗?Peter Zaitsev 在《Oracle最终还是杀死了MySQL》一文中控诉 —— Oracle 的不作为与瞎指挥最终害死了 MySQL;并在后续《Oracle还能挽救MySQL吗》一文中指出了真正的根因:
MySQL 的知识产权被 Oracle 所拥有,它不是像 PostgreSQL 那种 “由社区拥有和管理” 的数据库,也没有 PostgreSQL 那样广泛的独立公司贡献者。不论是 MySQL 还是其分叉 MariaDB,它们都不是真正意义上像 Linux,PostgreSQL,Kubernetes 这样由社区驱动的的原教旨纯血开源项目,而是由单一商业公司主导。
比起向一个商业竞争对手贡献代码,白嫖竞争对手的代码也许是更为明智的选择 —— AWS 和其他云厂商利用 MySQL 内核参与数据库领域的竞争,却不回馈任何贡献。于是作为竞争对手的 Oracle 也不愿意再去管理好 MySQL,而干脆自己也参与进来搞云 —— 仅仅只关注它自己的 MySQL heatwave 云版本,就像 AWS 仅仅专注于其 RDS 管控和 Aurora 服务一样。在 MySQL 社区凋零的问题上,云厂商也难辞其咎。
总结
尽管我是 PostgreSQL 的坚定支持者,但我也赞同 Peter Zaitsev 的观点:“如果 MySQL 彻底死掉了,开源关系型数据库实际上就被 PostgreSQL 一家垄断了,而垄断并不是一件好事,因为它会导致发展停滞与创新减缓。PostgreSQL 要想进入全盛状态,有一个 MySQL 作为竞争对手并不是坏事”
至少,MySQL 可以作为一个鞭策激励,让 PostgreSQL 社区保持凝聚力与危机感,不断提高自身的技术水平,并继续保持开放、透明、公正的社区治理模式,从而持续推动数据库技术的发展。
MySQL 曾经也辉煌过,也曾经是“开源软件”的一杆标杆,但再精彩的演出也会落幕。MySQL 正在死去 —— 更新疲软,功能落后,性能劣化,质量出血,生态萎缩,此乃天命,实非人力所能改变。而 PostgreSQL ,将带着开源软件的初心与愿景继续坚定前进 —— 它将继续走 MySQL 未走完的长路,写 MySQL 未写完的诗篇。
MySQL 的创新版正在逐渐失去它的意义,德哥看后写了 MySQL将保持平庸。 对于 MySQL 的 “创新版本”,Percona 的老板 Peter Zaitsev 也发三篇《MySQL将何去何从》,《Oracle最终还是杀死了MySQL》,《Oracle还能挽救MySQL吗》,公开表达了对 MySQL 的失望与沮丧。沮丧;
与此同时,MySQL 的生态正在不断萎缩,由于 MYSQL 属于 Oracle,其他生态参与者越来越没有兴趣为 Oracle 做贡献,Oracle 也将心思放在 了 MySQL 企业版上,导致 MySQL 的开源社区越来越小,越来越没有活力。 例如,最近的 MySQL 9.x “创新版本” 被社区评价为毫无诚意的平庸之作。
例如 MySQL 开源生态的关键参与者 Percona 老板 Peter Zaitsev 也Percona 的老板 Peter Zaitsev 也发三篇《MySQL将何去何从》,《Oracle最终还是杀死了MySQL》,《Oracle还能挽救MySQL吗》,公开表达了对 MySQL 的失望与沮丧。沮丧;
其实从 AWS 的产品发布与技术投入路线来看,不难看出全球云计算一哥已经把重注都下在了 PostgreSQL 上,首先整个 RDS (MySQL + PGSQL)的产品经理就是 PostgreSQL 社区核心组成员 Jonathan Katz ,近两年 PG/PGVECTOR 在向量数据库领域嘎嘎乱杀,背后的主要推手和贡献者就是 AWS。
对一项技术而言,用户的规模直接决定了生态的繁荣程度。瘦死的骆驼比马大,烂船也有三斤钉。 MySQL 曾经搭乘互联网东风扶摇而起,攒下了丰厚的家底,它的 Slogan 就很能说明问题 —— “世界上最流行的开源关系型数据库”。
不幸地是在 2023 年,至少根据全世界最权威的开发者调研之一的 StackOverflow Annual Developer Survey 结果来看,MySQL 的使用率已经被 PostgreSQL 反超了 —— 最流行数据库的桂冠已经被 PostgreSQL 摘取。
特别是,如果将过去七年的调研数据放在一起,就可以得到这幅 PostgreSQL / MySQL 在专业开发者中使用率的变化趋势图(左上) —— 在横向可比的同一标准下,PostgreSQL 流行与 MySQL 过气的趋势显得一目了然。
对于中国来说,此消彼长的变化趋势也同样成立。但如果对中国开发者说 PostgreSQL 比 MySQL 更流行,那确实是违反直觉与事实的。
将 StackOverflow 专业开发者按照国家细分,不难看出在主要国家中(样本数 > 600 的 31 个国家),中国的 MySQL 使用率是最高的 —— 58.2% ,而 PG 的使用率则是最低的 —— 仅为 27.6%,MySQL 用户几乎是 PG 用户的一倍。
与之恰好反过来的另一个极端是真正遭受国际制裁的俄联邦:由开源社区运营,不受单一主体公司控制的 PostgreSQL 成为了俄罗斯的数据库大救星 —— 其 PG 使用率以 60.5% 高居榜首,是其 MySQL 使用率 27% 的两倍。
中国因为同样的自主可控信创逻辑,最近几年 PostgreSQL 的使用率也出现了显著跃升 —— PG 的使用率翻了三倍,而 PG 与 MySQL 用户比例已经从六七年前的 5:1 ,到三年前的3:1,再迅速发展到现在的 2:1,相信会在未来几年内会很快追平并反超世界平均水平。 毕竟,有这么多的国产数据库,都是基于 PostgreSQL 打造而成 —— 如果你做政企信创生意,那么大概率已经在用 PostgreSQL 了。
抛开政治因素,用户选择使用一款数据库与否,核心考量还是质量、安全、效率、成本等各个方面是否“先进”。先进的因会反映为流行的果,流行的东西因为落后而过气,而先进的东西会因为先进变得流行,没有“先进”打底,再“流行”也难以长久。
如果你还在使用 MYSQL 准备做一些与数据库有关的新业务,是时候更新一下认知
都已经被 PostgreSQL 拉开了差距,而且这个差距还在进一步扩大中。
昨天,MySQL 发布了 “创新版本” 9.3 但是看上去和先前的 9.x 一样,都是些修修补补,看不到什么创新的东西。
MySQL 老司机丁奇看完 ReleaseNote 之后表示,MySQL 的创新版正在逐渐失去它的意义,德哥看后写了 MySQL将保持平庸。 对于 MySQL 的 “创新版本”,Percona 的老板 Peter Zaitsev 也发三篇《MySQL将何去何从》,《Oracle最终还是杀死了MySQL》,《Oracle还能挽救MySQL吗》,公开表达了对 MySQL 的失望与沮丧。沮丧;
然而和先前的几个版本一样,依然
PostgreSQL 正在高歌猛进,而 MySQL 却日薄西山,作为 MySQL 生态主要扛旗者的 Percona 也不得不悲痛地承认这一现实,连发三篇《MySQL将何去何从》,《Oracle最终还是杀死了MySQL》,《Oracle还能挽救MySQL吗》,公开表达了对 MySQL 的失望与沮丧;
Percona 的 CEO Peter Zaitsev 也表示:
有了 PostgreSQL,谁还需要 MySQL 呢? —— 但如果 MySQL 死了,PostgreSQL 就真的垄断数据库世界了,所以 MySQL 至少还可以作为 PostgreSQL 的磨刀石,让 PG 进入全盛状态。
有的数据库正在吞噬数据库世界,而有的数据库正在黯然地凋零死去。
MySQL is dead,Long live PostgreSQL!
空洞无物的创新版本
MySQL 官网发布的 “What’s New in MySQL 9.0” 介绍了 9.0 版本引入的几个新特性,而 MySQL 9.0 新功能概览 一文对此做了扼要的总结:
然后呢?就这些吗?这就没了!?
这确实是让人惊诧不已,因为 PostgreSQL 每年的大版本发布都有无数的新功能特性,例如计划今秋发布的 PostgreSQL 17 还只是 beta1,就已然有着蔚为壮观的新增特性列表:
而最近几年的 PostgreSQL 新增特性甚至足够专门编成一本书了。比如《快速掌握PostgreSQL版本新特性》便收录了 PostgreSQL 最近七年的重要新特性 —— 将目录塞的满满当当:
回头再来看看 MySQL 9 更新的六个特性,后四个都属于无关痛痒,一笔带过的小修补,拿出来讲都嫌丢人。而前两个 向量数据类型 和 JS存储过程 才算是重磅亮点。
BUT ——
MySQL 9.0 的向量数据类型只是 BLOB
类型换皮 —— 只加了个数组长度函数,这种程度的功能,28年前 PostgreSQL 诞生的时候就支持了。
而 MySQL Javascript 存储过程支持,竟然还是一个 企业版独占特性,开源版不提供 —— 而同样的功能,13年前 的 PostgreSQL 9.1 就已经有了。
时隔八年的 “创新大版本” 更新就带来了俩 “老特性”,其中一个还是企业版特供。“创新”这俩字,在这里显得如此辣眼与讽刺。
枯萎收缩的生态规模
对一项技术而言,用户的规模直接决定了生态的繁荣程度。瘦死的骆驼比马大,烂船也有三斤钉。 MySQL 曾经搭乘互联网东风扶摇而起,攒下了丰厚的家底,它的 Slogan 就很能说明问题 —— “世界上最流行的开源关系型数据库”。
不幸地是在 2023 年,至少根据全世界最权威的开发者调研之一的 StackOverflow Annual Developer Survey 结果来看,MySQL 的使用率已经被 PostgreSQL 反超了 —— 最流行数据库的桂冠已经被 PostgreSQL 摘取。
特别是,如果将过去七年的调研数据放在一起,就可以得到这幅 PostgreSQL / MySQL 在专业开发者中使用率的变化趋势图(左上) —— 在横向可比的同一标准下,PostgreSQL 流行与 MySQL 过气的趋势显得一目了然。
对于中国来说,此消彼长的变化趋势也同样成立。但如果对中国开发者说 PostgreSQL 比 MySQL 更流行,那确实是违反直觉与事实的。
将 StackOverflow 专业开发者按照国家细分,不难看出在主要国家中(样本数 > 600 的 31 个国家),中国的 MySQL 使用率是最高的 —— 58.2% ,而 PG 的使用率则是最低的 —— 仅为 27.6%,MySQL 用户几乎是 PG 用户的一倍。
与之恰好反过来的另一个极端是真正遭受国际制裁的俄联邦:由开源社区运营,不受单一主体公司控制的 PostgreSQL 成为了俄罗斯的数据库大救星 —— 其 PG 使用率以 60.5% 高居榜首,是其 MySQL 使用率 27% 的两倍。
中国因为同样的自主可控信创逻辑,最近几年 PostgreSQL 的使用率也出现了显著跃升 —— PG 的使用率翻了三倍,而 PG 与 MySQL 用户比例已经从六七年前的 5:1 ,到三年前的3:1,再迅速发展到现在的 2:1,相信会在未来几年内会很快追平并反超世界平均水平。 毕竟,有这么多的国产数据库,都是基于 PostgreSQL 打造而成 —— 如果你做政企信创生意,那么大概率已经在用 PostgreSQL 了。
抛开政治因素,用户选择使用一款数据库与否,核心考量还是质量、安全、效率、成本等各个方面是否“先进”。先进的因会反映为流行的果,流行的东西因为落后而过气,而先进的东西会因为先进变得流行,没有“先进”打底,再“流行”也难以长久。
究竟是谁杀死了MySQL?
究竟是谁杀死了 MySQL,难道是 PostgreSQL 吗?Peter Zaitsev 在《Oracle最终还是杀死了MySQL》一文中控诉 —— Oracle 的不作为与瞎指挥最终害死了 MySQL;并在后续《Oracle还能挽救MySQL吗》一文中指出了真正的根因:
MySQL 的知识产权被 Oracle 所拥有,它不是像 PostgreSQL 那种 “由社区拥有和管理” 的数据库,也没有 PostgreSQL 那样广泛的独立公司贡献者。不论是 MySQL 还是其分叉 MariaDB,它们都不是真正意义上像 Linux,PostgreSQL,Kubernetes 这样由社区驱动的的原教旨纯血开源项目,而是由单一商业公司主导。
比起向一个商业竞争对手贡献代码,白嫖竞争对手的代码也许是更为明智的选择 —— AWS 和其他云厂商利用 MySQL 内核参与数据库领域的竞争,却不回馈任何贡献。于是作为竞争对手的 Oracle 也不愿意再去管理好 MySQL,而干脆自己也参与进来搞云 —— 仅仅只关注它自己的 MySQL heatwave 云版本,就像 AWS 仅仅专注于其 RDS 管控和 Aurora 服务一样。在 MySQL 社区凋零的问题上,云厂商也难辞其咎。
逝者不可追,来者犹可待。PostgreSQL 应该从 MySQL 的衰亡中吸取教训 —— 尽管 PostgreSQL 社区非常小心地避免出现一家独大的情况出现,但生态确实在朝着一家/几家巨头云厂商独大的不利方向在发展。云正在吞噬开源 —— 云厂商编写了开源软件的管控软件,组建了专家池,通过提供维护攫取了软件生命周期中的绝大部分价值,但却通过搭便车的行为将最大的成本 —— 产研交由整个开源社区承担。而 真正有价值的管控/监控代码却从来不回馈开源社区 —— 在数据库领域,我们已经在 MongoDB,ElasticSearch,Redis,以及 MySQL 上看到了这一现象,而 PostgreSQL 社区确实应当引以为鉴。
好在 PG 生态总是不缺足够头铁的人和公司,愿意站出来维护生态的平衡,反抗公有云厂商的霸权。例如,我自己开发的 PostgreSQL 发行版 Pigsty,旨在提供一个开箱即用、本地优先的开源云数据库 RDS 替代,将社区自建 PostgreSQL 数据库服务的底线,拔高到云厂商 RDS PG 的水平线。而我的《云计算泥石流》系列专栏则旨在扒开云服务背后的信息不对称,从而帮助公有云厂商更加体面,亦称得上是成效斐然。
尽管我是 PostgreSQL 的坚定支持者,但我也赞同 Peter Zaitsev 的观点:“如果 MySQL 彻底死掉了,开源关系型数据库实际上就被 PostgreSQL 一家垄断了,而垄断并不是一件好事,因为它会导致发展停滞与创新减缓。PostgreSQL 要想进入全盛状态,有一个 MySQL 作为竞争对手并不是坏事”
至少,MySQL 可以作为一个鞭策激励,让 PostgreSQL 社区保持凝聚力与危机感,不断提高自身的技术水平,并继续保持开放、透明、公正的社区治理模式,从而持续推动数据库技术的发展。
MySQL 曾经也辉煌过,也曾经是“开源软件”的一杆标杆,但再精彩的演出也会落幕。MySQL 正在死去 —— 更新疲软,功能落后,性能劣化,质量出血,生态萎缩,此乃天命,实非人力所能改变。 而 PostgreSQL ,将带着开源软件的初心与愿景继续坚定前进 —— 它将继续走 MySQL 未走完的长路,写 MySQL 未写完的诗篇。
数据库火星撞地球:当PG爱上DuckDB
蹭热点引出的好话题
昨晚在直播间,我与几位国内 DuckDB 先锋进行了一场对谈。 话题跨度很大,从 DeepSeek 团队在开源周推出的分布式 DuckDB 分析框架 “Smallpond”,聊到 PostgreSQL 与 DuckDB 的深度融合,相当热闹。
DeepSeek 的开源的 “小池塘”用把 DuckDB 改为分布式的用法,从营销上给 DuckDB 打了很好的广告。 但从实用角度和影响力来说,我个人对分布式 DuckDB 的价值保持保留态度(那个3FS实际上更有用)。 因为这与 DuckDB 本身的核心价值主张(大数据已死)完全背道而驰。
那么如果不去折腾 “分布式”,DuckDB 未来更有前景的方向是什么? 相比之下,我更加看好 “ DuckDB + PostgreSQL深度融合” 这路径。 我甚至认为,它可能会引爆数据库世界下一场“火星撞地球”式的变革。
DuckDB:OLAP挑战者
DuckDB 由 Mark Raasveldt 和 Hannes Mühleisen 在荷兰的 CWI (国家数学与计算机科学研究所)开发 —— 而 CWI 不仅仅是一个研究机构,可以说是分析型数据库领域发展背后的幕后推手与功臣,是列式存储引擎与向量化查询执行的先驱。
现在你能看到的各种分析数据库产品 ClickHouse,Snowflake,Databricks 背后都有它的影子。 而现在这些分析领域的先锋们自己亲自下场来做分析数据库,他们选择了一个非常好的时机与生态位切入,搞出了一个嵌入式的 OLAP 分析数据库 —— DuckDB 。
DuckDB 的起源来自作者们对数据库用户痛点的观察:数据科学家主要使用像 Python 与 Pandas 这样的工具,不怎么熟悉经典的数据库。 经常被如何连接,身份认证,数据导入导出这些工作搞的一头雾水。那么有没有办法做一个简单易用的嵌入式分析数据库给他们用呢?—— 就像 SQLite 一样。
DuckDB 整个数据库软件源代码就是一个头文件一个c++文件,编译出来就是一个独立二进制,数据库本身也就一个简单的文件。 使用兼容 PostgreSQL 的解析器与语法,简单到几乎没有任何上手门槛。尽管 DuckDB 看上去非常简单,但它最了不起的一点在于 —— 简约而不简单,分析性能也是绝冠群雄。例如,在 ClickHouse 自己的主场 ClickBench 上,有着能够吊打东道主 ClickHouse 的表现。
同时,DuckDB 使用极为友善的 MIT 许可证开源:一个有着顶尖性能表现,而使用门槛低到地板,还开源免费,允许随意包装套壳的数据库,想不火都难。
取长补短的黄金组合
尽管 DuckDB 有着顶级的分析性能,但它也有自己的短处 —— 薄弱的数据管理能力, 也就是数据科学家们不喜欢的那些东西 —— 认证/权限,访问控制,高并发,备份恢复,高可用,导入导出,等等等, 而这恰好是经典数据库的长处,也是企业级分析系统的核心痛点。
从这个角度来说,duckdb 更像是 RocksDB 这样的底层 “存储引擎”,一个 OLAP 算子。 本身距离一个真正的 “数据库” / “大数据分析平台” 还有很多工作要做。
PostgreSQL 则在数据管理领域深耕多年,拥有完善的事务机制、权限控制、备份恢复和扩展机制。 传统的 OLTP 场景下 PostgreSQL 更是 “老牌猛将” 。 但 PostgreSQL 的一个主要遗憾就是:尽管PostgreSQL 本身提供了很强大的分析功能集,应付常规的分析任务绰绰有余。 但在较大数据量下全量分析的性能,相比专用的实时数仓仍然有些不够看。
那么人们自然而然会想到,如果我们可以结合两者的能力,PostgreSQL 提供全面的管理功能与生态支持,以及顶级的 OLTP 性能。 DuckDB 提供顶尖的分析算子和 OLAP 性能;二者深度融合,就能把各自优势叠加,取长补短,在数据库领域中产生一个“新物种”。
DuckDB 能很好的解决 PostgreSQL 的分析短板,甚至还可以通过读写远程对象存储上的 Parquet 等列存文件格式,实现无限存储的数据湖仓的效果;而 DuckDB 孱弱的管理能力也通过融合成熟的 PostgreSQL 生态得到完美解决。 比起给 DuckDB 套壳,搞一套什么新的 “大数据平台/数据管理系统”,或者给 PostgreSQL 发明一个新的分析引擎,两者融合起来,取长补短,是一条阻力最小,而价值最大的道路。
实际上,这正是数据库世界中正在发生的事情,许多团队和厂商已经投入到 DuckDB + PostgreSQL 的缝合当中,试图率先抢得这个潜力巨大的新市场。
群雄逐鹿的缝合赛道
当我们把目光投向业界,不难发现 已经有诸多玩家入局了:
最早进入这条赛道的是国内个人开发者李红艳开发的 duckdb_fdw
,一直算是不温不火的状态。
然而在 《PostgreSQL正在吞噬数据库世界》以向量数据库的例子预言了 OLAP 赛道的机会之后,
PG 社区对征服 OLAP 缝合 DuckDB 的热情被彻底点燃。
就在 2024 年 3 月的时间点上,一切骤然加速。ParadeDB 的 pg_analytics
插件立即切换了技术路线,改为缝合 DuckDB。
PG 生态发起 pg_quack
项目的 HYDRA 与 DuckDB 母公司 MotherDuck 开始合作,发起了 pg_duckdb
。
官方开始下场, “不误正业” 地不去折腾 DuckDB,反而搞起了 PostgreSQL 插件。
接下来,在数据库热点上一向嗅觉敏锐的 Neon 赞助了 pg_mooncake
项目,这个新玩家选择站在 pg_duckdb 的肩膀上,
不仅要把 DuckDB 的计算能力给缝合入 PG,还要将 DuckDB / Parquet 开放分析存储格式原生融合到 PG 的表访问接口中,把存储和计算能力一起缝合到 PG 中。
一些头部云厂商,比如 阿里云rds 也在尝试缝合包装 DuckDB,标志着已经有大玩家开始忍不住准备下场了。
这种场面很容易让人联想到早两年的“向量数据库热”。当 AI 检索和语义搜索成为热点,不少厂商都“闻风而动”,基于 PostgreSQL 开发向量数据库插件,
AI爆火之后,PG 生态里就涌现出了至少六款向量数据库扩展( pgvector
,pgvector.rs
,pg_embedding
,latern
,pase
,pgvectorscale
),
并在你追我赶的赛马中卷出了新高度。
最后 pgvector
在以 AWS 为代表的厂商大力投入加注之下,在其他数据库比如 Oracle / MySQL / MariaDB 的糊弄版本姗姗来迟之前,
就已经把整个专用向量数据库细分领域给荡平了,吃下了 RAG / 向量数据库带来巨大增量里的最大蛋糕。而现在,似乎是这种赛事在 OLAP 领域的又一次预演。
为什么是DuckDB+PG?
比较有趣的一点是,这样的奇景同样只出现在 PostgreSQL 生态中,而在其他数据库社区中依然一片寂静。 甚至 DuckDB 官方也在参加 PostgreSQL 缝合大赛,而不是其他什么 DB 的缝合大赛。
所以有人会问:既然要跟 DuckDB 融合,为什么不选 MySQL、Oracle、SQL Server 甚至 MongoDB? 难道这些数据库就没有管理能力,不需要取长补短,不能跟 DuckDB 融合,获取顶级 OLAP 能力吗?
PostgreSQL 与 DuckDB 是一对完美的组合,体现在三点上:功能互补,语法兼容,可扩展性:
首先,DuckDB 使用的是 PostgreSQL 语法,甚至语法解析器都是直接照搬 PG 的, 这意味着两者关键接口的差异性非常小,天然具有亲和力,也就是不怎么需要折腾。
第二,PostgreSQL 和 DuckDB 这两个数据库均是公认的“可扩展性怪物”。无论是 FDW、插件,还是新存储引擎、新类型,都能以扩展的形式接入。 比起魔改 PG + DuckDB 的内核源码,写一个粘合两边现有接口的扩展插件显然要简单太多了,
这种天生的技术血缘亲和力,让 “PG + DuckDB” 的融合成本显著低于所有其他组合,低到李老师这样的独立个人开发者都可以参赛(顺势而为是好事,无任何贬义)。
与此同时 PostgreSQL 是世界上最流行的数据库,也是主要数据库中唯一有增长而且是高速增长的玩家,缝合 PostgreSQL 带来的收益也要比其他数据库大得多。
这意味着,“PG + DuckDB” 的融合,是一条“阻力最小、价值最大”的康庄大道,大自然厌恶真空,因此自然会有许多玩家下场来填补这里的空白生态位。
统一 OLTP 与 OLAP 的梦想
在数据库领域,OLTP 与 OLAP 的分裂由来已久。所有的“数据仓库 + 关系型数据库”架构、ETL 和 CDC 流程,都是在修修补补这道裂痕。 如果 PostgreSQL 在保留其 OLTP 优势的同时,能嫁接上 DuckDB 的 OLAP 性能,企业还有必要再维护一套专门的分析数据库吗?
这不仅意味着**“降本增效”**,更可能带来工程层面的简化:无需再为数据迁移和多数据库同步烦恼,也无需支付昂贵的双套维护成本。 一旦有人把这件事做得足够好,就会像“一颗深水炸弹”般冲击现在大数据分析的市场格局。
PostgreSQL 被视为“数据库界的 Linux 内核” —— 它的开源精神和极强的可扩展性允许无限可能。 而我们目睹着 PostgreSQL 使用这种方式,依次占领了地理信息,时序数据,NoSQL文档,向量嵌入等领域。 而现在,它正准备向最后也是最大的生态位 —— OLAP 分析领域发起冲击。
只要一个优秀的集成方案出现,能让 DuckDB 提供的高性能分析与 PostgreSQL 的生态完美融合,整个大数据分析领域或许会因此大变天。 那些专门的 OLAP 产品,能否顶住这场“核爆级”冲击?是否会像那些 “专用向量数据库” 一样凋零, 现在没人愿意给出答案,但我相信到时候大家都会有自己的判断。
为PG与DuckDB缝合铺好路
PostgreSQL OLAP 生态的现状,正如同两年前的向量数据库插件一样,还处于早期阶段。 但这已经足够令人兴奋:新技术的魅力就在于,你能预见它的潜力,就能率先拥抱它的爆发。
两年前 PGVECTOR 爆火前夜,Pigsty 是第一波将其整合的 PostgreSQL 发行版,也是我将它提入 PGDG 官方扩展仓库的。 而这场 DuckDB 缝合大赛,干脆就是我点的火。能点燃这样的赛事,我自然也会在这场新的赛事中,提前为 PG 与 DuckDB 的融合铺好路。
作为一名深耕数据库领域的从业者,我正在把当前各家与 DuckDB 融合的 PostgreSQL 插件 全部打包成可直接安装使用的 RPM 或 DEB 包,并在主流的十个 Linux 发行版上提供, 与 PGDG 官方内核完全兼容,开箱即用,让普通用户也能轻松体验“DuckDB + PG”融合的威力。 最重要的是,提供一个缝合大赛的“竞技舞台”,让所有玩家都平等参与进来。
诚然,现在不少插件仍处于早期阶段,稳定性和功能尚未达到“企业级”的标准。也有很多细节待完善,比如并发访问控制、数据类型兼容、算子优化等。 机会总是属于勇于拥抱新技术的人,而我相信,这场“PG + DuckDB”融合的大戏,将会是数据库领域的下一个风口。
真正的爆炸即将开始
比起当下 AI 大模型落地在企业应用中仍显“虚浮”的热潮,OLAP 大数据分析 是一块更为庞大、也更现实的市场蛋糕。 而 PostgreSQL 与 DuckDB 的融合 则有望成为冲击这个市场的板块运动,引发行业格局的深度变革,甚至彻底打破“大数据系统 + 关系型数据库”两套架构并行的局面。
或许再过几个月到一两年的时间,我们就能看到一个或多个“融合怪物”从这些实验性项目中脱颖而出,成为数据库的新主角。 谁能先解决好扩展的易用性、集成度和性能优化,谁就能在这场新竞争中夺得先机。
对于数据库行业而言,这将是一次“火星撞地球”般的深度震荡;对于广大企业用户而言,或许会迎来一场“降本增效”的巨大机遇。 让我们拭目以待这场新趋势如何加速成长,并为数据分析与管理带来新的可能。
对比Oracle与PostgreSQL事务系统
事务系统是关系型数据库的核心组成部分,在应用开发中,为确保 数据完整性 提供了重要支持。 SQL 标准规范了数据库事务的一些功能,但并未明确规定许多细节。因此,关系型数据库的事务系统可能存在显著差异。
近年来,许多人尝试从 Oracle 数据库迁移到 PostgreSQL。为了顺利将应用从 Oracle 迁移到 PostgreSQL,理解两者事务系统之间的差异至关重要。 否则,您可能会遇到一些令人头痛的意外情况,危及到性能和数据完整性。所以,我认为有必要编写一篇文章,对比 Oracle 和 PostgreSQL 事务系统的特性。
ACID:数据库事务提供的服务
这里的 ACID 不是什么化学或药品术语,而是以下四个词的首字母缩写:
- Atomicity(原子性):保证在单个数据库事务中,所有语句作为一个整体执行,要么全部成功,要么全部不生效。这应涵盖所有类型的问题,包括硬件故障。
- Consistency(一致性):保证任何数据库事务都不会违反数据库中定义的约束。
- Isolation(隔离性):保证并发运行的事务不会导致某些“异常”(即数据库中一些不可由串行执行的事务产生的可见状态)。
- Durability(持久性):保证一旦数据库事务提交(完成),即使发生系统崩溃或硬件故障,事务也无法被撤销。
接下来,我们将详细讨论这些类别。
Oracle 与 PostgreSQL 事务的相似之处
首先,描述一下 Oracle 和 PostgreSQL 在事务管理中相同的部分是有帮助的。幸运的是,许多重要的特性都属于这一类:
- 两个数据库系统都使用多版本并发控制(MVCC):读取和写入操作互不阻塞。读取操作会读取旧数据,而在更新或删除事务进行时,不会阻塞读取。
- 两个数据库系统都在事务结束前保持锁定。
- 两个数据库系统都将 行锁 保存在行本身,而不是在锁表中。因此,锁定一行可能会导致额外的磁盘写入,但不需要进行 锁升级。
- 两个数据库系统都支持
SELECT ... FOR UPDATE
进行显式的并发控制。更多关于差异的讨论,后面会说。 - 两个数据库系统都使用
READ COMMITTED
作为默认的事务隔离级别,这在两个系统中的行为非常相似。
原子性对比
在这两个数据库中,原子性有一些微妙的差异:
自动提交
在 Oracle 中,任何 DML 语句会隐式启动一个数据库事务,除非已经有一个事务处于开启状态。您必须显式地使用 COMMIT
或 ROLLBACK
来结束这些事务。没有特定的语句来启动一个事务。
而 PostgreSQL 则处于 自动提交模式:除非您显式启动一个多语句事务(通过 START TRANSACTION
或 BEGIN
),每个语句都会在自己的事务中运行。在此类单语句事务结束时,PostgreSQL 会自动执行 COMMIT
。
许多数据库 API 允许您关闭自动提交。由于 PostgreSQL 服务器不支持禁用自动提交,客户端通过适当的时候自动发送 BEGIN
来模拟这一点。使用这样的 API,您无需担心这种差异。
语句级回滚
在 Oracle 中,导致错误的 SQL 语句不会中止事务。相反,Oracle 会回滚失败语句的效果,事务仍然可以继续。要回滚整个事务,您需要处理错误并主动调用 ROLLBACK
。
而在 PostgreSQL 中,如果事务中的 SQL 语句发生错误,整个事务会被中止。直到您使用 ROLLBACK
或 COMMIT
(两者都会回滚事务)结束事务时,所有后续的语句都会被忽略。
大多数编写良好的应用程序不会遇到这个差异的问题,因为通常情况下,当发生错误时,您会希望回滚整个事务。 然而,PostgreSQL 的这种行为在某些特定情况下可能会令人烦恼:想象一个长时间运行的批处理任务,其中坏数据可能会导致错误。 您可能希望能够处理错误,而不是回滚已经完成的所有操作。在这种情况下,您应该在 PostgreSQL 中使用(符合 SQL 标准的)保存点。 请注意,您应谨慎使用保存点:它们是通过 子事务实现的,可能会严重影响性能。
事务性DDL
在 Oracle 数据库中,任何 DDL 语句会自动执行 COMMIT
,因此 无法回滚 DDL 语句。
在 PostgreSQL 中则没有这种限制。除了少数例外(如 VACUUM
、CREATE DATABASE
、CREATE INDEX CONCURRENTLY
等),您可以 回滚任何 SQL 语句。
一致性对比
在这一领域,Oracle 和 PostgreSQL 之间差异不大;两者都会确保事务不违反约束。
或许值得一提的是,Oracle 允许您使用 ALTER TABLE
启用或禁用约束。例如,您可以禁用约束,执行违反约束的数据修改操作,然后使用 ENABLE NOVALIDATE
启用约束(对于主键和唯一约束,只有在它们是 DEFERRABLE
时才有效)。
而在 PostgreSQL 中,只有超级用户才能禁用实现外键约束以及可推迟唯一和主键约束的触发器。设置 session_replication_role = replica
也是一个禁用此类触发器的方式,但同样需要超级用户权限。
主键和唯一约束在 Oracle 和 PostgreSQL 中的验证时机
以下 SQL 脚本在 Oracle 中不会报错:
CREATE TABLE tab (id NUMBER PRIMARY KEY);
INSERT INTO tab (id) VALUES (1);
INSERT INTO tab (id) VALUES (2);
COMMIT;
UPDATE tab SET id = id + 1;
COMMIT;
在 PostgreSQL 中,同样的脚本会报错:
CREATE TABLE tab (id numeric PRIMARY KEY);
INSERT INTO tab (id) VALUES (1);
INSERT INTO tab (id) VALUES (2);
UPDATE tab SET id = id + 1;
ERROR: duplicate key value violates unique constraint "tab_pkey"
DETAIL: Key (id)=(2) already exists.
原因在于,PostgreSQL 默认在每行变化时检查约束(不同于SQL标准),而 Oracle 在语句结束时检查约束。
不过这个问题可以通过将约束创建为 DEFERRABLE
来解决,这样 PostgreSQL 会在语句结束时检查约束,并与 Oracle 的行为保持一致。
隔离性对比
这是 Oracle 和 PostgreSQL 差异最明显的领域。Oracle 对事务隔离的支持相对有限。
事务隔离级别的对比
SQL 标准定义了四个事务隔离级别:READ UNCOMMITTED
、READ COMMITTED
、REPEATABLE READ
和 SERIALIZABLE
。
但与标准的详细程度相比,单独的级别定义得比较模糊。例如,标准提到,“脏读”(读取其他事务未提交的数据)在 READ UNCOMMITTED
隔离级别下是“可能”的,但并没有明确指出这是否为必需。
Oracle 只提供 READ COMMITTED
和 SERIALIZABLE
隔离级别。然而后者其实并不完全准确;Oracle 提供的是快照隔离。例如,以下并发事务均会成功(第二个会话如下所示):
CREATE TABLE tab (name VARCHAR2(50), is_highlander NUMBER(1) NOT NULL);
-- start a new serializable transaction
SET TRANSACTION ISOLATION LEVEL SERIALIZABLE;
SELECT count(*) FROM tab WHERE is_highlander = 1;
COUNT(*)
----------
0
-- start a new serializable transaction
SET TRANSACTION ISOLATION LEVEL SERIALIZABLE;
SELECT count(*) FROM tab WHERE is_highlander = 1;
COUNT(*)
----------
0
-- the count is zero, so let's proceed
INSERT INTO tab VALUES ('MacLeod', 1);
COMMIT;
-- the count is zero, so let's proceed
INSERT INTO tab VALUES ('Kurgan', 1);
COMMIT;
如果这些事务串行执行,第二个事务的结果应该是 count 为 1。
除了不准确,Oracle 的实现还存在许多问题。例如,如果您创建一个表时未指定 SEGMENT CREATION IMMEDIATE
,然后在 SERIALIZABLE
事务中尝试插入第一行,就会遇到序列化错误。
虽然这在技术上是合法的,但如果在更高的隔离级别遇到问题时,Oracle 会经常抛出序列化错误。
PostgreSQL 支持所有四个隔离级别,但它会默默地将 READ UNCOMMITTED
升级为 READ COMMITTED
(这在 SQL 标准中可能并不符合要求)。
而 SERIALIZABLE
事务则是真正的串行化事务。PostgreSQL 的 REPEATABLE READ
行为类似于 Oracle 的 SERIALIZABLE
,但实际上 PostgreSQL 的实现更好。
READ COMMITTED
级别下并发数据修改的对比
默认的事务隔离级别 READ COMMITTED
是一个低隔离级别,这意味着许多异常仍然可能发生。
我在之前的文章中描述了其中的一种异常:事务异常与 SELECT FOR UPDATE
。简而言之,情况如下:
- 一个事务修改了表中的一行,但尚未提交
- 第二个事务执行了一个锁定行的语句(例如
SELECT ... FOR UPDATE
),并且挂起 - 第一个事务提交
在这种情况下,两个数据库系统会有什么结果?在 Oracle 和 PostgreSQL 中,您都能看到最新提交的数据,但细节有所不同:
- PostgreSQL 只重新评估被锁定的行,操作较快,但可能会导致不一致的结果
- Oracle 会 重新执行完整查询,尽管速度较慢,但能够提供一致的结果
持久性对比
两个数据库系统都通过事务日志实现持久性(Oracle 中为“REDO 日志”,PostgreSQL 中为“WAL日志”)。在这一领域,Oracle 和 PostgreSQL 提供的保证是相同的。
其他事务差异
事务的大小和持续时间限制
这一领域的差异主要源于 Oracle 和 PostgreSQL 实现多版本并发控制(MVCC)的方式不同。Oracle 使用 UNDO 表空间 来存储已修改行的旧版本,而 PostgreSQL 将多个版本的行存储在表中。
由于这个原因,Oracle 事务中数据修改的数量受限于 UNDO 表空间的大小。对于大批量删除或更新,Oracle 通常会采用分批处理并在每批之间执行 COMMIT
。
而在 PostgreSQL 中没有这种限制,但大规模更新会导致表膨胀,因此您也可能希望分批更新,并在更新间运行 VACUUM
。然而在 PostgreSQL 中,并没有理由限制大批量删除的规模。
长时间运行的事务在任何关系型数据库中都是一个问题,因为它们会占用锁并增加阻塞其他会话的几率,长事务也更容易遭遇死锁。 在 PostgreSQL 中,长事务会比 Oracle 更加棘手一些,因为它们还会阻塞“自动清理”(autovacuum)任务的进程,从而导致表膨胀,治理起来要费些事。
SELECT ... FOR UPDATE
的对比
两个数据库系统都知道这个命令,它用于同时读取并锁定一行。Oracle 和 PostgreSQL 都支持 NOWAIT
和 SKIP LOCKED
子句。
PostgreSQL 缺少 WAIT <integer>
子句,但是可以通过动态调整 lock_timeout
参数实现类似的功能。
这里最重要的区别在于,PostgreSQL 中如果你打算更新某一行,FOR UPDATE
并非 合适的语句 ——
除非你打算删除某行或修改主键或唯一键列,否则正确的锁定模式应为 FOR NO KEY UPDATE
。
事务ID回卷
事务ID回卷 只在 PostgreSQL 中存在。 PostgreSQL 的多版本控制通过在每一行中存储 事务ID 来管理行版本的可见性。
这些编号来自一个 32 位整型计数器,最终会发生回卷。
所以 PostgreSQL 需要执行维护操作(FREEZE
)来避免出现事务ID回卷。在高事务量(TPS)的系统中,这可能成为一个需要特别关注和调整的问题。
结论
在大多数方面,Oracle 和 PostgreSQL 的事务行为非常相似。但它们之间确实存在差异,如果您计划迁移到 PostgreSQL,了解这些差异是很重要的。本文中的对比有助于您在迁移过程中识别潜在的问题。
老冯评论
在 PostgreSQL 17 发布:摊牌了,我不装了! 中提到过,在最近一年,PostgreSQL 社区在心态与精神上有了显著的转变: 不再采用过去佛系与世无争的姿态,而是转变为一种积极进取的姿态,它已经做好了接管与征服整个数据库世界的心理建设与准备。喊出了干翻“顶级商业数据库”(Oracle)的口号。
现在看来,PostgreSQL 社区确实出息了,前有 EDB TPC-C 评测炮打 Oracle 性能,现在又有 Cybertec 点评 Oracle 事务正确性,开始炮轰 Oracle 了。
就比如这篇文章吧,看上去很中立的说了一堆事务系统的对比,也很客观的指出了 PG 和 Oracle 存在的问题。 但实际上在 ACID 的 ACD 上大家都是大同小异,真正的重点是在事务隔离等级上 —— Oracle 的缺陷实现。
是的,号称顶级商业数据库的 Oracle,Serializable 的隔离等级其实是虚标,实际上是 SI 快照隔离。 这个问题其实我在 MySQL的正确性为何如此拉垮? 中已经提到了。
在主流 DBMS 中,只有 PostgreSQL (以及基于PostgreSQL的CockroachDB)提供了真正的 Serializable 。
数据库即业务架构
数据库是业务架构的核心,是不言自明的共识。但如果我们更进一步,将数据库作为架构本身,又会发生什么? 未来会是一个数据库吞噬后端,前端,操作系统,甚至一切的世界吗?
数据库是架构的核心
“If you show me your software architecture, I learn nothing about your business. But if you show me your data model, I can guess exactly what your business is.”。 —— Michael Stonebraker
数据库祖师爷迈克·石破天有句名言:“如果你给我看软件架构,我对你的业务一无所知;但如果你给我看数据模型,我就能精准知道你的业务是干嘛的”
无独有偶,微软 CEO 纳德拉 也在最近公开表示:我们今天所称的软件,你喜欢的那些应用程序,不过是包装精美的数据库操作界面而已。
即使在 GenAI / LLM 爆火的当下,绝大多数信息系统依然是以数据库为核心设计。所谓的分库分表,几地几中心,异地多活这些架构花活,说到底也就是数据库的不同使用方式罢了。
数据库是业务架构的核心,早已是不言自明的共识。但如果我们更进一步,将数据库作为业务架构本身,又会如何?
驱动未来的数据库
不久之前,我邀请 Omnigres 创始人 Yurii 到 第七届PG生态大会 上进行分享。 他在题为《数据库驱动未来》 的演讲中抛出了一个有趣的观点 —— 数据库就是业务架构。(DBaaA, Database as an Architecture)
具体来说,他的开源项目做了一件“疯狂”的事:把所有应用逻辑,甚至 Web Server 都塞进 PostgreSQL 数据库。 不只是后端包个REST接口中间件这种形式,而是把前后端都整个塞进 PG 里去了!
CREATE EXTENSION omni_httpd CASCADE;
CREATE EXTENSION omni_vfs CASCADE;
CREATE FUNCTION mount_point()
returns omni_vfs.local_fs language sql AS $$SELECT omni_vfs.local_fs('/www')$$;
UPDATE omni_httpd.handlers
SET query = (SELECT omni_httpd.cascading_query(name, query) FROM
(SELECT * FROM omni_httpd.static_file_handlers('mount_point', 0,true)) routes);
几行 SQL 就能把 PostgreSQL 变成一个能跑在 8080 端口的 ‘Nginx’,并且还能用数据库函数编写业务逻辑,实现 HTTP API。 看到这种神奇玩法,我一度怀疑这玩意到底能不能行。但事实就是它居然跑了起来,还真挺像那么回事。
除了这里的 httpd
扩展外,Omnigres 还提供了另外 33 个扩展插件,
这套扩展全家桶,提供了在 PostgreSQL 中进行完整 Web 应用开发的能力!
类似 PostgREST 这样的工具,可以将设计良好的 PostgreSQL 模式直接转化为开箱即用的 RESTful API。 而 Omnigres 这样的工具则是百尺竿头更进一步,直接让 HTTP 服务器运行在了 PG 数据库内部!而这意味着,你不仅可以把后端放进数据库里,你甚至可以把前端也放进数据库中!
但这真的会是一个好主意吗?还是要看具体情况。 互联网企业出身的架构师或者数据库 DBA 看到这种玩法可能会大喊:“这也太离经叛道了!” 但是也不要着急下结论,我还真的看到过这种开发范式取得过成功。
DBaaA的利弊权衡
早先我在探探时(一个瑞典团队在中国运营的约会应用,Tinder in China),我们深度利用了 PostgreSQL:那时候我们所有的业务逻辑(包括一个 100ms 的推荐算法)都是 PG 存储过程。 后端应用只是一个 Go 写的非常轻薄一层转发代理,虽然没有把 Web Server 放到 PostgreSQL 中,但也基本差不多了。
靠着这种单一 PostgreSQL 选型的架构,我们在没有使用任何其他数据库(我们确实用了对象存储)的情况下,一路干到了在四百万日活,与 2.5M 数据库 QPS。 而建设这一切的只有三位工程师。其实有点儿像 Instagram,因为其实我们就是仿照着 Instagram 的架构搞的。 将业务逻辑,甚至业务架构整个放入数据库中(以下简称为 DBaaA)优点与缺点都非常明显。
这种玩法的优点在于出活快,架构简单、部署敏捷、延迟性能好,开发成本低:当你设计好 PostgreSQL 数据库模式后,用 PostgREST + Kong 几行配置就能自动生成后端,一套服务新鲜出炉。 很多管理操作可以用纯SQL完成,比如 CI/CD/发布/迁移/降级等;比如,要实现一个断路器,执行 SQL 刷新存储过程逻辑,提前返回即可。 架构设计与部署交付上非常简单,一个数据库 + 一个无状态的网关,复制一份数据目录,就是一套新的部署。 而且出乎意料的是,这种实践的性能并不差 —— 因为存储过程可以有效节省交互式查询多次网络往返的开销, 而内置的web服务器也省下了额外的交互,所以在响应时间/延迟(RT/Latency)表现上反而相当出色。
但这种架构的劣势也同样非常显著:数据库的吞吐确实会因为承担了更多计算逻辑而下降, 而在 2017 年那会的硬件性能还没有现在发达,数据库通常是整个架构中的性能瓶颈,单节点动辄大几万 TPS,确实没有多少性能余量给这些花活。
而比起吞吐量上的问题,人力的挑战更为棘手,而随着创始骨干的离开,编写存储过程开始成为一门逐渐失传的高深技术 —— 新员工不会写了!
所以我们最后还是逐渐将这些存储过程抽离出来在外部应用中实现,也开始使用 Redis 以及其他数据库给 PG 打辅助,但那已经是被收购之后的事了。 不过现在回头再看看,如果是在 2025 年的当下,我认为会有完全不一样的选择:因为无论是软件还是硬件都已经发生了翻天覆地的变化,而这些变化带来了全新的利弊权衡。
分久必合的架构钟摆
俗话说:“分久必合,合久必分”。在上古时期,许多应用使用的也是这种简单的 C/S,B/S 架构,几个客户端直接读写数据库。 但是后来,随着业务逻辑的复杂化,以及硬件性能(相对于业务需求)的捉襟见肘,许多东西从数据库中被剥离出来,形成了传统的三层架构,以及越来越多的组件。
但现在时过境迁,GPT 已经达到了能够熟练编写存储过程的中高级开发者的水准,而遵循摩尔定律发展的硬件直接把单机性能推到了一个匪夷所思的地步。 那么拆分剥离的趋势也很有可能会逆转,原本从数据库中分离出去的业务逻辑,又会重新回到数据库中。 将业务逻辑放到数据库中,甚至让数据库成为整个业务架构本身,可能重新成为一种流行的实践。
实际上,我们已经可以在《PostgreSQL吞噬数据库世界》,以及社区正在流行的 “一切皆用 PostgreSQL” 口号中,观察到观察到数据库领域正在出现的收敛趋势: 原先从数据库中分离出去的细分领域专用数据库,如全文搜索、向量,机器学习、图数据库、时序数据库等,现在都在重新以插件超融合的方式回归到 PostgreSQL 中。
相应地,前端,后端重新融合回归到数据库的实践也开始出现。我认为一个非常值得注意的例子是 Supabase,一个号称 “开源 Firebase” 的项目,据说 80% YC 创业公司都在使用它。 它将 PostgreSQL,对象存储,PostgREST,EdgeFunction 和各种工具封装成为一整个运行时,然后将后端与传统意义上的数据库整体打包成为一个 “新的数据库”。
Supabase 实际上就是 “吃掉” 了后端的数据库,如果这种架构走到极致,那大概会是像 Omnigres 这样的架构 —— 一个运行着 HTTP 服务器的 PostgreSQL,干脆把前端也吃下去了。
当然,可能还有更为激进的尝试 —— 例如 Stonebraker 老爷子的新创业项目 DBOS,甚至要把操作系统也给吞进数据库里去了!
这也许意味着软件架构领域的钟摆正在重新回归简单与常识 —— 前端绕开花里胡哨的中间件,直接访问数据库,螺旋上升回归到最初的 C/S,B/S 架构上去。 或者像纳德拉所说,Agent 可以直接绕过中间商,代替前后端与软件去读写数据库,出现一种新的 A(gent)/D(atabase) 架构也未尝不可。
拆分与合并的利弊权衡
在当下,
然而,吞吐和人力,这两个核心障碍在 2025 年的当下,已经被软硬件告诉
有机会规避一些复杂的并发争用,并通过节省了后端与数据库的网络 RT 带来更好的延迟性能表现; 而且在管理上也会有一些独特优势:所有业务逻辑、模式定义和数据都在同一个地方,用同样的方式处理,你的 CI/CD,发布/迁移/降级都可以用SQL实现。 你想部署一套新系统?把 PostgreSQL 数据目录复制一份,重新拉起一个 PostgreSQL 实例就可以了。一个数据库解决所有问题,架构简单无比。
而主要缺点在于人员要求高,运维复杂,最大吞吐下降。
类似 PostgREST 这样的工具,可以将设计良好的 PostgreSQL 模式直接转化为开箱即用的 RESTful API。 而 Supabase 这样的 BaaS 更是在其基础上将整个后端都变成了一项公用服务 —— 这意味着你只要设计好数据库模型,基本就没有什么后端开发的工作了。 Omnigres 这样的工具则是百尺竿头更进一步,直接让 HTTP 服务器运行在了 PG 数据库内部!而这意味着,你不仅可以把后端放进数据库里,你甚至可以把前端也放进数据库中!
这样做在管理上也有一些独特优势:所有业务逻辑、模式定义和数据都在同一个地方,用同样的方式处理,你的 CI/CD,发布/迁移/降级都可以用SQL实现。 你想部署一套新系统?把 PostgreSQL 数据目录复制一份,重新拉起一个 PostgreSQL 实例就可以了。一个数据库解决所有问题,架构简单无比。
使用 PostgreSQL 作为业务架构,你可以
由数据库处理业务逻辑,可以通过避免交互式查询的多次网络RT来提高查询(延迟)性能,但是消耗数据库服务器的 CPU 资源会导致最大吞吐量下降。 将业务逻辑作为 SQL / 存储过程会带来一些管理上的优势:用统一的方式管理代码与数据,CI/CD,发布/迁移/降级都可以用SQL实现,高度集成,非常灵活; 但也会带来新的挑战:在数据库里对模式变更,版本控制,备份恢复都是全新的玩法,一般的开发者和DBA恐怕并没有能力兜住这种“激进”的玩法。
有机会规避一些复杂的并发争用,并通过节省了后端与数据库的网络 RT 带来更好的延迟性能表现; 而且在管理上也会有一些独特优势:所有业务逻辑、模式定义和数据都在同一个地方,用同样的方式处理,你的 CI/CD,发布/迁移/降级都可以用SQL实现。 你想部署一套新系统?把 PostgreSQL 数据目录复制一份,重新拉起一个 PostgreSQL 实例就可以了。一个数据库解决所有问题,架构简单无比。
时将几乎所有的业务逻辑(甚至推荐算法)都在 PostgreSQL 里实现,后端只有很轻薄的一层转发。 只不过这种做法对开发者、DBA 的综合技能要求较高 —— 毕竟写存储过程、维护复杂的数据库逻辑不是一件轻松活儿。
老实说 DBA 和运维很难喜欢这些看上去 “离经叛道” 的玩意。但作为一个开发者,特我认为这个主意非常有趣,值得探索!
吞噬一切的数据库
俗话说:“分久必合,合久必分”。在上古时期,许多 C/S,B/S 架构的应用就是几个客户端直接读写数据库。 但是后来,随着业务逻辑的复杂化,以及硬件性能(相对于业务需求)的捉襟见肘,许多东西从数据库中被剥离出来,形成了传统的三层架构。
硬件的发展让数据库服务器的性能重新出现大量的富余,而数据库软件的发展让存储过程的编写变得更加容易, 那么拆分剥离的趋势也很有可能会逆转,原本从数据库中分离出去的业务逻辑,又会重新回到数据库中。
拥抱新趋势
如果你想试试在数据库里写应用,一套 PostgreSQL 打天下的刺激玩法,确实应该尝试一下 Supabase 或者 Omnigres! 我们最近实现了了在本地自建 Supabase 的能力(这涉及到二十多个扩展,其中有几个棘手的扩展使用Rust编写), 并在刚刚提供了对 Omnigres 扩展的支持 —— 这为 PostgreSQL 提供了 DBaaA 的能力。
如果你有几十万 TPS,几十 TB 的数据,或者运行着一些至关重要、人命关天、硕大无朋的核心系统,那么这种玩法可能不太合时宜。 但如果你运行的是一些个人项目,小网站,或者是初创公司与边缘创新系统,那么这种架构会让你的迭代更为敏捷,开发、运维更加简单。
当然不要忘记,除了二十多种可以用于编写存储过程的的语言支持外,PostgreSQL 生态中还有 1000+ 扩展插件可以提供各种强力功能。
除了大家都已经耳熟能详的 postgis
, timescaledb
, pgvector
, citus
之外,最近还有许多亮眼的新兴扩展:
比如在 PG 上提供 ClickHouse T0 分析性能的 pg_duckdb
与 pg_mooncake
,提供比肩 ES 全文检索的 pg_search
,
将 PG 转换为 S3 湖仓的 pg_analytics
与 pg_parquet
,……
我们将在 OLAP 领域再次见证一个类似 pgvector
的玩家出现,让许多 “大数据” 组件变成 Punchline。
而这正是我们 Pigsty 想要解决的问题 —— Extensible Postgres,让所有人都可以轻松使用这些扩展插件,让 PostgreSQL 成为一个真正的超融合数据库全能王。
在我们开源的 Pigsty 扩展仓库中,总共已经提供了将近 400 个开箱即用的扩展,你可以在主流Linux系统(amd/arm, EL 8/9, Debian 12, Ubuntu 22/24),使用 Pigsty 一键安装这些扩展。 但这些插件是一个可以独立使用的仓库(Apache 2.0),您非一定要使用 Pigsty 才能拥有这些扩展 —— Omnigres 与 AutoBase 这样的 PostgreSQL 也在使用这个仓库进行扩展交付,这确实是一个开源生态互惠共赢的大好例子。 如果您是 PostgreSQL 供应商,我们非常欢迎您使用 Pigsty 的扩展仓库作为上游安装源,或在 Pigsty 中分发您的扩展插件。
如果您是 PostgreSQL 用户,并对扩展插件感兴趣,也非常欢迎看一看我们开源的 PG 包管理器 [pig
] ,可以让您一键轻松解决 PostgreSQL 扩展插件的安装问题。
什么,还能这么玩
在 PG 生态大会上,尤里展示了一个想法:把所有业务逻辑,甚至是Web服务器和整个后端都塞进 PostgreSQL 数据库里。 比如可以通过写存储过程,把原本后端功能直接放到数据库里运行。为此,他还以 PG 扩展的形式实现了许多 “标准库”,从 http, vfs, os 到 python 模块。
让我们来看一个有趣的例子,在 PostgreSQL 中执行以下 SQL,将会启动一个 Web 服务器,将 /www
作为一个 Web 服务器的根目录对外提供服务。
CREATE EXTENSION omni_httpd CASCADE;
CREATE EXTENSION omni_vfs CASCADE;
CREATE EXTENSION omni_mimetypes CASCADE;
create function mount_point()
returns omni_vfs.local_fs language sql AS
$$select omni_vfs.local_fs('/www')$$;
UPDATE omni_httpd.handlers
SET query = (SELECT omni_httpd.cascading_query(name, query order by priority desc nulls last)
from (select * from omni_httpd.static_file_handlers('mount_point', 0,true)) routes);
是的,天啊,PostgreSQL 数据库竟然拉起来了一个 HTTP 服务器,默认跑在 8080
端口!你可以把它当成 Nginx 用!
当然,你可以选择任意你喜欢的编程语言来创建 PostgreSQL 函数,并将这些函数挂载到 HTTP 端点上,实现你想要的任何逻辑。
如果是熟悉 Oracle 的用户可能会发现,这有点类似于 Oracle Apex。但在 PostgreSQL 中,你可以用二十多种编程语言来开发存储过程,而不仅仅局限于 PL/SQL!
除了这里的 httpd
扩展外,Omnigres 还提供了另外 33 个扩展插件,这套扩展全家桶,提供了在 PostgreSQL 中进行完整 Web 应用开发的能力!
BTW 他还说 SaaS is Dead:因为以后 Agent 可以直接绕过中间商,代替前后端去读写数据库
这意味着,你可以把一个经典前端-后端-数据库三层架构的应用,完整地塞入一个数据库中!
当然,你可以选择任意你喜欢的编程语言来创建 PostgreSQL 函数,并将这些函数挂载到 HTTP 端点上,实现你想要的任何逻辑。
他是怎么做到的?Omnigres 提供了一套扩展全家桶,包括 httpd
、vfs
、os
、python
等33个 PG “标准库”扩展模块。
从浏览器可以访问 HTML 页面,而 HTML 页面可以通过 Javascript 动态访问 HTTP 服务器与数据库存储过程。
将业务逻辑,Web Server,甚至是整个前后端都放入数据库中,又会擦出怎么样的火花?
这个想法的实质是:把所有业务逻辑,甚至是Web服务器和整个前后端都塞进 PostgreSQL 数据库里。 让我们来看一个有趣的例子,在 PostgreSQL 中执行以下 SQL,将会启动一个 Web 服务器,将
/www
作为一个 Web 服务器的根目录对外提供服务: 天啊,PostgreSQL 数据库竟然拉起来了一个 HTTP 服务器,默认跑在8080
端口!你可以把它当成 Nginx 用! 除了实现 httpd 之外,他还以 PG 扩展的形式实现了许多 “标准库”,这包括一个由 33 个扩展插件组成的全家桶,提供在 PostgreSQL 中进行完整 Web 应用开发的能力!
熟悉 Oracle 的用户可能会发现,这有点类似于 Oracle Apex。但在 PostgreSQL 中,你可以用二十多种编程语言来开发存储过程,而不仅仅局限于 PL/SQL!
但如果你的性能主要指的是 “最大TPS吞吐量”,那么
但比起吞吐性能下降,这种实践不受待见的一个核心原因是,它对人的要求太高了 —— 这种实践通常只有精英小团队才能玩转。 你要会写存储过程,以及更重要的,你要知道在这种范式下如何实现各种各样的管理操作(PITR,备份恢复,高可用,CI/CD,模式变更,版本控制)。 而拥有这些经验的人要比拥有常规实践经验的人要稀少得多。
吞吐和人力,是这种范式的最大困难。
七周七数据库(2025年)
作者:Matt Blewitt,原文:七周七数据库(2025年)
译者:冯若航,数据库老司机,云计算泥石流
https://matt.blwt.io/post/7-databases-in-7-weeks-for-2025/
长期以来,我一直在运营数据库即服务(Databases-as-a-Service),这个领域总有新鲜事物需要跟进 —— 新技术、解决问题的不同方法,更别提大学里不断涌现的研究成果了。展望2025年,考虑花一周时间深入了解以下每项数据库技术吧。
前言
这不是 “七大最佳数据库” 之类的文章,更不是给报菜单念书名式的列表做铺垫——这里只是我认为值得你花一周左右时间认真研究的七个数据库。你可能会问,“为什么不选Neo4j、MongoDB、MySQL / Vitess 或者其他数据库呢?”答案大多是:我觉得它们没啥意思。同时,我也不会涉及 Kafka 或其他类似的流数据服务——它们确实值得你花时间学习,但不在本文讨论范围内。
目录
1. PostgreSQL
默认数据库
“一切皆用 Postgres” 几乎成了一个梗,原因很简单。PostgreSQL 是 枯燥技术 的巅峰之作,当你需要 客户端-服务器 模型的数据库时,它应该是你的首选。PG 遵循ACID原则,拥有丰富的复制方法 —— 包括物理和逻辑复制—— 并且在所有主要供应商中都有极好的支持。
然而,我最喜欢 Postgres 功能是 扩展。在这一点上,Postgres 展现出了其他数据库难以企及的生命力。几乎你想要的功能都有相应的扩展——AGE支持图数据结构和Cypher查询语言,TimescaleDB支持时间序列工作负载,Hydra Columnar提供了另一种列式存储引擎,等等。如果你有兴趣亲自尝试,我最近写了一篇关于编写扩展的文章。
正因为如此,Postgres 作为一个优秀的 “默认” 数据库熠熠生辉,我们还看到越来越多的非 Postgres 服务使用 Postgres 线缆协议 作为通用的七层协议,以提供客户端兼容性。拥有丰富的生态系统、合理的默认行为,甚至可以用 Wasm 跑在浏览器中,这使得它成为一个值得深入理解的数据库。
花一周时间了解 Postgres 的各种可能性,同时也了解它的一些限制 ——MVCC 可能有些任性。用你最喜欢的编程语言实现一个简单的CRUD应用程序,甚至可以尝试构建一个 Postgres 扩展。
2. SQLite
本地优先数据库
离开客户端-服务器模型,我们绕道进入 “嵌入式” 数据库,首先介绍 SQLite。我将其称为“本地优先”数据库,因为SQLite数据库与应用程序直接共存。一个更著名的例子是WhatsApp,它将聊天记录存储为设备上的本地 SQLite 数据库。Signal 也是如此。
除此之外,我们开始看到更多 SQLite 的创新玩法,而不仅仅是将其当成一个本地ACID数据库。像 Litestream 这样的工具提供了流式备份的能力, LiteFS 提供了分布式访问的能力,这让我们可以设计出更有趣的拓扑架构。像CR-SQLite 这样的扩展允许使用 CRDTs,以避免在合并变更集时需要冲突解决,正如 Corrosion 的例子一样。
得益于Ruby on Rails 8.0,SQLite也迎来了一个小型复兴 ——37signals 全面投入 SQLite,构建了一系列 Rails 模块,如 Solid Queue,并通过database.yml
配置 Rails 以操作多个 SQLite 数据库。Bluesky 使用SQLite作为个人数据服务器 —— 每个用户都有自己的 SQLite 数据库。
花一周时间使用 SQLite ,探索一下本地优先架构,你甚至可以研究下是否能将使用 Postgres 的客户端-服务器模型迁移到只使用 SQLite 的模式上。
3. DuckDB
万能查询数据库
接下来是另一个嵌入式数据库,DuckDB。与SQLite类似,DuckDB旨在成为一个内嵌于进程的数据库系统,但更侧重于在线分析处理(OLAP)而非在线事务处理(OLTP)。
DuckDB 的亮点在于它作为一个“万能查询”数据库,使用 SQL 作为首选方言。它可以原生地从 CSV、TSV、JSON ,甚至像 Parquet 这样的格式中导入数据 —— 看看 DuckDB的数据源列表 支持的数据源列表吧!这赋予了它极大的灵活性 —— 不妨看看 查询Bluesky火焰管道的这个示例。
与 Postgres 类似,DuckDB 也有 扩展,尽管生态系统没有那么丰富 —— 毕竟DuckDB还相对年轻。许多社区贡献的扩展可以在社区扩展列表中找到,我特别喜欢gsheets
。
花一周时间使用DuckDB进行一些数据分析和处理——无论是通过 Python Notebook,还是像Evidence这样的工具,甚至看看它如何与SQLite的“本地优先”方法结合,将SQLite数据库的分析查询卸载到DuckDB,毕竟 DuckDB 也可以读取SQLite数据。
4. ClickHouse
列式数据库
离开嵌入式数据库领域,但继续看看分析领域,我们会遇上 ClickHouse。如果我只能选择两种数据库,我会非常乐意只用 Postgres 和 ClickHouse——前者用于OLTP,后者用于OLAP。
ClickHouse 专注于分析工作负载,并且通过横向扩展和分片存储,支持非常高的摄取率。它还支持分层存储,允许你将“热”数据和“冷”数据分开—— GitLab对此有相当详尽的文档。
当你需要在一个 DuckDB 吃不下的大数据集上运行分析查询,或者需要 “实时” 分析时,ClickHouse 会有优势。关于这些数据集已经有很多 “Benchmarketing”(打榜营销)了,所以我就不再赘述了。
我建议你了解 ClickHouse 的另一个原因是它的操作体验极佳 —— 部署、扩展、备份等都有详尽的文档——甚至包括设置 合适的 CPU Governor。
花一周时间探索一些更大的分析数据集,或者将上面 DuckDB 分析转换为 ClickHouse 部署。ClickHouse 还有一个嵌入式版本 —— chDB—— 可以提供更直接的对比。
5. FoundationDB
分层数据库
现在我们进入了这个列表中的 “脑洞大开” 部分,FoundationDB 登场。可以说,FoundationDB 不是一个数据库,而是数据库的基础组件。被 Apple、Snowflake 和 Tigris Data 等公司用于生产环境,FoundationDB 值得你花点时间,因为它在键值存储世界中相当独特。
是的,它是一个有序的键值存储,但这并不是它有趣的点。乍看它有一些奇特的限制——例如事务不能影响超过10MB 以上的数据,事务首次读取后必须在五秒内结束。但正如他们所说,限制让我们自由。通过施加这些限制,它可以在非常大的规模上实现完整的 ACID 事务—— 我知道有超过 100 TiB 的集群在运行。
FoundationDB 针对特定的工作负载而设计,并使用仿真方法试进行了广泛地测试,这种测试方法被其他技术采纳,包括本列表中的另一个数据库和由一些前 FoundationDB 成员创立的 Antithesis。关于这一部分请参阅 Tyler Neely 和 PhilEaton 的相关笔记。
如前所述,FoundationDB 具有一些非常特定的语义,需要一些时间来适应——他们的 特性 文档和 反特性 (不打算在数据库中提供的功能)文档值得去了解,以理解他们试图解决的问题。
但为什么它是“分层”数据库?因为它提出了分层的概念,而不是选择将存储引擎与数据模型耦合在一起,而是设计了一个足够灵活的存储引擎,可以将其功能重新映射到不同的层面上。Tigris Data有一篇关于构建此类层的优秀文章,FoundationDB 组织还有一些示例,如 记录层 和 文档层。
花一周时间浏览 教程,思考如何使用FoundationDB替代像 RocksDB 这样的数据库。也许可以看看一些 设计方案 并阅读 论文。
6. TigerBeetle
极致正确数据库
继确定性仿真测试之后,TigerBeetle 打破了先前数据库的模式,因为它明确表示自己 不是一个通用数据库 —— 它完全专注于金融事务场景。
为什么值得一看?单一用途的数据库很少见,而像 TigerBeetle 这样痴迷于正确性的数据库更是稀有,尤其是考虑到它是开源的。它们包含了从 NASA的十律 和 协议感知恢复 到严格的串行化和 Direct I/O 以避免内核页面缓存问题,这一切的一切真是 非常 令人印象深刻——看看他们的 安全文档 和他们称之为 Tiger Style 的编程方法 吧!
另一个有趣的点是,TigerBeetle是用 Zig 编写的——这是一门相对新兴的系统编程语言,但显然与 TigerBeetle 团队的目标非常契合。
花一周时间在本地部署的 TigerBeetle 中建模你的金融账户——按照 快速入门 操作,并看看系统架构文档,了解如何将其与上述更通用的数据库结合使用。
7. CockroachDB
全球分布数据库
最后,我们回到了起点。在最后一个位置上,我有点纠结。我最初的想法是 Valkey,但 FoundationDB 已经满足了键值存储的需求。我还考虑过图数据库,或者像 ScyllaDB 或 Cassandra 这样的数据库。我还考虑过 DynamoDB,但无法本地/免费运行让我打消了这个想法。
最终,我决定以一个全球分布式数据库结束 —— CockroachDB。它兼容 Postgres 线缆协议,并继承了前面讨论的一些有趣特性——大规模横向扩展、强一致性——还拥有自己的一些有趣功能。
CockroachDB 实现了跨多个地理区域的数据库伸缩能力,生态位与 Google Spanner 系统重叠,但 Spanner 依赖原子钟和GPS时钟进行极其精确的时间同步,然而普通硬件没有这样的奢侈配置,因此 CockroachDB 有一些巧妙的解决方案,通过重试或延迟读取以应对 NTP 时钟同步延迟,节点之间还会比较时钟漂移,如果超过最大偏移量则会终止成员。
CockroachDB 的另一个有趣特性是如何使用多区域配置,包括表的本地性,根据你想要的读写利弊权衡提供不同的选项。花一周时间在你选择的语言和框架中重新实现 movr
示例吧。
总结
我们探索了许多不同的数据库,这些数据库都被地球上一些最大的公司在生产环境中使用,希望这能让你接触到一些之前不熟悉的技术。带着这些知识,去解决有趣的问题吧!
老冯评论
在 2013 年有一本书叫《七周七数据库》。那本书介绍了当时的 7 种 “新生(或者重生)” 的数据库技术,给我留下了印象。12 年后,这个系列又开始有更新了。
回头看看当年的七数据库,除了原本的 “锤子” PostgreSQL 还在,其他的数据库都已经物是人非了。而 PostgreSQL 已经从 “锤子” 成为了 “枯燥数据库之王” —— 成为了不会翻车的 “默认数据库”。
在这个列表中的数据库,基本都是我已经实践过或者感兴趣/有好感的对象。当然 ClickHouse 除外,CK 不错,但我觉得 DuckDB 以及其与 PostgreSQL 的组合有潜力把 CK 给拱翻,再加上是 MySQL 协议兼容生态,所以对它确实没有什么兴趣。如果让我来设计这份名单,我大概会把 CK 换成 Supabase 或 Neon 中的一个。
我认为作者非常精准的把握了数据库技术发展的趋势,我高度赞同他对数据库技术的选择。实际上在这七个数据库中,我已经深入涉猎了其中三个。Pigsty 本身是一个高可用的 PostgreSQL 发行版,里面也整合了 DuckDB,以及 DuckDB 缝合的PG扩展。Tigerbettle 我也做好了 RPM/DEB 包,作为专业版中默认下载的金融事务专用数据库。
另外两个数据库,正在我的整合 TODOLIST 中,SQLite 除了 FDW,下一步就是把 ElectricSQL 给弄进来;提供本地 PG 与远端 SQLite / PGLite 的同步能力;CockroachDB 则一直在我的 TODOLIST 中,准备一有空闲就做个部署支持。FoundationDB 是我感兴趣的对象,下一个我愿意花时间深入研究的数据库不出意料会是这个。
总的来说,我认为这些技术代表着领域前沿的发展趋势。如果让我设想一下十年后的格局,那么大概会是这样的: FoundationDB,TigerBeetle,CockRoachDB 能有自己的小众基本盘生态位。DuckDB 大概会在分析领域大放异彩,SQLite 会在本地优先的端侧继续攻城略地,而 PostgreSQL 会从 “默认数据库” 变成无处不在的的 “Linux 内核”,数据库领域的主旋律变成 Neon,Supabase,Vercel,RDS,Pigsty 这样 PostgreSQL 发行版竞争的战场。
毕竟,PostgreSQL 吞噬数据库世界可不只是说说而已,PostgreSQL生态的公司几乎拿光了这两年资本市场数据库领域的钱,早就有无数真金白银用脚投票押注上去了。当然,未来到底如何,还是让我们拭目以待吧。
自建Supabase:创业出海的首选数据库
Supabase 非常棒,拥有你自己的 Supabase 那就是棒上加棒!本文介绍了如何在本地/云端物理机/裸金属/虚拟机上自建企业级 Supabase。
目录
简短版本
curl -fsSL https://repo.pigsty.cc/get | bash; cd ~/pigsty
./bootstrap # 准备 Pigsty 依赖
./configure -c app/supa # 使用 Supabase 应用模板
./install.yml # 安装 Pigsty,以及各种数据库
./docker.yml # 安装 Docker 与 Docker Compose
./app.yml # 使用 Docker 拉起 Supabase 无状态部分
Supabase是什么?
Supabase 是一个开源的 Firebase,是一个 BaaS (Backend as Service)。 Supabase 对 PostgreSQL 进行了封装,并提供了身份认证,消息传递,边缘函数,对象存储,以及基于 PG 数据库模式自动生成的 REST API 与 GraphQL API。
Supabase 旨在为开发者提供一站式的后端解决方案,减少开发和维护后端基础设施的复杂性,使开发者专注于前端开发和用户体验。 用大白话来说就是:让开发者告别绝大部分后端开发的工作,只需要懂数据库设计与前端即可快速出活!
目前,Supabase 是 PostgreSQL 生态人气最高的开源项目,在 GitHub 上已经有高达7万4千的Star数。 并且和 Neon,Cloudflare 一起并称为赛博菩萨 —— 因为他们都提供了非常不错的云服务免费计划。 目前,Supabase 和 Neon 已经成为许多初创企业的首选数据库 —— 用起来非常方便,起步还是免费的。
为什么要自建Supabase?
小微规模(4c8g)内的 Supabase 云服务极富性价比,人称赛博菩萨。那么 Supabase 云服务这么香,为什么要自建呢?
最直观的原因是是我们在《云数据库是智商税吗?》中提到过的:当你的规模超出云计算适用光谱,成本很容易出现爆炸式增长。 而且在当下,足够可靠的本地企业级 NVMe SSD在性价比上与云端存储有着三到四个数量级的优势,而自建能更好地利用这一点。
另一个重要的原因是功能, Supabase 云服务的功能受限 —— 出于与RDS相同的逻辑, 很多 强力PG扩展 因为多租户安全挑战与许可证的原因无法作为云服务提供。 故而尽管PG扩展是 Supabase 的一个核心特色,在云服务上也依然只有 64 个可用扩展,而 Pigsty 提供了多达 400 个开箱即用的 PG 扩展。
此外,尽管 Supabase 虽然旨在提供一个无供应商锁定的 Google Firebase 开源替代,但实际上自建高标准企业级的 Supabase 门槛并不低: Supabase 内置了一系列由他们自己开发维护的 PG 扩展插件,而这些扩展在 PGDG 官方仓库中并没有提供。 这实际上是某种隐性的供应商锁定,阻止了用户使用除了 supabase/postgres Docker 镜像之外的方式自建。
Pigsty 解决了这些问题,我们将所有 Supabase 自研与用到的 10 个缺失的扩展打成开箱即用的 RPM/DEB 包,确保它们在所有主流Linux操作系统发行版上都可用:
- pg_graphql:提供PG内的GraphQL支持 (RUST),Rust扩展,由PIGSTY提供
- pg_jsonschema:提供JSON Schema校验能力,Rust扩展,由PIGSTY提供
- wrappers:Supabase提供的外部数据源包装器捆绑包,,Rust扩展,由PIGSTY提供
- index_advisor:查询索引建议器,SQL扩展,由PIGSTY提供
- pg_net:用 SQL 进行异步非阻塞HTTP/HTTPS 请求的扩展 (supabase),C扩展,由PIGSTY提供
- vault:在 Vault 中存储加密凭证的扩展 (supabase),C扩展,由PIGSTY提供
- pgjwt:JSON Web Token API 的PG实现 (supabase),SQL扩展,由PIGSTY提供
- pgsodium:表数据加密存储 TDE,扩展,由PIGSTY提供
- supautils:用于在云环境中确保数据库集群的安全,C扩展,由PIGSTY提供
- pg_plan_filter:使用执行计划代价过滤阻止特定查询语句,C扩展,由PIGSTY提供
我们还在 Supabase 中默认安装了以下扩展,您可以参考可用扩展列表启用更多。
- timescaledb postgis pg_graphql pg_jsonschema wrappers pg_search pg_analytics pg_parquet plv8 duckdb_fdw pg_cron pg_timetable pgqr
- supautils pg_plan_filter passwordcheck plpgsql_check pgaudit pgsodium pg_vault pgjwt pg_ecdsa pg_session_jwt index_advisor
- pgvector pgvectorscale pg_summarize pg_tiktoken pg_tle pg_stat_monitor hypopg pg_hint_plan pg_http pg_net pg_smtp_client pg_idkit
同时,Pigsty 还会负责好底层 高可用 PostgreSQL 数据库集群,高可用 MinIO 对象存储集群的自动搭建,甚至是 Docker 容器底座的部署与 Nginx 域名配置与证书签发。 最终,您可以使用 Docker Compose 拉起任意数量的无状态 Supabase 容器集群,并使用外部由 Pigsty 托管的企业级 PostgreSQL 数据库与 MinIO 对象存储,甚至连反向代理的 Nginx 等都已经为您配置准备完毕!
在这一自建部署架构中,您获得了使用不同内核的自由(PG 12-17),加装400个扩展的自由,扩容与伸缩Supabase/Postgres/MinIO的自由,免于数据库运维的自由,以及告别供应商锁定的自由。 而相比于使用 Supabase 云服务需要付出的代价,不过是准备一(几)台物理机/虚拟机 + 敲几行命令,等候十几分钟的区别。
单节点自建快速上手
让我们先从单节点 Supabase 部署开始,我们会在后面进一步介绍多节点高可用部署的方法。
首先,使用 Pigsty 标准安装流程 安装 Supabase 所需的 MinIO 与 PostgreSQL 实例;
然后额外运行 docker.yml
与 app.yml
完成剩余的工作,
拉起无状态部分的 Supabase 容器,Supabase 就可以使用了(默认端口 8000
/8433
)。
curl -fsSL https://repo.pigsty.cc/get | bash
./bootstrap # 环境检查,自动安装依赖:Ansible
./configure -c supa # 重要:请在配置文件中修改密码等关键信息!
./install.yml # 安装 Pigsty,拉起 PGSQL 与 MINIO!
./docker.yml # 安装 Docker 于 Docker Compose
./app.yml # 使用 Docker 拉起 Supabase 无状态部分!
请在部署 Supabase 前,根据您的实际情况,修改自动生成的 pigsty.yml
配置文件中的参数(主要是密码!)
如果您只是将其用于本地开发测试,可以先不管这些,我们将在后面介绍如何通过修改配置文件来定制您的 Supabase。
如果您的配置没有问题,那么大约在 10 分钟后,您就可以在本地网络通过 http://<your_ip_address>:8000
访问到 Supabase Studio 管理界面了。
检查清单
- 硬件/软件:准备所需的机器资源:Linux x86_64 服务器一台,全新安装主流 Linux 操作系统
- 网络/权限:有 ssh 免密登陆权限,所用用户有免密 sudo 权限
- 确保机器有内网静态IPv4网络地址,并可以访问互联网。中国地区 DockerHub 需要翻墙,需要有可用的代理或镜像站点
- 在
configure
过程中,请输入节点的内网首要 IP 地址,或直接通过-i <primary_ip>
命令行参数指定 - 如果您的网络环境无法访问 DockerHub,请指定
docker_registry_mirrors
使用镜像站 或proxy_env
参数翻墙。
- 在
- 确保使用了
supa
配置模板,并按需修改了参数- 您是否修改了所有与密码有关的配置参数?【可选】
- 您是否需要使用外部 SMTP 服务器?是否配置了
supa_config
中的 SMTP 参数?【可选】
中国地区的用户请注意,如果您没有配置好 Docker 镜像站点或代理服务器,那么会有极大概率会翻车在 ./supabase 最后一步的镜像拉取上。我们建议您掌握科学上网技巧,参考 Docker 模块 FAQ 的说明配置镜像或代理。 请注意,我们提供 Supabase 自建专门咨询服务,¥2000 / 例·半小时,购买附赠预制离线安装包,可以无需互联网(自然也无需翻墙)安装,将您的企业级自建 Supabase 安稳扶上马!
离线软件包使用说明:请在执行安装前,将收到的
pkg.tgz
放置于/tmp/pkg.tgz
,将supabase
目录整个放置在/tmp/supabase
即可。
修改后的配置文件,应该如下所示:
对默认生成的配置文件进行修改
all:
children:
# infra 集群,包含 Prometheus & Grafana 监控基础设施
infra: { hosts: { 10.10.10.10: { infra_seq: 1 } } }
# etcd 集群,本例为单节点 Etcd,用于提供 PG 高可用
etcd: { hosts: { 10.10.10.10: { etcd_seq: 1 } }, vars: { etcd_cluster: etcd } }
# minio 集群,单节点 SNSD 的 S3 兼容对象存储
minio: { hosts: { 10.10.10.10: { minio_seq: 1 } }, vars: { minio_cluster: minio } }
# pg-meta, Supabase 底层实际的 PostgreSQL 数据库
pg-meta:
hosts: { 10.10.10.10: { pg_seq: 1, pg_role: primary } }
vars:
pg_cluster: pg-meta
pg_users:
# supabase 使用的角色
- { name: anon ,login: false }
- { name: authenticated ,login: false }
- { name: dashboard_user ,login: false ,replication: true ,createdb: true ,createrole: true }
- { name: service_role ,login: false ,bypassrls: true }
# 【注意】如果你要修改 Supabase 业务用户的密码,请在这里统一修改所有用户的密码
- { name: supabase_admin ,password: 'DBUser.Supa' ,pgbouncer: true ,inherit: true ,roles: [ dbrole_admin ] ,superuser: true ,replication: true ,createdb: true ,createrole: true ,bypassrls: true }
- { name: authenticator ,password: 'DBUser.Supa' ,pgbouncer: true ,inherit: false ,roles: [ dbrole_admin, authenticated ,anon ,service_role ] }
- { name: supabase_auth_admin ,password: 'DBUser.Supa' ,pgbouncer: true ,inherit: false ,roles: [ dbrole_admin ] ,createrole: true }
- { name: supabase_storage_admin ,password: 'DBUser.Supa' ,pgbouncer: true ,inherit: false ,roles: [ dbrole_admin, authenticated ,anon ,service_role ] ,createrole: true }
- { name: supabase_functions_admin ,password: 'DBUser.Supa' ,pgbouncer: true ,inherit: false ,roles: [ dbrole_admin ] ,createrole: true }
- { name: supabase_replication_admin ,password: 'DBUser.Supa' ,replication: true ,roles: [ dbrole_admin ]}
- { name: supabase_read_only_user ,password: 'DBUser.Supa' ,bypassrls: true ,roles: [ dbrole_readonly, pg_read_all_data ] }
# 【注意】 这里定义了 Supabase 使用的底层 Postgres 业务数据库,
pg_databases:
- name: postgres
baseline: supabase.sql # 这里的 files/supabase.sql 文件包含了初始化 Supabase 所必需的模式迁移脚本,非常重要!
owner: supabase_admin # 这里的数据库所有者,必须是上面定义的 supabase_admin,我们建议使用此用户进行模式变更。
comment: supabase postgres database
schemas: [ extensions ,auth ,realtime ,storage ,graphql_public ,supabase_functions ,_analytics ,_realtime ]
extensions: # 定义在这里的扩展会默认在数据库中 “创建并启用”
- { name: pgcrypto ,schema: extensions } # 1.3
- { name: pg_net ,schema: extensions } # 0.9.2
- { name: pgjwt ,schema: extensions } # 0.2.0
- { name: uuid-ossp ,schema: extensions } # 1.1
- { name: pgsodium } # 3.1.9
- { name: supabase_vault } # 0.2.8
- { name: pg_graphql } # 1.5.9
- { name: pg_jsonschema } # 0.3.3
- { name: wrappers } # 0.4.3
- { name: http } # 1.6
- { name: pg_cron } # 1.6
- { name: timescaledb } # 2.17
- { name: pg_tle } # 1.2
- { name: vector } # 0.8.0
# 这些扩展默认需要动态加载
pg_libs: 'pg_stat_statements, plpgsql, plpgsql_check, pg_cron, pg_net, timescaledb, auto_explain, pg_tle, plan_filter'
# 如果你想安装其他扩展插件,请在这里指定,但请同样添加到下面的 repo_packages 中。
pg_extensions: # 在这里定义 “安装” 的扩展集合,安装后您可以按需手工 “启用/创建”
- supabase # Supabase 所必需的关键扩展集合,其他扩展为可选
- timescaledb postgis pg_graphql pg_jsonschema wrappers pg_search pg_analytics pg_parquet plv8 duckdb_fdw pg_cron pg_timetable pgqr
- supautils pg_plan_filter passwordcheck plpgsql_check pgaudit pgsodium pg_vault pgjwt pg_ecdsa pg_session_jwt index_advisor
- pgvector pgvectorscale pg_summarize pg_tiktoken pg_tle pg_stat_monitor hypopg pg_hint_plan pg_http pg_net pg_smtp_client pg_idkit
pg_parameters:
cron.database_name: postgres
pgsodium.enable_event_trigger: off
pg_hba_rules: # 额外的 HBA 规则,允许 Supabase 从容器网段访问
- { user: all ,db: postgres ,addr: intra ,auth: pwd ,title: 'allow supabase access from intranet' }
- { user: all ,db: postgres ,addr: 172.17.0.0/16 ,auth: pwd ,title: 'allow access from local docker network' }
pg_vip_enabled: true
pg_vip_address: 10.10.10.2/24
pg_vip_interface: eth1
# 这里定义的 Ansible 分组 supa 包含了 Docker 与 Supabase 相关的配置,您可以使用 docker.yml & app.yml 剧本直接将其拉起
supa:
hosts:
10.10.10.10: {}
vars:
app: supabase # 指定默认安装等应用名称(supabase)
apps: # 定义应用列表,这里只有 supabase
supabase: # Supabase 应用定义
conf: # 下面的 Supabase 配置项会自动覆盖或追加到 /opt/supabase/.env 文件中(模板路径:app/supabase/.env ,内容详见:https://github.com/pgsty/pigsty/blob/main/app/supabase/.env)
# 【非常重要】: 请修改下面的 JWT_SECRET 以及 ANON_KEY 与 SERVICE_ROLE_KEY : https://supabase.com/docs/guides/self-hosting/docker#securing-your-services
JWT_SECRET: your-super-secret-jwt-token-with-at-least-32-characters-long
ANON_KEY: eyJhbGciOiJIUzI1NiIsInR5cCI6IkpXVCJ9.eyAgCiAgICAicm9sZSI6ICJhbm9uIiwKICAgICJpc3MiOiAic3VwYWJhc2UtZGVtbyIsCiAgICAiaWF0IjogMTY0MTc2OTIwMCwKICAgICJleHAiOiAxNzk5NTM1NjAwCn0.dc_X5iR_VP_qT0zsiyj_I_OZ2T9FtRU2BBNWN8Bu4GE
SERVICE_ROLE_KEY: eyJhbGciOiJIUzI1NiIsInR5cCI6IkpXVCJ9.eyAgCiAgICAicm9sZSI6ICJzZXJ2aWNlX3JvbGUiLAogICAgImlzcyI6ICJzdXBhYmFzZS1kZW1vIiwKICAgICJpYXQiOiAxNjQxNzY5MjAwLAogICAgImV4cCI6IDE3OTk1MzU2MDAKfQ.DaYlNEoUrrEn2Ig7tqibS-PHK5vgusbcbo7X36XVt4Q
DASHBOARD_USERNAME: supabase
DASHBOARD_PASSWORD: pigsty
#【注意】请在下面填入 PostgreSQL 链接串信息
POSTGRES_HOST: 10.10.10.10
POSTGRES_PORT: 5436 # access via the 'default' service, which always route to the primary postgres
POSTGRES_DB: postgres
POSTGRES_PASSWORD: DBUser.Supa # password for supabase_admin and multiple supabase users
# 如果您使用自定义域名,请修改下面的 domain 字段,将 supa.pigsty 替换为您自己的域名
SITE_URL: http://supa.pigsty # <------- Change This to your external domain name
API_EXTERNAL_URL: http://supa.pigsty # <------- Otherwise the storage api may not work!
SUPABASE_PUBLIC_URL: http://supa.pigsty # <------- Do not forget!
# if using s3/minio as file storage
S3_BUCKET: supa
S3_ENDPOINT: https://sss.pigsty:9000
S3_ACCESS_KEY: supabase
S3_SECRET_KEY: S3User.Supabase
S3_FORCE_PATH_STYLE: true
S3_PROTOCOL: https
S3_REGION: stub
MINIO_DOMAIN_IP: 10.10.10.10 # sss.pigsty domain name will resolve to this ip statically
#【可选】 指定 SMTP 服务器发送邮件
#SMTP_ADMIN_EMAIL: admin@example.com
#SMTP_HOST: supabase-mail
#SMTP_PORT: 2500
#SMTP_USER: fake_mail_user
#SMTP_PASS: fake_mail_password
#SMTP_SENDER_NAME: fake_sender
#ENABLE_ANONYMOUS_USERS: false
docker_enabled: true # 在 supabase 分组上启用 Docker,因为我们要用 Docker Compose 拉起无状态的部分
# 【注意】中国大陆地区的用户请指定 DockerHub 镜像站点或代理服务器,否则拉取镜像会失败
#docker_registry_mirrors: ['https://docker.xxxxx.io']
#proxy_env: # add [OPTIONAL] proxy env to /etc/docker/daemon.json configuration file
# no_proxy: "localhost,127.0.0.1,10.0.0.0/8,192.168.0.0/16,*.pigsty,*.aliyun.com,mirrors.*,*.myqcloud.com,*.tsinghua.edu.cn"
# all_proxy: http://user:pass@host:port
#==============================================================#
# Global Parameters
#==============================================================#
vars:
version: v3.3.0 # pigsty version string
admin_ip: 10.10.10.10 # admin node ip address
region: default # upstream mirror region: default|china|europe
node_tune: oltp # node tuning specs: oltp,olap,tiny,crit
pg_conf: oltp.yml # pgsql tuning specs: {oltp,olap,tiny,crit}.yml
docker_enabled: true # enable docker on app group
# WARNING: YOU MAY HAVE
#docker_registry_mirrors: ["https://docker.m.daocloud.io"] # use dao cloud mirror in mainland china
proxy_env: # global proxy env when downloading packages & pull docker images
no_proxy: "localhost,127.0.0.1,10.0.0.0/8,192.168.0.0/16,*.pigsty,*.aliyun.com,mirrors.*,*.tsinghua.edu.cn"
#http_proxy: 127.0.0.1:12345 # add your proxy env here for downloading packages or pull images
#https_proxy: 127.0.0.1:12345 # usually the proxy is format as http://user:pass@proxy.xxx.com
#all_proxy: 127.0.0.1:12345
infra_portal: # domain names and upstream servers
home : { domain: h.pigsty }
grafana : { domain: g.pigsty ,endpoint: "${admin_ip}:3000" , websocket: true }
prometheus : { domain: p.pigsty ,endpoint: "${admin_ip}:9090" }
alertmanager : { domain: a.pigsty ,endpoint: "${admin_ip}:9093" }
minio : { domain: m.pigsty ,endpoint: "10.10.10.10:9001", https: true, websocket: true }
blackbox : { endpoint: "${admin_ip}:9115" }
loki : { endpoint: "${admin_ip}:3100" } # expose supa studio UI and API via nginx
supa : { domain: supa.pigsty ,endpoint: "10.10.10.10:8000", websocket: true }
# certbot --nginx --agree-tos --email your@email.com -n -d supa.your.domain # replace with your email & supa domain
#----------------------------------#
# Credential: CHANGE THESE PASSWORDS
#----------------------------------#
#grafana_admin_username: admin
grafana_admin_password: pigsty
#pg_admin_username: dbuser_dba
pg_admin_password: DBUser.DBA
#pg_monitor_username: dbuser_monitor
pg_monitor_password: DBUser.Monitor
#pg_replication_username: replicator
pg_replication_password: DBUser.Replicator
#patroni_username: postgres
patroni_password: Patroni.API
#haproxy_admin_username: admin
haproxy_admin_password: pigsty
#minio_access_key: minioadmin
minio_secret_key: minioadmin # minio root secret key, `minioadmin` by default
# use minio as supabase file storage, single node single driver mode for demonstration purpose
minio_buckets: [ { name: pgsql }, { name: supa } ]
minio_users:
- { access_key: dba , secret_key: S3User.DBA, policy: consoleAdmin }
- { access_key: pgbackrest , secret_key: S3User.Backup, policy: readwrite }
- { access_key: supabase , secret_key: S3User.Supabase, policy: readwrite }
minio_endpoint: https://sss.pigsty:9000 # explicit overwrite minio endpoint with haproxy port
node_etc_hosts: ["10.10.10.10 sss.pigsty"] # domain name to access minio from all nodes (required)
# use minio as default backup repo for PostgreSQL
pgbackrest_method: minio # pgbackrest repo method: local,minio,[user-defined...]
pgbackrest_repo: # pgbackrest repo: https://pgbackrest.org/configuration.html#section-repository
local: # default pgbackrest repo with local posix fs
path: /pg/backup # local backup directory, `/pg/backup` by default
retention_full_type: count # retention full backups by count
retention_full: 2 # keep 2, at most 3 full backup when using local fs repo
minio: # optional minio repo for pgbackrest
type: s3 # minio is s3-compatible, so s3 is used
s3_endpoint: sss.pigsty # minio endpoint domain name, `sss.pigsty` by default
s3_region: us-east-1 # minio region, us-east-1 by default, useless for minio
s3_bucket: pgsql # minio bucket name, `pgsql` by default
s3_key: pgbackrest # minio user access key for pgbackrest
s3_key_secret: S3User.Backup # minio user secret key for pgbackrest
s3_uri_style: path # use path style uri for minio rather than host style
path: /pgbackrest # minio backup path, default is `/pgbackrest`
storage_port: 9000 # minio port, 9000 by default
storage_ca_file: /pg/cert/ca.crt # minio ca file path, `/pg/cert/ca.crt` by default
bundle: y # bundle small files into a single file
cipher_type: aes-256-cbc # enable AES encryption for remote backup repo
cipher_pass: pgBackRest # AES encryption password, default is 'pgBackRest'
retention_full_type: time # retention full backup by time on minio repo
retention_full: 14 # keep full backup for last 14 days
# download docker and all available extensions
pg_version: 17
repo_modules: node,pgsql,infra,docker
repo_packages: [node-bootstrap, infra-package, infra-addons, node-package1, node-package2, pgsql-utility, docker ]
repo_extra_packages: [pg17-core ,pg17-time ,pg17-gis ,pg17-rag ,pg17-fts ,pg17-olap ,pg17-feat ,pg17-lang ,pg17-type ,pg17-util ,pg17-func ,pg17-admin ,pg17-stat ,pg17-sec ,pg17-fdw ,pg17-sim ,pg17-etl ]
pg_extensions: [pg17-time ,pg17-gis ,pg17-rag ,pg17-fts ,pg17-olap ,pg17-feat ,pg17-lang ,pg17-type ,pg17-util ,pg17-func ,pg17-admin ,pg17-stat ,pg17-sec ,pg17-fdw ,pg17-sim ,pg17-etl ]
...
自建关键技术决策
以下是一些自建 Supabase 会涉及到的关键技术决策,供您参考:
使用默认的单节点部署 Supabase 无法享受到 PostgreSQL / MinIO 的高可用能力。 尽管如此,单节点部署相比官方纯 Docker Compose 方案依然要有显著优势: 例如开箱即用的监控系统,自由安装扩展的能力,各个组件的扩缩容能力,以及数据库时间点恢复能力等。
如果您只有一台服务器,Pigsty 建议您直接使用外部的 S3 作为对象存储,存放 PostgreSQL 的备份,并承载 Supabase Storage 服务。 这样的部署在故障时可以提供一个最低标准的 RTO (小时级恢复时长)/ RPO (MB级数据损失)兜底容灾水平。 此外,如果您选择在云上自建,我们也建议您直接使用 S3,而非默认使用的本体 MinIO ,单纯在本地 EBS 上再套一层 MinIO 转发,除了便于开发测试外,对生产实用并没有意义。
在严肃的生产部署中,Pigsty 建议使用至少3~4个节点的部署策略,确保 MinIO 与 PostgreSQL 都使用满足企业级高可用要求的多节点部署。
在这种情况下,您需要相应准备更多节点与磁盘,并相应调整 pigsty.yml
配置清单中的集群配置,以及 supabase 集群配置中的接入信息。
部分 Supabase 的功能需要发送邮件,所以要用到 SMTP。除非单纯用于内网,否则对于严肃的生产部署,我们建议您考虑使用外部的 SMTP 服务。 自建的邮件服务器发送的邮件可能会被对方邮件服务器拒收,或者被标记为垃圾邮件。
如果您的服务直接向公网暴露,我们建议您使用 Nginx 进行反向代理,使用真正的域名与 HTTPS 证书,并通过不同的域名区分不同的多个实例。
接下来,我们会依次讨论这几个主题:
- 进阶主题:安全加固
- 高可用的 PostgreSQL 集群部署与接入
- 高可用的 MinIO 集群部署与接入
- 使用 S3 服务替代 MinIO
- 使用外部 SMTP 服务发送邮件
- 使用真实域名,证书,通过 Nginx 反向代理
进阶主题:安全加固
Pigsty基础组件
对于严肃的生产部署,我们强烈建议您修改 Pigsty 基础组件的密码。因为这些默认值是公开且众所周知的,不改密码上生产无异于裸奔:
grafana_admin_password
:pigsty
,Grafana管理员密码pg_admin_password
:DBUser.DBA
,PG超级用户密码pg_monitor_password
:DBUser.Monitor
,PG监控用户密码pg_replication_password
:DBUser.Replicator
,PG复制用户密码patroni_password
:Patroni.API
,Patroni 高可用组件密码haproxy_admin_password
:pigsty
,负载均衡器管控密码minio_access_key
:minioadmin
,MinIO 根用户名minio_secret_key
:minioadmin
,MinIO 根用户密钥- 此外,强烈建议您修改 Supabase 使用的 PostgreSQL 业务用户 密码,默认为
DBUser.Supa
以上密码为 Pigsty 组件模块的密码,强烈建议在安装部署前就设置完毕。
Supabase密钥
除了 Pigsty 组件的密码,你还需要 修改 Supabase 的密钥,包括
jwt_secret
:anon_key
:service_role_key
:dashboard_username
: Supabase Studio Web 界面的默认用户名,默认为supabase
dashboard_password
: Supabase Studio Web 界面的默认密码,默认为pigsty
这里请您务必参照 Supabase教程:保护你的服务 里的说明:
- 生成一个长度超过 40 个字符的
jwt_secret
,并使用教程中的工具签发anon_key
与service_role_key
两个 JWT。 - 使用教程中提供的工具,根据
jwt_secret
以及过期时间等属性,生成一个anon_key
JWT,这是匿名用户的身份凭据。 - 使用教程中提供的工具,根据
jwt_secret
以及过期时间等属性,生成一个service_role_key
,这是权限更高服务角色的身份凭据。 - 如果您使用的 PostgreSQL 业务用户使用了不同于默认值的密码,请相应修改 `postgres_password`` 的值
- 如果您的对象存储使用了不同于默认值的密码,请相应修改
s3_access_key``](https://github.com/pgsty/pigsty/blob/main/conf/app/supa.yml#136) 与 [
s3_secret_key`` 的值
Supabase 部分的凭据修改后,您可以重启 Docker Compose 容器以应用新的配置:
cd /opt/supabase; docker compose up
进阶主题:域名接入
如果你在本机或局域网内使用 Supabase,那么可以选择 IP:Port 直连 Kong 对外暴露的 HTTP 8000 端口,当然这样并不好,我们建议您使用域名与 HTTPS 来访问。
使用默认的本地域名 supa.pigsty
时,您可以在浏览器本机的 /etc/hosts
或局域网 DNS 里来配置它的解析,将其指向安装节点的【对外】IP地址。
Pigsty 管理节点上的 Nginx 会为此域名申请自签名的证书(浏览器显示《不安全》),并将请求转发到 8000 端口的 Kong,由 Supabase 处理。
不过,更为实用与常见的用例是:Supabase 通过公网队外提供服务。在这种情况下,通常您需要进行以下准备:
- 您的服务器应当有一个公网 IP 地址
- 购买域名,使用 云/DNS/CDN 供应商提供的 DNS 解析服务,将其指向安装节点的公网 IP(下位替代:本地
/etc/hosts
) - 申请证书,使用 Let’s Encrypt 等证书颁发机构签发的免费 HTTPS 证书,用于加密通信(下位替代:默认自签名证书,手工信任)
准备完成后,请修改 pigsty.yml
配置文件中 all.vars.infra_portal
部分的 supa
域名,以及 all.children.supabase.vars.supa_config
中的三个域名字段。
这里我们假设您使用的自定义域名是: supa.pigsty.cc
all:
vars: # 全局配置
#.....
infra_portal: # domain names and upstream servers
home : { domain: h.pigsty }
grafana : { domain: g.pigsty ,endpoint: "${admin_ip}:3000" , websocket: true }
prometheus : { domain: p.pigsty ,endpoint: "${admin_ip}:9090" }
alertmanager : { domain: a.pigsty ,endpoint: "${admin_ip}:9093" }
minio : { domain: m.pigsty ,endpoint: "10.10.10.10:9001", https: true, websocket: true }
blackbox : { endpoint: "${admin_ip}:9115" }
loki : { endpoint: "${admin_ip}:3100" }
#supa : { domain: supa.pigsty ,endpoint: "10.10.10.10:8000", websocket: true } # 如果使用申请的 HTTPS 证书,请在这里指定证书的存放路径
supa : { domain: supa.pigsty.cc ,endpoint: "10.10.10.10:8000", websocket: true ,cert: /etc/cert/suap.pigsty.cc.crt ,key: /etc/cert/supa.pigsty.cc.key }
children: # 集群定义
supabase: # supabase 分组
vars: # supabase 分组集群配置
supa_config: # supabase 配置项
# 请在这里更新 Supabase 使用的域名
site_url: http://supa.pigsty
api_external_url: http://supa.pigsty
supabase_public_url: http://supa.pigsty
申请 HTTPS 证书超出了本文范畴,请您自行用 certbot 之类的工具处理。
./infra.yml -t nginx_config,nginx_launch
./supabase.yml -t supa_config,supa_launch
使用以上命令重新加载 Nginx 和 Supabase 的配置。
进阶主题:外部对象存储
您可以使用 S3 或 S3 兼容的服务,来作为 PGSQL 备份与 Supabase 使用的对象存储。这里我们使用一个 阿里云 OSS 对象存储作为例子。
Pigsty 提供了一个
terraform/spec/aliyun-meta-s3.tf
模板,用于在阿里云上拉起一台服务器,以及一个 OSS 存储桶。
首先,我们修改 all.children.supa.vars.apps.[supabase].conf
中 S3 相关的配置,将其指向阿里云 OSS 存储桶:
# if using s3/minio as file storage
S3_BUCKET: supa
S3_ENDPOINT: https://sss.pigsty:9000
S3_ACCESS_KEY: supabase
S3_SECRET_KEY: S3User.Supabase
S3_FORCE_PATH_STYLE: true
S3_PROTOCOL: https
S3_REGION: stub
MINIO_DOMAIN_IP: 10.10.10.10 # sss.pigsty domain name will resolve to this ip statically
同样使用以下命令重载 Supabase 配置:
./app.yml -t app_config,app_launch
您同样可以使用 S3 作为 PostgreSQL 的备份仓库,在 all.vars.pgbackrest_repo
新增一个 aliyun
备份仓库的定义:
all:
vars:
pgbackrest_method: aliyun # pgbackrest 备份方法:local,minio,[其他用户定义的仓库...],本例中将备份存储到 MinIO 上
pgbackrest_repo: # pgbackrest 备份仓库: https://pgbackrest.org/configuration.html#section-repository
aliyun: # 定义一个新的备份仓库 aliyun
type: s3 # 阿里云 oss 是 s3-兼容的对象存储
s3_endpoint: oss-cn-beijing-internal.aliyuncs.com
s3_region: oss-cn-beijing
s3_bucket: pigsty-oss
s3_key: xxxxxxxxxxxxxx
s3_key_secret: xxxxxxxx
s3_uri_style: host
path: /pgbackrest
bundle: y
cipher_type: aes-256-cbc
cipher_pass: PG.${pg_cluster} # 设置一个与集群名称绑定的加密密码
retention_full_type: time
retention_full: 14
然后在 all.vars.pgbackrest_mehod
中指定使用 aliyun
备份仓库,重置 pgBackrest 备份:
./pgsql.yml -t pgbackrest
Pigsty 会将备份仓库切换到外部对象存储上。
进阶主题:备份策略
你可以使用操作系统的 Crontab 来设置定时备份策略,例如,向默认的 all.children.pg-meta.vars
中添加 node_crontab
参数:
all:
children:
pg-meta:
hosts: { 10.10.10.10: { pg_seq: 1, pg_role: primary } }
vars:
pg_cluster: pg-meta # 每天凌晨一点做个全量备份
node_crontab: [ '00 01 * * * postgres /pg/bin/pg-backup full' ]
然后执行以下命令,将 Crontab 配置应用到节点上:
./node.yml -t node_crontab
更多关于备份策略的主题,请参考 备份策略
进阶主题:使用SMTP
你可以使用 SMTP 来发送邮件,修改 supabase 配置,添加 SMTP 信息:
all:
children:
supabase:
vars:
supa_config:
smtp_host: smtpdm.aliyun.com:80
smtp_port: 80
smtp_user: no_reply@mail.your.domain.com
smtp_pass: your_email_user_password
smtp_sender_name: MySupabase
smtp_admin_email: adminxxx@mail.your.domain.com
enable_anonymous_users: false
不要忘了使用 supabase.yml -t supa_config,supa_launch
来重载配置
进阶主题:真·高可用
经过上面的配置,您已经可以使用一个带有公网域名,HTTPS 证书,SMTP 邮件服务器,备份的 Supabase 了。
如果您的这个节点挂了,起码外部 S3 存储中保留了备份,您可以在新的节点上重新部署 Supabase,然后从备份中恢复。 这样的部署在故障时可以提供一个最低标准的 RTO (小时级恢复时长)/ RPO (MB级数据损失)兜底容灾水平 兜底。
但如果您想要达到 RTO < 30s ,零数据丢失,那么就需要用到多节点高可用集群了。多节点部署有三个维度:
- ETCD: DCS 需要使用三个节点或以上,才能容忍一个节点的故障。
- PGSQL: PGSQL 同步提交不丢数据模式,建议使用至少三个节点。
- INFRA:监控基础设施故障影响稍小,但我们建议生产环境使用三副本
- Supabase 本身也可以是多节点的副本,实现高可用
我们建议您参考 trio 与 safe 中的集群配置,将您的集群配置升级到三节点或以上。
在这种情况下,您还需要修改 PostgreSQL 与 MinIO 的接入点,使用 DNS / L2 VIP / HAProxy 等 高可用接入点
例如,假设您使用 L2 VIP 接入 MinIO 集群与 PostgreSQL 集群,那么就需要相应修改配置:
all:
children:
supabase:
hosts:
10.10.10.10: { supa_seq: 1 }
10.10.10.11: { supa_seq: 2 }
10.10.10.12: { supa_seq: 3 }
vars:
supa_cluster: supa # cluster name
supa_config:
postgres_host: 10.10.10.2 # 例如,使用 PG 集群上的 L2 VIP 接入服务
postgres_port: 5436 # 使用 5436 端口,始终直连主库,也可以使用 5433,通过连接池访问主库
s3_endpoint: https://sss.pigsty:9002 # 假如您的负载均衡器使用了 9002 端口,那么请更改这里的 Endpoint
minio_domain_ip: 10.10.10.3 # 修改此参数,将 sss.pigsty 的域名指向挂载在 MinIO 集群前面的 L2 VIP
应用 Supabase 的配置后,您可能还需要在 Supabase 集群前套上一个负载均衡器,用于将请求分发到后端的多个节点上。
以下是一个三节点的 高可用 Supabase 自建的参考配置文件:
3-Node HA Supabase Config Template
all:
#==============================================================#
# Clusters, Nodes, and Modules
#==============================================================#
children:
# infra cluster for proxy, monitor, alert, etc..
infra:
hosts:
10.10.10.10: { infra_seq: 1 ,nodename: infra-1 }
10.10.10.11: { infra_seq: 2 ,nodename: infra-2, repo_enabled: false, grafana_enabled: false }
10.10.10.12: { infra_seq: 3 ,nodename: infra-3, repo_enabled: false, grafana_enabled: false }
vars:
vip_enabled: true
vip_vrid: 128
vip_address: 10.10.10.3
vip_interface: eth1
haproxy_services:
- name: minio # [REQUIRED] service name, unique
port: 9002 # [REQUIRED] service port, unique
balance: leastconn # [OPTIONAL] load balancer algorithm
options: # [OPTIONAL] minio health check
- option httpchk
- option http-keep-alive
- http-check send meth OPTIONS uri /minio/health/live
- http-check expect status 200
servers:
- { name: minio-1 ,ip: 10.10.10.10 ,port: 9000 ,options: 'check-ssl ca-file /etc/pki/ca.crt check port 9000' }
- { name: minio-2 ,ip: 10.10.10.11 ,port: 9000 ,options: 'check-ssl ca-file /etc/pki/ca.crt check port 9000' }
- { name: minio-3 ,ip: 10.10.10.12 ,port: 9000 ,options: 'check-ssl ca-file /etc/pki/ca.crt check port 9000' }
etcd: # dcs service for postgres/patroni ha consensus
hosts: # 1 node for testing, 3 or 5 for production
10.10.10.10: { etcd_seq: 1 } # etcd_seq required
10.10.10.11: { etcd_seq: 2 } # assign from 1 ~ n
10.10.10.12: { etcd_seq: 3 } # odd number please
vars: # cluster level parameter override roles/etcd
etcd_cluster: etcd # mark etcd cluster name etcd
etcd_safeguard: false # safeguard against purging
etcd_clean: true # purge etcd during init process
# minio cluster 4-node
minio:
hosts:
10.10.10.10: { minio_seq: 1 , nodename: minio-1 }
10.10.10.11: { minio_seq: 2 , nodename: minio-2 }
10.10.10.12: { minio_seq: 3 , nodename: minio-3 }
vars:
minio_cluster: minio
minio_data: '/data{1...4}'
minio_buckets: [ { name: pgsql }, { name: supa } ]
minio_users:
- { access_key: dba , secret_key: S3User.DBA, policy: consoleAdmin }
- { access_key: pgbackrest , secret_key: S3User.Backup, policy: readwrite }
- { access_key: supabase , secret_key: S3User.Supabase, policy: readwrite }
# pg-meta, the underlying postgres database for supabase
pg-meta:
hosts:
10.10.10.10: { pg_seq: 1, pg_role: primary }
10.10.10.11: { pg_seq: 2, pg_role: replica }
10.10.10.12: { pg_seq: 3, pg_role: replica }
vars:
pg_cluster: pg-meta
pg_users:
# supabase roles: anon, authenticated, dashboard_user
- { name: anon ,login: false }
- { name: authenticated ,login: false }
- { name: dashboard_user ,login: false ,replication: true ,createdb: true ,createrole: true }
- { name: service_role ,login: false ,bypassrls: true }
# supabase users: please use the same password
- { name: supabase_admin ,password: 'DBUser.Supa' ,pgbouncer: true ,inherit: true ,roles: [ dbrole_admin ] ,superuser: true ,replication: true ,createdb: true ,createrole: true ,bypassrls: true }
- { name: authenticator ,password: 'DBUser.Supa' ,pgbouncer: true ,inherit: false ,roles: [ dbrole_admin, authenticated ,anon ,service_role ] }
- { name: supabase_auth_admin ,password: 'DBUser.Supa' ,pgbouncer: true ,inherit: false ,roles: [ dbrole_admin ] ,createrole: true }
- { name: supabase_storage_admin ,password: 'DBUser.Supa' ,pgbouncer: true ,inherit: false ,roles: [ dbrole_admin, authenticated ,anon ,service_role ] ,createrole: true }
- { name: supabase_functions_admin ,password: 'DBUser.Supa' ,pgbouncer: true ,inherit: false ,roles: [ dbrole_admin ] ,createrole: true }
- { name: supabase_replication_admin ,password: 'DBUser.Supa' ,replication: true ,roles: [ dbrole_admin ]}
- { name: supabase_read_only_user ,password: 'DBUser.Supa' ,bypassrls: true ,roles: [ dbrole_readonly, pg_read_all_data ] }
pg_databases:
- name: postgres
baseline: supabase.sql
owner: supabase_admin
comment: supabase postgres database
schemas: [ extensions ,auth ,realtime ,storage ,graphql_public ,supabase_functions ,_analytics ,_realtime ]
extensions:
- { name: pgcrypto ,schema: extensions } # 1.3 : cryptographic functions
- { name: pg_net ,schema: extensions } # 0.9.2 : async HTTP
- { name: pgjwt ,schema: extensions } # 0.2.0 : json web token API for postgres
- { name: uuid-ossp ,schema: extensions } # 1.1 : generate universally unique identifiers (UUIDs)
- { name: pgsodium } # 3.1.9 : pgsodium is a modern cryptography library for Postgres.
- { name: supabase_vault } # 0.2.8 : Supabase Vault Extension
- { name: pg_graphql } # 1.5.9 : pg_graphql: GraphQL support
- { name: pg_jsonschema } # 0.3.3 : pg_jsonschema: Validate json schema
- { name: wrappers } # 0.4.3 : wrappers: FDW collections
- { name: http } # 1.6 : http: allows web page retrieval inside the database.
- { name: pg_cron } # 1.6 : pg_cron: Job scheduler for PostgreSQL
- { name: timescaledb } # 2.17 : timescaledb: Enables scalable inserts and complex queries for time-series data
- { name: pg_tle } # 1.2 : pg_tle: Trusted Language Extensions for PostgreSQL
- { name: vector } # 0.8.0 : pgvector: the vector similarity search
# supabase required extensions
pg_libs: 'pg_stat_statements, plpgsql, plpgsql_check, pg_cron, pg_net, timescaledb, auto_explain, pg_tle, plan_filter'
pg_extensions: # extensions to be installed on this cluster
- supabase # essential extensions for supabase
- timescaledb postgis pg_graphql pg_jsonschema wrappers pg_search pg_analytics pg_parquet plv8 duckdb_fdw pg_cron pg_timetable pgqr
- supautils pg_plan_filter passwordcheck plpgsql_check pgaudit pgsodium pg_vault pgjwt pg_ecdsa pg_session_jwt index_advisor
- pgvector pgvectorscale pg_summarize pg_tiktoken pg_tle pg_stat_monitor hypopg pg_hint_plan pg_http pg_net pg_smtp_client pg_idkit
pg_parameters:
cron.database_name: postgres
pgsodium.enable_event_trigger: off
pg_hba_rules: # supabase hba rules, require access from docker network
- { user: all ,db: postgres ,addr: intra ,auth: pwd ,title: 'allow supabase access from intranet' }
- { user: all ,db: postgres ,addr: 172.17.0.0/16 ,auth: pwd ,title: 'allow access from local docker network' }
pg_vip_enabled: true
pg_vip_address: 10.10.10.2/24
pg_vip_interface: eth1
node_crontab: [ '00 01 * * * postgres /pg/bin/pg-backup full' ] # make a full backup every 1am
# launch supabase stateless part with docker compose: ./supabase.yml
supabase:
hosts:
10.10.10.10: { supa_seq: 1 } # instance 1
10.10.10.11: { supa_seq: 2 } # instance 2
10.10.10.12: { supa_seq: 3 } # instance 3
vars:
supa_cluster: supa # cluster name
docker_enabled: true # enable docker
# use these to pull docker images via proxy and mirror registries
#docker_registry_mirrors: ['https://docker.xxxxx.io']
#proxy_env: # add [OPTIONAL] proxy env to /etc/docker/daemon.json configuration file
# no_proxy: "localhost,127.0.0.1,10.0.0.0/8,192.168.0.0/16,*.pigsty,*.aliyun.com,mirrors.*,*.myqcloud.com,*.tsinghua.edu.cn"
# #all_proxy: http://user:pass@host:port
# these configuration entries will OVERWRITE or APPEND to /opt/supabase/.env file (src template: app/supabase/.env)
# check https://github.com/pgsty/pigsty/blob/main/app/supabase/.env for default values
supa_config:
# IMPORTANT: CHANGE JWT_SECRET AND REGENERATE CREDENTIAL ACCORDING!!!!!!!!!!!
# https://supabase.com/docs/guides/self-hosting/docker#securing-your-services
jwt_secret: your-super-secret-jwt-token-with-at-least-32-characters-long
anon_key: eyJhbGciOiJIUzI1NiIsInR5cCI6IkpXVCJ9.eyAgCiAgICAicm9sZSI6ICJhbm9uIiwKICAgICJpc3MiOiAic3VwYWJhc2UtZGVtbyIsCiAgICAiaWF0IjogMTY0MTc2OTIwMCwKICAgICJleHAiOiAxNzk5NTM1NjAwCn0.dc_X5iR_VP_qT0zsiyj_I_OZ2T9FtRU2BBNWN8Bu4GE
service_role_key: eyJhbGciOiJIUzI1NiIsInR5cCI6IkpXVCJ9.eyAgCiAgICAicm9sZSI6ICJzZXJ2aWNlX3JvbGUiLAogICAgImlzcyI6ICJzdXBhYmFzZS1kZW1vIiwKICAgICJpYXQiOiAxNjQxNzY5MjAwLAogICAgImV4cCI6IDE3OTk1MzU2MDAKfQ.DaYlNEoUrrEn2Ig7tqibS-PHK5vgusbcbo7X36XVt4Q
dashboard_username: supabase
dashboard_password: pigsty
# postgres connection string (use the correct ip and port)
postgres_host: 10.10.10.3 # use the pg_vip_address rather than single node ip
postgres_port: 5433 # access via the 'default' service, which always route to the primary postgres
postgres_db: postgres
postgres_password: DBUser.Supa # password for supabase_admin and multiple supabase users
# expose supabase via domain name
site_url: http://supa.pigsty
api_external_url: http://supa.pigsty
supabase_public_url: http://supa.pigsty
# if using s3/minio as file storage
s3_bucket: supa
s3_endpoint: https://sss.pigsty:9002
s3_access_key: supabase
s3_secret_key: S3User.Supabase
s3_force_path_style: true
s3_protocol: https
s3_region: stub
minio_domain_ip: 10.10.10.3 # sss.pigsty domain name will resolve to this l2 vip that bind to all nodes
# if using SMTP (optional)
#smtp_admin_email: admin@example.com
#smtp_host: supabase-mail
#smtp_port: 2500
#smtp_user: fake_mail_user
#smtp_pass: fake_mail_password
#smtp_sender_name: fake_sender
#enable_anonymous_users: false
#==============================================================#
# Global Parameters
#==============================================================#
vars:
version: v3.1.0 # pigsty version string
admin_ip: 10.10.10.10 # admin node ip address
region: china # upstream mirror region: default|china|europe
node_tune: oltp # node tuning specs: oltp,olap,tiny,crit
pg_conf: oltp.yml # pgsql tuning specs: {oltp,olap,tiny,crit}.yml
infra_portal: # domain names and upstream servers
home : { domain: h.pigsty }
grafana : { domain: g.pigsty ,endpoint: "${admin_ip}:3000" , websocket: true }
prometheus : { domain: p.pigsty ,endpoint: "${admin_ip}:9090" }
alertmanager : { domain: a.pigsty ,endpoint: "${admin_ip}:9093" }
minio : { domain: m.pigsty ,endpoint: "10.10.10.10:9001", https: true, websocket: true }
blackbox : { endpoint: "${admin_ip}:9115" }
loki : { endpoint: "${admin_ip}:3100" } # expose supa studio UI and API via nginx
supa : { domain: supa.pigsty ,endpoint: "10.10.10.10:8000", websocket: true }
#----------------------------------#
# Credential: CHANGE THESE PASSWORDS
#----------------------------------#
#grafana_admin_username: admin
grafana_admin_password: pigsty
#pg_admin_username: dbuser_dba
pg_admin_password: DBUser.DBA
#pg_monitor_username: dbuser_monitor
pg_monitor_password: DBUser.Monitor
#pg_replication_username: replicator
pg_replication_password: DBUser.Replicator
#patroni_username: postgres
patroni_password: Patroni.API
#haproxy_admin_username: admin
haproxy_admin_password: pigsty
# use minio as supabase file storage, single node single driver mode for demonstration purpose
minio_access_key: minioadmin # root access key, `minioadmin` by default
minio_secret_key: minioadmin # root secret key, `minioadmin` by default
minio_buckets: [ { name: pgsql }, { name: supa } ]
minio_users:
- { access_key: dba , secret_key: S3User.DBA, policy: consoleAdmin }
- { access_key: pgbackrest , secret_key: S3User.Backup, policy: readwrite }
- { access_key: supabase , secret_key: S3User.Supabase, policy: readwrite }
minio_endpoint: https://sss.pigsty:9000 # explicit overwrite minio endpoint with haproxy port
node_etc_hosts: ["10.10.10.3 sss.pigsty"] # domain name to access minio from all nodes (required)
# use minio as default backup repo for PostgreSQL
pgbackrest_method: minio # pgbackrest repo method: local,minio,[user-defined...]
pgbackrest_repo: # pgbackrest repo: https://pgbackrest.org/configuration.html#section-repository
local: # default pgbackrest repo with local posix fs
path: /pg/backup # local backup directory, `/pg/backup` by default
retention_full_type: count # retention full backups by count
retention_full: 2 # keep 2, at most 3 full backup when using local fs repo
minio: # optional minio repo for pgbackrest
type: s3 # minio is s3-compatible, so s3 is used
s3_endpoint: sss.pigsty # minio endpoint domain name, `sss.pigsty` by default
s3_region: us-east-1 # minio region, us-east-1 by default, useless for minio
s3_bucket: pgsql # minio bucket name, `pgsql` by default
s3_key: pgbackrest # minio user access key for pgbackrest
s3_key_secret: S3User.Backup # minio user secret key for pgbackrest
s3_uri_style: path # use path style uri for minio rather than host style
path: /pgbackrest # minio backup path, default is `/pgbackrest`
storage_port: 9002 # minio port, 9000 by default
storage_ca_file: /pg/cert/ca.crt # minio ca file path, `/pg/cert/ca.crt` by default
bundle: y # bundle small files into a single file
cipher_type: aes-256-cbc # enable AES encryption for remote backup repo
cipher_pass: pgBackRest # AES encryption password, default is 'pgBackRest'
retention_full_type: time # retention full backup by time on minio repo
retention_full: 14 # keep full backup for last 14 days
# download docker and supabase related extensions
pg_version: 17
repo_modules: node,pgsql,infra,docker
repo_packages: [node-bootstrap, infra-package, infra-addons, node-package1, node-package2, pgsql-utility, docker ]
repo_extra_packages:
- pgsql-main
- supabase # essential extensions for supabase
- timescaledb postgis pg_graphql pg_jsonschema wrappers pg_search pg_analytics pg_parquet plv8 duckdb_fdw pg_cron pg_timetable pgqr
- supautils pg_plan_filter passwordcheck plpgsql_check pgaudit pgsodium pg_vault pgjwt pg_ecdsa pg_session_jwt index_advisor
- pgvector pgvectorscale pg_summarize pg_tiktoken pg_tle pg_stat_monitor hypopg pg_hint_plan pg_http pg_net pg_smtp_client pg_idkit
面向未来数据库的现代硬件
作者:Alex Miller 2024-11-19 @ Snowflake, Apple, Google
译者:冯若航 & GPT o1,PG 大法师,数据库老司机,云计算泥石流
译者推荐:本文是一篇关于硬件发展如何影响数据库设计的综述,分别介绍了在网络,存储,计算三个领域的关键硬件进展。我一直都认为,充分利用好新硬件(而非折腾所谓分布式)才是数据库内核发展的正路。 请看《重新拿回计算机硬件的红利》与《分布式数据库是伪需求吗》。 而这篇文章很好地介绍了一些数据库领域的前沿软硬件结合实践,值得一读。
原文:Modern Hardware for Future Databases
我们正处于一个令人兴奋的数据库时代,每个主要资源领域都在不断进步,每一项进步都有可能影响最优的数据库架构。总的来说,我希望在未来十年内,能看到数据库架构发生一些有趣的转变,但我不确定是否能有必要的硬件支持。
网络
根据 Stonebraker 在 HPTS 2024 的演讲,使用 VoltDB 的一些基准测试发现,其服务器端大约 60% 的 CPU 时间花在了 TCP/IP 协议栈上。VoltDB 本身就是一种旨在尽可能消除非查询处理工作以服务请求的数据库架构,所以这是一个极端的例子。然而,这仍然有效地指出了 TCP 的计算开销并不小,且随着网络带宽的增加,这一问题会变得更加明显。尽管这并不是新的观察结果,但已有一系列逐步升级的解决方案被提出。
一种被提议的解决方案是用另一种基于 UDP 的协议替换 TCP,QUIC 就是一个常被选择的例子。然而,这种想法存在误区。“虽然这是一个严重不准确的简化,但在最简单的层面上,QUIC 只是将 TCP 封装并加密在 UDP 负载中。” TCP 和 QUIC 的 CPU 开销也非常相似。要想实现显著的改进,需要进一步偏离 TCP 并针对特定环境进行专门化,例如 Homa 这样的论文展示了在数据中心环境中的一些改进。但即使有了更好的协议,更大的优化潜力还是在于减少内核网络栈的开销。
注释:如果你在阅读时想知道为什么这里提到了 QUIC,那是因为我多次参与了关于 TCP 或 TLS 被指责为某些问题的讨论,而迁移到 QUIC 被建议为解决方案。QUIC 确实能帮助解决一些问题,但也有一些问题它并不能改善,甚至可能使其更糟。需要理解的是,在稳定状态下的延迟和带宽属于后者。
一种减少内核工作量的方法是将计算密集但简单的部分移至硬件。这在一段时间内已经逐步实现,例如增强了将分段和校验任务卸载到网卡。更近期的改进是 KTLS,它允许将 TLS 中的数据包加密也卸载到网卡。尝试将整个 TCP 卸载到硬件中,以 TCP 卸载引擎(TOE) 的形式,已被 Linux 维护者系统性地拒绝了。因此,尽管有了这些不错的改进,但 TCP 协议栈的主要部分仍然是内核的责任。
因此,另一种解决方案是去除内核作为网卡和应用程序之间的中间层。像 数据平面开发套件(DPDK) 这样的框架允许用户空间轮询网卡以获取数据包,消除了中断的开销,将所有处理保留在用户空间意味着不需要进入和退出内核。DPDK 在采用方面也遇到了困难,因为它需要对网卡的独占控制。因此,每个主机需要有两个网卡,一个用于 DPDK,另一个用于操作系统和其他所有进程。Marc Richards 制作了一个不错的Linux 内核 vs DPDK基准测试,结果显示 DPDK 提供了 50% 的吞吐量提升,随后列举了为获得这 50% 增益而需要接受的一系列缺点。看来这是大多数数据库不感兴趣的权衡,甚至 ScyllaDB 也基本上放弃了对此的投入。
更新的硬件提供了一个有趣的新选项:将 CPU 从网络路径中移除。RDMA(远程直接内存访问) 提供了 verbs,一组有限的操作(主要是读、写和 8 字节的 CAS),这些操作可以完全在网卡内执行,无需 CPU 交互。切断 CPU 后,远程读取的延迟接近 1 微秒,而 TCP 的延迟则超过 100 微秒。作为 RDMA 的一部分,数据包丢失和流量控制的责任也完全下放到网卡。切断 CPU 还意味着可以在不使 CPU 成为瓶颈的情况下传输大量数据。
注释:为什么将丢包检测和流量控制下放到硬件对于 RDMA 是可接受的,但 Linux 维护者一直拒绝对 TCP 这样做?因为这是一个不同且受限得多的 API,减少了网卡与主机之间的复杂性。《TCP 卸载是一个愚蠢但已经到来的想法》 是在这个领域一篇有趣的阅读材料。(来自 2003 年!)
将 RDMA 作为低延迟和高吞吐量的网络原语,改变了人们设计数据库的方式。《神话的终结:分布式事务可以扩展》 显示了 RDMA 的低延迟使经典的 2PL+2PC 能够扩展到大型集群。《云中可扩展的 OLTP 是一个已解决的问题吗?》 提出了在节点之间共享可写页面缓存的想法,因为低延迟使组件的更紧密耦合变得可行。RDMA 不仅适用于 OLTP 数据库;BigQuery 使用了基于 RDMA Shuffle 的连接,因为其高吞吐量。改变给定吞吐量下的延迟和 CPU 利用率,改变了最佳设计的选择,或者解锁了以前被认为不可行的新设计[^3]。
注释:要使用 RDMA,我强烈建议使用 libfabric,因为它对所有不同的 RDMA 供应商和库进行了抽象。RDMAmojo 博客 有多年关于 RDMA 的专业内容,是学习 RDMA 各个方面的最佳资源之一。
最后,还有一类更新的硬件,延续了将更多计算能力放入网卡本身的趋势,即 SmartNIC 或数据处理单元(DPUs)。它们允许将任意计算下放到网卡,并可能响应其他网卡的请求而被调用。这些技术相当新颖,我建议查看 《DPDPU:使用 DPU 进行数据处理》 以获取概览,《DDS:DPU 优化的分布式存储》 了解如何将它们集成到数据库中,以及 《Azure 加速网络:公共云中的 SmartNIC》 了解部署细节。总体而言,我预计 SmartNIC 会将 RDMA 从简单的读写扩展到允许绕过 CPU 的通用 RPC(用于计算成本低的请求回复)。
存储
在存储设备方面,有一些旨在降低特定用例中存储设备总拥有成本的进展。制造商巧妙地发现,可以读取比写入产生的磁化硬盘盘片的磁道宽度更小的条带,因此可以重叠磁道以达到最小宽度。于是,我们有了叠瓦式磁记录(SMR)硬盘驱动器,引入了将存储划分为区域(zones)的概念,这些区域只支持追加或擦除。SMR HDD 针对的是像对象存储这样访问不频繁但需要存储大量数据的用例。
类似的想法已被应用到 SSD,分区 SSD(Zoned SSDs)也已出现。在 SSD 中暴露区域意味着驱动器不需要提供闪存转换层(FTL)或复杂的垃圾回收过程。与 SMR 类似,这降低了 ZNS SSD 相对于“常规”SSD 的成本,但还特别关注应用驱动的垃圾回收效率更高,从而减少总的写放大效应并延长驱动器寿命。考虑在 SSD 上的 LSM(Log-Structured Merge Trees),它们已经通过增量追加和大擦除块进行操作。移除 LSM 和 SSD 之间的 FTL,打开了优化的机会。最近,Google 和 Meta 合作提出了灵活数据放置(FDP)的提案,它更像是对具有相关生命周期的写入进行分组的提示,而不是像 ZNS 那样严格执行分区。目标是实现更容易的升级路径,使 SSD 可以忽略写请求的 FDP 部分,仍然在语义上正确,只是性能或写放大效应更差。
注释:如果你期待关于持久内存的讨论,遗憾的是 Intel 已经终止了 Optane,所以目前这是一个死胡同。似乎还有一些公司,如 Kioxia 或 Everspin 继续在这方面努力,但我还没有听说过它们的实际应用。
其他改进并非针对成本效率,而是提高存储设备支持的功能集。特别关注 NVMe,NVMe 添加了复制命令,以消除读取和写入相同数据的浪费。融合的比较与写入命令允许将 CAS 操作下放到驱动器本身,从而实现诸如将乐观锁耦合下放到驱动器的创新设计。NVMe 从 SCSI 继承了数据完整性字段(DIF)和数据完整性扩展(DIX)的支持,这使得可以将页面校验和下放到驱动器中(Oracle 就显著地使用了这一点)。还有像 KV-SSD这样的项目,将整个数据模型从按索引存储块改变为按键存储对象,甚至走向完全取代软件存储引擎。SSD 制造商持续让 SSD 具备更多的操作能力。
注释:截至 2024 年 7 月 25 日,AWS 已取消发布 S3 Select,可能是为了支持 S3 Object Lambda。
作为 SSD 功能的倒数第二步,SmartSSD 正在出现,它允许在 SSD 中集成任意计算。《在 SmartSSD 上进行查询处理:机会与挑战》 综述了它们在查询处理任务中的应用。将过滤器下推到存储总是有利的;我经常引用之前的工作,如利用 S3 Select 的 PushdownDB,作为分析领域的优秀案例。使用 SmartSSD,我们有像 《POLARDB 与计算存储的融合》 这样的论文。即使没有专门的集成,也有人认为,即使是透明的驱动器内压缩也能在写放大方面缩小 B+ 树和 LSM 之间的差距(参考)。利用 SmartSSD 仍然是一个新兴的研究领域,但其潜在影响巨大。
计算
事务处理
在最近的 VLDB 会议上,两位数据库研究领域的权威发表了一篇立场论文:《云原生数据库系统和 Unikernels:为现代硬件重新想象操作系统抽象》,主张 Unikernel 允许数据库针对其确切需求定制操作系统。早期关于 VMCache 的工作特别强调了高效数据库缓冲区管理的挑战,在这个领域,要么接受指针变换(pointerswizzling)的复杂性,要么频繁地挂钩内核并调用 mmap()
相关的系统调用。
两种选择都不理想,而 Unikernel 则提供了对虚拟内存原语的直接访问。随着该领域受到更多关注,开发 Unikernel 所需的努力正在减少。黑金章(Akira Kurogane) 通过 Unikraft 以极小的代价就让 MongoDB 作为Unikernel 运行,后续的帖子显示,在没有任何 MongoDB 内部更改的情况下,性能有所提升。一直以来都有一个无休止的笑话,称数据库想要成为操作系统,因为对性能改进的渴望需要对网络、文件系统、磁盘 I/O、内存等有更多的控制,而 Unikernel 数据库正好提供了这一切,使其成为可能。
为了实现超越 TLS 或磁盘加密的数据机密性,安全飞地(secure enclaves)允许执行可验证的未被篡改的代码,使所操作的数据免受被破坏的操作系统的侵害。可信平台模块(TPM) 允许密钥在机器中安全保存,而安全飞地则扩展到任意的代码和数据。这使得构建对恶意攻击具有极高弹性的数据库成为可能,但对其设计有若干限制。微软已经发表了将安全飞地集成到 Hekaton 中的研究,并已将该工作作为 SQL Server Always Encrypted 的一部分发布。阿里巴巴也发表了他们在为担心数据机密性的企业客户构建飞地原生存储引擎方面的努力。数据库一直以来通过合规监管这一渠道推广安全改进,安全飞地在数据机密性方面是一个有意义的进步。
自从 Spanner 引入 TrueTime 以来,时钟同步在地理分布式数据库的事务排序中变得备受关注。每个主要的云提供商都有一个与原子钟或 GPS 卫星连接的 NTP 服务(AWS、Azure、GCP)。这对任何类似的设计都非常有用,例如 CockroachDB 或 Yugabyte,它们的正确性对时钟同步至关重要,而保守的宽误差范围会降低性能。AWS 最近的 Aurora Limitless 也使用了类似 TrueTime 的设计。这是唯一提到的特定云的、并非完全硬件的内容,因为这是主要的云供应商向用户提供昂贵的硬件(原子钟),而用户原本不会考虑自行购买。
硬件事务内存有着相当不幸的历史。Sun 的 Rock 处理器具备硬件事务内存功能,直到 Sun 被收购并且 Rock 项目被终止。英特尔曾两次尝试发布它,但两次都不得不禁用。在将硬件事务内存应用于内存数据库的主题上有一些有趣的工作,但除了找到一些旧的 CPU 进行实验之外,我们都必须等待 CPU 制造商宣布他们计划再次尝试。
注释:第一次是由于一个错误,第二次是由于一个破坏 KASLR 的侧信道攻击。还有一个通过误解CTF 挑战的意图而发现的投机执行定时攻击。
查询处理
一直以来,不断有公司成立,试图利用专用硬件来加速查询处理,以实现比仅使用 CPU 的竞争对手更好的性能和成本效率。像 Voltron、HEAVY.ai 和 Brytlyt 这样的 GPU 驱动数据库,就是朝这个方向迈出的第一步。如果英特尔或 AMD 的集成显卡在未来某个时候获得 OpenCL 支持,我不会感到太惊讶,这将为所有数据库在更广泛的硬件配置中假设一定程度的 GPU 能力打开大门。
注释:OpenGL 计算着色器是使用 GPU 进行任意计算的最通用和可移植的形式,而集成显卡芯片组已经支持这些。不过,我找不到任何关于使用它们的数据库相关论文。
还有机会使用更高能效的硬件。最新的神经处理单元(NPU)和张量处理单元(TPU)已经在类似 《TCUDB:使用张量处理器加速数据库》 的工作中被证明可用于查询处理。一些公司尝试利用 FPGA。Swarm64 曾试图(但可能失败了)进入这个市场。AWS 自己也以 Redshift AQUA 进行了尝试。即使是最大的公司,走到 ASIC 这一步似乎也不值得,因为连 Oracle 都在 2017 年停止了他们的 SPARC 开发。我对 FPGA 到 ASIC 的前景并不十分乐观,因为内存带宽无论如何都会在某个时候成为主要瓶颈,但 ADMS 是关注该领域论文的会议。
注释:严格来说,ADMS 是附属于 VLDB 的一个研讨会,但我不知道泛指会议、期刊和研讨会的词是什么。
云端可用性
最后,让我们直面这个令人沮丧的事实:如果无法获得,这些硬件进步都无关紧要。对于当今的系统,这意味着云端,而云端并未向客户提供最前沿的硬件进步。
在网络方面,情况并不理想。DPDK 是相对容易获取的最先进网络技术,因为大多数云允许某些类型的实例拥有多个网卡。AWS 以 安全可靠数据报(SRD) 的形式提供了伪 RDMA,根据基准测试,其性能大约介于 TCP 和 RDMA 之间。真正的 RDMA 仅在 Azure、GCP 和 OCI 的高性能计算实例中可用。只有阿里巴巴在通用计算实例上提供了 RDMA。
注释:尽管可能会有类似于 SRD 较差的延迟影响。阿里巴巴通过 iWARP 部署了 RDMA,速度可能会稍慢一些,但我还没有看到任何基准测试。
SmartNIC 在任何公开场合都不可用。这其中有充分的理由:微软发表的论文指出,部署 RDMA 是困难的。事实上,非常困难。即使是他们关于成功使用 RDMA 的论文也强调了这非常困难。距离微软开始在内部使用 RDMA 已经接近十年了,但它仍未在他们的云端提供。我无法猜测它是否或何时会出现。
在存储方面,情况并没有好多少。SMR HDD 少数几次进入消费市场时,仍以支持块存储 API 的驱动器形式出现,消费者对此非常反感。ZNS SSD 似乎同样被锁定在仅限企业采购的协议背后。有人可能认为英特尔停止了 Optane 品牌的持久内存和 SSD,这意味着它们在云端不可用,但阿里巴巴仍然提供了持久内存优化的实例。Spare Cores 的优秀团队实际上向我提供了每个云供应商的 nvme id-ctrl
输出,他们获取的 NVMe 设备都没有支持任何可选功能:复制、融合的比较和写入、数据完整性扩展,或多块原子写入。
注释:尽管 AWS 支持防止撕裂写入,GCP 以前也有类似的文档。
阿里巴巴也是唯一一家在 SmartSSD 上进行投资的云供应商,与 ScaleFlux 合作在 PolarDB 上进行了研究。这仍然意味着 SmartSSD 对公众不可用,但即使论文也承认,这是“首次在公开文献中报道的、使用计算存储驱动器的云原生数据库的实际部署”。
在计算方面,情况终于有所改善。云完全允许 Unikernel,TPM 也广泛可用,但据我所知,只有 AWS 和 Azure支持安全飞地。时间同步已可用,但没有承诺的误差范围使得无法关键依赖。(硬件事务内存不可用,但这很难责怪云供应商。)AI 的爆炸式增长意味着有足够的资金支持更高效的计算资源。GPU 在所有云中都可用。AWS[^5]、Azure、IBM 和阿里巴巴提供了 FPGA 实例。(GCP 和 OCI 没有。)不幸的现实是,只有当计算成为瓶颈时,更快的计算才有意义。GPU 和 FPGA 都受到内存限制的影响,因此无法在其本地内存中维护数据库。相反,需要依赖数据的流入和流出,这意味着受到 PCIe 速度的限制。所有这些都会鼓励在本地设备中进行周到的主板布局和总线设计,但这在云中是不可行的。
注释:理想情况下,人们希望有对等 DMA 支持,能够直接从磁盘读取数据到 FPGA 中,而至少 AWS 的 F1 不支持这一点。
因此,我对下一代数据库的看法是悲观的:在新硬件进步可用之前,没人能够构建严重依赖它们的数据库,但没有云供应商愿意部署无法立即使用的硬件。下一代数据库正被这种循环依赖所束缚,因为它们尚未存在。
注释:除了云供应商自己。最值得注意的是,微软和谷歌在内部已经拥有 RDMA 并在他们的数据库产品中广泛利用,同时不允许公众使用。我一直有一篇草稿文章的提纲,标题是“云供应商的 RDMA 竞争优势”。
然而,阿里巴巴的表现令人惊讶地出色。他们始终处于让所有硬件进步可用的前沿。我很惊讶在学术界和工业界中没有经常看到使用阿里巴巴进行基准测试。
PZ:MySQL还有机会赶上PostgreSQL的势头吗?
Percona 的老板 Peter Zaitsev最近发表一篇博客,讨论了MySQL是否还能跟上PostgreSQL的脚步。
Percona 作为MySQL 生态扛旗者,Percona 开发了知名的PT系列工具,MySQL备份工具,监控工具与发行版。他们的看法在相当程度上代表了 MySQL 社区的想法。
作者:Peter Zaitsev,Percona 老板,原文:How Can MySQL Catch Up with PostgreSQL’s Momentum?
MySQL还能跟上PostgreSQL的步伐吗?
当我与MySQL社区的老前辈交谈时,我经常听到这样的问题:“为什么MySQL如此出色,依然比PostgreSQL更受欢迎(至少根据DB-Engines的统计方法),但它的地位却在不断下降,而PostgreSQL的受欢迎程度却在不可阻挡地增长?” 在MySQL 生态能做些什么扭转这一趋势吗?让我们来深入探讨一下!
让我们看看为什么PostgreSQL一直表现如此强劲,而MySQL却在走下坡路。我认为这归结为所有权与治理、许可证、社区、架构以及开源产品的势能。
所有权和治理
MySQL 从未像 PostgreSQL 那样是“社区驱动”的。然而,当 MySQL 由瑞典小公司 MySQL AB 拥有,且由终身仁慈独裁者(BDFL)Michael “Monty” Widenius掌舵时,它获得了大量的社区信任,更重要的是,大公司并没有将其视为特别的威胁。
现在情况不同了——Oracle 拥有 MySQL,业界的许多大公司,特别是云厂商,将 Oracle 视为竞争对手。显然它们没有理由去贡献代码与营销,为你的竞争对手创造价值。此外,拥有 MySQL 商标的 Oracle 在 MySQL 上总是会有额外的优先权。
相比之下,PostgreSQL 由社区运营,领域内的每个商业供应商都站在同一起跑线上—— 像 EDB 这样的大公司与PostgreSQL 生态系统中的小公司相比,没有特殊的优待。
这意味着大公司更愿意贡献并推荐 PostgreSQL 作为首选,因为这不会为他们的竞争对手创造价值,而且他们对PostgreSQL 项目的方向有更大的影响力。数百家小公司通过本地“草根”社区的开发和营销努力,使 PostgreSQL 在全球无处不在。
MySQL社区能做些什么来解决这个问题? MySQL 社区能做的很少——这完全掌握在 Oracle 手中。正如我在《Oracle能拯救MySQL吗?》中所写,将 MySQL 移交给一个中立的基金会(如 Linux 或 Kubernetes 项目)将提供与 PostgreSQL 竞争的机会。不过,我并不抱太大希望,因为我认为Oracle此刻更感兴趣的是“硬性”变现,而不是扩大采用率。
许可证
MySQL 采用双许可证模式: GPLv2 和可从 Oracle 购买的商业许可证,而PostgreSQL则采用非常宽松的 PostgreSQL 许可证。
这实际上意味着您可以轻松创建使用商业许可的 PostgreSQL衍生版本,或将其嵌入到商业许可的项目中,而无需任何“变通方法”。构建此类产品的人们当然是在支持和推广 PostgreSQL。
MySQL 确实允许云供应商创建自己的商业分支,具有MySQL兼容性的 Amazon Aurora 是最知名和最成功的此类分支,但在软件发行时这样做是不允许的。
MySQL社区能做什么? 还是那句话,能做的不多 ——唯一能在宽松许可证下重新授权MySQL的公司是Oracle,而我没有理由相信他们会想要放松控制,尽管“开放核心”和“仅限云”的版本通常与宽松许可的“核心”软件配合良好。
社区
我认为,当我们考虑开源社区时,最好考虑 三个不同的社区,而不仅仅是一个。
首先,用户社区。MySQL在这方面仍然表现不错,尽管 PostgreSQL 正日益成为新应用的首选数据库。然而,用户社区往往是其他几个社区工作的成果。
其次,贡献者社区。PostgreSQL 有着更强大的贡献者社区,这并不奇怪,因为它是由众多组织而非单一组织驱动的。我们举办了针对贡献者的活动,还编写了关于如何为 PostgreSQL 作出贡献的书籍。PostgreSQL 的可扩展架构也有助于轻松扩展 PostgreSQL,并公开分享工作成果。
最后,供应商社区。我认为这正是主要问题所在,没有那么多公司有兴趣推广 MySQL,因为这样做可能只是为Oracle 创造价值。你可能会问,这难道不会鼓励所有 Oracle 的“合作伙伴”去推广 MySQL 吗?可能会,在全球范围内也确实有一些合作伙伴支持的MySQL活动,但这些与供应商对 PostgreSQL 的支持相比,简直微不足道,因为这是 “属于他们的项目”。
MySQL社区能做什么? 这里社区还是可以发挥一点作用的 —— 尽管当前的状况使得工作更困难,回报更少,但我们仍然可以做很多事情。如果你关心 MySQL 的未来,我鼓励你组织与参与各种活动,尤其是在狭窄的 MySQL生态之外,去撰写文章、录制视频、出版书籍。在社交媒体上推广它们,并将它们提交到 Hacker News。
特别是,不要错过 FOSDEM 2025 MySQL Devroom 的征稿!
这也是 Oracle 可以参与的部分,它们可以在不减少盈利的情况下参与这些活动,并与潜在的贡献者互动 —— 举办一些外部贡献者可以参与的活动,与他们分享计划,支持他们的贡献 —— 至少在他们与你的“MySQL社区”蓝图一致的情况下。
架构
一些 PostgreSQL 同行认为,PostgreSQL 发展势头更好的原因源于更好的架构和更干净的代码库。我认为这可能是一个因素,但并非主要原因,这里的原因值得讨论。
PostgreSQL 的设计高度可扩展,而且已经实现有大量强大的扩展插件,而 MySQL 的扩展可能性则非常有限。一个显著例外是存储引擎接口 —— MySQL支持多种不同的存储引擎,而 PostgreSQL 只有一个(尽管像 Neon 或 OrioleDB 这样的分叉可以通过打补丁来改变这一点)。
这种可扩展性使得在 PostgreSQL 上进行创新更加容易,(特别是PG还有着一个更强大的贡献者社区支持),而无需将新功能纳入核心代码库中。
MySQL社区能做些什么? 我认为即使 MySQL 的可扩展性很有限,我们仍然可以通过MySQL已经支持的各种类型的插件和“组件”来实现很多功能。
我们首先需要为MySQL建立一个“社区插件市场”,这将鼓励开发者构建更多插件并让它们得到更多曝光。我们还需要Oracle的支持 —— 承诺扩展MySQL的插件架构,赋能开发者构建插件 —— 即使这会与Oracle的产品产生一些竞争。例如,如果 MySQL 有插件可以创建自定义数据类型和可插拔索引,或许我们已经会看到 MySQL 的 PGVector替代品了。
开源产品的势头
选择数据库是一个长期的赌注,因为更换数据库并不容易。去问问那些几十年前选择了 Oracle 而现在被其束缚的人吧。这意味着在选择数据库时,你需要考虑未来,不仅要考虑这些数据库在十年后是否依然存在,而且要考虑随着时间的发展,它是否还能满足未来的技术需求。
正如我在文章 《Oracle最终还是杀死了MySQL!》 中所写到的,我认为Oracle已经将大量开发重心转移到专有商业版和云专属的 MySQL 版本上 —— 几乎放弃了 MySQL 社区版。虽然今日的 MySQL 仍然在许多应用中表现出色,但它确实正在落后过气中,MySQL 社区中的许多人都在质疑它是还有未来。
MySQL社区能做什么? 还是那句话,决定权在 Oracle 手中,因为他们是唯一能决定 MySQL 官方路线的人。你可能会问,那么我们的 Percona Server for MySQL 呢?我相信在Percona,我们确实提供了一个领先的 Oracle MySQL的开源替代品,但因为我们专注于完整的 MySQL 兼容性,所以必须谨慎对待对 MySQL 所做的变更,以避免破坏这种兼容性或使上游合并成本过高。MariaDB 做出了不同的利弊权衡;不受限制的创新使其与MySQL 的兼容性越来越差,而且每个新版本都离 MySQL 越来越远。
MariaDB
既然提到了MariaDB,你可能会问,MariaDB 不是已经尽可能地解决了所有这些问题吗?—— 毕竟 MariaDB 不是由 MariaDB基金会等机构管理的吗?别急,我认为MariaDB是 一个有缺陷的基金会,它并不拥有所有的知识产权,尤其是商标,无法为所有供应商提供公平的竞争环境。它仍然存在商标垄断问题,因为只有一家公司可以提供所有 “MariaDB” 相关的服务,地位高于其他所有公司。
然而,MariaDB 可能有一个机会窗口;随着 MariaDB(公司)刚刚被K1收购,MariaDB的治理和商标所有权有机会向 PostgreSQL 的模式靠近。不过,我并不抱太大希望,因为放松对商标知识产权的控制并不是私募股权公司所惯常做的。
当然,MariaDB 基金会也可以选择通过将项目更名为 SomethingElseDB 来获得对商标的完全控制,但这意味着MariaDB 将失去所有的品牌知名度;这也不太可能发生。
MariaDB 也已经与 MySQL 有了显著的分歧,调和这些差异将需要多年的努力,但我认为如果有足够的资源和社区意愿,这也许是一个可以解决的问题。
总结
正如你所看到的,由于 MySQL 的所有权和治理方式,MySQL 社区在其能做的事情上受到限制。从长远来看,我认为 MySQL 社区唯一能与 PostgreSQL 竞争的方法是所有重要的参与者联合起来(就像Valkey项目那样),在不同的品牌下创建一个 MySQL 的替代品 —— 这可以解决上述大部分问题。
开源“暴君”Linus清洗整风
最近Linus在项目中踢出了几位俄罗斯籍开发者,引发开源世界中的一片哀嚎声。但其实很多人都忘记了,Linux 是 Linus 的个人项目,三十年前是,现在也依然是。Linus 本人始终亲自掌握着开源项目的最高权力 —— Linux 的发布权。Linux 社区本质是帝制的 —— 而 Linus 本人就是最早且最成功的技术独裁者。
Ok, lots of Russian trolls out and about.
It’s entirely clear why the change was done, it’s not getting reverted, and using multiple random anonymous accounts to try to “grass root” it by Russian troll factories isn’t going to change anything. And FYI for the actual innocent bystanders who aren’t troll farm accounts - the “various compliance requirements” are not just a US thing.
If you haven’t heard of Russian sanctions yet, you should try to read the news some day. And by “news”, I don’t mean Russian state-sponsored spam.
As to sending me a revert patch - please use whatever mush you call brains. I’m Finnish. Did you think I’d be supporting Russian aggression? Apparently it’s not just lack of real news, it’s lack of history knowledge too.
Linus
在开源/自由软件社区,有 BDFL(“Benevolent Dictator for Life”,译为“仁慈的终身独裁者”)的说法。例如 Python 之父 Guido van Rossum,与 Linux 之父 Linus Torvalds。当然在很多人眼中,Linus 算不上 “仁君”,而是一个“暴君”,比如,Linus 经常使用直白粗俗的语言,公开斥责羞辱批评其他技术,参与者,厂商。
但这个 “暴君” 几十年如一日地在挖土,并且把自己的劳动毫无保留的贡献给别人,无数操作系统公司籍此赚的钵满盆翻。而正所谓 “升米恩,斗米仇” —— 时间一长,大家习惯了他的慷慨,却忘记了这个项目从头到尾,都是 Linus 本人的 “兴趣” 。在 Linus 自传的书名《Just for Fun》中,这一点体现的淋漓尽致 —— Linus 项目只是 Linux 本人的 Hobby。
能够约束 Linus 本人的,也就只有 Linux 项目使用的 GPL 协议 —— 他既没有成立公司搞商业化,也没有阻止其他人复制它。开源社区就是这样,太平洋也没加盖,代码都放在那里,你行你就上,搞个 fork 分叉呗?我一点儿也不怀疑,如果 Linus 本人哪天薨了,Linux 项目很快就会散作满天星,分叉满天飞了。
按照开源社区的习惯法,如果有人对此感到不满,完全可以自己做个 Fork 和上游比拼生产力,发起一场斯巴达克斯式的造反运动。例如 GCC 之前由于理念不同也分裂过,后来支线干的比主线好,更受开发者欢迎,这个支线(EGCS)就成新主线了。正所谓:“Talk is cheap, show me the code”, “You can you up, no can no BB” —— 而不是逼逼叨跟怨妇似的高呼:“ Linus 大王你变了” 或者 “Linus 大傻逼”,并指望天降正义。
当然,在我看来,Linus 这次做法并不好,但不是因为他把老毛子开发者给踢了。而是因为他没有用光明正大,堂堂正正的方式踢掉老毛子。而是由二号位采取比较遮掩,含糊的形式做了这件事,然后 Linus 合并,并在事后用胡扯蛋式的回复来回应,留下了一些破坏开源社区习惯法的污点瑕疵。
他要是光明正大的说:“我收到米帝的制裁禁令,要干老毛子”。或者干脆就两手一摊 “老子爱咋样咋样,你们管不着” —— Which is fact —— 说不定就没这么多事了。
老冯评论
全球化的时代过去了,逆全球化的风雨已经吹进了开源社区中。上古竞于道德的时代过去了,而当今争于气力。在从全球化走向区域化的大趋势中,一定会发生的事情就是 “共同体(社区)边界的重新划定”,或者干脆就是老的全球性大社区分裂成几个新的小社区。
而在这个划界过程中,必然会出现“他者”与“敌人”。有实质内容的理想,必然会制造出敌人 —— 没有敌人,说明你的社区理念没有实质内容,也就不会有真正的支持者。理想是权力欲望的最高形态,而邪恶是权力的内在本质,理想和邪恶不可分离,犹如爱情和嫉妒不可分离一样。
Linus 很明显已经划出了一道新的边界,将老毛子划出了社区边界之外 —— 一场 “清洗整风” 运动,尽管被许多人认为这是“邪恶”的,然而这正是其权力意志与“主权”的体现,嘴炮与谴责在实力面前太过廉价,改变不了什么。
而被划除在社区边界之外的老毛子,以及有较大概率步其后尘的老中,确实应该好好思考一下以后的道路该怎么走了。
参考阅读
先优化碳基BIO核,再优化硅基CPU核
先优化生物核,再优化硅内核
企业痴迷于 AI 的一个重要原因是,它有可能显著降低程序员的薪酬成本。如果一个公司需要 10 名程序员完成一项任务,而每个程序员的年薪为 20 万美元,那这就是一个每年 200 万美元的问题。如果 AI 能砍掉四分之一的成本,他们就能省出 50 万美元!如果能砍一半那就是 100 万美元!提高效率在程序员的薪资成本上会很快转化为利润!
这就是为什么我喜欢 Ruby!这就是我搞 Rails 的原因!过去 20 年,我一直坚信编程领域的趋势是:程序员的成本会越来越高,而计算机的成本却在不断下降。因此,聪明的做法是提高程序员的生产力,即使以牺牲计算机资源为代价!
很多程序员难以理解这一点 —— 他们实际上是非常昂贵的“生物计算核”,而且是真正稀缺的资源。而硅制计算内核却非常丰富,成本也在不断下降。所以随着时间推移,用计算机的时间换取程序员生产力的交易会越来越划算。AI 是实现此目标的方式之一,但像 Ruby on Rails 这样的工具从一开始关注的也是这个问题。
我们再看看那个年薪 20 万美元的程序员。你可以从 Hetzner 租用 1 个 AMD EPYC CPU核,年租金是 55 美元(批发模式,一台 48 核的服务器月租金 220 $元,所以 220 x 12 / 48 = 55)。这意味着一个生物核的价格,相当于 3663 个硅基核。如果你能让生物核的效率提高 10%,你就相当于节省了 366 个硅核的成本。如果你能让生物核的效率提高 25%,那你就相当于节省了接近一千个硅基核!
但是,许多“软绵绵的”生物编程内核对它们的硅制同类怀有一种独特的人类同情,这种情感超越了理性的数学计算。他们单纯地觉得 —— 自己可以花更多时间,通过使用对自己不高效、但对硅内核更高效的工具和技术,来减少硅内核的负担,而不是要求硅内核做更多的工作。对于某些人来说,减轻硅内核的负担几乎成了一种道德责任,似乎他们认为自己有义务尽量承担这些任务。
从艺术和精神层面上讲,我其实还挺尊重这种做法的!让计算机用更少的资源完成更多任务,确实有一种美好的感觉。我依然对 Commodore 64 和 Amiga 时代的 Demo 充满怀念。当年那些技术高手仅用 区区4KB 就能让计算机呈现出惊艳的音画效果,实在是令人难以置信。
然而在大多数情况下,这种做法在经济上并不划算。当然,在计算性能的前沿阵地上依然需要有人去挖掘最后一丝性能。比如,需要有人从 NVIDIA 4090 显卡中榨干最后一滴性能,我们的 3D 引擎才能在 4K / 120FPS 下进行光线追踪。但这对于软件行业中的绝大多数业务场景都不现实 —— 它们的业务是写业务软件!对于这类工作,不需要什么史诗级优化,计算机在很久以前就已经足够快了。
这也是我过去 20 年来,我一直在做的工作!开发业务软件并将其作为 SaaS 销售。整个行业都在做同样的事情,带来了巨大的利润和就业机会。这是一次历史性的牛市行情,主要由使用高级语言解决业务逻辑的程序员驱动 —— 他们找出产品与市场的契合点(PMF)来推动进步。
所以,每当听到关于计算效率的讨论时,你应该想起这个“软绵绵的”生物核。世界上大多数软件的价格都是基于它们的人工成本,而不是所需的硅内核。因而哪怕只是稍微提高生物核的生产力,也值得在硅芯片采购上花大钱。而且这种成本效益的比例,只会年复一年更偏向于充分利用生物核。
—— 至少在 AGI 霸主到来前,生物核都不会彻底过时!但没有人知道这一天何时会来,或者是否会到来。所以最好着眼于当下的经济学,选择对你来说最能提高生产力的工具链,并相信快乐的程序员将是你投资中最划算的一笔。
作者:David Heinemeier Hansson,DHH,37 Signal CTO,Ruby on Rails 作者
译者:冯若航,PostgreSQL Hacker,开源 RDS PG —— Pigsty 作者,数据库老司机,云计算泥石流。
优先优化生物内核,其次是硅内核 @ 2024-09-06
老冯评论
DHH 的博客一如既往地充满洞见 —— 虽然事实听上去可能并不讨喜,但程序员本质上也是一种生物计算核 —— Bio Core ,而很多程序员已经忘记了这一点。
实际上在一百年前,Computer 指的还是 “计算员” 而非 “计算机”;而在上世纪四五十 年代,算力的衡量单位更一度是 —— “Kilo-Girls”,即一千名女孩的计算速度,类似的单位还有 kilo-girl-hour
等。当然,随着信息技术的突飞猛进,这些枯燥乏味的计算活计都交给计算机了,程序员得以专注于更高层次的抽象和创造。
对于我所在的数据库行业,我认为这篇文章能带给用户的一个启示是 —— 数据库的真正瓶颈早就不是 CPU 硅基核了,而是能用好数据库的生物核。对于绝大多数用例,数据库的瓶颈早已不再是 CPU,内存, I/O,网络,存储,而是开发者与 DBA 的思维,认知,经验,智慧。
因此,没人会在乎你的数据库能否支持 100 万 TPS,而是你的软件是否能用最小的时间成本,复杂度成本,认知成本解决问题。 易用性、简单性、可维护性成为了竞争的焦点 —— 专注于此道的 RDS 数据库服务也因此大获成功(同理还有 Neon, Supabase,Pigsty 等)。
像 AWS 这样的云厂商拿着开源的 MySQL 和 PostgreSQL 内核一路杀到了数据库市场一哥的位置,是因为 AWS 比 Oracle / EDB 有更深的数据库内核造诣,懂得如何利用硅基核吗?非也。而是因为比起优化硅基核,他们更懂得如何优化生物核 —— 他们懂得如何让开发者,DBA,运维更容易用好数据库 —— 用好数据库,而非制造数据库,成为了新的核心瓶颈点。
所以,传统数据库内核是一个夕阳产业,将与格力空调,联想电脑一样成为低毛利的制造业。 而真正的高科技与技术创新,将发生在数据库管控上 —— 用软件辅助、赋能、甚至冒天下之大不韪的“替代” 一部分开发者 —— 如何用好数据库内核与硅基CPU核,提高生物核的生产力,降低认知成本,简化复杂度,提高易用性。这才是未来数据库行业的发展方向。
高科技行业就是要依靠技术创新驱动。如果你能用开源 PG 内核替代 Oracle ,SQL Server,那别人也能 —— 最好的结果无非就是甲骨文微软都放弃传统数据库转型做云服务,传统数据库成为低利润的制造业。正如二十年的 PC 行业一样。二十年前 IBM 戴尔惠普都是国际玩家,中国联想说要做到世界一流。今天看联想确实做到了,但是 PC 行业早就不是高科技行业了,只是一个最无聊普通的制造业。
即使是在国内看起来很能打的真自研分布式数据库内核,如果选错了赛道,那所能期待的最好结局也不过是成为数据库行业的长虹,赚五个点的利润。然后被拿着开源 PostgreSQL 内核提供服务的 云厂商 RDS 和本地优先 RDS 骑脸输出,最终成为数据库领域的 “Kilo-Girl”。
Optimize for bio cores first, silicon cores second
David Heinemeier Hansson 2024-09-06
A big part of the reason that companies are going ga-ga over AI right now is the promise that it might materially lower their payroll for programmers. If a company currently needs 10 programmers to do a job, each have a cost of $200,000/year, then that’s a $2m/year problem. If AI could even cut off 1/4 of that, they would have saved half a million! Cut double that, and it’s a million. Efficiency gains add up quick on the bottom line when it comes to programmers!
That’s why I love Ruby! That’s why I work on Rails! For twenty years, it’s been clear to me that this is where the puck was going. Programmers continuing to become more expensive, computers continuing to become less so. Therefore, the smart bet was on making those programmers more productive EVEN AT THE EXPENSE OF THE COMPUTER!
That’s what so many programmers have a difficult time internalizing. They are in effect very expensive biological computing cores, and the real scarce resource. Silicon computing cores are far more plentiful, and their cost keeps going down. So as every year passes, it becomes an even better deal trading compute time for programmer productivity. AI is one way of doing that, but it’s also what tools like Ruby on Rails were about since the start.
Let’s return to that $200,000/year programmer. You can rent 1 AMD EPYC core from Hetzner for $55/year (they sell them in bulk, $220/month for a box of 48, so 220 x 12 / 48 = 55). That means the price of one biological core is the same as the price of 3663 silicon cores. Meaning that if you manage to make the bio core 10% more efficient, you will have saved the equivalent cost of 366 silicon cores. Make the bio core a quarter more efficient, and you’ll have saved nearly ONE THOUSAND silicon cores!
But many of these squishy, biological programming cores have a distinctly human sympathy for their silicon counterparts that overrides the math. They simply feel bad asking the silicon to do more work, if they could spend more of their own time to reduce the load by using less efficient for them / more efficient for silicon tools and techniques. For some, it seems to be damn near a moral duty to relieve the silicon of as many burdens they might believe they’re able carry instead.
And I actually respect that from an artsy, spiritual perspective! There is something beautifully wholesome about making computers do more with fewer resources. I still look oh-so-fondly back on the demo days of the Commodore 64 and Amiga. What those wizards were able to squeeze out of a mere 4kb to make the computer dance in sound and picture was truly incredible.
It just doesn’t make much economic sense, most of the time. Sure, there’s still work at the vanguard of the computing threshold. Somebody’s gotta squeeze the last drop of performance out of that NVIDIA 4090, such that our 3D engines can raytrace at 4K and 120FPS. But that’s not the reality at most software businesses that are in the business of making business software (say that three times fast!). Computers have long since been way fast enough for that work to happen without heroic optimization efforts.
And that’s the kind of work I’ve been doing for said twenty years! Making business software and selling it as SaaS. That’s what an entire industry has been doing to tremendous profit and gainful employment across the land. It’s been a bull run for the ages, and it’s been mostly driven by programmers working in high-level languages figuring out business logic and finding product-market fit.
So whenever you hear a discussion about computing efficiency, you should always have the squishy, biological cores in mind. Most software around the world is priced on their inputs, not on the silicon it requires. Meaning even small incremental improvements to bio core productivity is worth large additional expenditures on silicon chips. And every year, the ratio grows greater in favor of the bio cores.
At least up until the point that we make them obsolete and welcome our AGI overlords! But nobody seems to know when or if that’s going to happen, so best you deal in the economics of the present day, pick the most productive tool chain available to you, and bet that happy programmers will be the best bang for your buck.
MongoDB没有未来:好营销救不了烂芒果
这两天 MongoDB 整的营销花活让人眼花缭乱:《MongoDB向PostgreSQL宣战》,《MongoDB 击败 PostgreSQL 赢下价值 300 亿美元项目》,以及原文 The Register 的《MongoDB在战胜强敌之后准备乱拳干翻 PostgreSQL》,活生生一副要乱拳打死老师傅的架势。
有朋友得意洋洋的特意转给我想看 PG 的笑话,这着实让我感到无奈 —— 这么离谱的新闻都有人信! 但事实是 —— 这么离谱的东西真就有人信! 包括某些CEO也照样会中招翻车。诚如石破天祖师爷所说:“永远不要低估好营销对烂产品的影响”。
把东西卖给估值300亿的公司,和做 300 亿的项目完全是两码事。当然,这不能怪人家眼拙,这是 MongoDB 在营销上的一贯伎俩 —— 如果不仔细看原文,很难区分这个 300 亿指的是项目价值还是公司估值。
在当下,MongoDB 在产品和技术上乏善可陈;在正确性,性能,功能以及各种维度上被 PostgreSQL 按在地上摩擦;在开发者中的流行度与口碑,以及DB-Engine 热度都不断下滑,MongoDB 公司本身也不赚钱,股价也刚经过大腰斩,亏损继续扩大;“营销” 也许是 MongoDB 唯一能拿出手的东西了。
然而诚信是商业的根本,“好营销救不了烂芒果”,建立在谎言与忽悠之上的营销不会有好下场。今天我就来带大家看看,MongoDB 营销的锦绣丝绸被套里,填进去的都是些什么烂棉花。
烂产品靠营销上位
图灵奖得主,数据库祖师爷 Stonebraker 老爷子在最近在 SIGMOD 2024 发表的名著级论文《What goes around comes around… And Around》中对此有过精辟的评价:“绝对不要低估好营销对烂产品的影响 —— 比如 MySQL 与 MongoDB”。
这个世界上有许多烂数据库 —— 但能用三寸不烂之舌把烂货成功吹成宝贝卖出去的,MongoDB 说自己是第一,MySQL 也只自认老二屈居人下。
在所有关于 MongoDB 大忽悠的故事中,最让人印象深刻的是 LinkedIn 上的这篇《MongoDB 3.2 —— 现由 PostgreSQL 强力驱动》 。 这篇文章的精彩之处在于,它是由 MongoDB 合作伙伴发出的血泪控诉:MongoDB 无视了自己合作伙伴的忠言劝告,拿了一个 PostgreSQL 伪装成自己的分析引擎,并在发布会上忽悠用户。
作者作为 MongoDB 在分析领域的合作伙伴彻底灰心丧气,公开撰文发起控诉 —— “MongoDB 的分析引擎是一个 PostgreSQL ,那你们真还不如直接去用 PostgreSQL”。
像这样刻意造假忽悠的案例绝非个例,MongoDB 还在贬低同业产品自抬身价上有诸多记录。例如在官网文章《从PostgreSQL迁移到MongoDB》中,MongoDB 宣称自己是 “可扩展灵活的新一代现代通用数据库”, 而 PostgreSQL 是 “复杂且容易出错的老旧单片关系数据库”。完全无视了其实自己在整体的性能,功能,正确性,甚至自己标榜的应对大数据量的吞吐与可伸缩性上完全被 PostgreSQL 吊打的事实。
功能被PGSQL覆盖
JSON 文档确实是一个很受互联网应用开发者喜爱的特性。然而提供这一能力的数据库并非只有 MongoDB 。PostgreSQL 在十年前就已经提供了 SOTA 水平的 JSON 支持,并且仍然在不断演进改善。
PostgreSQL 的 JSON 支持是所有关系型数据库中最成熟与最早的(2012-2014),早于 SQL/JSON 标准或者说直接影响了 SQL/JSON 标准建立(2016)。 更重要的是,它的文档特性实现质量很高。相比之下 —— 同样在营销上号称支持 JSON 的MySQL,实际上是个简陋的 BLOB 换皮,跟 9.0 向量类型有一拼)。
数据库祖师爷 Stonebraker 表示过,带有可扩展类型的关系模型早已覆盖了数据库世界的各个角落,而 NoSQL 运动是数据库发展历史上的一段弯路:关系模型是向下兼容文档模型的。 文档模型跟几十年前范式化 vs 反范式化的大讨论实质是一样的 —— 1.只有有任何非一对多的关系,就会出现数据重复;2. 用预计算的JOIN未必比现场JOIN更快;3 数据没有独立性。 用户可以假设自己的应用场景是独立 KV 式缓存访问,但哪怕只要添加一个稍微复杂一点的功能,开发者就会面临几十年前就讨论过的数据重复困境。
PostgreSQL 在功能上是 MongoDB 的上位替代,所以可以对 MongoDB 的用例做到向下兼容 —— PostgreSQL 能做的MongoDB 做不了;而 MongoDB 能做的 PostgreSQL 也能做:你可以在PG中创建一个只有 data JSONB
列的表,然后使用各种 JSON 查询与索引来处理这里的数据;如果你确实觉得花几秒钟建表仍然是一个额外负担,那么在生态中还有各种各样基于 PostgreSQL 提供 MongoDB API,甚至 MongoDB 线缆协议的解决方案。
例如,FerretDB 项目通过中间件的方式在 PostgreSQL 集群上实现了 MongoDB 线缆协议兼容性 —— MongoDB 应用甚至都不需要更换客户端驱动,修改业务代码就能迁移到 PostgreSQL 上。
(另一被原位兼容的是 SQL Server ); PongoDB 则是直接在 NodeJS 客户端驱动侧将 PG 仿真成一个 MongoDB。
此外还有 mongo_fdw
,可以让 PG 从 MongoDB 中用 SQL 读取数据,wal2mongo
将 PG 变更抽取为 BSON。
例如 FerretDB 项目通过中间件的方式在 PostgreSQL 集群上实现了 MongoDB 线缆协议兼容性 —— MongoDB 应用甚至都不需要更换客户端驱动,修改业务代码就能迁移到 PostgreSQL 上。(另一被原位线缆兼容的是 SQL Server );PongoDB 则是直接在 NodeJS 客户端驱动侧将 PG 仿真成一个 MongoDB。此外还有 mongo_fdw
,可以让 PG 从 MongoDB 中用 SQL 读取数据,wal2mongo
将 PG 变更抽取为 BSON。
在易用性上,各家云厂商都推出了开箱即用的 PG RDS 服务,想要开源自建也有 Pigsty 这样开箱即用的解决方案,还有 Serverless 的 Neon 更是让PG上手门槛低到一行命令就能直接用起来。
此外,相比于 MongoDB 使用的 SSPL 协议(已经不再是一个开源协议了),PostgreSQL 使用的类 BSD 开源协议显然要友善的多,PG可以在不需要软件授权费的情况下,提供更好的上位功能替代 —— Do more pay less! 不赢都难。
正确性与性能被吊打
对于数据库来说,正确性至关重要 —— 中立的分布式事务测试框架 JEPSEN 对 MongoDB 的正确性做过评测:结果可以用 “一塌糊涂”形容(BTW:另一个难兄难弟是 MySQL)。
当然,MongoDB 的强项就是面不改色心不跳的 “忽悠“,尽管 JEPSEN 提了这么多的问题,在 MongoDB 官网上,关于 Jespen 的评测是这么介绍的:”到目前为止,因果一致性通常仅限于研究项目……MongoDB 是我们所知的第一个提供实现的商业数据库之一“
这个例子再次体现了 MongoDB 在营销上的脸皮 —— 用一种极其精致的语言艺术,从一大坨 Bullshit 中精心挑选出了一颗未消化的花生米,而一笔带过在正确性/一致性上的各种致命硬伤。
另一个有趣的点是性能。作为一个专用的文档数据库,性能 应当是其相对于通用数据库的杀手级特性。
先前有一篇《《从 MongoDB 到 PostgreSQL 的大迁移》引发了 MongoDB 用户的关注,我的用户群里有位朋友 @flyingcrp 问了这样一个问题 —— 为什么PG上的一个插件或者功能点就能顶得上别人一个完整的产品?
当然也不乏持相反观点的朋友 —— PG的 JSON 性能肯定比不过细分领域的专业产品 —— 一个专用数据库如果连性能都干不过通用数据库,那还活个什么劲儿?
这个讨论引起了我的兴趣,这些命题成立吗?于是,我做了一些简单的检索与研究,结果发现了一些非常有趣且震惊的结论:例如,在 MongoDB 的看家本领 —— JSON 存储与检索性能上,PostgreSQL 已经吊打 MongoDB 了。
来自 ONGRES 与 EDB 的一份 PG vs Mongo 性能对比评测报告 详细对比了两者在 OLTP / OLAP 上的性能,结果一目了然。
另一份更近一点的性能对比 着重测试了 JSONB / GIN 索引下的表现对比,得出的结论也是:PostgreSQL JSONB 列是 MongoDB 的替代。
在当下,单机 PostgreSQL 性能 可以轻松 Scale 到几十TB ~ 几百TB数量级,支撑几十万的点写入 QPS 与几百万的点查询 QPS。只用 PostgreSQL 支撑业务到百万日活 / 百万美元营收甚至直接 IPO 都毫无问题。
老实说,MongoDB 的性能已经完全跟不上时代了,而它引以为傲的“内置分片”可伸缩性,在软件架构与性能突飞猛进,硬件遵循摩尔定律指数发展 的当下显得毫无意义。
流行度热度在衰退
如果我们观察 DB-Engine 热度分数,不难看出过去十年中,拥有最大增长的两个数据库就是 PostgreSQL 与 MongoDB 。可以说这两者是移动互联网时代中数据领域的最大赢家。
但它们的区别在于,PostgreSQL 仍然在继续增长,甚至已经在 StackOverflow 全球开发者调研中,连续三年成为 最流行的数据库 并势头不减赢麻了。而 MongoDB 在 2021 年开始就掉头向下开始过气。使用率,口碑,需求度都出现了停滞或扭头向下的发展趋势:
在 StackOverflow 年度全球开发者调研中,提供了主要的数据库用户的转移关系图。不难看出,MongoDB 用户的最大流出项就是 PostgreSQL。而会去使用 MongoDB 的往往是 MySQL 用户。
MongoDB 和 MySQL 属于那种典型的 “面向初学者” 的数据库,针对小白做了许多无底线讨好性的妥协设计 —— 从统计中不难看出它们在新手中的使用率比专业开发者中更高。 与之相反的则是 PostgreSQL,在专业开发者中的使用比例要比新手中高得多。
任何开发者都会经历初学者状态,我最初也是从 MySQL / Mongo 开始与数据库打交道的,但很多人就止步于此,而有追求的工程师则会不断学习进步,提升自己的品味与技术鉴别力,使用更好用、更强大的技术来更新自己的武器库。
而趋势是:越来越多的用户在提升的过程中,从 MongoDB 和 MySQL 迁移到了上位替代 PostgreSQL 中。从而成就了新一代世界上最流行的数据库 —— PostgreSQL。
风评已然臭不可闻
许多使用过 MongoDB 的开发者都对其留下了极其恶劣的印象,包括我自己。我上一次和 MongoDB 打交道是在 2016 年。我们部门先前用 MongoDB 搭建了一套实时统计平台,存放全网应用下载/安装/启动计数器,几 TB 规模的数据。我负责把这套在线业务的 MongoDB 迁移到 PostgreSQL。
在这个过程中,我对 MongoDB 留下了糟糕的印象 —— 我花费了很多时间清洗 MongoDB 中模式错乱的垃圾数据。包括一些匪夷所思的问题(比如 Collection 里有整本的小说,SQL 注入的脚本,非法的零字符、Unicode码位与Surrogate Pair,各种花里胡哨的模式),堪称是一个史诗级的垃圾箱。
在这个过程中,我也深入研究了 MongoDB 的查询语言,并将其翻译为标准 SQL。我甚至使用 Multicorn 写了一个 MongoDB 的外部数据源包装器 FDW 来做到这一点,顺便还水了篇 关于 Mongo/HBase FDW 的论文。(比较巧的是,我那时候确实不知道 —— MongoDB 官方竟然也是这么用FDW干分析的!)
总体来说,在这趟深度使用与迁移过程中,我对 MongoDB 感到非常失望,感觉到自己的时间被毫无意义的东西给浪费掉了。 当然后来我也发现,并不是只有我一个人有这种感受,在 HN 和 Reddit 上有无数关于 MongoDB 的嘲讽与吐槽:
- 告别 MongoDB。迎接 PostgreSQL
- Postgres 性能优于 MongoDB,并引领开发者新现实
- MongoDB 已死。PostgreSQL 万岁 :)
- 你永远不应该使用 MongoDB 的理由
- SQL vs NoSQL 决斗。Postgres vs Mongo
- 为什么我放弃了 MongoDB
- 为什么你永远永远永远不该使用 MongoDB
- Postgres NoSQL 比 MongoDB 更好吗?
- 再见 MongoDB。你好 PostgreSQL
关于这篇《MongoDB挑战PG》的新闻,HN评论区是这样的:
关于 MongoDB,Reddit 里的评论是这样的:
能让开发者专门抽出时间写文章来骂它,MongoDB 的恶劣营销功不可没:
能让合作伙伴破口大骂,吹哨揭发,我看 MongoDB 也是独此一家:
MongoDB没有未来
Stonebraker 表示过,带有可扩展类型的关系模型早已覆盖了数据库世界的各个角落,而 NoSQL 运动是数据库发展历史上的一段弯路。 《种瓜得瓜》一文认为未来文档数据库的发展趋势是向关系数据库靠拢,重新把自己当初“鄙视”的 SQL / ACID 给加回来,以弥补自己与 RDBMS 的智力差距,最终趋同于 RDBMS 。
但是问题就来了,如果这些文档数据库最终还是要变成关系数据库,那么为什么不直接用 PostgreSQL 关系数据库呢?难道用户可以指望 MongoDB 这孤家寡人的一家商业数据库公司,能够在这个赛道赶上整个 PostgreSQL 开源生态?—— 这个生态可是包含了几乎所有软件/云/科技巨头在内 —— 能战胜一个生态的,只有另一个生态。
在 MongoDB 不断重新发明 RDBMS 世界的各种轮子,拙劣地跟在 PG 后面亦步亦趋补课,又同时把 PG 描述为 “复杂且容易出错旧的单片关系数据库” 时。PostgreSQL 已经成长为一个超出 MongoDB 想象的多模态超融合数据库,它已经通过几百个扩展插件成为数据库领域的全能王霸主。JSON 仅仅是其武库中的冰山一角,还有 XML,全文检索,向量嵌入,AIML,地理信息,时序数据,分布式,消息队列,FDW,以及二十多种存储过程语言支持。
使用 PostgreSQL ,你可以做到许多超出想象的事情:你可以在数据库内发 HTTP 请求,用XPATH 解析,用 Cron 插件调度写爬虫,原地入库后用机器学习扩展分析,调用大模型创建向量Embedding 用图扩展构建知识图谱,用包括JS在内的二十多种语言编写存储过程,并在库内拉起 HTTP 服务器对外 Serve。这种匪夷所思的能力是 MongoDB 以及其他“纯”关系型数据库难望其项背的。
MongoDB 根本没有与 PostgreSQL 在产品、技术上堂堂正正一战的能力,因此只能在营销上使阴招,暗搓搓的下绊子,但这种做法只会让更多人看清它的真面目。
作为一个上市公司,MongoDB 的股价也已经经历了一次大腰斩,而且亏损持续扩大。产品与技术上的落后,以及运营上的不诚信,都让人怀疑它的未来。
我认为任何开发者,创业者,投资人都不应该把赌注押在 MongoDB 上 —— 这确实是一个没有希望,也没有未来的数据库。
MongoDB: 现在由PostgreSQL强力驱动?
前言
明天我会发一篇批判 MongoDB 的文章,作为对其近期恶劣营销碰瓷 PostgreSQL 的回应。在那之前,我想先分享一篇在 2015 年时的精彩文章,揭露了 MongoDB 的一些黑历史。
这篇文章最经典的一点在于,它是由 MongoDB 的合作伙伴发出的血泪控诉,MongoDB 对尝试在生态中做分析的伙伴不屑一顾,而是跑去拿了一个 PostgreSQL 作为自己的分析引擎忽悠用户,从而让合作伙伴彻底灰心丧气的故事。
本文原文链接:https://www.linkedin.com/pulse/mongodb-32-now-powered-postgresql-john-de-goes (双向被墙状态,你需要开无痕模式挂代理方能访问)
作者:John De Goes —— 挑战 Ziverge 的现状
发布日期: 2015年12月8日
本文所述观点仅为个人看法,不代表我雇主的立场或观点。
在将各种线索综合起来后,我感到极度震惊。若我的猜测属实,MongoDB可能正要犯下我认为是数据库公司历史上最大的错误。
我是一个开源分析工具的开发者,该工具支持连接至如 MongoDB 这类的 NoSQL 数据库,我每天都在努力推动这些新一代数据库供应商更加走向成功。
事实上,我不久前在 MongoDB Days Silicon Valley 上向一室之内的众人进行了演讲,阐述了采纳这种新型数据库的诸多益处。
因此当我意识到这一潜在的破坏性秘密时,我立即敲响了警钟。在 2015 年 11 月 12 日,我发送了一封邮件至 MongoDB 的首席产品经理 Asya Kamsky。
尽管措辞谦和,但我表达得十分明确:MongoDB 正在犯下一个巨大的错误,并应当在还有机会纠正前,重新考虑自身决策。
然而我没有再接收到 Asya 或其他任何人的回应。我曾成功劝说 MongoDB 改变策略,避免将错误的功能商业化的经历,这一次未能重演。
以下我如何从新闻稿、YouTube 视频以及散布在 Github 上的源代码中找到线索,以及我最终未能说服 MongoDB 改变方向的经过。
故事起始于 2015 年 6 月 1 日,在纽约市举行的年度 MongoWorld 大会上。
MongoWorld 2015
SlamData 是我新创办的分析初创公司,它赞助了 2015 年的 MongoWorld,因此我得到了一个难得的 VIP 派对门票,得以参加大会前夜的活动。
活动在 NASDAQ MarketWatch 举行,地点优雅,俯瞰时代广场。我穿着工装裤和初创公司的 T 恤,明显感觉自己穿得不合时宜。高级小吃和酒水随意畅饮,MongoDB 的管理团队也全员出动。
我与 MongoDB 的新任 CEO Dev (“Dave”) Ittycheria 握了手,并对他未来的工作表示了几句鼓励。
今年早些时候,富达投资公司Fidelity Investments 将 MongoDB 的估值削减至 2013 年的一半(16 亿美元),将这个初创公司从“独角兽”降级为“驴子”。Dev 的任务就是证明 Fidelity 以及其他质疑者是错误的。
Dev 从 Max Schireson 手中接过了公司(Max 在 2014 年著名辞职),在任期间,Dev 组建了一个新的管理团队,对 MongoDB 整个公司产生了深远影响。
虽然我只与 Dev 交谈了几分钟,但他给我的感觉是聪明、友善的,而且非常渴望了解我公司正在做的事情。他递给我一张名片,并表示如果我有需要可以随时联系他。
接下来是 MongoDB 的 CTO 兼联合创始人 Eliot Horowitz。我与他握手,自我介绍,并用 30 秒钟介绍了我的初创公司。
当时,我觉得我的介绍一定很糟糕,因为 Eliot 对我说的每一句话似乎都不感兴趣。事实证明,Eliot 讨厌 SQL,把分析工具视为一种麻烦,所以不难理解我为什么让他感到无聊!
不过,Eliot 确实听到了“分析”这个词,并透露说第二天的大会上,MongoDB 将发布 3.2 版本的一些有趣的新消息。
我请求他透露更多细节,但不行,这些内容严格保密。我只能等到第二天,与全世界一同揭晓答案。
我将这个消息告诉了我的联合创始人 Jeff Carr,我们短暂地感到了一丝恐慌。对于我们这个由四个人组成、完全自筹资金的初创公司来说,最大的担忧是 MongoDB 会宣布推出自己的分析工具,这可能会影响我们融资的机会。
令人宽慰的是,第二天我们发现 MongoDB 的重大宣布并不是分析工具,而是一个名为 MongoDB BI Connector的解决方案,这是即将发布的 3.2 版本中的一个重要功能。
MongoDB 3.2 BI Connector
Eliot 得以宣布 BI 连接器的推出。尽管当天的公告众多,但他对这一连接器似乎不甚感兴趣,因此仅略加提及。
然而,详细信息很快通过一份官方新闻稿发布,其概括如下:
MongoDB 今日宣布推出一款全新的 BI 和数据可视化连接器,该连接器将 MongoDB 数据库与业界标准的商业智能(BI)及数据可视化工具相连。该连接器设计以兼容市场上所有符合 SQL 标准的数据分析工具,如 Tableau、SAP Business Objects、Qlik 以及 IBM Cognos Business Intelligence 等。目前该连接器处于预览阶段,预计将在 2015 年第四季度全面发布。
根据该新闻稿,BI 连接器将使全球任何 BI 软件都能与 MongoDB 数据库进行交互。
这则消息迅速在 Twitter 上[传播开来](https://twitter.com/search?f=tweets&vertical=default&q=mongodb bi connector&src=typd),并引发了媒体广泛报道。TechCrunch 等多家媒体均转载了这一消息,每次报道都为人们提供了新的细节,甚至《财富》杂志还宣称该 BI 连接器实际上已在 MongoWorld 发布!
考虑到公告的性质,媒体的这种热烈反响似乎是合理的。
当世界碰撞
MongoDB 与许多其他 NoSQL 数据库一样,不存储关系型数据。它存储的是复杂的数据结构,这些结构是传统的关系型 BI 软件无法理解的。MongoDB 的战略副总裁 Kelly Stirman 对此进行了精辟的解释:
“这些被称为现代应用的软件之所以如此命名,是因为它们采用了不适用于传统数据库行列格式的复杂数据结构。”
一个能让全球任何 BI 软件在这些复杂数据结构上进行强力分析而且不损失分析精度的连接器,无疑是重大新闻。
MongoDB 是否真的做到了不可能的事?他们是否开发出了一种既能满足所有 NoSQL 分析需求,又能在扁平化、统一的数据上暴露关系语义,使传统 BI 软件能够处理的连接器?
几个月前,我曾与 MongoDB 的产品副总裁 Ron Avnur 交谈。Ron 表示,所有 MongoDB 的客户都在寻求分析功能,但公司尚未决定是自主开发还是寻找合作伙伴。
这意味着 MongoDB 可能在短短几个月内,从一无所有迅速转变为拥有神奇解决方案。
掀开内幕
发布会结束后,我和 Jeff 回到了我们赞助商的展位。Jeff 问了我一个显而易见的问题:“他们怎么能在短短几个月内,从无到有做出一个能兼容所有 BI 工具的 BI 连接器?!”
我仔细思考了这个问题。
BI 连接器需要解决的诸多问题中,有一个是如何在 MongoDB 上高效执行类似 SQL 的分析任务。凭借我在分析领域的深厚背景,我知道要在像 MongoDB 这样现代数据库上高效地执行通用分析是非常具有挑战性的。
这些数据库支持非常丰富的数据结构,并且它们的接口是为所谓的操作型用例设计的(而不是分析型用例)。能够利用操作型接口在丰富的数据结构上运行任意分析的技术,需要多年的开发。不是你能在两个月内搞定的事情。
于是我直觉性地回答了 Jeff:“他们没有开发新的 BI 连接器。这不可能。这里面肯定有其他问题!”
具体是什么问题,我并不清楚。但在握手和发名片的间隙,我做了一些调查。
Tableau 展示了他们的软件与 MongoDB BI 连接器配合使用的演示,这引起了我的好奇心。Tableau 在关系型数据库上的可视化分析领域设立了标准,而他们前瞻性的大数据团队一直在认真思考 NoSQL。
借助与 MongoDB 的关系,Tableau 发布了一份与 MongoWorld 发布会同步的新闻稿,我在他们的网站上找到了这篇新闻稿。
我仔细阅读了这篇新闻稿,想要了解一些新的细节。在深处,我发现了一个微弱的线索:
MongoDB 将很快宣布连接器的测试版,计划在今年晚些时候 MongoDB 3.2 版本发布时提供正式版。在 MongoDB 的测试期间,Tableau 将通过我们的 PostgreSQL 驱动程序在 Windows 和 Mac 上支持 MongoDB 连接器。
这些话给了我第一个线索:通过我们的 PostgreSQL 驱动程序。这至少意味着 MongoDB 的 BI 连接器将使用与 PostgreSQL 数据库相同的“语言”(wire protocol)。
这让我感到有些可疑:MongoDB 是否真的在重新实现整个 PostgreSQL 的通信协议,包括对数百个 PostgreSQL 函数的支持?
虽然可能,但这看起来极其不可能。
我转向 Github,寻找 MongoDB 可能借鉴的开源项目。由于会议的 WiFi 不稳定,我不得不通过手机热点,查找了几十个同时提到 PostgreSQL 和 MongoDB 的仓库。
最终,我找到了我要找的东西:mongoose_fdw,一个由 Asya Kamsky(当时我不认识她,但她的简介提到她为 MongoDB 工作)分叉的开源仓库。
这个仓库包含一个所谓的 Foreign Data Wrapper (FDW) for PostgreSQL 数据库。FDW 接口允许开发者插入其他数据源,以便 PostgreSQL 可以提取数据并在这些数据上执行 SQL(为使 BI 工具正常工作,NoSQL 数据必须被展平、填充空值,并做其他简化处理)。
“我想我知道是怎么回事了。” 我对 Jeff 说。“看起来,他们可能在原型中将数据展平,然后使用另一个数据库来执行由 BI 软件生成的 SQL 语句。”
“什么数据库?” 他马上问道。
“PostgreSQL。”
Jeff 哑口无言。他一句话也没说。但我能完全明白他在想什么,因为我也在想同样的事。
糟了。这对 MongoDB 来说是个坏消息。真的很糟糕。
PostgreSQL:MongoDB 终结者
PostgreSQL 是一个流行的开源关系型数据库。它的受欢迎程度如此之高,以至于目前在排名上几乎与 MongoDB 并驾齐驱。
这个数据库对 MongoDB 构成了激烈竞争,主要原因是它已经获得了 MongoDB 的一些功能,包括存储、验证、操作和索引 JSON 文档的能力。第三方软件甚至赋予了它横向扩展的能力(或者我该说,巨量扩展的能力)。
每隔一个月左右,就会有人写文章推荐使用 PostgreSQL 而不是 MongoDB。这些文章往往会迅速传播,飙升到 HackerNews 网站的热榜。以下是其中一些文章的链接:
- 告别 MongoDB。迎接 PostgreSQL
- Postgres 性能优于 MongoDB,并引领开发者新现实
- MongoDB 已死。PostgreSQL 万岁 :)
- 你永远不应该使用 MongoDB 的理由
- SQL vs NoSQL 决斗。Postgres vs Mongo
- 为什么我放弃了 MongoDB
- 为什么你永远永远永远不该使用 MongoDB
- Postgres NoSQL 比 MongoDB 更好吗?
- 再见 MongoDB。你好 PostgreSQL
商业化 PostgreSQL 的最大公司是 EnterpriseDB(虽然还有很多其他公司,一些历史更悠久或同样活跃),它在官网上维护了一个大量的内容库,主张 PostgreSQL 是比 MongoDB 更好的 NoSQL 数据库。
无论你对这个观点有何看法,有一点是明确的:MongoDB 和 PostgreSQL 在开发者的认知中正进行着一场激烈而血腥的争夺战。
从原型到生产
任何有经验的工程师都会告诉你,原型不能直接用于生产环境。
即便 MongoDB 确实在使用 PostgreSQL 作为原型的 BI 连接器,也许某些聪明的 MongoDB 工程师正被关在某个房间里,努力开发一个独立的生产版本。
实际上,从 Tableau 的新闻稿措辞来看,依赖 PostgreSQL 驱动的情况可能只是暂时的:
在 MongoDB 的测试阶段,Tableau 将通过我们的 PostgreSQL 驱动程序,在 Windows 和 Mac 上支持 MongoDB 连接器。
我猜测,或许 MongoDB 3.2 版本发布时会带来真正的产品:一个能够暴露 MongoDB 所支持的丰富数据结构(而不是展平、填充空值和丢弃数据)、完全在数据库内执行所有查询,并且无需依赖竞争数据库的 BI 连接器。
七月,在 MongoWorld 结束一个多月后,我在一次商务旅行中顺道拜访了 MongoDB 在帕洛阿尔托的办公室。我所了解到的情况让我倍感鼓舞。
拜访 MongoDB
以帕洛阿尔托的标准来看,MongoDB 的办公室相当大。
之前几次去硅谷时,我看到过这家公司的招牌,但这是我第一次有机会进去看看。
就在前一周,我通过邮件与 Asya Kamsky 和 Ron Anvur 聊过天。我们讨论了我公司在 MongoDB 内部直接执行高级分析的开源工作。
由于我们恰巧同时在帕洛阿尔托,Asya 邀请我过去,一边吃着外卖披萨喝着办公室的汽水,一边聊聊。
刚聊了几分钟,我就能感觉到 Asya 非常聪明、技术过硬且注重细节——这些正是你希望在像 MongoDB 这样高度技术化产品的产品经理身上看到的特质。
我向 Asya 解释了我们公司正在做的事情,并帮助她在她的电脑上运行我们的开源软件,让她可以亲自试试。我们聊着聊着,自然而然地谈到了 MongoDB 的 BI 连接器市场,上面有很多产品(如 Simba、DataDirect、CData 等等)。
我们似乎都持有相同的观点:BI 软件需要具备理解更复杂数据的能力。另一种选择是简化数据以适应老旧 BI 软件的限制,但这意味着会丢失大量信息,从而失去解决 NoSQL 分析中关键问题的能力。
Asya 认为,MongoDB 的 BI 连接器应该能够暴露原生的 MongoDB 数据结构,比如数组,而不需要进行任何展平或转换。这种特性,我称之为同构数据模型,是通用 NoSQL 分析系统的关键需求之一,我在多篇文章中对此进行了深入讨论。
让我感到非常鼓舞的是,Asya 独立得出了相同的结论,这让我相信 MongoDB 已经充分理解了这个问题。我当时认为,MongoDB 的分析未来一片光明。
然而,不幸的是,我错得离谱。
MongoDB:为巨大的创意错误而生
在得知 MongoDB 走在正确的道路上之后,我对 BI 连接器放松了警惕,在接下来的几个月里没怎么关注它,虽然期间我和 Asya 以及 Ron 交换了几封电子邮件。
然而,到了九月份,我发现 MongoDB 的产品团队陷入了沉默。经过几周没有回复的电子邮件,我变得不安,开始自己四处查找线索。
我发现 Asya 分叉了一个名为 Multicorn 的项目,这个项目允许 Python 开发者为 PostgreSQL 编写 Foreign Data Wrappers(外部数据封装器)。
糟糕,我心想,MongoDB 又要故技重施了。
进一步挖掘后,我发现了所谓的“圣杯”:一个名为 yam_fdw (Yet Another MongoDB Foreign Data Wrapper)的新项目,这是一个基于 Multicorn 用 Python 编写的全新 FDW。
根据提交日志(用于追踪代码库的更改),该项目是在我与 Asya Kamsky 于七月份会面之后才开发的。换句话说,这已经是原型之后的开发工作了!
最后一根稻草让我确信 MongoDB 计划将 PostgreSQL 数据库作为其“BI 连接器”发布,是当有人转发给我一个 YouTube 视频,视频中 Asya 演示了该连接器。
视频中的措辞非常谨慎,省去了任何可能带来麻烦的信息,但视频最后总结道:
BI 连接器接收连接,并且可以使用与 Postgres 数据库相同的网络协议,因此如果你的报表工具可以通过 ODBC 连接,我们将提供一个 ODBC 驱动程序,您可以使用该驱动程序从您的工具连接到 BI 连接器。
此时,我毫无疑问地确认:即将随 MongoDB 3.2 一起发布的 BI 连接器实际上是伪装成 PostgreSQL 数据库的产物!
很可能,将 MongoDB 数据吸入 PostgreSQL 的实际逻辑是我之前发现的基于 Python 的 Multicorn 封装器的强化版。
到这个时候,MongoDB 的任何人都没有回复我的邮件,这对任何理智的人来说,应该已经足够让他们放弃了。
然而,我决定再尝试一次,机会是在 12 月 2 日的 MongoDB Days 会议上,那时距离 3.2 发布只有一周时间。
Eliot Horowitz 将发表主题演讲,Asya Kamsky 将会发言,Ron Avnur 可能也会出席。甚至 Dev 自己也可能会来。
那将是我说服 MongoDB 放弃 BI 连接器把戏的最佳机会。
MongoDB Days 2015,硅谷
感谢 MongoDB 出色的市场团队,再加上我在西雅图 MongoDB 巡回演讲中类似演讲的成功经验,我在 MongoDB Days 会议上获得了 45 分钟的演讲时间。
我在会议上的官方任务是做一场关于 MongoDB 驱动的分析的演讲,并让用户了解我们公司开发的开源软件。
但我的个人议程却截然不同:在 3.2 版本即将发布前,说服 MongoDB 放弃 BI 连接器。如果这个宏大的目标(很可能是妄想)无法实现,我至少想确认自己对该连接器的怀疑。
在会议当天,我特意向 MongoDB 的老朋友和新面孔打招呼。尽管我可能不同意某些产品决策,但公司里还是有很多出色的人,他们只是努力在做好自己的工作。
几天前我刚刚生病,但大量的咖啡让我(大部分时间)保持清醒。随着时间的推移,我在圣何塞会议中心长长的走廊里反复练习了几次演讲。
下午时分,我已经准备就绪,并在满员的会场上做了我的演讲。让我兴奋的是,有这么多人对MongoDB 上的视觉分析这一深奥的主题感兴趣(显然这个领域正在发展壮大)。
在与一些与会者握手并交换名片后,我开始寻找 MongoDB 的管理团队。
我首先遇到了 Eliot Horowitz,就在他即将开始主题演讲前几分钟。我们聊了聊孩子和美食,我还告诉他我公司的一些近况。
主题演讲在下午 5:10 准时开始。Eliot 先谈到了一些 3.0 版本的功能,因为显然很多公司仍停留在旧版本上。随后,他快速介绍了 MongoDB 3.2 的各种新功能。
我当时在想,Eliot 会说些什么关于 BI 连接器的内容呢?他会提到它吗?
结果发现,BI 连接器是主题演讲的一个重要内容,不仅有专门的介绍环节,甚至还进行了一场精彩的演示。
BI Connector
Eliot 大声宣布:“MongoDB 没有原生的分析工具”,由此引出了 BI 连接器。
我觉得这有点儿好笑,因为我曾为 MongoDB 写过一篇题为 Native Analytics for MongoDB with SlamData 的客座文章。(编辑注:MongoDB 已经撤下了这篇博客,但截至山地时间15:30,它仍然在搜索索引中 仍在搜索索引中)。SlamData 也是 MongoDB 的合作伙伴,并赞助了 MongoDB Days 大会。
在介绍 BI 连接器的用途时,Eliot 似乎有些磕磕绊绊(从…可操作的洞察中获取操作?麻烦的分析!)。当他把演示交给 Asya Kamsky 时,似乎松了一口气,后者为活动准备了一个不错的演示。
在演示过程中,我发现 Asya 表现得比平时紧张。她斟词酌句,省略了关于连接器是什么的所有细节,只提到了它如何工作的无罪部分(比如它依赖 DRDL 来定义 MongoDB 的 schema)。大部分演示内容并没有集中在 BI 连接器上,而是更多地展示了 Tableau(当然,Tableau 的演示效果确实很好!)。
我所有的反馈都没能减缓 BI 连接器的进展。
全力以赴
主题演讲结束后,大批与会者前往隔壁房间参加鸡尾酒会。与会者大多在与其他与会者交谈,而 MongoDB 的员工则倾向于聚在一起。
我看到 Ron Avnur 与服务器工程副总裁 Dan Pasette 聊天,距离他们几英尺远的地方,正为与会者提供 Lagunitas IPA 的啤酒。
现在是行动的时候了。3.2 版本即将发布,几天之内就会面世。MongoDB 的人都不再回复邮件。Eliot 刚刚告诉全世界 MongoDB 没有原生的分析工具,并将 BI 连接器定位为 NoSQL 分析的革命性工具。
没有什么可失去的了,我走到 Ron 面前,加入了他们的对话,然后开始了一段大约两分钟的激烈独白,猛烈抨击 BI 连接器。
我告诉他,我对 MongoDB 的期望不仅仅是把 PostgreSQL 数据库伪装成 MongoDB 分析的神奇解决方案。我告诉他,MongoDB 应该表现出诚信和领导力,推出一个支持 MongoDB 丰富数据结构的解决方案,将所有计算都推送到数据库中,并且不依赖于竞争对手的数据库。
Ron 被震住了。他开始用模糊的语言为 BI 连接器的“下推”(pushdown)辩护,我意识到这是确认我怀疑的机会。
“Postgres 外部数据封装器几乎不支持下推”,我不动声色地说道。“这在你用于 BI 连接器的 Multicorn 封装器中更是如此,这个封装器基于较旧的 Postgres 版本,甚至不支持 Postgres FDW 的完整下推功能。”
Ron 承认失败。“这是事实”,他说。
我逼他为这个决定辩护。但他无言以对。我告诉他,在 MongoDB 发布“BI 连接器”之前,立即拉下停止绳。Ron 对这个可能性不以为然,我告诉他,这件事会彻底让他难堪。“你可能是对的,”他说,“但我现在有更大的事情要担心,”可能指的是即将发布的 3.2 版本。
我们三个人一起喝了杯啤酒。我指着 Dan 说,“这家伙的团队开发了一个真正能够进行分析的数据库。你们为什么不在 BI 连接器中使用它?”但这没有用,Ron 不为所动。
我们分道扬镳,同意彼此保留不同意见。
我从房间另一头看到了 Dev Ittycheria,走过去和他聊了几句。我称赞了市场部的工作,然后开始批评产品。我告诉 Dev,“在我看来,产品团队正在犯一些错误。”他想了解更多,所以我告诉了他我的看法,我已经重复了很多次,以至于我都能倒背如流了。他让我发邮件跟进,当然我发了,但再也没收到回复。
与 Dev 交谈后,我终于意识到我无法改变 MongoDB 3.2 的发布进程。它将与 BI 连接器一起发布,而我对此无能为力。
我很失望,但同时也感觉到一阵巨大的解脱。我已经和我能接触到的每个人都谈过了。我已经全力以赴。我已经竭尽全力。
当我离开鸡尾酒会,回到酒店时,不禁猜测公司为什么会做出我如此强烈反对的决定。
MongoDB:孤家寡人,孤岛一座
经过深思熟虑,我现在认为 MongoDB 做出糟糕产品决策的原因在于无法专注于核心数据库。而这种无法专注的原因,则在于其未能培育出一个 NoSQL 生态系统。
关系型数据库之所以能占据主导地位,部分原因在于围绕这些数据库发展的庞大生态系统。
这个生态系统催生了备份、复制、分析、报告、安全、治理以及许多其他类别的应用程序。它们相互依赖,并促进彼此的成功,形成了网络效应和高转换成本,这些都对当今的 NoSQL 厂商构成了挑战。
与之形成鲜明对比的是,MongoDB 周围几乎没有生态系统,而且不仅我一个人注意到了这一点 注意到这一点。
为什么 MongoDB 没有生态系统?
我带有讽刺意味的回答是,如果你是一个为 MongoDB 提供原生分析的合作伙伴,MongoDB 的 CTO 会站在舞台上说,没有工具可以为 MongoDB 提供原生分析。
然而,更客观地说,我认为上述现象只是一个表象。真正的问题是 MongoDB 的合作伙伴计划完全失效了。
MongoDB 的合作伙伴团队直接向首席营收官(Carlos Delatorre)汇报工作,这意味着合作伙伴团队的主要任务是从合作伙伴那里获取收入。这种设置本质上会让合作伙伴活动偏向那些没有兴趣推动 NoSQL 生态系统发展的大型公司(事实上,其中许多公司还在生产与 MongoDB 竞争的关系型解决方案)。
这与 SlamData、Datos IO 等小型、以 NoSQL 为中心的公司形成鲜明对比。这些公司之所以能够成功,正是因为 NoSQL 的成功,它们提供了关系型数据库世界中标准的功能,而 NoSQL 数据库需要这些功能才能在企业级环境中蓬勃发展。
作为合作伙伴已经超过一年,我可以告诉你,几乎没有人知道 SlamData 的存在,尽管 SlamData 是企业选择 MongoDB 而不是其他 NoSQL 数据库(例如 MarkLogic)的一个强大动因,也是那些考虑从关系型技术(例如 Oracle)转向 MongoDB 的公司转型的推动者。
尽管合作伙伴们在努力,但 MongoDB 似乎对由 NoSQL 为中心的合作伙伴所带来的联合收入和销售机会完全不感兴趣。没有转售协议、没有收入分享、没有销售简介、没有联合营销,只有一个 Logo。
这意味着在组织上,MongoDB 忽视了那些可能对他们最有帮助的 NoSQL 为中心的合作伙伴。同时,他们的最大客户和潜在客户不断要求提供在关系型世界中常见的基础设施,比如备份、复制、监控、分析、数据可视化、报告、数据治理、查询分析等。
这些来自大公司源源不断的需求,结合未能培养出生态系统的无力,形成了一种有毒的组合。它导致 MongoDB 产品团队试图通过构建所有可能的产品来创造自己的生态系统!
备份?有。复制?有。监控?有。BI 连接性?有。数据发现?有。可视化分析?有。
但一个有限资源的 NoSQL 数据库供应商不可能围绕自己建立一个生态系统,去与围绕关系型技术的庞大生态系统竞争(代价太高了!)。因此,这导致了像 MongoDB Compass 这样的分散注意力的项目,以及像 BI 连接器这样的“伪”技术。
替代方案是什么?在我看来,非常简单。
首先,MongoDB 应该培育一个充满活力的、由风险投资支持的 NoSQL 为中心的合作伙伴生态系统(而不是拥有雄厚资金的关系型合作伙伴!)。这些合作伙伴应该在各自的领域内具有深厚的专业知识,并且它们都应该在 MongoDB 成功的情况下成功。
MongoDB 的销售代表和客户经理应该掌握由合作伙伴提供的信息,这些信息可以帮助他们克服异议并减少客户流失,而 MongoDB 应该将其构建为一个健康的收入来源。
其次,在通过 NoSQL 为中心的合作伙伴满足了客户对相关基础设施的需求之后,MongoDB 应该将产品和销售重点放在核心数据库上,这才是数据库供应商应当赚钱的方式!
MongoDB 应该开发对企业有重要价值的功能(例如 ACID 事务、NVRAM 存储引擎、列式存储引擎、跨数据中心复制等),并仔细划定社区版和企业版之间的界限。所有这一切都应该以一种方式进行,使开发者在不同版本中拥有相同的能力。
目标应该是让 MongoDB 从数据库中获得足够的收入,以至于产品团队不会再受到诱惑去发明一个劣质的生态系统。
你可以自己判断,但我认为哪个是更有可能成功的战略已经非常明显了。
再见了,MongoDB!
显然,我无法支持这样的产品决策——推出一个竞争性的关系型数据库,作为在像 MongoDB 这样后关系型数据库上进行分析的最终解决方案。
在我看来,这个决定对社区不利,对客户不利,对新兴的 NoSQL 分析领域也不利。
此外,如果这种做法没有 完全透明,它对诚信也是有害的,而诚信是 所有公司 的基石(尤其是开源公司)。
所以,通过这篇文章,我正式放弃。
不再给 MongoDB 发狂热的邮件。不再在 MongoDB 的鸡尾酒会上缠着管理层。不再与一个连邮件都不回的公司私下分享我的意见。
这条路我走过了,做过了,但没有效果。
显然,我现在要揭发这个事实。当你读到这篇文章时,全世界都将知道 MongoDB 3.2 BI 连接器实际上就是 PostgreSQL 数据库,附带一些拼接数据的工具,把一些数据丢弃,然后把剩下的部分吸入 PostgreSQL。
这对那些正在评估 MongoDB 的公司意味着什么?
这取决于你们自己,但就我个人而言,如果你在寻找一个 NoSQL 数据库,同时需要传统的 BI 连接性,并且也在考虑 PostgreSQL,那么你可能应该直接选择 PostgreSQL。
毕竟,MongoDB 对 MongoDB 上的分析问题的 自己答案 就是将数据从 MongoDB 中导出,扁平化,然后倒入 PostgreSQL。如果你的数据最终会变成在 PostgreSQL 中的扁平化关系数据,那为什么不直接从那里开始呢?一石二鸟!
至少你可以指望 PostgreSQL 社区在 NoSQL 领域的创新,他们已经这么做很多年了。社区绝不会将 MongoDB 数据库打包成一个假的“PostgreSQL NoSQL”产品,然后称其为 NoSQL 数据库技术的革命。
而遗憾的是,这恰恰就是 MongoDB 反其道而行之的做法。
这张“Shame”的照片由 Grey World 拍摄,版权归 Grey World 所有,并根据 CC By 2.0 许可发布。
MongoDB 3.2: Now Powered by PostgreSQL
John De Goes —— Challenging the status quo at Ziverge
发布日期: 2015年12月8日
Opinions expressed are solely my own, and do not express the views or opinions of my employer.
When I finally pieced together all the clues, I was shocked. If I was right, MongoDBwas about to make what I would call the biggest mistake ever made in the history of database companies.
I work on an open source analytics tool that connects to NoSQL databases like MongoDB, so I spend my days rooting for these next-generation database vendors to succeed.
In fact, I just presented to a packed room at MongoDB Days Silicon Valley, making a case for companies to adopt the new database.
So when I uncovered a secret this destructive, I hit the panic button: on November 12th, 2015, I sent an email to Asya Kamsky, Lead Product Manager at MongoDB.
While polite, I made my opinion crystal clear: MongoDB is about to make a giant mistake, and should reconsider while there’s still time.
I would never hear back from Asya — or anyone else about the matter. My earlier success in helping convince MongoDB to reverse course when they tried to monetize the wrong feature would not be repeated.
This is the story of what I discovered, how I pieced together the clues from press releases, YouTube videos, and source code scattered on Github, and how I ultimately failed to convince MongoDB to change course.
The story begins on June 1st 2015, at the annual MongoWorld conference in New York City.
MongoWorld 2015
SlamData, my new analytics startup, was sponsoring MongoWorld 2015, so I got a rare ticket to the VIP party the night before the conference.
Hosted at NASDAQ MarketWatch, in a beautiful space overlooking Times Square, I felt distinctly underdressed in my cargo pants and startup t-shirt. Fancy h’ordeuvres and alcohol flowed freely, and MongoDB’s management team was out in full force.
I shook hands with MongoDB’s new CEO, Dev (“Dave”) Ittycheria, and offered him a few words of encouragement for the road ahead.
Only this year, Fidelity Investments slashed its valuation of MongoDB to 50% of what it was back in 2013 ($1.6B), downgrading the startup from “unicorn” to “donkey”.
It’s been Dev’s job to prove Fidelity and the rest of the naysayers wrong.
Dev inherited the company from Max Schireson (who famously resigned in 2014), and in his tenure, Dev has built out a new management team at MongoDB, with ripples felt across the company.
Though I only spoke with Dev for a few minutes, he seemed bright, friendly, and eager to learn about what my company was doing. He handed me his card and asked me to call him if I ever needed anything.
Next up was Eliot Horowitz, CTO and co-founder of MongoDB. I shook his hand, introduced myself, and delivered a 30 second pitch for my startup.
At the time, I thought my pitch must have been terrible, since Eliot seemed disinterested in everything I was saying. Turns out Eliot hates SQL and views analytics as a nuisance, so it’s not surprising I bored him!
Eliot did catch the word “analytics”, however, and dropped that tomorrow at the conference, MongoDB would have some news about the upcoming 3.2 release that I would find very interesting.
I pleaded for more details, but nope, that was strictly confidential. I’d find out the following day, along with the rest of the world.
I passed along the tip to my co-founder, Jeff Carr, and we shared a brief moment of panic. The big fear for our four-person, self-funded startup was that MongoDB would be announcing their own analytics tool for MongoDB, which could hurt our chances of raising money.
Much to our relief, we’d find out the following day that MongoDB’s big announcement wasn’t an analytics tool. Instead, it was a solution called MongoDB BI Connector, a headline feature of the upcoming 3.2 release.
The MongoDB 3.2 BI Connector
Eliot had the honor of announcing the BI connector. Of all the things he was announcing, Eliot seemed least interested in the connector, so it got barely more than a mention.
But details soon spread like wildfire thanks to an official press release, which contained this succinct summary:
MongoDB today announced a new connector for BI and visualization, which connects MongoDB to industry-standard business intelligence (BI) and data visualization tools. Designed to work with every SQL-compliant data analysis tool on the market, including Tableau, SAP Business Objects, Qlik and IBM Cognos Business Intelligence, the connector is currently in preview release and expected to become generally available in the fourth quarter of 2015.
According to the press release, the BI connector would allow any BI software in the world to interface with the MongoDB database.
News of the connector [caught fire](https://twitter.com/search?f=tweets&vertical=default&q=mongodb bi connector&src=typd) on Twitter, and the media went into a frenzy. The story was picked up by TechCrunch and many others. Every retelling added new embellishments, with Fortune even claiming the BI connector had actually been released at MongoWorld!
Given the nature of the announcement, the media hoopla was probably justified.
When Worlds Collide
MongoDB, like many other NoSQL databases, does not store relational data. It stores rich data structures that relational BI software cannot understand.
Kelly Stirman, VP of Strategy at MongoDB, explained the problem well:
“The thing that defines these apps as modern is rich data structures that don’t fit neatly into rows and columns of traditional databases."
A connector that enabled any BI software in the world to do robust analytics on rich data structures, with no loss of analytic fidelity, would be giant news.
Had MongoDB really done the impossible? Had they developed a connector which satisfies all the requirements of NoSQL analytics, but exposes relational semantics on flat, uniform data, so legacy BI software can handle it?
A couple months earlier, I had chatted with Ron Avnur, VP of Products at MongoDB. Ron indicated that all of MongoDB’s customers wanted analytics, but that they hadn’t decided whether to build something in-house or work with a partner.
This meant that MongoDB had gone from nothing to magic in just a few months.
Pulling Back the Curtain
After the announcement, Jeff and I headed back to our sponsor booth, and Jeff asked me the most obvious question: “How did they go from nothing to a BI connector that works with all possible BI tools in just a couple months?!?”
I thought carefully about the question.
Among other problems that a BI connector would need to solve, it would have to be capable of efficiently executing SQL-like analytics on MongoDB. From my deepbackground in analytics, I knew that efficiently executing general-purpose analytics on modern databases like MongoDB is very challenging.
These databases support very rich data structures and their interfaces are designed for so-called operational use cases (not analytical use cases). The kind of technology that can leverage operational interfaces to run arbitrary analytics on rich data structures takes years to develop. It’s not something you can crank out in two months.
So I gave Jeff my gut response: “They didn’t create a new BI connector. It’s impossible. Something else is going on here!”
I didn’t know what, exactly. But in between shaking hands and handing out cards, I did some digging.
Tableau showed a demo of their software working with the MongoDB BI Connector, which piqued my curiosity. Tableau has set the standard for visual analytics on relational databases, and their forward-thinking big data team has been giving NoSQL some serious thought.
Thanks to their relationship with MongoDB, Tableau issued a press release to coincide with the MongoWorld announcement, which I found on their website.
I pored through this press release hoping to learn some new details. Burried deep inside, I discovered the faintest hint about what was going on:
MongoDB will soon announce beta availability of the connector, with general availability planned around the MongoDB 3.2 release late this year. During MongoDB’s beta, Tableau will be supporting the MongoDB connector on both Windows and Mac via our PostgreSQL driver.
These were the words that gave me my first clue: via our PostgreSQL driver. This implied, at a minimum, that MongoDB’s BI Connector would speak the same “language” (wire protocol) as the PostgreSQL database.
That struck me as more than a little suspicious: was MongoDB actually re-implementing the entirety of the PostgreSQL wire protocol, including support for hundreds of PostgreSQL functions?
While possible, this seemed extremely unlikely.
I turned my gaze to Github, looking for open source projects that MongoDB might have leveraged. The conference Wifi was flaky, so I had to tether to my phone while I looked through dozens of repositories that mentioned both PostgreSQL and MongoDB.
Eventually, I found what I was looking for: mongoose_fdw, an open source repository forked by Asya Kamsky (whom I did not know at the time, but her profile mentioned she worked for MongoDB).
The repository contained a so-called Foreign Data Wrapper (FDW) for the PostgreSQL database. The FDW interface allows developers to plug in other data sources, so that PostgreSQL can pull the data out and execute SQL on the data (NoSQL data must be flattened, null-padded, and otherwise dumbed-down for this to work properly for BI tools).
“I think I know what’s going on”, I told Jeff. “For the prototype, it looks like they might be flattening out the data and using a different database to execute the SQL generated by the BI software.”
“What database?” he shot back.
“PostgreSQL.”
Jeff was speechless. He didn’t say a word. But I could tell exactly what he was thinking, because I was thinking it too.
Shit. This is bad news for MongoDB. Really bad.
PostgreSQL: The MongoDB Killer
PostgreSQL is a popular open source relational database. So popular, in fact, it’s currently neck-and-neck with MongoDB.
The database is fierce competition for MongoDB, primarily because it has acquired some of the features of MongoDB, including the ability to store, validate, manipulate, and index JSON documents. Third-party software even gives it the ability to scale horizontally (or should I say, humongously).
Every month or so, someone writes an article that recommends PostgreSQL over MongoDB. Often, the article goes viral and skyrockets to the top of hacker websites. A few of these articles are shown below:
- Goodbye MongoDB. Hello PostgreSQL
- Postgres Outperforms MongoDB and Ushers in New Developer Reality
- MongoDB is dead. Long live Postgresql :)
- Why You Should Never Use MongoDB
- SQL vs NoSQL KO. Postgres vs Mongo
- Why I Migrated Away from MongoDB
- Why you should never, ever, ever use MongoDB
- Is Postgres NoSQL Better than MongoDB?
- Bye Bye MongoDB. Guten Tag PostgreSQL
The largest company commercializing PostgreSQL is EnterpriseDB (though there are plenty of others, some older or just as active), which maintains a large repository of content on the official website arguing that PostgreSQL is a better NoSQL database than MongoDB.
Whatever your opinion on that point, one thing is clear: MongoDB and PostgreSQL are locked in a vicious, bloody battle for mind share among developers.
From Prototype to Production
As any engineer worth her salt will tell you, prototypes aren’t for production.
Even if MongoDB was using PostgreSQL as a prototype BI connector, maybe some brilliant MongoDB engineers were locked in a room somewhere, working on a standalone production version.
Indeed, the way Tableau worded their press release even implied the dependency on the PostgreSQL driver might be temporary:
During MongoDB’s beta, Tableau will be supporting the MongoDB connector on both Windows and Mac via our PostgreSQL driver.
Perhaps, I thought, the 3.2 release of MongoDB would ship with the real deal: a BI connector that exposes the rich data structures that MongoDB supports (instead of flattening, null-padding, and throwing away data), executes all queries 100% in-database, and has no dependencies on competing databases.
In July, more than a month after MongoWorld, I dropped by MongoDB’s offices in Palo Alto during a business trip. And I was very encouraged by what I learned.
A Trip to MongoDB
By Palo Alto’s standards, MongoDB’s office is quite large.
I had seen the company’s sign during previous trips to the Valley, but this was the first time I had a chance to go inside.
The week before, I was chatting with Asya Kamsky and Ron Anvur by email. We were discussing my company’s open source work in executing advanced analytics on rich data structures directly inside MongoDB.
Since we happened to be in Palo Alto at the same time, Asya invited me over to chat over catered pizza and office soda.
Within the first few minutes, I could tell that Asya was smart, technical, and detail-oriented — exactly the traits you’d hope for in a product manager for a highly technical product like MongoDB.
I explained to Asya what my company was doing, and helped her get our open source software up and running on her machine so she could play with it. At some point, we started chatting about BI connectors for MongoDB, of which there were several in the market (Simba, DataDirect, CData, and others).
We both seemed to share the same view: that BI software needs to gain the ability to understand more complex data. The alternative, which involves dumbing down the data to fit the limitations of older BI software, means throwing away so much information, you lose the ability to solve key problems in NoSQL analytics.
Asya thought a BI connector for MongoDB should expose the native MongoDB data structures, such as arrays, without any flattening or transformations. This characteristic, which I have termed isomorphic data model, is one of the key requirements for a general-purpose NoSQL analytics, a topic I’ve written about extensively.
I was very encouraged that Asya had independently come to the same conclusion, and felt confident that MongoDB understood the problem. I thought the future of analytics for MongoDB looked very bright.
Unfortunately, I could not have been more wrong.
MongoDB: For Giant IdeasMistakes
Delighted that MongoDB was on the right track, I paid little attention to the BI connector for the next couple of months, though I did exchange a few emails with Asya and Ron.
Heading into September, however, I encountered utter silence from the product team at MongoDB. After a few weeks of unreturned emails, I grew restless, and started poking around on my own.
I discovered that Asya had forked a project called Multicorn, which allows Python developers to write Foreign Data Wrappers for PostgreSQL.
Uh oh, I thought, MongoDB is back to its old tricks.
More digging turned up the holy grail: a new project called yam_fdw (Yet Another MongoDB Foreign Data Wrapper), a brand new FDW written in Python using Multicorn.
According to the commit log (which tracks changes to the repository), the project had been built recently, after my July meeting with Asya Kamsky. In other words, this was post-prototype development work!
The final nail in the coffin, which convinced me that MongoDB was planning on shipping the PostgreSQL database as their “BI connector”, happened when someone forwarded me a video on YouTube, in which Asya demoed the connector.
Worded very cautiously, and omitting any incriminating information, the video nonetheless ended with this summary:
The BI Connector receives connections and can speak the same wire protocol that the Postgres database****does, so if your reporting tool can connect via ODBC, we will have an ODBC driver that you will be able to use from your tool to the BI Connector.
At that point, I had zero doubt: the production version of the BI connector, to be shipped with MongoDB 3.2, was, in fact, the PostgreSQL database in disguise!
Most likely, the actual logic that sucked data out of MongoDB into PostgreSQL was a souped-up version of the Python-based Multicorn wrapper I had discovered earlier.
At this point, no one at MongoDB was returning emails, which to any sane person, would have been enough to call it quits.
Instead, I decided to give it one more try, at the MongoDB Days conference on December 2, just one week before the release of 3.2.
Eliot Horowitz was delivering a keynote, Asya Kamsky would be speaking, and Ron Avnur would probably attend. Possibly, even Dev himself might drop by.
That’s when I’d have my best chance of convincing MongoDB to ditch the BI connector shenanigans.
MongoDB Days 2015, Silicon Valley
Thanks to the wonderful marketing team at MongoDB, and based on the success of a similar talk I gave in Seattle at a MongoDB road show, I had a 45 minute presentation at the MongoDB Days conference.
My official purpose at the conference was to deliver my talk on MongoDB-powered analytics, and make users aware of the open source software that my company develops.
But my personal agenda was quite different: convincing MongoDB to can the BI connector before the impending 3.2 release. Failing that lofty and most likely delusional goal, I wanted to confirm my suspicions about the connector.
On the day of the conference, I went out of my way to say hello to old and new faces at MongoDB. Regardless of how much I may disagree with certain product decisions, there are many amazing people at the company just trying to do their jobs.
I had gotten sick a few days earlier, but copious amounts of coffee kept me (mostly) awake. As the day progressed, I rehearsed my talk a few times, pacing the long corridors of the San Jose Convention Center.
When the afternoon rolled around, I was ready, and gave my talk to a packed room. I was excited about how many people were interested in the esoteric topic of visual analytics on MongoDB (clearly the space was growing).
After shaking hands and exchanging cards with some of the attendees, I went on the hunt for the MongoDB management team.
I first ran into Eliot Horowitz, moments before his keynote. We chatted kids and food, and I told him how things were going at my company.
The keynote started sharply at 5:10. Eliot talked about some of the features in 3.0, since a lot of companies are apparently stuck on older versions. He then proceeded to give a whirlwind tour of the features of MongoDB 3.2.
I wondered what Eliot would say about the BI connector. Would he even mention it?
Turns out, the BI connector was a leading feature of the keynote, having its own dedicated segment and even a whiz-bang demo.
The BI Connector
Eliot introduced the BI connector by loudly making the proclamation, “MongoDB has no native analytics tools.”
I found that somewhat amusing, since I wrote a guest post for MongoDB titled Native Analytics for MongoDB with SlamData (Edit: MongoDB has taken down the blog post, but as of 15:30 MDT, it’s still in the search index). SlamData is also a MongoDB partner and sponsored the MongoDB Days conference.
Eliot seemed to stumble a bit when describing the purpose of the BI connector (getting actions from… actionable insights? Pesky analytics!). He looked relieved when he handed the presentation over to Asya Kamsky, who had prepared a nice demo for the event.
During the presentation, Asya seemed uncharacteristically nervous to me. She chose every word carefully, and left out all details about what the connector was, only covering the non-incriminating parts of how it worked (such as its reliance on DRDL to define MongoDB schemas). Most of the presentation focused not on the BI connector, but on Tableau (which, of course, demos very well!).
All my feedback hadn’t even slowed the BI connector down.
Pulling Out All the Stops
After the keynote, the swarm of conference attendees proceeded to the cocktail reception in the adjacent room. Attendees spent most of their time talking to other attendees, while MongoDB employees tended to congregate in bunches.
I saw Ron Avnur chatting with Dan Pasette, VP of Server Engineering, a few feet from the keg of Lagunitas IPA they were serving attendees.
Now was the time to act.
The 3.2 release was coming out in mere days. No one at MongoDB was returning emails. Eliot had just told the world there were no native analytics tools for MongoDB, and had positioned the BI connector as a revolution for NoSQL analytics.
With nothing to lose, I walked up to Ron, inserted myself into the conversation, and then began ranting against the BI connector in what was probably a two-minute, highly-animated monologue.
I told him I expected more from MongoDB than disguising the PostgreSQL database as the magical solution to MongoDB analytics. I told him that MongoDB should have demonstrated integrity and leadership, and shipped a solution that supports the rich data structures that MongoDB supports, pushes all computation into the database, and doesn’t have any dependencies on a competing database.
Ron was stunned. He began to defend the BI connector’s “pushdown” in vague terms, and I realized this was my chance to confirm my suspicions.
“Postgres foreign data wrappers support barely any pushdown,” I stated matter-of-factly. “This is all the more true in the Multicorn wrapper you’re using for the BI connector, which is based on an older Postgres and doesn’t even support the full pushdown capabilities of the Postgres FDW.”
Ron admitted defeat. “That’s true,” he said.
I pushed him to defend the decision. But he had no answer. I told him to pull the stop cord right now, before MongoDB released the “BI connector”. When Ron shrugged off that possibility, I told him the whole thing was going to blow up in his face. “You might be right,” he said, “But I have bigger things to worry about right now,” possibly referring to the upcoming 3.2 release.
We had a beer together, the three of us. I pointed to Dan, “This guy’s team has built a database that can actually do analytics. Why aren’t you using it in the BI connector?” But it was no use. Ron wasn’t budging.
We parted ways, agreeing to disagree.
I spotted Dev Ittycheria from across the room, and walked over to him. I complimented the work that the marketing department was doing, before moving on to critique product. I told Dev, “In my opinion, product is making some mistakes.” He wanted to know more, so I gave him my spiel, which I had repeated often enough to know by heart. He told me to followup by email, and of course I did, but I never heard back.
After my conversation with Dev, it finally sunk in that I would not be able to change the course of MongoDB 3.2. It would ship with the BI connector, and there wasn’t a single thing that I could do about it.
I was disappointed, but at the same time, I felt a huge wave of relief. I had talked to everyone I could. I had pulled out all the stops. I had given it my all.
As I left the cocktail reception, and headed back to my hotel, I couldn’t help but speculate on why the company was making decisions that I so strongly opposed.
MongoDB: An Island of One
After much reflection, I now think that MongoDB’s poor product decisions are caused by an inability to focus on the core database. This inability to focus is caused by an inability to cultivate a NoSQL ecosystem.
Relational databases rose to dominance, in part, because of the astounding ecosystem that grew around these databases.
This ecosystem gave birth to backup, replication, analytics, reporting, security, governance, and numerous other category-defining applications. Each depended on and contributed to the success of the others, creating network benefits and high switching costs that are proving troublesome for modern-day NoSQL vendors.
In contrast, there’s virtually no ecosystem around MongoDB, and I’m not the only one to notice this fact.
Why isn’t there an ecosystem around MongoDB?
My snarky answer is that because, if you are a MongoDB partner that provides native analytics for MongoDB, the CTO will get up on stage and say there are no tools that provide native analytics for MongoDB.
More objectively, however, I think the above is just a symptom. The actual problem is that the MongoDB partner program is totally broken.
The partner team at MongoDB reports directly to the Chief Revenue Officer (Carlos Delatorre), which implies the primary job of the partner team is to extract revenue from partners. This inherently skews partner activities towards large companies that have no vested interest in the success of the NoSQL ecosystem (indeed, many of them produce competing relational solutions).
Contrast that with small, NoSQL-centric companies like SlamData, Datos IO, and others. These companies succeed precisely in the case that NoSQL succeeds, and they provide functionality that’s standard in the relational world, which NoSQL databases need to thrive in the Enterprise.
After being a partner for more than a year, I can tell you that almost no one in MongoDB knew about the existence of SlamData, despite the fact that SlamData acted as a powerful incentive for companies to choose MongoDB over other NoSQL databases (e.g. MarkLogic), and an enabler for companies considering the switch from relational technology (e.g. Oracle).
Despite the fact that partners try, MongoDB appears completely unconcerned about the joint revenue and sales opportunities presented by NoSQL-centric partners. No reseller agreements. No revenue sharing. No sales one-pagers. No cross-marketing. Nothing but a logo.
This means that organizationally, MongoDB ignores the NoSQL-centric partners who could most benefit them. Meanwhile, their largest customers and prospects keep demanding infrastructure common to the relational world, such as backup, replication, monitoring, analytics, data visualization, reporting, data governance, query analysis, and much more.
This incessant demand from larger companies, combined with the inability to cultivate an ecosystem, forms a toxic combination. It leads MongoDB product to try to create its own ecosystem by building all possible products!
Backup? Check. Replication? Check. Monitoring? Check. BI connectivity? Check. Data discovery? Check. Visual analytics? Check.
But a single NoSQL database vendor with finite resources cannot possibly build an ecosystem around itself to compete with the massive ecosystem around relational technology (it’s far too expensive!). So this leads to distractions, like MongoDB Compass, and “sham” technology, like the BI connector.
What’s the alternative? In my humble opinion, it’s quite simple.
First, MongoDB should nurture a vibrant, venture-funded ecosystem of NoSQL-centric partners (not relational partners with deep pockets!). These partners should have deep domain expertise in their respective spaces, and all of them should succeed precisely in the case that MongoDB succeeds.
MongoDB sales reps and account managers should be empowered with partner-provided information that helps them overcome objections and reduce churn, and MongoDB should build this into a healthy revenue stream.
Second, with customer demand for related infrastructure satisfied by NoSQL-centric partners, MongoDB should focus both product and sales on the core database, which is how a database vendor should make money!
MongoDB should develop features that have significant value to Enterprise (such as ACID transactions, NVRAM storage engines, columnar storage engines, cross data center replication, etc.), and thoughtfully draw the line between Community and Enterprise. All in a way that gives developers the same capabilities across editions.
The goal should be for MongoDB to drive enough revenue off the database that product won’t be tempted to invent an inferior ecosystem.
You be the judge, but I think it’s pretty clear which is the winning strategy.
Bye-Bye, MongoDB
Clearly, I cannot get behind product decisions like shipping a competing relational database as the definitive answer to analytics on a post-relational database like MongoDB.
In my opinion, this decision is bad for the community, it’s bad for customers, and it’s bad for the emerging space of NoSQL analytics.
In addition, to the extent it’s not done with full transparency, it’s also bad for integrity, which is a pillar on which all companies should be founded (especially open source companies).
So with this post, I’m officially giving up.
No more frantic emails to MongoDB. No more monopolizing management at MongoDB cocktail parties. No more sharing my opinions in private with a company that doesn’t even return emails.
Been there, done that, didn’t work.
I’m also, obviously, blowing the whistle. By the time you’re reading this, the whole world will know that the MongoDB 3.2 BI Connector is the PostgreSQL database, with some glue to flatten data, throw away bits and pieces, and suck out whatever’s left into PostgreSQL.
What does all this mean for companies evaluating MongoDB?
That’s your call, but personally, I’d say if you’re in the market for a NoSQL database, you need legacy BI connectivity, and you’re also considering PostgreSQL, you should probably just pick PostgreSQL.
After all, MongoDB’s own answer to the problem of analytics on MongoDB is to pump the data out of MongoDB, flatten it out, and dump it into PostgreSQL. If your data is going to end up as flat relational data in PostgreSQL, why not start out there, too? Kill two birds with one stone!
At least you can count on the PostgreSQL community to innovate around NoSQL, which they’ve been doing for years. There’s zero chance the community would package up the MongoDB database into a sham “PostgreSQL NoSQL” product, and call it a revolution in NoSQL database technology.
Which is, sadly, exactly what MongoDB has done in reverse.
The Shame photo taken by Grey World, copyright Grey World, and licensed under CC By 2.0.
瑞士强制政府软件开源
瑞士政府通过开源立法走在时代前沿,给 IT 后发国家如何保证软件自主可控打了个样。真正的自主可控根源在于“开源社区”,而不是某些“民族主义”式的“国产软件”。 老冯评论
作者:Steven Vaughan-Nichols,原文地址
美国政府仍然对使用开源软件不情不愿,而欧洲国家则更为勇敢。
几个欧洲国家正在押注开源软件,至于美国嘛,就没那么多了。来自欧洲最新的消息是,瑞士在其《联邦使用电子手段履行政府职责法》(EMBAG)中迈出了重大一步。这项开创性的立法,强制要求在公共部门(政府)使用开源软件(OSS)。
这项新法律规定,除非涉及第三方版权和安全保密问题,所有公共机构必须公开其开发或为其开发的软件的源代码。这种“公共资金,公共代码” 的方法旨在提升政府运作的透明度、安全性与效率。
参考阅读:德国州政府弃用微软,转投 Linux 和 LibreOffice
做出这一决定并不容易。早在2011年,瑞士联邦最高法院就将其法院应用程序 Open Justitia 使用开源许可证发布。而这让专有法律软件公司 Weblaw 感到不满。十多年来,围绕这一问题的政治和法律争斗不断。最终,EMBAG 于 2023 年通过。这项法律不仅允许瑞士政府或其承包商发布开源软件,还要求代码必须以开源许可证发布,“除非第三方版权或安全相关原因排除或限制了这一点。”
伯尔尼应用科学大学公共部门转型研究所的负责人 Matthias Stürmer 教授领导了这场立法斗争。他将这项法律称为“政府、IT行业和社会的巨大机遇”。Stürmer 认为,所有人都将从这项法规中受益,因为它减少了公共部门的供应商锁定,并允许企业扩展其数字业务解决方案,并有潜力降低IT成本并提高纳税人服务质量。
除了强制使用开源软件(OSS)外,EMBAG 还要求政府将非个人和非敏感安全的数据也作为开放政府数据(OGD)发布。这种双重的 “默认开放” 策略标志着一场范式转移 —— 通往更大的开放性和软件及数据实际再利用的重大范式转变。
EMBAG 的实施预计将成为其他国家考虑类似措施的典范。它旨在促进数字主权,鼓励公共部门内的创新和合作。瑞士联邦统计局(BFS)正在主导这项法律的实施,但OSS发布的组织和财务方面仍需明确。
参考阅读:为什么更多的人不使用桌面Linux?我有一个你可能不喜欢的理论
其他欧洲国家也长期支持开源软件。例如,2023年,,法国总统马克龙表示,“我们热爱开源” 。 而法国国家宪兵队(类似美国的FBI)在其PC上使用Linux。欧盟(EU)通过其自由和开源软件审计(FOSSA)项目,长期致力于保障开源软件的安全。
不过,欧盟内部也并非一帆风顺。有些人担心欧洲委员会会削减 NGI Zero Commons Fund的资金,这一资金是OSS项目的重要来源。
在美国,虽然也有一些对开源的支持,但远不及欧洲。例如,联邦源代码政策要求联邦机构至少发布20%的新定制开发代码作为开源软件,但并没有强制要求使用开源软件。总务管理局(GSA)也有一项开源政策,要求GSA组织考虑并发布其开源代码,提倡新定制代码开发的“开放优先”方法。
总的来说,尽管瑞士的立法举措将其置于全球开源运动的前沿,但在欧洲和美国仍需做更多工作以推动开源软件的普及和应用。
参考阅读
MySQL 已死,PostgreSQL 当立
本月,MySQL 9.0 终于发布了(@2024-07),距离上一次大版本更新 8.0 (@2016-09) 已经过去八年了。然而这个空洞无物的所谓“创新版本”却犹如一个恶劣的玩笑,宣告着 MySQL 正在死去。
PostgreSQL 正在高歌猛进,而 MySQL 却日薄西山,作为 MySQL 生态主要扛旗者的 Percona 也不得不悲痛地承认这一现实,连发三篇《MySQL将何去何从》,《Oracle最终还是杀死了MySQL》,《Oracle还能挽救MySQL吗》,公开表达了对 MySQL 的失望与沮丧;
Percona 的 CEO Peter Zaitsev 也表示:
有了 PostgreSQL,谁还需要 MySQL 呢? —— 但如果 MySQL 死了,PostgreSQL 就真的垄断数据库世界了,所以 MySQL 至少还可以作为 PostgreSQL 的磨刀石,让 PG 进入全盛状态。
有的数据库正在吞噬数据库世界,而有的数据库正在黯然地凋零死去。
MySQL is dead,Long live PostgreSQL!
空洞无物的创新版本
MySQL 官网发布的 “What’s New in MySQL 9.0” 介绍了 9.0 版本引入的几个新特性,而 MySQL 9.0 新功能概览 一文对此做了扼要的总结:
然后呢?就这些吗?这就没了!?
这确实是让人惊诧不已,因为 PostgreSQL 每年的大版本发布都有无数的新功能特性,例如计划今秋发布的 PostgreSQL 17 还只是 beta1,就已然有着蔚为壮观的新增特性列表:
而最近几年的 PostgreSQL 新增特性甚至足够专门编成一本书了。比如《快速掌握PostgreSQL版本新特性》便收录了 PostgreSQL 最近七年的重要新特性 —— 将目录塞的满满当当:
回头再来看看 MySQL 9 更新的六个特性,后四个都属于无关痛痒,一笔带过的小修补,拿出来讲都嫌丢人。而前两个 向量数据类型 和 JS存储过程 才算是重磅亮点。
BUT ——
MySQL 9.0 的向量数据类型只是 BLOB
类型换皮 —— 只加了个数组长度函数,这种程度的功能,28年前 PostgreSQL 诞生的时候就支持了。
而 MySQL Javascript 存储过程支持,竟然还是一个 企业版独占特性,开源版不提供 —— 而同样的功能,13年前 的 PostgreSQL 9.1 就已经有了。
时隔八年的 “创新大版本” 更新就带来了俩 “老特性”,其中一个还是企业版特供。“创新”这俩字,在这里显得如此辣眼与讽刺。
糊弄了事的向量类型
这两年 AI 爆火,也带动了向量数据库赛道。当下几乎所有主流 DBMS 都已经提供向量数据类型支持 —— MySQL 除外。
用户可能原本期待着在 9.0 创新版,向量支持能弥补一些缺憾,结果发布后等到的只有震撼 —— 竟然还可以这么糊弄?
在 MySQL 9.0 的 官方文档 上,只有三个关于向量类型的函数。抛开与字符串互转的两个,真正的功能函数就一个 VECTOR_DIM
:返回向量的维度!(计算数组长度)
向量数据库的门槛不是一般的低 —— 有个向量距离函数就行(内积,10行C代码,小学生水平编程任务),这样至少可以通过全表扫描求距离 + ORDER BY d LIMIT n
实现向量检索,是个可用的状态。
但 MySQL 9 甚至连这样一个最基本的向量距离函数都懒得去实现,这绝对不是能力问题,而是 Oracle 根本就不想好好做 MySQL 了。
老司机一眼就能看出这里的所谓 “向量类型” 不过是 BLOB
的别名 —— 它只管你写入二进制数据,压根不管用户怎么查找使用。
当然,也不排除 Oracle 在自己的 MySQL Heatwave 上有一个不糊弄的版本。可在 MySQL 上,最后实际交付的东西,就是一个十分钟就能写完的玩意糊弄了事。
不糊弄的例子可以参考 MySQL 的老对手 PostgreSQL。在过去一年中,PG 生态里就涌现出了至少六款向量数据库扩展( pgvector
,pgvector.rs
,pg_embedding
,latern
,pase
,pgvectorscale
),并在你追我赶的赛马中卷出了新高度。
最后的胜出者是 2021 年就出来的 pgvector
,它在无数开发者、厂商、用户的共同努力下,站在 PostgreSQL 的肩膀上,很快便达到了许多专业向量数据库都无法企及的高度,甚至可以说凭借一己之力,干死了这个数据库细分领域 —— 《专用向量数据库凉了吗?》。
在这一年内,pgvector
性能翻了 150 倍,功能上更是有了翻天覆地的变化 —— pgvector
提供了 float向量,半精度向量,bit向量,稀疏向量几种数据类型;提供了L1距离,L2距离,内积距离,汉明距离,Jaccard距离度量函数;提供了各种向量、标量计算函数与运算符;支持 IVFFLAT,HNSW 两种专用向量索引算法(扩展的扩展 pgvectorscale 还提供了 DiskANN 索引);支持了并行索引构建,向量量化处理,稀疏向量处理,子向量索引,混合检索,可以使用 SIMD 指令加速。这些丰富的功能,加上开源免费的协议,以及整个 PG 生态的合力与协同效应 —— 让 pgvector
大获成功,并与 PostgreSQL 一起,成为无数 AI 项目使用的默认(向量)数据库。
拿 pgvector
与来比似乎不太合适,因为 MySQL 9 所谓的“向量”,甚至都远远不如 1996 年 PG 诞生时自带的“多维数组类型” —— “至少它还有一大把数组函数,而不是只能求个数组长度”。
向量是新的JSON,然而向量数据库的宴席都已经散场了,MySQL 都还没来得及上桌 —— 它完美错过了下一个十年 AI 时代的增长动能,正如它在上一个十年里错过互联网时代的JSON文档数据库一样。
姗姗来迟的JS函数
另一个 MySQL 9.0 带来的 “重磅” 特性是 —— Javascript 存储过程。
然而用 Javascript 写存储过程并不是什么新鲜事 —— 早在 2011 年,PostgreSQL 9.1 就已经可以通过 plv8
扩展编写 Javascript 存储过程了,MongoDB 也差不多在同一时期提供了对 Javascript 存储过程的支持。
如果我们查看 DB-Engine 近十二年的 “数据库热度趋势” ,不难发现只有 PostgreSQL 与 Mongo 两款 DBMS 在独领风骚 —— MongoDB (2009) 与 PostgreSQL 9.2 (2012) 都极为敏锐地把握住了互联网开发者的需求 —— 在 “JSON崛起” 的第一时间就添加 JSON 特性支持(文档数据库),从而在过去十年间吃下了数据库领域最大的增长红利。
当然,MySQL 的干爹 —— Oracle 也在2014年底的12.1中添加了 JSON 特性与 Javascript 存储过程的支持 —— 而 MySQL 自己则不幸地等到了 2024 年才补上这一课 —— 但已经太迟了!
Oracle 支持用 C,SQL,PL/SQL,Pyhton,Java,Javascript 编写存储过程。但在 PostgreSQL 支持的二十多种存储过程语言面前,只能说也是小巫见大巫,只能甘拜下风了:
不同于 PostgreSQL 与 Oracle 的开发理念,MySQL 的各种最佳实践里都不推荐使用存储过程 —— 所以 Javascript 函数对于 MySQL 来说是个鸡肋特性。 然而即便如此,Oracle 还是把 Javascript 存储过程支持做成了一个 MySQL企业版专属 的特性 —— 考虑到绝大多数 MySQL 用户使用的都是开源社区版本,这个特性属实是发布了个寂寞。
日渐落后的功能特性
MySQL 在功能上缺失的绝不仅仅是是编程语言/存储过程支持,在各个功能维度上,MySQL 都落后它的竞争对手 PostgreSQL 太多了 —— 功能落后不仅仅是在数据库内核功能上,更发生在扩展生态维度。
来自 CMU 的 Abigale Kim 对主流数据库的可扩展性进行了研究:PostgreSQL 有着所有 DBMS 中最好的 可扩展性(Extensibility),以及其他数据库生态难望其项背的扩展插件数量 —— 375+,这还只是 PGXN 注册在案的实用插件,实际生态扩展总数已经破千。
这些扩展插件为 PostgreSQL 提供了各种各样的功能 —— 地理空间,时间序列,向量检索,机器学习,OLAP分析,全文检索,图数据库,让 PostgreSQL 真正成为一专多长的全栈数据库 —— 单一数据库选型便可替代各式各样的专用组件: MySQL,MongoDB,Kafka,Redis,ElasticSearch,Neo4j,甚至是专用分析数仓与数据湖。
当 MySQL 还局限在 “关系型 OLTP 数据库” 的定位时, PostgreSQL 早已经放飞自我,从一个关系型数据库发展成了一个多模态的数据库,成为了一个数据管理的抽象框架与开发平台。
PostgreSQL正在吞噬数据库世界 —— 它正在通过插件的方式,将整个数据库世界内化其中。“一切皆用 Postgres” 也已经不再是少数精英团队的前沿探索,而是成为了一种进入主流视野的最佳实践。
而在新功能支持上,MySQL 却显得十分消极 —— 一个应该有大量 Breaking Change 的“创新大版本更新”,不是糊弄人的摆烂特性,就是企业级的特供鸡肋,一个大版本就连鸡零狗碎的小修小补都凑不够数。
越新越差的性能表现
缺少功能也许并不是一个无法克服的问题 —— 对于一个数据库来说,只要它能将自己的本职工作做得足够出彩,那么架构师总是可以多费些神,用各种其他的数据积木一起拼凑出所需的功能。
MySQL 曾引以为傲的核心特点便是 性能 —— 至少对于互联网场景下的简单 OLTP CURD 来说,它的性能是非常不错的。然而不幸地是,这一点也正在遭受挑战:Percona 的博文《Sakila:你将何去何从》中提出了一个令人震惊的结论:
MySQL 的版本越新,性能反而越差。
根据 Percona 的测试,在 sysbench 与 TPC-C 测试下,最新 MySQL 8.4 版本的性能相比 MySQL 5.7 出现了平均高达 20% 的下降。而 MySQL 专家 Mark Callaghan 进一步进行了 详细的性能回归测试,确认了这一现象:
MySQL 8.0.36 相比 5.6 ,QPS 吞吐量性能下降了 25% ~ 40% !
尽管 MySQL 的优化器在 8.x 有一些改进,一些复杂查询场景下的性能有所改善,但分析与复杂查询本来就不是 MySQL 的长处与适用场景,只能说聊胜于无。相反,如果作为基本盘的 OLTP CRUD 性能出了这么大的折损,那确实是完全说不过去的。
ClickBench:MySQL 打这个榜确实有些不明智
Peter Zaitsev 在博文《Oracle最终还是杀死了MySQL》中评论:“与 MySQL 5.6 相比,MySQL 8.x 单线程简单工作负载上的性能出现了大幅下滑。你可能会说增加功能难免会以牺牲性能为代价,但 MariaDB 的性能退化要轻微得多,而 PostgreSQL 甚至能在 新增功能的同时显著提升性能”。
MySQL的性能随版本更新而逐步衰减,但在同样的性能回归测试中,PostgreSQL 性能却可以随版本更新有着稳步提升。特别是在最关键的写入吞吐性能上,最新的 PostgreSQL 17beta1 相比六年前的 PG 10 甚至有了 30% ~ 70% 的提升。
在 Mark Callaghan 的 性能横向对比 (sysbench 吞吐场景) 中,我们可以看到五年前 PG 11 与 MySQL 5.6 的性能比值(蓝),与当下 PG 16 与 MySQL 8.0.34 的性能比值(红)。PostgreSQL 和 MySQL 的性能差距在这五年间拉的越来越大。
几年前的业界共识是 PostgreSQL 与 MySQL 在 简单 OLTP CRUD 场景 下的性能基本相同。然而此消彼长之下,现在 PostgreSQL 的性能已经远远甩开 MySQL 了。 PostgreSQL 的各种读吞吐量相比 MySQL 高 25% ~ 100% 不等,在一些写场景下的吞吐量更是达到了 200% 甚至 500% 的恐怖水平。
MySQL 赖以安身立命的性能优势,已经不复存在了。
无可救药的质量水平
如果新版本只是性能不好,总归还有办法来优化修补。但如果是质量出了问题,那真就是无可救药了。
例如,Percona 最近刚刚在 MySQL 8.0.38 以上的版本(8.4.x, 9.0.0)中发现了一个 严重Bug —— 如果数据库里表超过 1万张,那么重启的时候 MYSQL 服务器会直接崩溃! 一个数据库里有1万张表并不常见,但也并不罕见 —— 特别是当用户使用了一些分表方案,或者应用会动态创建表的时候。而直接崩溃显然是可用性故障中最严重的一类情形。
但 MySQL 的问题不仅仅是几个软件 Bug,而是根本性的问题 —— 《MySQL正确性竟有如此大的问题?》一文指出,在正确性这个体面数据库产品必须的基本属性上,MySQL 的表现一塌糊涂。
权威的分布式事务测试组织 JEPSEN 研究发现,MySQL 文档声称实现的 可重复读/RR 隔离等级,实际提供的正确性保证要弱得多 —— MySQL 8.0.34 默认使用的 RR 隔离等级实际上并不可重复读,甚至既不原子也不单调,连 单调原子视图/MAV 的基本水平都不满足。
MySQL 的 ACID 存在缺陷,且与文档承诺不符 —— 而轻信这一虚假承诺可能会导致严重的正确性问题,例如数据错漏与对账不平。对于一些数据完整性很关键的场景 —— 例如金融,这一点是无法容忍的。
此外,能“避免”这些异常的 MySQL 可串行化/SR 隔离等级难以生产实用,也非官方文档与社区认可的最佳实践;尽管专家开发者可以通过在查询中显式加锁来规避此类问题,但这样的行为极其影响性能,而且容易出现死锁。
与此同时,PostgreSQL 在 9.1 引入的 可串行化快照隔离(SSI) 算法可以用极小的性能代价提供完整可串行化隔离等级 —— 而且 PostgreSQL 的 SR 在正确性实现上毫无瑕疵 —— 这一点即使是 Oracle 也难以企及。
李海翔教授在《一致性八仙图》论文中,系统性地评估了主流 DBMS 隔离等级的正确性,图中蓝/绿色代表正确用规则/回滚避免异常;黄A代表异常,越多则正确性问题就越多;红“D”指使用了影响性能的死锁检测来处理异常,红D越多性能问题就越严重;
不难看出,这里正确性最好(无黄A)的实现是 PostgreSQL SR,与基于PG的 CockroachDB SR,其次是略有缺陷 Oracle SR;主要都是通过机制与规则避免并发异常;而 MySQL 出现了大面积的黄A与红D,正确性水平与实现手法糙地不忍直视。
做正确的事很重要,而正确性是不应该拿来做利弊权衡的。在这一点上,开源关系型数据库两巨头 MySQL 和 PostgreSQL 在早期实现上就选择了两条截然相反的道路: MySQL 追求性能而牺牲正确性;而学院派的 PostgreSQL 追求正确性而牺牲了性能。
在互联网风口上半场中,MySQL 因为性能优势占据先机乘风而起。但当性能不再是核心考量时,正确性就成为了 MySQL 的致命出血点。 更为可悲的是,MySQL 连牺牲正确性换来的性能,都已经不再占优了,这着实让人唏嘘不已。
枯萎收缩的生态规模
对一项技术而言,用户的规模直接决定了生态的繁荣程度。瘦死的骆驼比马大,烂船也有三斤钉。 MySQL 曾经搭乘互联网东风扶摇而起,攒下了丰厚的家底,它的 Slogan 就很能说明问题 —— “世界上最流行的开源关系型数据库”。
不幸地是在 2023 年,至少根据全世界最权威的开发者调研之一的 StackOverflow Annual Developer Survey 结果来看,MySQL 的使用率已经被 PostgreSQL 反超了 —— 最流行数据库的桂冠已经被 PostgreSQL 摘取。
特别是,如果将过去七年的调研数据放在一起,就可以得到这幅 PostgreSQL / MySQL 在专业开发者中使用率的变化趋势图(左上) —— 在横向可比的同一标准下,PostgreSQL 流行与 MySQL 过气的趋势显得一目了然。
对于中国来说,此消彼长的变化趋势也同样成立。但如果对中国开发者说 PostgreSQL 比 MySQL 更流行,那确实是违反直觉与事实的。
将 StackOverflow 专业开发者按照国家细分,不难看出在主要国家中(样本数 > 600 的 31 个国家),中国的 MySQL 使用率是最高的 —— 58.2% ,而 PG 的使用率则是最低的 —— 仅为 27.6%,MySQL 用户几乎是 PG 用户的一倍。
与之恰好反过来的另一个极端是真正遭受国际制裁的俄联邦:由开源社区运营,不受单一主体公司控制的 PostgreSQL 成为了俄罗斯的数据库大救星 —— 其 PG 使用率以 60.5% 高居榜首,是其 MySQL 使用率 27% 的两倍。
中国因为同样的自主可控信创逻辑,最近几年 PostgreSQL 的使用率也出现了显著跃升 —— PG 的使用率翻了三倍,而 PG 与 MySQL 用户比例已经从六七年前的 5:1 ,到三年前的3:1,再迅速发展到现在的 2:1,相信会在未来几年内会很快追平并反超世界平均水平。 毕竟,有这么多的国产数据库,都是基于 PostgreSQL 打造而成 —— 如果你做政企信创生意,那么大概率已经在用 PostgreSQL 了。
抛开政治因素,用户选择使用一款数据库与否,核心考量还是质量、安全、效率、成本等各个方面是否“先进”。先进的因会反映为流行的果,流行的东西因为落后而过气,而先进的东西会因为先进变得流行,没有“先进”打底,再“流行”也难以长久。
究竟是谁杀死了MySQL?
究竟是谁杀死了 MySQL,难道是 PostgreSQL 吗?Peter Zaitsev 在《Oracle最终还是杀死了MySQL》一文中控诉 —— Oracle 的不作为与瞎指挥最终害死了 MySQL;并在后续《Oracle还能挽救MySQL吗》一文中指出了真正的根因:
MySQL 的知识产权被 Oracle 所拥有,它不是像 PostgreSQL 那种 “由社区拥有和管理” 的数据库,也没有 PostgreSQL 那样广泛的独立公司贡献者。不论是 MySQL 还是其分叉 MariaDB,它们都不是真正意义上像 Linux,PostgreSQL,Kubernetes 这样由社区驱动的的原教旨纯血开源项目,而是由单一商业公司主导。
比起向一个商业竞争对手贡献代码,白嫖竞争对手的代码也许是更为明智的选择 —— AWS 和其他云厂商利用 MySQL 内核参与数据库领域的竞争,却不回馈任何贡献。于是作为竞争对手的 Oracle 也不愿意再去管理好 MySQL,而干脆自己也参与进来搞云 —— 仅仅只关注它自己的 MySQL heatwave 云版本,就像 AWS 仅仅专注于其 RDS 管控和 Aurora 服务一样。在 MySQL 社区凋零的问题上,云厂商也难辞其咎。
逝者不可追,来者犹可待。PostgreSQL 应该从 MySQL 的衰亡中吸取教训 —— 尽管 PostgreSQL 社区非常小心地避免出现一家独大的情况出现,但生态确实在朝着一家/几家巨头云厂商独大的不利方向在发展。云正在吞噬开源 —— 云厂商编写了开源软件的管控软件,组建了专家池,通过提供维护攫取了软件生命周期中的绝大部分价值,但却通过搭便车的行为将最大的成本 —— 产研交由整个开源社区承担。而 真正有价值的管控/监控代码却从来不回馈开源社区 —— 在数据库领域,我们已经在 MongoDB,ElasticSearch,Redis,以及 MySQL 上看到了这一现象,而 PostgreSQL 社区确实应当引以为鉴。
好在 PG 生态总是不缺足够头铁的人和公司,愿意站出来维护生态的平衡,反抗公有云厂商的霸权。例如,我自己开发的 PostgreSQL 发行版 Pigsty,旨在提供一个开箱即用、本地优先的开源云数据库 RDS 替代,将社区自建 PostgreSQL 数据库服务的底线,拔高到云厂商 RDS PG 的水平线。而我的《云计算泥石流》系列专栏则旨在扒开云服务背后的信息不对称,从而帮助公有云厂商更加体面,亦称得上是成效斐然。
尽管我是 PostgreSQL 的坚定支持者,但我也赞同 Peter Zaitsev 的观点:“如果 MySQL 彻底死掉了,开源关系型数据库实际上就被 PostgreSQL 一家垄断了,而垄断并不是一件好事,因为它会导致发展停滞与创新减缓。PostgreSQL 要想进入全盛状态,有一个 MySQL 作为竞争对手并不是坏事”
至少,MySQL 可以作为一个鞭策激励,让 PostgreSQL 社区保持凝聚力与危机感,不断提高自身的技术水平,并继续保持开放、透明、公正的社区治理模式,从而持续推动数据库技术的发展。
MySQL 曾经也辉煌过,也曾经是“开源软件”的一杆标杆,但再精彩的演出也会落幕。MySQL 正在死去 —— 更新疲软,功能落后,性能劣化,质量出血,生态萎缩,此乃天命,实非人力所能改变。 而 PostgreSQL ,将带着开源软件的初心与愿景继续坚定前进 —— 它将继续走 MySQL 未走完的长路,写 MySQL 未写完的诗篇。
PG驶向云外,MySQL安魂九霄
我那些残梦,灵异九霄
徒忙漫奋斗,满目沧愁
在滑翔之后,完美坠落
在四维宇宙,眩目遨游
我那些烂曲,流窜九州
云游魂飞奏,音愤符吼
在宿命身后,不停挥手
视死如归仇,毫无保留
黑色的不是夜晚,是漫长的孤单
看脚下一片黑暗,望头顶星光璀璨
叹世万物皆可盼,唯真爱最短暂
失去的永不复返,世守恒而今倍还
摇旗呐喊的热情,携光阴渐远去
人世间悲喜烂剧,昼夜轮播不停
纷飞的滥情男女,情仇爱恨别离
一代人终将老去,但总有人正年轻
参考阅读
PostgreSQL 17 Beta1 发布!牙膏管挤爆了!
CVE-2024-6387 SSH 漏洞修复
漏洞描述,CVE-2024-6387: https://nvd.nist.gov/vuln/detail/CVE-2024-6387
基本上影响的都是比较新版本的操作系统,老的系统,比如 CentOS 7.9,RockyLinux 8.9 ,Ubuntu 20.04,Debian 11 因为 OpenSSH 版本老逃过一劫。
在 Pigsty 支持的操作系统发行版中,RockyLinux 9.3,Ubuntu 22.04,Debian 12 受到影响:
ssh -V
OpenSSH_8.7p1, OpenSSL 3.0.7 1 Nov 2022 # rockylinux 9.3
OpenSSH_8.9p1 Ubuntu-3ubuntu0.6, OpenSSL 3.0.2 15 Mar 2022 # ubuntu 22.04
OpenSSH_9.2p1 Debian-2+deb12u2, OpenSSL 3.0.11 19 Sep 2023 # debian 12
诊断方法
漏洞公告:
RockyLinux 9+: https://rockylinux.org/news/2024-07-01-openssh-sigalrm-regression
Debian 12+: https://security-tracker.debian.org/tracker/CVE-2024-6387
Ubuntu 22.04+: https://ubuntu.com/security/CVE-2024-6387
处理方法
使用系统的默认包管理器升级 openssh-server
即可。
升级后的版本参考:
# rockylinux 9.3 : 8.7p1-34.el9 -------> 8.7p1-38.el9_4.1
# ubuntu 22.04 : -------> 8.9p1-3ubuntu0.6
# debian 12 : -------> 1:9.2p1-2+deb12u2
systemctl restart sshd
rocky9.3
$ rpm -q openssh-server
openssh-server-8.7p1-34.el9.x86_64 # vulnerable
$ yum install openssh-server
openssh-server-8.7p1-38.el9_4.1.x86_64 # fixed
debian12
$ dpkg -s openssh-server
$ apt install openssh-server
Version: 1:9.2p1-2+deb12u2 # fixed
ubuntu22.04
$ dpkg -s openssh-server
$ apt install openssh-server
Version: 1:8.9p1-3ubuntu0.6
后续改进
在 Pigsty 的下个版本 v2.8 中,默认会下载并安装当前最新版本的 openssh-server
,从而修复此漏洞。
Oracle 还能挽救 MySQL 吗?
Percona 作为 MySQL 生态的主要扛旗者,开发了一系列用户耳熟能详的工具:PMM 监控,XtraBackup 备份,PT 系列工具,以及 MySQL 发行版。 然而近日,Percona 创始人 Peter Zaitsev 在官方博客上公开表达了对 MySQL,及其知识产权属主 Oracle 的失望,以及对版本越高性能越差的不满,这确实是一个值得关注的信号。
作者:Percona Blog,Marco Tusa,MySQL 生态的重要贡献者,开发了知名的PT系列工具,MySQL备份工具,监控工具与发行版。
译者:冯若航,网名 Vonng,Pigsty 作者,PostgreSQL 专家与布道师。下云倡导者,数据库下云实践者。
我之前写了篇文章 Oracle最终还是杀死了MySQL ,引发了不少回应 —— 包括 The Register 上的几篇精彩文章(1, 2)。这确实引出了几个值得讨论的问题:
AWS和其他云厂商参与竞争,却不回馈任何贡献,那你还指望 Oracle 做啥呢?
首先 —— 我认为 AWS 和其他云厂商如果愿意对 MySQL 作出更多贡献,那当然是一件好事。 不过我们也应该注意到, Oracle 与这些公司都是竞争关系,并且在 MySQL 这并没有一个公平的竞争环境(AWS 为什么会来参与这种不公平的竞争是另一个话题)。
对你的竞争对手贡献知识产权可能并不是一个很好的商业决策,特别是 Oracle 还要求贡献者签署的 CLA(贡献者授权协议)。 只要 Oracle 拥有这些知识产权,合理的预期就是由 Oracle 自己来承担大部分维护、改进和推广 MySQL 的责任。
没错 …… ,但如果 Oracle 不愿意,或不再有能力管理好 MySQL,而仅仅只关注它自己的云版本,就像 AWS 仅仅专注于其 RDS 和 Aurora 服务,我们又能怎么办呢?
有一个解决方案 —— Oracle 应该将 MySQL Community 转让给 Linux Foundation、Apache Foundation 或其他独立实体,允许公平竞争,并专注于他们的 Cloud(Heatwave)和企业级产品。 有趣的是,Oracle 已经有了这样的先例:将 OpenOffice 转交给 Apache 软件基金会。
另一个很好的例子是 LinkerD —— 它由 Buoyant 公司 引入 CNCF —— 而 Buoyant 也在持续构建它的扩展版本 — Buoyant Enterprise for LinkerD。
在这种情况下,维护和发展开源的 MySQL 成为了一个生态问题:我很确信,如果不是向竞争对手拥有的知识产权贡献,AWS 与其他云厂商肯定愿意参与更多。实际上我们确实可以在 PostgreSQL、Linux 或 Kubernetes 项目中看到云厂商在大力参与。
有了 PostgreSQL;谁还需要 MySQL 呢?
PostgreSQL 确实是一个出色的数据库,有着活跃的社区,并且近年来发展迅速。然而仍有很多人更偏好于 MySQL ,也有很多现有应用程序仍然在使用 MySQL —— 因此我们希望 MySQL 能继续健康发展,长命百岁。
当然还有一点:如果 MySQL 死掉了,开源关系型数据库实际上就被 PostgreSQL 一家垄断了,在我看来,垄断并不是一件好事,因为它会导致发展停滞与创新减缓。PostgreSQL 要想进入全盛状态,有一个 MySQL 作为竞争对手并不是坏事。
难道 MariaDB 不是一个新的、更好的、由社区管理的 MySQL 吗?
我认为 MariaDB 的存在很好地向 Oracle 施加了压力,迫使其不得不投资 MySQL 。虽然我们没法确定地说如果没有 MariaDB 会怎样,但如果没有它,很可能 MySQL 很久以前就被 Oracle 忽视了。
话虽如此,虽然 MariaDB 在组织架构上与 Oracle 大有不同,但它也显然不是像 PostgreSQL 那种 “由社区拥有和管理” 的数据库,也没有 PostgreSQL 那样广泛的独立公司贡献者。我认为 MariaDB 确实可以采取一些措施,争取 MySQL 领域的领导地位,但这值得另单一篇文章展开。
总结一下
PostgreSQL 和 MariaDB 是出色的数据库,如果没有它们,开源社区将被绑死在 Oracle 的贼船上,陷入糟糕的境地,但它们今天都还不能完全替代 MySQL。 MySQL 社区的最好结果应该是 Oracle 与达成协议,共同努力,尽可能一起建设好 MySQL。如果不行,MySQL 社区需要一个计划B。
参考阅读
Is Oracle Finally Killing MySQL?
Postgres vs MySQL: the impact of CPU overhead on performance
Perf regressions in MySQL from 5.6.21 to 8.0.36 using sysbench and a small server
英文原文
I got quite a response to my article on whether Oracle is Killing MySQL, including a couple of great write-ups on The Register (1, 2) on the topic. There are a few questions in this discussion that I think are worth addressing.
AWS and other cloud vendors compete, without giving anything back, what else would you expect Oracle to do ?
First, yes. I think it would be great if AWS and other cloud providers would contribute more to MySQL. We should note, though, that Oracle is a competitor for many of those companies, and there is no “level playing field” when it comes to MySQL (the fact AWS is willing on this unlevel field is another point). Contributing IP to your competitor, especially considering CLA Oracle requires might not be a great business decision. Until Oracle owns that IP, it is reasonable to expect, for Oracle to have most of the burden to maintain, improve, and promote MySQL, too.
Yes… but what if Oracle is unwilling or unable to be a great MySQL steward anymore and would rather only focus on its cloud version, similar to AWS being solely focused on its RDS and Aurora offerings? *There is a solution for that – Oracle should transfer MySQL Community to Linux Foundation, Apache Foundation, or another independent entity, open up the level playing field, and focus on their Cloud (Heatwave) and Enterprise offering.* Interestingly enough, there is already a precedent for that with Oracle transferring OpenOffice to Apache Software Foundation.
Another great example would be LinkerD — which was brought to CNCF by Buyant — which continues to build its extended edition – Buoyant Enterprise for LinkerD.
In this case, maintaining and growing open source MySQL will become an ecosystem problem and I’m quite sure AWS and other cloud vendors will participate more when they are not contributing to IP owned by their competitors. We can actually see it with PostgreSQL, Linux, or Kubernetes projects which have great participation from cloud vendors.
There is PostgreSQL; who needs MySQL anyway?
Indeed, PostgreSQL is a fantastic database with a great community and has been growing a lot recently. Yet there are still a lot of existing applications on MySQL and many folks who prefer MySQL, and so we need MySQL healthy for many years to come. But there is more; if MySQL were to die, we would essentially have a monopoly with popular open source relational databases, and, in my opinion, monopoly is not a good thing as it leads to stagnation and slows innovation. To have PostgreSQL to be as great as it can be it is very helpful to have healthy competition from MySQL!
Isn’t MariaDB a new, better, community-governed MySQL ?
I think MariaDB’s existence has been great at putting pressure on Oracle to invest in MySQL. We can’t know for certain “what would have been,” but chances are we would have seen more MySQL neglect earlier if not for MariaDB. Having said that, while organizationally, MariaDB is not Oracle, it is not as cleanly “community owned and governed” as PostgreSQL and does not have as broad a number of independent corporate contributors as PostgreSQL.I think there are steps MariaDB can do to really take a leadership position in MySQL space… but it deserves another article.
To sum things up
PostgreSQL and MariaDB are fantastic databases, and if not for them, the open source community would be in a very bad bind with Oracle’s current MySQL stewardship. Neither is quite a MySQL replacement today, and the best outcome for the MySQL community would be for Oracle to come to terms and work with the community to build MySQL into the best database it can be. If not, the MySQL community needs to come up with a plan B.
Oracle最终还是杀死了MySQL
大约15年前,Oracle收购了Sun公司,从而也拥有了MySQL,互联网上关于Oracle何时会“扼杀MySQL”的讨论此起彼伏。当时流传有各种理论:从彻底扼杀 MySQL 以减少对 Oracle 专有数据库的竞争,到干掉 MySQL 开源项目,只留下 “MySQL企业版” 作为唯一选择。这些谣言的传播对 MariaDB,PostgreSQL 以及其他小众竞争者来说都是好生意,因此在当时传播得非常广泛。
作者:Percona Blog,Marco Tusa,MySQL 生态的重要贡献者,开发了知名的PT系列工具,MySQL备份工具,监控工具与发行版。
译者:冯若航,网名 Vonng,Pigsty 作者,PostgreSQL 专家与布道师。下云倡导者,数据库下云实践者。
然而实际上,Oracle 最终把 MySQL 管理得还不错。MySQL 团队基本都保留下来了,由 MySQL 老司机 Tomas Ulin 掌舵。MySQL 也变得更稳定、更安全。许多技术债务也解决了,许多现代开发者想要的功能也有了,例如 JSON支持和高级 SQL 标准功能的支持。
虽然确实有 “MySQL企业版” 这么个东西,但它实际上关注的是开发者不太在乎的企业需求:可插拔认证、审计、防火墙等等。虽然也有专有的 GUI 图形界面、监控与备份工具(例如 MySQL 企业监控),但业内同样有许多开源和商业软件竞争者,因此也说不上有特别大的供应商锁定。
在此期间我也常为 Oracle 辩护,因为许多人都觉得 MySQL 会遭受虐待,毕竟 —— Oracle 的名声确实比较糟糕。
不过在那段期间,我认为 Oracle 确实遵守了这条众所周知的开源成功黄金定律:“转换永远不应该妨碍采用”
注:“Conversion should never compromise Adoption” 这句话指在开发或改进开源软件时,转换或升级过程中的任何变动都不应妨碍现有用户的使用习惯或新用户的加入。
然而随着近些年来 Oracle 推出了 “MySQL Heatwave”(一种 MySQL 云数据库服务),事情开始起变化了。
MySQL Heatwave 引入了许多 MySQL 社区版或企业版中没有的功能,如 加速分析查询 与 机器学习。
在“分析查询”上,MySQL 的问题相当严重,到现在甚至都还不支持 并行查询。市场上新出现的 CPU 核数越来越多,都到几百个了,但单核性能并没有显著增长,而不支持并行严重制约了 MySQL 的分析性能提升 —— 不仅仅影响分析应用的查询,日常事务性应用里面简单的 GROUP BY
查询也会受影响。(备注:MySQL 8 对 DDL 有一些 并行支持,但查询没有这种支持)
这么搞的原因,是不是希望用户能够有更多理由去买 MySQL Heatwave?但或者,人们其实也可以直接选择用分析能力更强的 PostgreSQL 和 ClickHouse。
另一个开源 MySQL 极为拉垮的领域是 向量检索。其他主流开源数据库都已经添加了向量检索功能,MariaDB 也正在努力实现这个功能,但就目前而言,MySQL 生态里只有云上限定的 MySQL Heatwave 才有这个功能,这实在是令人遗憾。
然后就是最奇怪的决策了 —— Javascript 功能只在企业版中提供,我认为 MySQL 应该尽可能去赢得 Javascript 开发者的心,而现在很多 JS 开发者都已经更倾向于更简单的 MongoDB 了。
我认为这些决策都违背了前面提到的开源黄金法则 —— 它们显然限制了 MySQL 的采用与普及 —— 不论是这些“XX限定”的特定功能,还是对 MySQL 未来政策变化的担忧。
这还没完,MySQL 的性能也出现了严重下降,也许是因为 多年来无视性能工程部门。与MySQL 5.6 相比,MySQL 8.x 单线程简单工作负载上的性能出现了大幅下滑。你可能会说增加功能难免会以牺牲性能为代价,但 MariaDB 的性能退化要轻微得多,而 PostgreSQL 甚至能在 新增功能的同时 显著提升性能。
显然,我不知道 Oracle 管理团队是怎么想的,也不能说这到底是蠢还是坏,但过去几年的这些产品决策,显然不利于 MySQL 的普及,特别是在同一时间,PostgreSQL 在引领用户心智上高歌猛进,根据 DB-Engines 热度排名,大幅缩小了与 MySQL 的差距;而根据 StackOverflow开发者调查 ,甚至已经超过 MySQL 成为最流行的数据库了。
无论如何,除非甲骨文转变其关注点,顾及现代开发者对关系数据库的需求,否则 MySQL 迟早要完 —— 无论是被 Oracle 的行为杀死,还是被 Oracle 的不作为杀死。
参考阅读
Is Oracle Finally Killing MySQL?
Postgres vs MySQL: the impact of CPU overhead on performance
Perf regressions in MySQL from 5.6.21 to 8.0.36 using sysbench and a small server
MySQL性能越来越差,Sakila将何去何从?
在 Percona,我们时刻关注用户的需求,并尽力满足他们。我们特别监控了 MySQL 版本的分布和使用情况,发现了一个引人注目的趋势:从版本 5.7 迁移到 8.x 的步伐明显缓慢。更准确地说,许多用户仍需坚持使用 5.7 版本。
基于这一发现,我们采取了几项措施。首先,我们与一些仍在使用 MySQL 5.7 的用户聊了聊,探究他们不想迁移到 8.x 的原因。为此,我们制定了 EOL 计划,为 5.7 版本提供延长的生命周期支持,确保需要依赖旧版本、二进制文件及代码修复的用户能够得到专业支持。
同时,我们对不同版本的 MySQL 进行了广泛测试,以评估是否有任何性能下降。虽然测试尚未结束,但我们已经收集了足够的数据,开始绘制相关图表。本文是对我们测试结果的初步解读。
剧透警告:对于像我这样热爱 Sakila 的人来说,这些发现可能并不令人高兴。
译者注:Sakila 是 MySQL 的吉祥物海豚
作者:Percona Blog,Marco Tusa,MySQL 生态的重要贡献者,开发了知名的PT系列工具,MySQL备份工具,监控工具与发行版。
译者:冯若航,网名 Vonng,Pigsty 作者,PostgreSQL 专家与布道师。下云倡导者,数据库下云实践者。
测试
假设
测试的方法五花八门,我们当然明白,测试结果可能因各种要素而异,(例如:运行环境, MySQL 服务器配置)。但如果我们在同样的平台上,比较同一个产品的多个版本,那么可以合理假设,在不改变 MySQL 服务器配置的前提下,影响结果的变量可以最大程度得到控制。
因此,我首先根据 MySQL 默认配置 运行性能测试,这里的工作假设很明确,你发布产品时使用的默认值,通常来说是最安全的配置,也经过了充分的测试。
当然,我还做了一些 配置优化 ,并评估优化后的参数配置会如何影响性能。
我们进行哪些测试?
我们跑了 sysbench 与 TPC-C Like 两种 Benchmark。 可以在这里找到完整的测试方法与细节,实际执行的命令则可以在这里找到:
结果
我们跑完了上面一整套测试,所有的结果都可以在这里找到。
但为了保持文章的简洁和高质量,我在这里只对 Sysbench 读写测试和 TPC-C 的结果进行分析与介绍。 之所以选择这两项测试,是因为它们直接且全面地反映了 MySQL 服务器的表现,同时也是最常见的应用场景。其他测试更适合用来深入分析特定的问题。
在此报告中,下面进行的 sysbench 读写测试中,写操作比例约为 36%,读操作比例约为 64%,读操作由点查询和范围查询组成。而在 TPC-C 测试中,读写操作的比例则均为 50/50 %。
sysbench 读写测试
首先我们用默认配置来测试不同版本的 MySQL。
小数据集,默认配置:
小数据集,优化后的结果:
大数据集,默认配置:
大数据集,优化配置:
前两幅图表很有趣,但很显然说明了一点,我们不能拿默认配置来测性能,我们可以用它们作为基础,从中找出更好的默认值。
Oracle 最近决定在 8.4 中修改许多参数的默认值,也证实了这一点(参见文章)。
有鉴于此,我将重点关注通过优化参数配置后进行的性能评测结果。
看看上面的图表,我们不难看出:
- 使用默认值的 MySQL 5.7 ,在两种情况(大小数据集)下的表现都更好。
- MySQL 8.0.36 因为默认配置参数不佳,使其在第一种(小数据集)的情况表现拉垮。但只要进行一些优化调整,就能让它的性能表现超过 8.4,并更接近 5.7。
TPC-C 测试
如上所述,TPC-C 测试应为写入密集型,会使用事务,执行带有 JOIN,GROUP,以及排序的复杂查询。
我们使用最常用的两种 隔离等级,可重复读(Repeatable Read),以及读已提交(Read Committed),来运行 TPC-C 测试。
尽管我们在多次重复测试中遇到了一些问题,但都是因为一些锁超时导致的随机问题。因此尽管图中有一些空白,但都不影响大趋势,只是压力打满的表现。
TPC-C,优化配置,RR隔离等级:
TPC-C,优化配置,RC隔离等级:
在本次测试中,我们可以观察到,MySQL 5.7 的性能比其他 MySQL 版本要更好。
与 Percona 的 MySQL 和 MariaDB 比会怎样?
为了简洁起见,我将仅在这里介绍优化参数配置的测试,原因上面说过了,默认参数没毛用没有。
sysbench读写,小数据集的测试结果:
sysbench读写,大数据集的测试结果:
当我们将 MySQL 的各个版本与 Percona Server MySQL 8.0.36 以及 MariaDB 11.3 进行对比时, 可以看到 MySQL 8.4 只有和 MariaDB 比时表现才更好,与 MySQL 8.0.36 比较时仍然表现落后。
TPC-C
TPC-C,RR隔离等级的测试结果:
TPC-C,RC隔离等级的测试结果:
正如预期的那样,MySQL 8.4 在这里的表现也不佳,只有 MariaDB 表现更差来垫底。 顺便一提,Percona Server for MySQL 8.0.36 是唯一能处理好并发争用增加的 MySQL。
这些测试说明了什么?
坦白说,我们在这里测出来的结果,也是我们大多数用户的亲身经历 —— MySQL 的性能随着版本增加而下降。
当然,MySQL 8.x 有一些有趣的新增功能,但如果你将性能视为首要且最重要的主题,那么 MySQL 8.x 并没有更好。
话虽如此,我们必须承认 —— 大多数仍在使用 MySQL 5.7 的人可能是对的(有成千上万的人)。为什么要冒着极大的风险进行迁移,结果发现却损失了相当大一部分的性能呢?
关于这一点,可以用 TPC-C 测试结果来说明,我们可以把数据转换为每秒事务数吞吐量,然后比较性能损失了多少:
TPC-C,RR隔离等级,MySQL 8.4 的性能折损:
TPC-C,RC隔离等级,MySQL 8.4 的性能折损:
我们可以看到,在两项测试中,MySQL 8.x 的性能劣化都非常明显,而其带来的好处(如果有的话)却并不显著。
使用数据的绝对值:
TPC-C,RR隔离等级,MySQL 8.4 的性能折损:
TPC-C,RC隔离等级,MySQL 8.4 的性能折损:
在这种情况下,我们需要问一下自己:我的业务可以应对这样的性能劣化吗?
一些思考
当年 MySQL 被卖给 SUN Microsystems 时,我就在 MySQL AB 工作,我对这笔收购非常不高兴。 当 Oracle 接管 SUN 时,我非常担心 Oracle 可能会决定干掉 MySQL,我决定加入另一家公司继续搞这个。
此后几年里,我改了主意,开始支持和推广 Oracle 在 MySQL 上的工作。从各种方面来看,我现在依然还在支持和推广它。
他们在规范开发流程方面做得很好,代码清理工作也卓有成效。但是,其他代码上却没啥进展,我们看到的性能下降,就是这种缺乏进展的代价;请参阅 Peter 的文章《Oracle 最终会杀死 MySQL 吗?》。
另一方面,我们不得不承认 Oracle 确实在 OCI/MySQL/Heatwave 这些产品的性能和功能上投资了很多 —— 只不过这些改进没有体现在 MySQL 的代码中,无论是社区版还是企业版。
再次强调,我认为这一点非常可悲,但我也能理解为什么。
当 AWS 和 Google 等云厂商使用 MySQL 代码、对其进行优化以供自己使用、赚取数十亿美元,甚至不愿意将代码回馈时,凭什么 Oracle 就要继续免费优化 MySQL 的代码?
我们知道这种情况已经持续了很多年了,我们也知道这对开源生态造成了极大的负面影响。
MySQL 只不过是更大场景中的一块乐高积木而已,在这个场景中,云计算公司正在吞噬其他公司的工作成果,自己用来发大财。
我们又能做什么?我只能希望我们能很快看到不一样的东西:开放代码,投资项目,帮助像 MySQL 这样的社区收复失地。
与此同时,我们必须承认,许多客户与用户使用 MySQL 5.7 是有非常充分的理由的。 在我们能解决这个问题之前,他们可能永远也不会决定迁移,或者,如果必须迁移的话,迁移到其他替代上,比如 PostgreSQL。
然后,Sakila 将像往常一样,因为人类的贪婪而缓慢而痛苦地死去,从某种意义上说,这种事儿并不新鲜,但很糟糕。
祝大家使用 MySQL 快乐。
参考阅读
Perf regressions in MySQL from 5.6.21 to 8.0.36 using sysbench and a small server
国产数据库到底能不能打?
总有朋友问我,国产数据库到底能不能打?说实话,是个得罪人的问题。所以我们不妨试试用数据说话 —— 希望本文提供的图表,能够帮助读者了解数据库生态格局,并建立更为准确的比例感认知。
数据来源与研究方法
评价一个数据库“能不能打”有许多种方式,但 “流行度” 是最常见的指标。对一项技术而言,流行度决定了用户的规模与生态的繁荣程度,唯有这种最终存在意义上的结果才能让所有人心服口服。
关于数据库流行度这个问题,我认为有三份数据可以作为参考:StackOverflow 全球开发者调研[1],DB-Engine 数据库流行度排行榜[2],以及墨天轮国产数据库排行榜[3]。
其中最有参考价值的是 StackOverflow 2017 - 2023 年的全球开发者问卷调研 —— 样本调查获取的第一手数据具有高度的可信度与说服力,并且具有极好的 横向可比性(在不同数据库之间水平对比);连续七年的调查结果也有着足够的 纵向可比性 (某数据库和自己过去的历史对比)。
其次是 DB-Engine 数据库流行度排行榜, DB-Engine 属于综合性热搜指数,将 Google, Bing, Google Trends,StackOverflow,DBA Stack Exchange,Indeed, Simply Hired, LinkedIn,Twitter 上的间接数据合成了一个热搜指数。
热度指数有着很好的 纵向可比性 —— 我们可以用它来判断某个数据库的流行度走势 —— 是更流行了还是更过气了,因为评分标准是一样的。但在 横向可比性 上表现不佳 —— 例如你没办法细分用户搜索的目的。所以热度指标在横向对比不同数据库时只能作为一个模糊的参考 —— 但在数量级上的准确性还是OK的。
第三份数据是墨天轮的 “国产数据库排行榜”,这份榜单收录了 287 个国产数据库,主要价值是给我们提供了一份国产数据库名录。这里我们简单认为 —— 收录在这里的数据库,就算“**国产数据库”**了 —— 尽管这些数据库团队不一定会自我认知为国产数据库。
有了这三份数据,我们就可以尝试回答这个问题 —— 国产数据库在国际上的流行度与影响力到底是什么水平?
锚点:TiDB
TiDB 是唯一一个,同时出现在三个榜单里的数据库,因此可以作为锚点。
在 StackOverflow 2023 调研 中,TiDB 作为最后一名,首次出现在数据库流行度榜单里,也是唯一入选的 “国产数据库”。图左中,TiDB 的开发者使用率为 0.20%,与排名第一的 PostgreSQL (45.55%) 和排名第二的 MySQL (41.09%) 相比,流行度相差了大约 两三百倍。
第二份 DB-Engine 数据可以交叉印证这一点 —— TiDB 在 DB-Engine 上的评分是国产数据库中最高的 —— 在2024年4月份,为 5.14 分。关系型数据库四大天王( PostgreSQL,MySQL,Oracle,SQL Server)相比,也是小几百倍的差距。
在墨天轮国产数据库排名中,TiDB 曾经长时间占据了榜首的位置,尽管最近两年前面加塞了 OceanBase, PolarDB,openGauss 三个数据库,但它还在第一梯队里,称其为国产数据库标杆没有太大问题。
如果我们以 TiDB 作为参考锚点,将这三份数据融合,立即就能得出一个有趣的结论:国产数据库看上去人才济济,群英荟萃,但即使是最能打的国产数据库,流行度与影响力也不及头部开源数据库的百分之一… 。
整体来看,这些被归类为“国产数据库”的产品,绝大多数在国际上的影响力可以评为:微不足道。
微不足道的战五渣
在 DB-Engine 收录的全球 478 款数据库中,可以找到 46 款列入墨天轮国产数据库名单的产品。将其过去十二年间的流行度绘制在图表上,得到下图 —— 乍看之下,好一片 “欣欣向荣”,蓬勃发展的势头。
然而,当我们把关系数据库四大天王:PostgreSQL,MySQL,Oracle,SQL Server 的热度趋势同样画在这张图上后,看上去就变得大不一样了 —— 你几乎看不到任何一个“国产数据库”了。
把整个国产数据库的热度分数全加起来,也甚至还达不到 PostgreSQL 流行度的零头。 整体合并入 “其他” 统计项中毫无任何违和感。
如果把所有国产数据库视作一个整体,在这个榜单里面可以凭 34.7 分排到第 26 名,占总分数的千分之五。(最上面一条黑带)
这个数字,差不多就是国产数据库国际影响力(DB-Engine)的一个摘要概括:尽管在数量上占了 1/10(如果以墨天轮算可以近半),但总影响力只有千分之五。其中的最强者 TiDB,战斗力也只有5 ……
当然再次强调,热度/指数类数据横向可比性非常一般,仅适合在数量级层面用作参考 —— 但这也足够得出一些结论了……
过气中的数据库们
从 DB-Engine 的热度趋势上看,国产数据库从 2017 - 2020 年开始起势,从 2021 年进入高潮,在 23年5月进入平台期,从今年年初开始,出现掉头过气的趋势。这和许多业内专家的判断一致 —— 2024 年,国产数据库进入洗牌清算期 —— 大量数据库公司将倒闭破产或被合并收编。
如果我们去掉个别出海开源做的还不错的头部“国产”数据库 —— 这个掉头而下的过气趋势会更加明显。
但过气这件事,并非国产数据库所独有 — 其实绝大多数的数据库其实都正在过气中。DB-Engine 过去12 年中的流行度数据趋势可以揭示这一点 —— 尽管 DB-Engine 热度指标的的横向可比性很一般,但纵向可比性还是很不错的 —— 因此在判断流行 & 过气趋势上仍然有很大的参考价值。
我们可以对图表做一个加工处理 —— 以某一年为零点,来看热度分数从此刻起的变化,从而看出那些数据库正在繁荣发展,哪些数据库正在落伍过气。
如果我们将目光聚焦在最近三年,不难发现在所有数据库中,只有 PostgreSQL 与 Snowflake 的流行度有显著增长。而最大的输家是 SQL Server,Oracle,MySQL,与 MongoDB …… 。分析数仓类组件(广义上的数据库)在最近三年有少量增长,而绝大部分其他数据库都处在过气通道中。
如果我们以 DB-Engine 最早有记录的 2012-11 作为参考零点,那么 PostgreSQL 是过去 12 年中数据库领域的最大赢家;而最大的输家依然是 SQL Server,Oracle,MySQL 御三家关系型数据库。
NoSQL 运动的兴起,让 MongoDB ,ElasticSearch,Redis 在 2012 - 2022 互联网黄金十年中获得了可观的增长,但这个增长的势头在最近几年已经结束了,并进入过气下降通道中,进入吃存量老本的状态。
至于 NewSQL 运动,即所谓的新一代分布式数据库。如果说 NoSQL 起码辉煌过,那么可以说 NewSQL 还没辉煌就已经熄火了。“分布式数据库” 在国内营销炒作的非常火热,以至于大家好像把它当作一个可以与 “集中式数据库” 分庭抗礼的数据库品类来看待。但如果我们深入研究就不难发现 —— 这其实只是一个非常冷门的数据库小众领域。
一些 NoSQL 组件的流行度还能和 PostgreSQL 放到同一个坐标图中而不显突兀,而所有 NewSQL 玩家加起来的流行度分数也比不上 PostgreSQL 的零头 —— 和“国产数据库”一样。
这些数据为我们揭示出数据库领域的基本格局:除了 PostgreSQL 之外的主要数据库都在过气中…
改头换面的 PostgreSQL 内战
这几份数据为我们揭示出数据库领域的基本格局 —— 除了 PostgreSQL 之外的主要数据库都在过气中,无论是 SQL,NoSQL,NewSQL,还是 国产数据库 。这确实抛出了一个有趣的问题,让人想问 —— 为什么?。
对于这个问题,我在 《PostgreSQL 正在吞噬数据库世界》中提出了一种简单的解释:PostgreSQL 正在凭借其强大的扩展插件生态,内化吞噬整个数据库世界。根据奥卡姆剃刀原理 —— 最简单的解释往往也最接近真相。
整个数据库世界的核心焦点,都已经聚焦在了金刚大战哥斯拉上:两个开源巨无霸数据库 PostgreSQL 与 MySQL 的使用率与其他数据库远远拉开了距离。其他一切议题与之相比都显得微不足道,无论是 NewSQL 还是 国产数据库。
看上去这场搏杀还要再过几年才能结束,但在远见者眼中,这场纷争几年前就已经尘埃落定了。
Linux 内核一统服务器操作系统天下后,曾经的同台竞争者 BSD,Solaris,Unix 都成为了时代的注脚。而我们正在目睹同样的事情在数据库领域发生 —— 在这个时代里,想发明新的实用数据库内核,约等于堂吉柯德撞风车。
好比今天尽管市面上有这么多的 Linux 操作系统发行版,但大家都选择使用同样的 Linux 内核,吃饱了撑着魔改 OS 内核属于没有困难创造困难也要上,会被业界当成 山炮 看待。
所以,并非所有国产数据库都不能打,而是能打的国产数据库,其实是改头换面的 PostgreSQL 与 MySQL 。如果 PostgreSQL 注定成为数据库领域的 Linux 内核,那么谁会成为 Postgres 的 Debian / Ubuntu / Suse / RedHat ?
国产数据库的竞争,变成了 PostgreSQL / MySQL 生态内部的竞争。一个国产数据库能打与否,取决于其 “含P量” —— 含有 PostgreSQL 内核的纯度与版本新鲜度。版本越新,魔改越少,附加值越高,使用价值就越高,也就越能打。
国产数据库看起来最能打的阿里 PolarDB (唯一入选 Gartner 领导者象限),基于三年前的 PostgreSQL 14 进行定制,且保持了 PG 内核的主体完整性,拥有最高的含P量。相比之下,openGauss 选择基于 12 年前的 PG 9.2 进行分叉,并魔改的亲爹都不认识了,所以含P量较低。介于两者中间的还有:PG 13 的 AntDB,PG 12 的人大金仓,PG 11 的老 Polar,PG XL 的 TBase ,……
因此,国产数据库到底能不能打 —— 真正的本质问题是:谁能代表 PostgreSQL 世界的先进生产力?
做内核的厂商不温不火,MariaDB 作为 MySQL 的亲爹 Fork 甚至都已经濒临退市,而白嫖内核自己做服务与扩展卖 RDS 的 AWS 可以赚的钵满盆翻,甚至凭借这种模式一路干到了全球数据库市场份额的榜首 —— 毫无疑问地证明:数据库内核已经不重要了,市场上稀缺的是服务能力整合。
在这场竞赛中,公有云 RDS 拿到了第一张入场券。而尝试在本地提供更好、更便宜、 RDS for PostgreSQL 的 Pigsty 对云数据库这种模式提出了挑战,同时还有十几款尝试用 云原生方式解决 RDS 本地化挑战的 Kubernetes Operator 正在摩拳擦掌,跃跃欲试,要把 RDS 拉下马来。
真正的竞争发生在服务/管控维度,而不是内核。
数据库领域正在从寒武纪大爆发走向侏罗纪大灭绝,在这一过程中,1% 的种子将会继承 99% 的未来,并演化出新的生态与规则。我希望数据库用户们可以明智地选择与决策,站在未来与希望的一侧,而不要把生命浪费在没有前途的事物上,比如……
References
注:本文使用的图表与数据,公开发布于 Pigsty Demo 站点:https://demo.pigsty.cc/d/db-analysis/
[1]
StackOverflow 全球开发者调研: https://survey.stackoverflow.co/2023/?utm_source=so-owned&utm_medium=blog&utm_campaign=dev-survey-results-2023&utm_content=survey-results#most-popular-technologies-database-prof
[2]
DB-Engine 数据库流行度排行榜: https://db-engines.com/en/ranking_trend
[3]
墨天轮国产数据库排行榜: https://www.modb.pro/dbRank
[4]
DB-Engine 数据分析: https://demo.pigsty.cc/d/db-analysis
[5]
StackOverflow 7年调研数据: https://demo.pigsty.cc/d/sf-survey
20刀好兄弟PolarDB:论数据库该卖什么价?
昨天一篇中标新闻引起关注与热议:《IT 行业烂了。。。1610 万大单。。。290 万(中)。。。维保 0.01 元。。。PolarDB 单价 130 元》。刚看到这个标题时,我也没特别惊讶,因为云上 PolarDB 数据库的单价我是非常清楚的,每vCPU·每月的价格在 ¥250 - ¥400,考虑到大客可以干到两三折的折扣也就是 50 ~ 120 块钱,“单价” 130 ¥ 并不算是什么离谱的报价数字。
所以当我看到 PolarDB 130块单价计费单位是 ”节点“ 时,绷不住了。这个“单价”指的不是云上常用的 ”每vCPU·包月“ 单价,而是线下私有化部署数据库部署,每个物理机节点的许可证单价,这就挺离谱。
PolarDB V2.0 不是什么魔改换皮的野鸡数据库,也算是正儿八经通过两次国测的国产信创数据库。这样一门Oracle能卖上亿的生意,现在卖出了总价两千块的白菜价。这让几万块一个节点的国产数据库友商们情何以堪?国内 IT 已经卷到这个阶段了吗?
今天我们就来聊一聊,数据库到底应该卖什么价?
商业数据库卖多少钱?
数据库管理系统软件,曾经(现在依然)是一门非常有利可图的生意。
作为商业数据库软件的标杆,Oracle 数据库的许可证授权 ,以常规买 Enterprise + RAC (47500 $ / 4vCPU + 23000 $ / 4vCPU) 计,约合 50 万人民币。一次性购买许可后,还有每年 22% 的服务费。
但是请注意,Oracle 上面单价的 “计费单位” 是 Processor,等于两个 Intel 物理核,4 线程 vCPU 虚拟核。也就是说如果折算成当代常用单位 vCPU·月,那么单个 vCPU 核的价格就是 12.7 万的一次性许可 + 2.8 万/年的服务费。
1 Oracle Processor = 2 Intel Core = 4 vCPU Thread-
假设你有一台 64 核的服务器用于跑 Oracle 数据库,那么许可证成本就是八百万,然后加上每年两百万的服务费。对了,所谓服务就是你有问题提个工单,原厂的人给你答疑。如果你要找人上门来服务,还有单独的人天费用。
当然众所周知,Oracle 使用 Paper License,你可以随便下载用(盗版)。当然 Oracle 最强大的部门 —— 法务也不是吃素的 —— 对中小用户来说还没这么快 —— 你可以先买一份 50万的许可证交个保护费份子钱,然后这事暂时就过去了。然后等肥了,这些欠下的保护费可是一分都少不了的。
https://www.oracle.com/a/ocom/docs/corporate/pricing/technology-price-list-070617.pdf
总的来说,传统商业数据库的定价模型就是这样的,按 processor 计费,单价几十万。
Oracle: y = a * x + b, a = 28K, b = 127K
当然,要我说的话,这种商业模式已经不合时宜了 —— 硬件已经今非昔比:当年处理器也就几核,现代物理机动辄就是小几百核;更重要的是,软件也有了开源平替:开源的 PostgreSQL 和 MySQL(划掉)已经足够好了。
开源数据库卖多少钱?
Oracle 的 CEO Larry 说过:“一旦开源替代变得足够好,与其竞争是疯狂与愚蠢的”。而现在,Oracle 的开源替代 “PostgreSQL” 已经远远超过 “足够好” 的程度了,实际上,它正如当年 Linux 横扫操作系统领域一样,正在疯狂吞噬着整个数据库世界,并且在最近已经隐晦地喊出了 “干翻 Oracle” 的口号。
像PostgreSQL / MySQL 为代表的的开源数据库,可以省掉软件 许可证 的费用。也就是说,你爱跑多少核就跑多少核,软件本身的成本归零了。实际上,这正是互联网繁荣的核心原因之一:Linux ,MySQL,PG,Apache,PHP 这样的开源软件让建设网站的软件边际成本无限趋近于零。
然而,这对于绝大多数的公司与企业来说并不是一个可行的选择。因为真正能玩转开源数据库的专家要比商业数据库 DBA 稀缺得多,而且大多数这类专家都集中在互联网公司中且待遇优惠,薪资不菲。对于许多中小公司来说,第一;很难找得到,认得出对的人;第二:不一定付得起这个钱,专家也不见得愿意去那里。
开源真正的 “商业模式”其实是:免费的开源软件吸引用户,用户需求产生开源专家岗位,开源软件专家作为企业的代理人,从开源世界公共软件池中汲取力量,并作为产销合一者产出更好的开源软件。所以,开源模式中,软件是不卖钱的,卖的本质上是专家服务。
买 RHEL,EDB 名义上买的是 “操作系统”,“数据库”,本质上买的是专家服务,更具体的说就是答疑咨询与人天。用开源数据库的成本,核心是人的成本。开源数据库卖多少钱,取决于专家应该卖多少钱。有人觉得用开源就一分钱不用花了,这就纯属YY —— 能把开源数据库用到商业数据库水平的专家可真不便宜。
专家的定价,取决于专家的质量水平,供需关系。有一种简单可靠的锚定方法就是,对标 Oracle / SQL Server 那个每年加收许可 20% 的“支持服务”,这一部分实际上就是市场对专家服务的定价。我们可以看到像 EDB,Fujitsu 这样的公司就是按照这个思路来定价的。
例如,富士通卖 PG 服务支持的价格是 3200 $ / 物理Core,也就是每 vCPU 1.1 万¥/年。EDB 的价格没有公开,但据我所知的单价比富士通贵一倍,也就是差不多与 Oracle 每年 20% 那个支持费用接近。
PostgreSQL: y = a * vCPU , a = 11K ~ 22K
当然,专家可以从专业服务公司租,也可以直接去市场上租 —— 只要你的规模足够大,直接买断专家总是更合算的 (例如弄两个年薪百万的专家 = 2000K / 20K = 100 vCPU )你可以直接把数据库的成本模型从与 vCPU 的线性增长,转变为对数增长或固定常数 —— 前提是你真的能找到,人家也愿意。但即使如此,绝大部分中小公司也是不愿意或者没有能力支付这个最小规模的启动成本的,于是就有了云数据库。
云数据库卖多少钱?
无论是雇佣专家,还是购买专业数据库服务,都有一个启动成本与最小规模 —— 在时间上是一年起步,在空间上一般好几个核起卖,起步成本在十几万到几十万这个数量级。云数据库用于解决这个问题:它用批发的方式采购专家,然后揉碎进行零售,极好地解决了中小型企业起步的需求。
云数据库的计费模式与商业数据库/开源服务支持一脉相承,采用与 CPU 规模绑定的做法。即定价模型为:
y = a * vCPU
这个 a
,就是云数据库的核月单价。国际上,云数据库的单价,在 150 $ ~ 250 $ / vCPU·月 上下浮动,再加上存储部分的钱,也就是这个 a 大概会在 13K ~ 21K 这个范围内。基本与开源数据库公司提供的服务支持在一个范围。考虑到 AWS 这些云厂商还提供了硬件,起步规模更小,也更省事,不挑客户,在中小规模的竞争力还是非常显著的。
当然,国内云厂商比较卷,专家的平均水平也比国际同行拉垮不少,所以云数据库也卖的便宜些。例如:国内,以阿里云为例,RDS / PolarDB 的单价在 250 ~ 400 ¥ / vCPU·月 上下浮动。那么这里的 a 就是 3K ~ 5K。
总体来说,云数据库的定价依然锚定的是专家服务费用,更具体的说就是盯着传统企业级市场数据库专业服务的定价设计的。只不过针对 SMB 极小微场景会有一些优待 —— 因为凑补的超卖实例反正也没啥成本。像 Neon / Supabase 干脆就对这种场景直接免费了。
当然,一核数据库一年一两万(PolarDB 大约三五千)听上去不贵,但考虑到现在一个机柜就能塞下小几千核的服务器,对于那些有着很大规模数据库的用户来讲,每年几千万与上亿的成本就很骇人了 —— 毕竟单从健全直觉来讲,这样的事找两个数据库专家用硬件自建,也就千万出头。
例如最近由雷锋网爆料的《独家丨米哈游或将大幅「下云」,对某云大厂预算减半》就讲到一个鲜活的案例 —— PolarDB 的标杆案例米哈游在数据库上暴砍近四亿预算……
换个角度看,云上年消费过百万的公司,就应该开始仔细算帐了;过千万就该全面下云了;云上年消费上亿的公司如果还不自建,那真的就是在头上顶着一个 “人傻钱多” 的肉猪 Flag,招杀猪盘。米哈游下云,亡羊补牢为时未晚,至于这种规模还要往云上搬的小红书,那就祝他们好运了。
数据库自建要多少钱?
无论是商业数据库的订阅支持,还是开源数据库专业服务,还是云数据库,都不难看出这里的核心生产要素是 “专家”,而不是 “软件” 和 ”硬件“。
从成本上看,当下的综合硬件单位成本约 60 ~ 300 ¥/ vCPU · 年,在数据库服务中的成本可以说微不足道。因为开源数据库的出现,绝大多数商业数据库产品的许可证价值直接归零;很多国产数据库也就是 PG 换皮,没有什么研发成本;所以核心成本就是专家和销售的成本。
因此在2024年的当下,靠 许可 赚钱的数据库,要么是垄断/供应商锁定的保护费,要么是认知不对称的杀猪钱。要么本质上还是挂羊头卖狗肉,把专家费摊丁入亩抹入到许可费用中。
数据库公司,真正出售的不是软件,而是专家的服务支持。从上面的例子不难看出,专家的服务支持价格与数据库规模绑定,国际市场公允售价为 1 ~ 2 万人民币 / vCPU 每年。无论从数据库服务公司采购,还是从云厂商采购,都差不多是这个范围。
当然,如果你的数据库规模足够大,vCPU 核数足够多,那么最经济的做法就是直接雇佣数据库专家,而不是从别处租赁。比如,如果你有 100 vCPU 规模的数据库,那么就对应着 100 ~ 200 万的专家维护预算,在当下招聘一个足够好的数据库专家是完全可行的。如果你有 10000 vCPU,也许需要两到三个数据库专家,但他们的工资相比上亿的采购费用可是有数量级的差距 …
当然,理想很美好,现实很骨感。实事求是的讲,数据库专家也并不是那么好招的。别说小厂小甲方了,大云厂商巨头也照样找不到留不住这些人。
例如,Apple 当年在上海招聘一个 PostgreSQL 专家的坑位,一直找了两年没有找到合适的人选。其实逻辑也很简单,真正牛逼的专家干嘛不自己开个公司赚上面的 1~2 万 ¥/vCPU 每年的钱,而跑过来给雇主打工呢?
最典型的例子就是 PolarDB 的创始人曹伟,花名鸣嵩,前年就从阿里云跑出来单干,搞了个数据库管控公司 Kubeblocks。此外还有斗佛叶正盛的九章科技等。根据投中网报道:一位早期投资人告诉我们,最近一两年,她接触的从阿里出来做数据库创业者已经达到两位数了。
回到二十块好兄弟
回过头来看我们单价二十刀乐好兄弟 PolarDB ,按照市场定价,每 vCPU 每年费用在 1 ~ 2 万是体面数据库服务的公允价。咱们就考虑比较低端的场景,(挺好玩的事:很多云厂商把 4c8g 的规格标记为“入门企业级服务器”),弄个 4c8thread 低端服务器,那一个节点的报价也应该在10万块左右(前提是含对应的专家支持服务)。130 块钱那纯属倒贴,还不够销售收钱的打车钱呢。
很明显这不是一个符合市场规律的报价,而是想要倒贴钱做标杆案例。毕竟是人民银行,做成了之后销售出去吹牛腰杆都要硬几分。不过价格战可是双刃剑,你报 130 块单价搅乱市场,自然会有各种同行报1块钱倒贴更多,拼老命赔钱也要给你拉下马 —— 绝对不能让你做成这种标杆案例。
这里再解释一下,PolarDB 并不是单一数据库,而是一个数据库品牌。品牌的意思就是这个篮子里有好几个数据库,有 PolarDB for MySQL(拳头产品),PolarDB for PostgreSQL(开源),以及 PolarDB for Oracle (for PG 改),blahblah。这里打人行单子打 PolarDB v2.0 其实是 PolarDB for Oracle ,这是一个在 PolarDB for PG 的基础上修改的 Oracle 兼容版。
当然,PolarDB for MySQL 在云上卖的不错。作为国内早期大规模的 MySQL 用户,阿里在 MySQL 上有很深厚的功力,向输出了许多 MySQL 专家, PolarDB for MySQL 其实是也是 PolarDB 里的拳头产品。作为标杆案例的米哈游,用的也是这个。但线下私有化部署和国产信创的 for PostgreSQL/Oracle 还没有 for MySQL 像米哈游这样的标杆。
但现在,下云开始成为一种潮流,根据雷锋网报道,PolarDB MySQL 标杆大客米哈游一口气砍了四亿(每年)数据库预算 …… 四亿是什么概念呢?虽然阿里云每年营收千亿,但过去一年利润也就是不到九十个亿。数据库的毛利率都是 50% 起步,70% 也不让人惊奇,这一刀下去,确实太伤了。
那么,云上遭遇滑铁卢,自然就要开辟第二战场,打造第二增长曲线,做线下私有化部署。虽然阿里云嘴上喊着公有云优先,但 PolarDB 身体还是很诚实的去搞了那个信安国测,弄了个自主可控国产数据库的身份。看着庶长子 OceanBase 四处收割眼热,教练我也要打篮球!我也要做国产数据库!
数据库老司机评论
作为数据库老司机,我认为庶长子 OceanBase 的技术路线双重押错宝了,首先押分布式,这在当代硬件条件下已经成了一个伪需求;其次押 MySQL 生态,天花板已经锁死了。而阿里数据库嫡子 PolarDB (PG/Oracle)认清现状,在技术路线上拨乱反正,重新回到 RAC 集中式,PostgreSQL 生态的道路上来,很明显在产品/技术路线上前途要光明的多。
当然,有前途没前途都是相对其他“国产数据库“来说的,基于开源数据库主干提供专家服务,发行版,扩展,等增量价值才是正路,土法自研是封闭僵化的老路,魔改开源是改旗易帜的邪路。PolarDB for PostgreSQL 总体来说对 PostgreSQL 的魔改程度不大,基本可以复用 PG 生态的扩展,工具与组件,这一点我觉得是非常明智的。
所以,在我们的开源开箱即用 PostgreSQL 发行版 Pigsty v3.0 中,我们也提供了针对开源的 PolarDB for PostgreSQL 的支持,意思是你可以直接用 PolarDB for PostgreSQL 替换掉原生的 PG 内核,拥有开箱即用的监控系统,高可用,备份恢复,IaC,连接池,负载均衡,故障自愈能力,把一个 RPM 包变成一套本地运行的企业级 RDS 服务。至于 PolarDB for Oracle,因为是基于 for PG 的版本做的,所以也支持,但为了支持国产数据库事业,这个部分就不开源免费了,关于 Pigsty 与 PolarDB v2 打包的国产化本地 RDS 方案,欢迎感兴趣的朋友联系我。
Redis不开源是“开源”之耻,更是公有云之耻
最近 Redis 修改其协议引发了争议:它从 7.4 起使用 RSALv2 与 SSPLv1,不再满足 OSI 关于 “开源软件” 的定义。但不要搞错:Redis “不开源” 不是 Redis 的耻辱,而是“开源/OSI”的耻辱 —— 它反映出开源组织/理念的过气。
当下软件自由的头号敌人是公有云服务。“开源” 与 “闭源” 也不再是软件行业的核心矛盾,斗争的焦点变为 “云上服务” 与 “本地优先”。公有云厂商搭着开源软件的便车白嫖社区的成果,这注定会引发社区的强烈反弹。
在抵御云厂商白嫖的实践中,修改协议是最常见的做法:但AGPLv3 过于严格容易敌我皆伤,SSPL 因为明确表达这种敌我歧视,不被算作开源。业界需要一种新的歧视性软件许可证协议,来达到名正言顺区分敌我的效果。
真正重要的事情一直都是软件自由,而“开源”只是实现软件自由的一种手段。而如果“开源”的理念无法适应新阶段矛盾斗争的需求,甚至会妨碍软件自由,它一样会过气,并不再重要,并最终被新的理念与实践所替代。
修改协议的开源软件
“我想直率地说:多年来,我们就像个傻子一样,他们拿着我们开发的东西大赚了一笔”。
Redis Labs 首席执行官 Ofer Bengal
Redis 在过去几年中一直都是开发者最喜爱的数据库系统(在去年被 PostgreSQL 超过),采用了非常友善的 BSD-3 Clause 协议,并被广泛应用在许多地方。然而,几乎所有的公有云上都可以看到云 Redis 数据库服务,云厂商靠它赚的钵满盆翻,而支付研发成本的 Redis 公司和开源社区贡献者被搁在一边。这种不公平的生产关系,注定会招致猛烈的反弹。
Redis 切换为更为严格的 SSPL 协议的核心原因,用 Redis Labs CEO 的话讲就是:“多年来,我们就像个傻子一样,他们拿着我们开发的东西大赚了一笔”。“他们”是谁? —— 公有云。切换 SSPL 的目的是,试图通过法律工具阻止这些云厂商白嫖吸血开源,成为体面的社区参与者,将软件的管理、监控、托管等方面的代码开源回馈社区。
不幸的是,你可以强迫一家公司提供他们的 GPL/SSPL 衍生软件项目的源码,但你不能强迫他们成为开源社区的好公民。公有云对于这样的协议往往也嗤之以鼻,大多数云厂商只是简单拒绝使用AGPL许可的软件:要么使用一个采用更宽松许可的替代实现版本,要么自己重新实现必要的功能,或者直接购买一个没有版权限制的商业许可。
当 Redis 宣布更改协议后,马上就有 AWS 员工跳出来 Fork Redis —— “Redis 不开源了,我们的分叉才是真开源!” 然后 AWS CTO 出来叫好,并假惺惺的说:这是我们员工的个人行为 —— 堪称是现实版杀人诛心。
图:AWS CTO 转评员工 Fork Redis
被这样搞过的并非只有 Redis 一家。发明 SSPL 的 MongoDB 也是这个样子 —— 当 2018 年 MongoDB 切换至 SSPL 时,AWS 就搞了一个所谓 “API兼容“ 的 DocumentDB 来恶心它。ElasticSearch 修改协议后,AWS 就推出了 OpenSearch 作为替代。头部 NoSQL 数据库都已经切换到了 SSPL,而 AWS 也都搞出了相应的“开源替代”。
因为引入了额外的限制与所谓的“歧视”条款,OSI 并没有将 SSPL 认定为开源协议。因此使用 SSPL 的举措被解读为 —— “Redis 不再开源”,而云厂商的各种 Fork 是“开源”的。从法律工具的角度来说,这是成立的。但从朴素道德情感出发,这样的说法对于 Redis 来说是极其不公正地抹黑与羞辱。
正如罗翔老师所说:法律工具的判断永远不能超越社区成员朴素的道德情感。如果协和与华西不是三甲,那么丢脸的不是这些医院,而是三甲这个标准。如果年度游戏不是巫师3,荒野之息,博德之门,那么丢脸的不是这些厂商,而是评级机构。如果 Redis 不再算“开源”,真正应该感到耻辱的是OSI,与开源这个理念。
越来越多的知名开源软件,都开始切换到敌视针对云厂商白嫖的许可证协议上来。不仅仅是 Redis,MongoDB,与 ElasticSearch 。MinIO 与 Grafana 分别在 2020,2021年从 Apache v2 协议切换到了 AGPLv3 协议。HashipCrop 的各种组件,MariaDB MaxScale, Percona MongoDB 也都使用了风格类似的 BSL 协议。
一些老牌的开源项目例如 PostgreSQL ,正如PG核心组成员 Jonathan 所说,三十年的声誉历史沉淀让它们已经在事实上无法变更开源协议 了。但我们可以看到,许多新强力的 PostgreSQL 扩展插件开始使用 AGPLv3 作为默认的开源协议,而不是以前默认使用的 BSD-like / PostgreSQL 友善协议。例如分布式扩展 Citus,列存扩展 Hydra,ES全文检索替代扩展 BM25,OLAP 加速组件 PG Analytics …… 等等等等。
包括我们自己的 PostgreSQL 发行版 Pigsty,也在 2.0 的时候由 Apache 协议切换到了 AGPLv3 协议,背后的动机都是相似的 —— 针对软件自由的最大敌人 —— 云厂商进行反击。我们改变不了存量,但对于增量功能,是可以做出有效的回击与改变的。
在抵御云厂商白嫖的实践中,修改协议是最常见的做法:AGPLv3 是一种比较主流的实践,更激进的 SSPL 因为明确表达这种敌我歧视,不被算作开源。使用双协议进行明确的边界区分,也开始成为一种主流的开源商业化实践。但重要的是:业界需要一种新的歧视性软件许可证协议,达到名正言顺辨识敌我,区别对待的效果 —— 来解决软件自由在当下面临的最大挑战 —— 云服务。
软件行业的范式转移
软件吞噬世界,开源吞噬软件,云吞噬开源。
在当下,软件自由的头号敌人是云计算租赁服务。“开源” 与 “闭源” 也不再是软件行业的核心矛盾,斗争的焦点变为 “云上服务” 与 “本地优先”。要理解这一点,我们要回顾一下软件行业的几次主要范式转移,以数据库为例:
最初,软件吞噬世界,以 Oracle 为代表的商业数据库,用软件取代了人工簿记,用于数据分析与事务处理,极大地提高了效率。不过 Oracle 这样的商业数据库非常昂贵,vCPU·月光是软件授权费用就能破万,往往只有金融行业,大型机构才用得起,即使像如淘宝这样的互联网巨头,上了量后也不得不”去O“。
接着,开源吞噬软件,像 PostgreSQL 和 MySQL 这样”开源免费“的数据库应运而生。软件开源本身是免费的,每核每月只需要几十块钱的硬件成本。大多数场景下,如果能找到一两个数据库专家帮企业用好开源数据库,那可是要比傻乎乎地给 Oracle 送钱要实惠太多了。
然后,云吞噬开源。公有云软件,是互联网大厂将自己使用开源软件的能力产品化对外输出的结果。公有云厂商把开源数据库内核套上壳,包上管控软件跑在托管硬件上,并建设共享开源专家池提供咨询与支持,便成了云数据库服务 (RDS)。20 ¥/核·月的硬件资源通过包装,变为了 300 ~ 1300 ¥/核·月的天价 RDS 服务。
曾经,软件自由的最大敌人是商业闭源软件,以微软,甲骨文为代表 —— 许多开发者依然对拥抱开源之前的微软名声有着深刻印象,甚至可以说整个自由软件运动正是源于 1990 年代的反微软情绪。但是,自由软件与开源软件的概念已经彻底改变了软件世界:商业软件公司耗费了海量资金与这个想法斗争了几十年。最终还是难以抵挡开源软件的崛起 —— 开源软件打破了商业软件的垄断,让软件这种IT业的核心生产资料变为全世界开发者公有,按需分配。开发者各尽所能,人人为我,我为人人,这直接催生了互联网的黄金繁荣时代。
开源并不是一种商业模式,甚至是一种强烈违反商业化逻辑的模式。然而,任何可持续发展的模式都需要获取资源以支付成本,开源也不例外。开源真正的模式是 —— 通过免费的软件创造高薪技术专家岗位。分散在不同企业组织中的开源专家,产消合一者 (Pro-sumer),是(纯血)开源软件社区的核心力量 —— 免费的开源软件吸引用户,用户需求产生开源专家岗位,开源专家共创出更好的开源软件。开源专家作为组织的代理人,从开源社区,集体智慧成果中汲取力量。组织享受到了开源软件的好处(软件自由,无商业软件授权费),而分散的雇主可以轻松兜住住这些专家的薪资成本。
然而公有云,特别是云软件的出现破坏了这种生态循环 —— 几个云巨头尝试垄断开源专家供给,重新尝试在 用好开源软件(服务)这个维度上,实现商业软件没能实现的垄断。 云厂商编写了开源软件的管控软件,组建了专家池,通过提供维护攫取了软件生命周期中的绝大部分价值,并通过搭便车的行为将最大的成本 —— 产研交由整个开源社区承担。而 真正有价值的管控/监控代码却从来不回馈开源社区。而更大的伤害在于 —— 公有云就像头部带货主播消灭大量本地便利店一样,摧毁了大量的开源就业岗位,掐断了开源社区的人才流动与供给。
计算自由的头号敌人
在 2024 年,软件自由的真正敌人,是云服务软件!
开源软件带来了巨大的行业变革,可以说,互联网的历史就是开源软件的历史。互联网公司是依托开源软件繁荣起来的,而公有云是从头部互联网公司孵化出来的。公有云的历史,就是一部屠龙勇者变为新恶龙的故事。
云刚出现的时候,它也曾经是一位依托开源 挑战传统 IT 市场恶龙的勇者,挥舞着大棒砸烂“企业级”杀猪盘。他们关注的是硬件 / IaaS层 :存储、带宽、算力、服务器。云厂商的初心故事是:让计算和存储资源像水电一样,自己扮演基础设施的提供者的角色。这是一个很有吸引力的愿景:公有云厂商可以通过规模效应,压低硬件成本并均摊人力成本;理想情况下,在给自己留下足够利润的前提下,还可以向公众提供比 IDC 价格更有优势,更有弹性的存储算力(实际上也并不便宜!)。
然而随着时间的推移,这位曾经的屠龙英雄逐渐变成了他曾经发誓打败的恶龙 —— 一个新的“杀猪盘”,对用户征收高昂无专家税与“保护费”。这对应着云软件( PaaS / SaaS ),它与云硬件有着迥然不同的商业逻辑:云硬件靠的是规模效应,优化整体效率赚取资源池化超卖的钱,算是一种效率进步。而云软件则是靠共享专家,提供运维外包来收取服务费。公有云上大量的软件,本质是吸血白嫖开源社区搭便车,抢了分散在各个企业中开源工程师的饭碗,依靠的是信息不对称、专家垄断、用户锁定收取天价服务费,是一种价值的攫取转移,对原有的生态模式的破坏。
不幸的是,出于混淆视线的目的,云软件与云硬件都使用了“云”这个名字。因而在云的故事中,同时混掺着将算力普及到千家万户的理想主义光辉,与达成垄断攫取不义利润的贪婪。
在 2024 年,软件自由的真正敌人,是云服务软件!
云软件,即主要在供应商的服务器上运行的软件,而你的所有数据也存储在这些服务器上。以云数据库为代表的 PaaS ,以及各类只能通过租赁提供服务的 SaaS 都属于此类。这些“云软件”也许有一个客户端组件(手机App,网页控制台,跑在你浏览器中的 JavaScript),但它们只能与供应商的服务端共同工作。而云软件存在很多问题:
- 如果云软件供应商倒闭或停产,您的云软件就歇菜了,而你用这些软件创造的文档与数据就被锁死了。例如,很多初创公司的 SaaS 服务会被大公司收购,而大公司没有兴趣继续维护这些产品。
- 云服务可能在没有任何警告和追索手段的情况下突然暂停您的服务(例如 Parler )。您可能在完全无辜的情况下,被自动化系统判定为违反服务条款:其他人可能入侵了你的账户,并在你不知情的情况下使用它来发送恶意软件或钓鱼邮件,触发违背服务条款。因而,你可能会突然发现自己用各种云文档或其它App创建的文档全部都被永久锁死无法访问。
- 运行在你自己的电脑上的软件,即使软件供应商破产倒闭,它也可以继续跑着,想跑多久跑多久。相比之下,如果云软件被关闭,你根本没有保存的能力,因为你从来就没有服务端软件的副本,无论是源代码还是编译后的形式。
- 云软件极大加剧了软件的定制与扩展难度,在你自己的电脑上运行的闭源软件,至少有人可以对它的数据格式进行逆向工程,这样你至少有个使用其他替代软件的PlanB。而云软件的数据只存储在云端而不是本地,用户甚至连这一点都做不到了。
如果所有软件都是免费和开源的,这些问题就都自动解决了。然而,开源和免费实际上并不是解决云软件问题的必要条件;即使是收钱的或者闭源的软件,也可以避免上述问题:只要它运行在你自己的电脑、服务器、机房上,而不是供应商的云服务器上就可以。拥有源代码会让事情更容易,但这并不是不关键,最重要的还是要有一份软件的本地副本。
在当今,云软件,而不是闭源软件或商业软件,成为了软件自由的头号威胁。云软件供应商可以在您无法审计,无法取证,无法追索的情况下访问您的数据,或突然心血来潮随心所欲地锁定你的所有数据,这种可能性的潜在危害,要比无法查看和修改软件源码的危害大得多。与此同时,也有不少公有云厂商渗透进入开源社区,并将“开源”视作一种获客营销包装、或形成垄断标准的手段,作为吸引用户的钓饵,而不是真正追求“软件自由”的目的。
”开源“ 与 ”闭源“ 已经不再是软件行业中最核心的矛盾,斗争的焦点变为 “云” 与 “本地优先”。
自由世界如何应对挑战?
重要的事情,一直都是软件自由
有力,就会有反作用力,云软件的崛起会引发新的制衡力量。面对云服务的挑战,已经有许多软件组织/公司做出了反应,包括但不限于:使用歧视性开源协议,法律工具与集体行动,抢夺云计算的定义权。
修改开源许可证
软件社区应对云服务挑战的最常见反应是修改许可证,如 Grafana,MinIO,Pigsty 那样修改为 AGPLv3,或者像 Redis,MongoDB,ElasticSearch 那样修改为 SSPL,或者使用双协议 / BSL 的方式。大的方向是一致的 —— 重新划定社区共同体边界,将竞争者、与敌人直接排除在社区之外。
友善、自由的互联网/软件世界离我们越来越远 —— 大爱无疆,一视同仁,始终无私奉献的圣母精神固然值得敬佩,但真正能靠自己力量活下来的,是爱憎分明,以德报德,以直报怨的勇者。这里的核心问题在于 “歧视” / 区别对待 —— 对待同志要像春天般的温暖,对待敌人要像严冬一样残酷。
业界需要一种实践来做到这一点。AGPL,SSPL,BSL 这样的协议就是一种尝试 —— 这些协议通常并不影响终端用户使用这些软件;也不影响普通的服务提供商在遵循开源义务的前提下提供支持与咨询服务;而是专门针对公有云厂商设计的 —— 管控软件 作为公有云厂商摇钱树,在事实上是难以选择开源的,因此公有云厂商被歧视性地排挤出软件社区之外。
使用 Copyleft 协议族可以将公有云厂商排除在社区之外,从而保护软件自由。然而这些协议也容易出现伤敌一千,自损一百的情况。 在更为严格的许可要求下,一部分软件自由也受到了不必要的连带损失,例如:Copyleft 协议族也与其他广泛使用的许可证不兼容,这使得在同一个项目中使用某些库的组合变得更为困难。因此业界需要更好的实践来真正落实好这一点。
例如,我们的自由 PostgreSQL 发行版 Pigsty 使用了 AGPLv3 协议,但我们添加了对普通用户的 补充豁免条款 —— 我们只保留对公有云供应商,与换皮套壳魔改同行进行违规追索的权利,对于普通终端用户来说实际执行的是 Apache 2.0 等效条款 —— 采购我们服务订阅的客户也可以得到书面承诺:不就违反 AGPLv3 的协议进行任何追索 —— 从某种意义上来说,这也是一种 “双协议” 实践。
法律工具与集体行动
Copyleft软件许可证是一种法律工具,它试图迫使更多的软件供应商公开其源码。但是对于促进软件自由而言,Martin Keppmann 相信更有前景的法律工具是政府监管。例如,GDPR提出了数据可移植权,这意味着用户必须可以能将他们的数据从一个服务转移到其它的服务中。另一条有希望的途径是,推动 公共部门的采购倾向于开源、本地优先的软件,而不是闭源的云软件。这为企业开发和维护高质量的开源软件创造了积极的激励机制,而版权条款却没有这样做。
我认为,有效的改变来自于对大问题的集体行动,而不仅仅来自于一些开源项目选择一种许可证而不是另一种。公有云反叛军联盟应该团结一切可以团结的有生力量 —— 开源平替软件社群,开发者与用户;服务器与硬件厂商,坚守 IaaS 阵地的资源云,运营商云,IDC 与 IDC 2.0,甚至是公有云厂商的 IaaS 部门。采取一切法律框架内允许的行动去推进这一点。
一种有效的对抗措施是为整个云计算技术栈提供开源替代品,例如在《云计算反叛军》中就提到 —— 云计算世界需要一个能代表开源价值观的替代解决方案。开源软件社区可以与云厂商比拼生产力 —— 组建一个反叛军同盟采取集体行动。针对公有云厂商提供服务所必不可少的管控软件,逐一研发开源替代。
在云软件没有出现开源/本地优先的替代品前,公有云厂商可以大肆收割,攫取垄断利润。而一旦更好用,更简易,成本低得多的开源替代品出现,好日子便将到达终点。例如,Kubernetes / SealOS / OpenStack / KVM / Proxmox,可以理解为云厂商 EC2 / ECS / VPS 管控软件的开源替代;MinIO / Ceph 旨在作为为云厂商 S3 / OSS 管控软件的开源替代;而 Pigsty / 各种数据库 Operator 就是 RDS 云数据库管控软件的开源替代。这些开源替代品将直接击碎公有云计算的核心技术壁垒 —— 管控软件,让云的能力民主化,直接普及到每一个用户手中。
抢夺云计算的定义权
公有云可以渗透到开源社区中兴风作浪,那么开源社区也可以反向渗透,抢夺云计算的定义权。例如,对于 Cloud Native 的不同解释就生动地体现了这一点。云厂商将 Native 解释 “原生”:“原生诞生在公有云环境里的软件” 以混淆视听;但究其目的与效果而言,Native 真正的含义应为 “本地”,即与 Cloud 相对应的 “Local” —— 本地云 / 私有云 / 专有云 / 原生云 / 主权云,叫什么不重要,重要的是它运行在用户想运行的任何地方(包括云服务器),而不是仅仅是只能从公有云租赁!
这一理念,用一个单独的术语,可以概括为 “本地优先”,它与云服务针锋相对。“本地优先” 与 “云” 的对立体现为多种不同的形式:有时候是 “Native Cloud” vs “Cloud Native”,有时候叫体现为 “私有云” vs “公有云”,大部分时候与 ”开源“ vs “闭源”重叠,某种意义上也牵扯着 “自主可控” vs “仰人鼻息”。
本地优先的软件在您自己的硬件上运行,并使用本地数据存储,但也不排斥运行在云 IaaS 上,同时也保留云软件的优点,比如实时协作,简化运维,跨设备同步,资源调度,灵活伸缩等等。开源的本地优先的软件当然非常好,但这并不是必须的,本地优先软件90%的优点同样适用于闭源的软件。同理,免费的软件当然好,但本地优先的软件也不排斥商业化与收费服务。
理直气壮地争取资源
最后,不得不说的一点,就是开源商业化,收钱的问题。开源软件社区应该理直气壮地赚钱与筹款 —— 自由不是免费的! Freedom is not free 早已经是老生常谈。然而,相当一部分开源贡献者与开源用户都对开源软件有着不切实际的期待与错觉。
一些用户误以为他们与维护者的关系是商业关系,因此期望获得商业供应商的客户服务标准;而一些开源贡献者也期待开源用户给予金钱、声望、场景上的互惠与回馈贡献。一方认为他们应得的比另一方认为的要多,这种不明确的结果就会走向怨恨。
开源不等于免费,尽管大部分开源软件都提供了让用户免费使用的条件,但免费的开源软件是一种没有条件的礼物。作为收礼人,用户只有选择收或不收的权利;作为送礼人,期待得到特定回报是愚蠢的。作为开源贡献者,给别人戴上氧气面罩前,请务必先戴好自己的氧气面罩。如果某个开源企业自己都无法养活自己,那么选择用爱发电,四处慷慨送礼就是不明智的。
因此,全职的开源软件的开发者与公司,必须审慎思考自己的商业模式 —— 想让项目与组织持续发展,资源是必不可少的。无论是做专门的企业版,提供服务支持与订阅,设置双协议,去拉赞助化缘卖周边,或者干脆像 Redis 一样使用所谓 “不开源” 的协议,这都无可厚非,应当是光明正大,且理直气壮的。实际上,因为开源软件为用户提供了额外的“软件自由” —— 因此在质量相同的前提下,收取比云租赁软件更高的费用也是完全合乎道德情理的!
博弈均衡会走向哪里
云计算的故事与电力的推广过程如出一辙,让我们把目光回退至上个世纪初,从电力的推广普及垄断监管中汲取历史经验 —— 供电也许会走向垄断、集中、国有化,但你管不住电器。如果云硬件(算力)类似于电力,那么云软件便是电器。生活在现代的我们难以想象:洗衣机,冰箱,热水器,电脑,竟然还要跑到电站边的机房去用,我们也很难想象,居民要由自己的发电机而不是公共发电厂来供电。
因此从长期来看,公有云厂商大概也会有这么一天:在云硬件上通过类似于电力行业,通过垄断并购与兼并形成“规模效应”,利用“峰谷电”,“弹性定价”等各种方式优化整体资源利用率,在相互斗兽竞争中将算力成本不断压低至新的底线,实现“家家有电用”。当然,最后也少不了政府监管介入,公私合营收归国有,成为如同国家电网与电信运营商类似的存在,最终实现 IaaS 层的存储带宽算力的垄断。
而与之对应,制造灯泡、空调、洗衣机这类电器的职能会从电力公司中剥离,百花齐放。云厂商的 PaaS / SaaS 在被更好,更优质,更便宜的开源替代冲击下逐渐萎缩,或回归到足够低廉的价格水平。
正如当年开源运动的死对头微软,现在也选择拥抱开源。公有云厂商肯定也会有这一天,与自由软件世界达成和解,心平气和地接受基础设施供应商的角色定位,为大家提供水与电一般的存算资源。而云软件终将会回归正常毛利,希望那一天人们能记得,这不是因为云厂商大发慈悲,而是有人带来过开源平替。
MySQL正确性竟如此垃圾?
MySQL 曾经是世界上最流行的开源关系型数据库,然而流行并不意味着先进,流行的东西也会出大问题。JEPSEN 对 MySQL 的隔离等级评测捅穿了这层窗户纸 —— 在正确性这个体面数据库产品必须的基本属性上,MySQL 的表现一塌糊涂。
MySQL 文档声称实现了 可重复读/RR 隔离等级,但实际提供的正确性保证却弱得多。JEPSEN 在 Hermitage 的研究基础上进一步指出,MySQL 的 可重复读/RR 隔离等级实际上并不可重复读,甚至既不原子也不单调,连 单调原子视图/MAV 的基本水平都不满足。
此外,能“避免”这些异常的 MySQL 可串行化/SR 隔离等级难以生产实用,也非官方文档与社区认可的最佳实践;而且在AWS RDS默认配置下,MySQL SR 也没有真正达到“串行化”的要求;而李海翔教授的对 MySQL 一致性的分析进一步指出了 SR 的设计缺陷与问题。
综上, MySQL 的 ACID 存在缺陷,且与文档承诺不符 —— 这可能会导致严重的正确性问题。尽管可以通过显式加锁等方式规避此类问题,但用户确实应当充分意识到这里的利弊权衡与风险:在对正确性/一致性有要求的场景中选用 MySQL 时,请务必保持谨慎。
正确性为什么很重要?
可靠的系统需要应对各种错误,在数据系统的残酷现实中,更是很多事情都可能出错。要保证数据不丢不错,实现可靠的数据处理是一件工作量巨大且极易错漏的事情。而事务的出现解决了这个问题。事务是数据处理领域最伟大的抽象之一,也是关系型数据库引以为傲的金字招牌和尊严所在。
事务这个抽象让所有可能的结果被归结为两种情况:要么成功完事 COMMIT,要么失败了事 ROLLBACK,有了后悔药,程序员不用再担心处理数据时半路翻车,留下数据一致性被破坏的惨不忍睹的车祸现场。应用程序的错误处理变得简单多了,因为它不用再担心部分失败的情况了。而它提供的保证,用四个单词的缩写,被概括为 ACID。
事务的原子性/A让你在提交前能随时中止事务并丢弃所有写入,相应地,事务的持久性/D则承诺一旦事务成功提交,即使发生硬件故障或数据库崩溃,写入的任何数据也不会丢失。事务的隔离性/I确保每个事务可以假装它是唯一在整个数据库上运行的事务 —— 数据库会确保当多个事务被提交时,结果与它们一个接一个地串行运行是一样的,尽管实际上它们可能是并发运行的。而原子性与隔离性则服务于 一致性/Consistency —— 也就是应用的正确性/Correctness —— ACID 中的C是应用的属性而非事务本身的属性,属于用来凑缩写的。
然而在工程实践中,完整的隔离性/I是很少见的 —— 用户很少会使用所谓的 “可串行化/SR” 隔离等级,因为它有可观的性能损失。一些流行的数据库如 Oracle 甚至没有实现它 —— 在 Oracle 中有一个名为 “可串行化” 的隔离级别,但实际上它实现了一种叫做 快照隔离(snapshot isolation) 的功能,这是一种比可串行化更弱的保证。
RDBMS 允许使用不同的隔离级别,供用户在性能与正确性之间进行权衡。ANSI SQL92 用三种并发异常(Anomaly),划分出四种不同的隔离级别,将这种利弊权衡进行了(糟糕的)标准化。:更弱的隔离级别“理论上”可以提供更好的性能,但也会出现更多种类的并发异常(Anomaly),这会影响应用的正确性。
为了确保正确性,用户可以使用额外的并发控制机制,例如显式加锁或 SELECT FOR UPDATE ,但这会引入额外的复杂度并影响系统的简单性。对于金融场景而言,正确性是极其重要的 —— 记账错漏,对账不平很可能会在现实世界中产生严重后果;然而对于糙猛快的互联网场景而言,错漏几条数据并非不可接受 —— 正确性的优先级通常会让位于性能。 这也为伴随互联网东风而流行的 MySQL 的正确性问题埋下了祸根。
Hermitage的结果怎么说?
在介绍 JEPSEN 的研究之前,我们先来回顾一下 Hermitage 项目。。这是互联网名著 《DDIA》 作者 Martin Kelppmann 在 2014 年发起的项目,旨在评测各种主流关系数据库的正确性。项目设计了一系列并发运行的事务场景,用于评定数据库标称隔离等级的实际水平。
从 Hermitage 的评测结果表格中不难看出,在主流数据库的隔离级别实现里有两处缺陷,用红圈标出:Oracle 的 可串行化/SR 因无法避免 G2 异常,而被认为实际上是 “快照隔离/SI”。
MySQL 的问题更为显著:因为默认使用的 可重复读/RR 隔离等级无法避免 PMP / G-Single 异常,Hermitage 将其实际等级定为 单调原子视图/MAV。
需要指出 ANSI SQL 92 隔离等级是一个糟糕简陋且广为诟病的标准,它只定义了三种异常现象并用它们区分出四个隔离等级 —— 但实际上的异常种类/隔离等级要多得多。著名的《A Critique of ANSI SQL Isolation Levels》论文对此提出了修正,并介绍了几种重要的新隔离等级,并给出了它们之间的强弱关系偏序图(图左)。
在新的模型下,许多数据库的 “读已提交/RC” 与 “可重复读/RR” 实际上是更为实用的 “单调原子视图/MAV” 和 “快照隔离/SI” 。但 MySQL 确实别具一格:在 Hermitage 的评测中,MySQL的 可重复读/RR 与 快照隔离/SI 相距甚远,也不满足 ANSI 92 可重复读/RR 的标准,实际水平为 **单调原子视图/MAV。**而 JEPSEN 的研究进一步指出,MySQL 可重复读/RR 实际上连 单调原子视图/MAV 都不满足,仅仅略强于 读已提交/RC 。
JEPSEN 又有什么新发现?
JEPSEN 是分布式系统领域最为权威的测试框架,他们最近发布了针对 MySQL 最新的 8.0.34 版本的研究与测评。建议读者直接阅读原文,以下是论文摘要:
MySQL 是流行的关系型数据库。我们重新审视了 Kleppmann (DDIA作者)在2014年发起的 Hermitage 项目结果,并确认了在当下 MySQL 的 可重复读/RR 隔离等级依然会出现 G2-item、G-single 和丢失更新异常。我们用事务一致性检查组件 —— Elle,发现了 MySQL 可重复读隔离等级也违反了内部一致性。更有甚者 —— 它违反了单调原子视图(MAV):即一个事务可以先观察到另一个事务的结果,再次尝试观察后却又无法复现同样的结果。作为彩蛋,我们还发现 AWS RDS 的 MySQL集群经常出现违反串行要求的异常。这项研究是独立进行的,没有报酬,并遵循 Jepsen研究伦理。
MySQL 8.0.34 的 RU,RC,SR 隔离等级符合 ANSI 标准的描述。且默认配置(RR,且innodb_flush_log_at_trx_commit = on
)下的 持久性/D 并没有问题。问题出在MySQL 默认的 可重复读/RR 隔离等级上:
- 不满足 ANSI SQL92 可重复读(G2,WriteSkew)
- 不满足快照隔离(G-single, ReadSkew, LostUpdate)
- 不满足游标稳定性(LostUpdate)
- 违反内部一致性(Hermitage 披露)
- 违反读单调性(JEPSEN新披露)
MySQL RR 下的事务观察到了违反内部一致性、单调性、原子性的现象。这使得其评级被进一步调整至一个仅略高于 RC 的未定隔离等级水平上。
在 JEPSEN 的测试中共披露了六项异常,其中在2014年已知的问题我们先跳过,这里重点关注 JEPSEN 的新发现的异常,下面是几个具体的例子。
隔离性问题:不可重复读
在这个测试用例(JEPSEN 2.3)中是用来一张简单的表 people
,id
作为主键,预填充一行数据。
CREATE TABLE people (
id int PRIMARY KEY,
name text not null,
gender text not null
);
INSERT INTO people (id, name, gender) VALUES (0, "moss", "enby");
随即并发运行一系列写事务 —— 每个事务先读这一行的 name
字段;然后更新 gender
字段,随即再次读取 name
字段。正确的可重复读意味着在这个事务中,两次对 name
的读取返回的结果应该是一致的。
SET TRANSACTION ISOLATION LEVEL REPEATABLE READ;
START TRANSACTION; -- 开启RR事务
SELECT name FROM people WHERE id = 0; -- 结果为 "pebble"
UPDATE people SET gender = "femme" WHERE id = 0; -- 随便更新点什么
SELECT name FROM people WHERE id = 0; -- 结果为 “moss”
COMMIT;
但是在测试结果中 ,9048个事务中的126个出现了内部一致性错误 —— 尽管是在 可重复读 隔离等级上运行的,但是实际读到的名字还是出现了变化。这样的行为与 MySQL 的隔离级别文档矛盾,该文档声称:“同一事务中的一致读取,会读取第一次读取建立的快照”。它与 MySQL 的一致性读文档相矛盾,该文档特别指出,“InnoDB 在事务的第一次读时分配一个时间点,并发事务的影响不应出现在后续的读取中”。
ANSI / Adya 可重复读实质是:一旦事务观察到某个值,它就可以指望该值在事务的其余部分保持稳定。MySQL 则相反:写入请求是邀请另一个事务潜入,并破坏用户刚刚读取的状态。这样的隔离设计与行为表现确实是难以置信地愚蠢。但这儿还有更离谱的事情 —— 比如单调性和原子性问题。
原子性问题:非单调视图
Kleppmann 在 Hermitage 中将 MySQL 可重复读评级为单调原子视图/MAV。根据 Bailis 等 的定义,单调原子视图确保一旦事务 T2 观察到事务T1 的任意结果,T2 即观察到 T1 的所有结果。
如果 MySQL 的 RR 只是在每次执行写入查询时重新获取一个快照,那么如果快照是单调的,它还是可以提供 MAV 等级的隔离保证 —— 而这正是 PostgreSQL 读已提交/RC 隔离级别的工作原理。
然而在常规的 MySQL 单节点部署中,情况并非如此:MySQL 在 RR 隔离等级时经常违反单调原子视图。JEPSEN(2.4)的这个例子用于说明这一点:这里有一张 mav
表,预填充两条记录(id=0,1
),value
字段初始值都是 0
。
CREATE TABLE mav (
id int PRIMARY KEY,
`value` int not null,
noop int not null
);
INSERT INTO mav (id, `value`, noop) VALUES (0, 0, 0);
INSERT INTO mav (id, `value`, noop) VALUES (1, 0, 0);
负载是读写混合事务:有写入事务会在同一个事务里去同时自增这两条记录的 value
字段;根据事务的原子性,其他事务在观察这两行记录时,value
的值应当是保持同步锁定增长的。
START TRANSACTION;
SELECT value FROM mav WHERE id = 0; --> 0 读取到了0
update mav SET noop = 73 WHERE id = 1; --> “邀请”新的快照进来
SELECT value FROM mav WHERE id = 1; --> 1 读取到了新值1,那么另一行的值应该也是1才对
SELECT value FROM mav WHERE id = 0; --> 0 结果读取到了旧值0
COMMIT;
然而在上面这个读取事务看来,它观察到了“中间状态”。读取事务首先读0号记录的 value
,然后将 1 号记录的 noop
设置为一个随机值(根据上面一个案例,就能看见其他事务的变更了),接着再依次读取 0/1 号记录的 value
值。结果出现这种情况:读取0号记录拿到了新值,读取1号记录时获取到了旧值,这意味着单调性和原子性都出现了严重缺陷。
MySQL 的一致性读取文档广泛讨论了快照,但这种行为看起来根本不像快照。快照系统通常提供数据库状态的一致的、时间点的视图。它们通常是原子性的:要么包含事务的所有结果,要么全都不包含。即使 MySQL 以某种方式从写入事务的中间状态拿到了非原子性快照,它也必须得在获取行1新值前看到行0的新值。然而情况并非如此:此读取事务观察到行1的变化,但却没有看到行0的变化结果,这算哪门子快照?
因此,MySQL 的可重复读/RR 隔离等级既不原子,也不单调。在这一点上它甚至比不上绝大多数数据库的 读已提交/RC,起码它们实质上还是原子且单调的 单调原子视图/MAV。
另外一个值得一提的问题是:MySQL 默认配置下的事务会出现违背原子性的现象。我已经在两年前的文章中抛出该问题供业界讨论,MySQL 社区的观点认为这是一个可以通过 sql_mode
进行配置的特性而非缺陷。
但这种说法无法改变这一事实:MySQL确实违反了最小意外原则,在默认配置下允许用户做出这种违背原子性的蠢事来。与之类似的还有 replica_preserve_commit_order
参数。
串行化问题:鸡肋且糟糕
可串行化/SR 可以阻止上面的并发异常出现吗?理论上可以,可串行化就是为这个目的而设计的。但令人深感不安的是,JEPSEN 在 AWS RDS 集群中观察到,MySQL 在 可串行化/SR 隔离等级下也出现了 “Fractured Read-Like” 异常,这是G2异常的一个例子。这种异常是被 RR 所禁止的,应该只会在 RC 或更低级别出现。
深入研究发现这一现象与 MySQL 的 replica_preserve_commit_order
参数有关:禁用此参数允许 MySQL 以正确性作为代价,在重放日志时提供更高的并行性。当此选项被禁用时,JEPSEN在本地集群的 SR 隔离级别中也观察到了类似的 G-Single 和 G2-Item 异常。
可串行化系统应该保证事务(看起来是)全序执行,不能在副本上保留这个顺序是一件非常糟糕的事情。因此这个参数过去(8.0.26及以下)默认是禁用的,而在 MySQL 8.0.27 (2021-10-19)中被修改为默认启用。但是 AWS RDS 集群的参数组仍然使用“OFF”的旧默认值,并且缺少相关的文档说明,所以才会出现这样的现象。
尽管这一异常行为可以通过启用该参数进行规避,但使用 Serializable 本身也并非 MySQL 官方/社区鼓励的行为。MySQL 社区中普遍的观点是:除非绝对必要,否则应避免使用 可串行化/SR 隔离等级;MySQL 文档声称:“SERIALIZABLE
执行比 REPEATABLE READ
更严格的规则,主要用于特殊情况,例如 XA 事务以及解决并发和死锁问题。”
无独有偶,专门研究数据库一致性的李海翔教授(前鹅厂T14)在其《第三代分布式数据库》系列文章中,也对包括 MySQL (InnoDB/8.0.20)在内的多种数据库的实际隔离等级进行了测评,并从另一个视角给出了下面这幅更为细化的 “《一致性八仙图》”。
在图中蓝/绿色代表正确用规则/回滚避免异常;黄色的A代表出现异常,黄色“A”越多,正确性问题就越多;红色的“D”指使用了影响性能的死锁检测来处理异常,红色D越多,性能问题就越严重;
不难看出,正确性最好的是 PostgreSQL SR 与基于其构建的 CockroachDB SR,其次是 Oracle SR;都主要是通过机制与规则避免并发异常;而 MySQL 的正确性水平令人不忍直视。
李海翔教授在专文《一无是处的MySQL》对此有过详细分析:尽管MySQL的 可串行化/SR 可以通过大面积使用死锁检测算法保证正确性,但这样处理并发异常,会严重影响数据库的性能与实用价值。
正确性与性能的利弊权衡
李海翔教授在《第三代分布式数据库:踢球时代》中抛出了一个问题:如何对系统的正确性与性能进行利弊权衡?
数据库圈有一些“习惯成自然”的怪圈,例如很多数据库的默认隔离等级都是 读已提交/RC,有很多人会说“数据库的隔离级别设置为 RC 足够了”!可是为什么?为什么要设置为 RC ?因为他们觉得 RC 级别数据库性能好。
可如下图所示,这里存在一个死循环:用户希望数据库性能更好,于是开发者把应用的隔离级别设置为RC。然而用户,特别是金融保险证券电信等行业的用户,又期望保证数据的正确性,于是开发者不得不在 SQL 语句中加入 SELECT FOR UPDATE
加锁以确保数据的正确性。而此举又会导致数据库系统性能下降严重。在TPC-C和YCSB场景下测试结果表明,用户主动加锁的方式会导致数据库系统性能下降严重,反而是强隔离级别下的性能损耗并没有那么严重。
使用弱隔离等级其实严重背离了“事务”这个抽象的初衷 —— 在较低隔离级别的重要数据库中编写可靠事务极其复杂,而与弱隔离等级相关的错误的数量和影响被广泛低估[13]。使用弱隔离级别本质上是把本应由数据库来保证的正确性 & 性能责任踢给了应用开发者。
惯于使用弱隔离等级这个问题的根,可能出在 Oracle 和 MySQL 上。例如,Oracle 从来没有提供真正的可串行化隔离等级(SR 实际上是 快照隔离/SI),直到今天亦然。因此他们必须将*“使用 RC 隔离等级”* 宣传为一件好事。Oracle 是过去最流行的数据之一,所以后来者也纷纷效仿。
而使用弱隔离等级性能更好的刻板印象可能源于 MySQL —— 大面积使用死锁检测(标红)实现的 SR 性能确实糟糕。但对于其他 DBMS 来说并非必然如此。例如,PostgreSQL 在 9.1 引入的 可串行化快照隔离(SSI) 算法可以在提供完整可串行化前提下,相比快照隔离/SI 并没有多少性能损失。
更进一步讲,摩尔定律加持下的硬件的性能进步与价格坍缩,让OLTP性能不再成为稀缺品 —— 在单台服务器就能跑起推特的当下,超配充裕的硬件性能实在用不了几个钱。而比起数据错漏造成的潜在损失与心智负担,担心可串行化隔离等级带来的性能损失确实是杞人忧天了。
时过境迁,软硬件的进步让 “默认可串行化隔离,优先确保100%正确性” 这件事切实可行起来。为些许性能而牺牲正确性这样的利弊权衡,即使对糙猛快的互联网场景也开始显得不合时宜了。新一代的分布式数据库诸如 CockroachDB 与 FoundationDB 都选择了默认使用 可串行化 隔离等级。
做正确的事很重要,而正确性是不应该拿来做利弊权衡的。在这一点上,开源关系型数据库两巨头 MySQL 和 PostgreSQL 在早期实现上就选择了两条截然相反的道路:MySQL 追求性能而牺牲正确性;而学院派的 PostgreSQL 追求正确性而牺牲了性能。在互联网风口上半场中,MySQL 因为性能优势占据先机乘风而起。但当性能不再是核心考量时,正确性就成为了 MySQL 的致命出血点。
解决性能问题有许多种办法,甚至坐等硬件性能指数增长也是一种切实可行的办法(如 Paypal);而正确性问题往往涉及到全局性的架构重构,解决起来绝非一夕之功。过去十年间,PostgreSQL守正出奇,在确保最佳正确性的前提下大步前进,很多场景的性能都反超了 MySQL;而在功能上更是籍由其扩展生态引入的向量、JSON,GIS,时序,全文检索等扩展特性全方位碾压 MySQL。
PostgreSQL 在 2023 年 StackOverflow 的全球开发者用户调研中,开发者使用率正式超过了 MySQL ,成为世界上最流行的数据库。而在正确性上一塌糊涂,且与高性能难以得兼的 MySQL ,确实应该好好思考一下自己的破局之路了。
参考
[1]
JEPSEN: https://jepsen.io/analyses/mysql-8.0.34
[2]
Hermitage: https://github.com/ept/hermitage
[4]
Jepsen研究伦理: https://jepsen.io/ethics
[5]
innodb_flush_log_at_trx_commit
: https://dev.mysql.com/doc/refman/8.0/en/innodb-parameters.html#sysvar_innodb_flush_log_at_trx_commit
[6]
隔离级别文档: https://dev.mysql.com/doc/refman/8.0/en/innodb-transaction-isolation-levels.html#isolevel_repeatable-read
[7]
一致性读文档: https://dev.mysql.com/doc/refman/8.0/en/innodb-consistent-read.html
[9]
单调原子视图/MAV: https://jepsen.io/consistency/models/monotonic-atomic-view
[10]
Highly Available Transactions: Virtues and Limitations,Bailis 等: https://amplab.cs.berkeley.edu/wp-content/uploads/2013/10/hat-vldb2014.pdf
[12]
replica_preserve_commit_order
: https://dev.mysql.com/doc/refman/8.0/en/replication-options-replica.html#sysvar_replica_preserve_commit_order
[13]
与弱隔离等级相关的错误的数量和影响被广泛低估: https://dl.acm.org/doi/10.1145/3035918.3064037
[14]
测试PostgreSQL的并行性能: https://lchsk.com/benchmarking-concurrent-operations-in-postgresql
[15]
在单台服务器上跑起推特: https://thume.ca/2023/01/02/one-machine-twitter/
数据库应该放入K8S里吗?
数据库是否应该放入 Kubernetes / Docker 里,到今天仍然是一个充满争议的话题。k8s 作为一个先进的容器编排工具,在无状态应用管理上非常趁手;但其在处理有状态服务 —— 特别是PostgreSQL和MySQL这样的数据库时,有着本质上的局限性。
在上一篇文章《数据库放入Docker是个好主意吗?》中,我们已经讨论了容器化数据库的利弊权衡;今天我们就来聊一聊将数据库放入 K8S 中编排调度所涉及的利弊权衡 —— 并深入探讨为什么将数据库放入 K8S 中不是一个明智的选择。
摘要
Kubernetes (k8s)是一个非常优秀的容器编排工具,它的目标是帮助开发者更好地管理海量复杂的无状态应用服务。尽管它提供了诸如 StatefulSet、PV、PVC、LocalhostPV 等抽象原语用于支持有状态服务(i.e. 数据库),但这些东西对于运行有着更高可靠性要求的生产级数据库服务来说仍然远远不够。
数据库是“宠物”而非“家畜”,需要细心地照料呵护。将数据库放入K8S作为“牲畜”对待,本质上是将外部的磁盘/文件系统/存储服务变为了新的“数据库宠物”。使用 EBS/网络存储/云盘运行数据库,在可靠性与性能上有巨大劣势;然而如果使用高性能本地NVMe磁盘,与节点绑定无法调度的数据库又失去了放入K8S的主要意义。
将数据库放入 K8S 中会导致 “双输” —— K8S 失去了无状态的简单性,不能像纯无状态使用方式那样灵活搬迁调度销毁重建;而数据库也牺牲了一系列重要的属性:可靠性,安全性,性能,以及复杂度成本,却只能换来有限的“弹性”与资源利用率 —— 但虚拟机也可以做到这些!对于公有云厂商之外的用户来说,几乎都是弊远大于利的。
以 K8S为代表的“云原生”狂热已经成为了一种畸形的现象:为了k8s而上k8s。工程师想提高不可替代性堆砌额外复杂度,管理者怕踩空被业界淘汰互相卷着上线。骑自行车就能搞定的事情非要开坦克来刷经验值/证明自己,却不考虑要解决的问题是否真的需要这些屠龙术 —— 这种架构杂耍行为终将招致恶果。
我们认为在分布式网络存储的可靠性与性能超过本地存储前,将数据库放入 K8S 是一种不明智的选择。解决数据库管理复杂度并非只有 K8S 一条道路,开箱即用的开源RDS —— Pigsty 基于裸操作系统提供了另一种选择。用户应当擦亮双眼,根据自己的真实情况与需求做出明智的利弊权衡与技术决策。
当下的现状
K8S 在无状态应用服务编排领域内表现出色,但一开始对于有状态的服务极其有限 —— 尽管运行数据库并不是 K8S 与 Docker 的本意,然而这阻挡不了社区对于扩张领地的狂热 —— 布道师们将 K8S 描绘为下一代云操作系统,断言数据库必将成为 Kubernetes 中的普通应用一员。而各种用于支持有状态服务的抽象也开始涌现:StatefulSet、PV、PVC、LocalhostPV。
有无数云原生狂热者开始尝试将现有数据库搬入 K8S 中,各种数据库的 CRD 与 Operator 开始出现 —— 仅以 PostgreSQL 为例,在市面上就已经可以找到至少十款以上种不同的 K8S 部署方案:PGO,StackGres,CloudNativePG,TemboOperator,PostgresOperator,PerconaOperator,Kubegres,KubeDB,KubeBlocks,……,琳琅满目。CNCF 的景观图就这样开始迅速扩张,成为了复杂度乐园。
然而复杂度也是一种成本,随着“降本增效”成为主旋律,反思的声音开始出现 —— 下云先锋 DHH 在公有云上深度使用了 K8S,但在回归开源自建的过程中也因为过分复杂而放弃了它,仅仅用 Docker 与一个名为 Kamal 的Ruby小工具作为替代。许多人开始思考,像数据库这样的有状态服务到底适合放入 Kuberentes 中吗?
而 K8S 本身为了支持有状态应用,也变得越来越复杂,远离了容器编排平台的初心。以至于 Kubernetes 的联合创始人Tim Hockin 也在今年的 KubeCon 上罕见地发了声:《K8s在被反噬!》:“Kubernetes 变得太复杂了,它需要学会克制,否则就会停止创新,直至丢失自己的基本盘 ” 。
双输的选择
对于有状态的服务,云原生领域非常喜欢用一个“宠物”与“牲畜”的类比 —— 前者需要精心照料,细心呵护,例如数据库;而后者可以随意处置,一次性用完即丢,就是普通的无状态应用(Disposability)。
云原生应用12要素: Disposability
K8S的一个主要架构目标就是,把能当畜生的都当畜生处理。对数据库进行 “存算分离”就是这样一种尝试:把有状态的数据库服务拆分为K8S外的状态存储与K8S内的纯计算部分,状态放在云盘/EBS/分布式存储上,而“无状态”的数据库进程就可以塞进K8S里随意创建销毁与调度了。
不幸的是,数据库,特别是 OLTP 数据库是重度依赖磁盘硬件的,而网络存储的可靠性与性能相比本地磁盘仍然有数量级上的差距。因而 K8S 也提供了LocalhostPV 的选项 —— 允许用户在容器上打一个洞,直接使用节点操作系统上的数据卷,直接使用高性能/高可靠性的本地 NVMe 磁盘存储。
但这让用户面临着一个抉择:是使用垃圾云盘并忍受糟糕数据库的可靠性/性能,换取K8S的调度编排统一管理能力?还是使用高性能本地盘,但与宿主节点绑死,基本丧失所有灵活调度能力?前者是把压舱石硬塞进 K8S 的小船里,拖慢了整体的灵活性与速度;后者则是用几根钉子把 K8S 的小船锚死在某处。
运行单独的纯无状态的K8S集群是非常简单可靠的,运行在物理机裸操作系统上的有状态数据库也是十分可靠的。然而将两者混在一起的结果就是双输:K8S失去了无状态的灵活与随意调度的能力,而数据库牺牲了一堆核心属性:可靠性、安全性、效率与简单性,换来了对数据库根本不重要的“弹性”、资源利用率与Day1交付速度。
关于前者,一个鲜活的案例是由 KubeBlocks 贡献的 PostgreSQL@K8s 性能优化记。k8s 大师上了各种高级手段,解决了裸金属/裸OS上根本不存在的性能问题。关于后者的鲜活的案例是滴滴的K8S架构杂耍大翻车,如果不是将有状态的 MySQL 放在K8S里,单纯重建无状态 K8S 集群并重新发布应用,怎么会要12小时这么久才恢复?
利弊的权衡
对于严肃的生产技术选型决策,最重要的永远是利弊权衡。这里我们按照常用的“质量、安全、效率、成本”顺序,来聊一下K8S放数据库相对于经典裸金属/VM部署在技术上的利弊权衡。我并不想在这里写一篇面面俱到,好像什么都说了的论文,而是抛出一些具体问题,供大家思考与讨论。
在质量上:K8S相比物理部署新增了额外的失效点与架构复杂度,拉高了爆炸半径,并且会显著拉长故障的平均恢复时长。在《数据库放入Docker是个好主意吗?》一文中,我们已经给出了关于可靠性的论证,同样的结论也可以适用于 Kubernetes —— K8S 与 Docker 会为数据库引入额外且不必要的依赖与失效点,而且缺乏社区故障知识积累与可靠性战绩证明(MTTR/MTBF)。
在云厂商的分类体系中,K8S属于PaaS,而RDS属于更底层的IaaS。数据库服务比K8S有着更高的可靠性要求:例如,许多公司的云管平台都会依赖一个额外的 CMDB 数据库。那么这个数据库应该放在哪里呢?你不应该把 K8S 依赖的东西交给 K8S 自己来管理,也不应该添加没有必要的额外依赖,阿里云全球史诗大故障 与 滴滴K8S架构杂耍大翻车 为我们普及了这个常识。而且,如果已经有了K8S外的数据库,再去维护一套K8S内的数据库体系就更得不偿失了。
在安全 上: 多租户环境中的数据库新增了额外的攻击面,带来了更高的风险与更复杂的审计合规挑战。K8S 会让你的数据库更安全吗?也许K8S架构杂耍的复杂度景象会劝退不熟悉K8S的脚本小子,但对真正的攻击者而言,更多的组件与依赖往往意味着更广的攻击面。
在《BrokenSesame 阿里云PostgreSQL 漏洞技术细节》中,安全人员利用一个自己的 PostgreSQL 容器逃脱到K8S主机节点中,并可以访问 K8S API 与其他租户的容器与数据。而这很明显是 K8S 专有的问题 —— 风险是真实存在的,这样的攻击已经发生,并让本土云厂领导者阿里云中招翻车。
《The Attacker Perspective - Insights From Hacking Alibaba Cloud》
在效率上:如《数据库放入Docker是个好主意吗?》所述,不论是额外的网络开销,Ingress 瓶颈,拉垮的云盘,对于数据库的性能都会产生负面影响。又比如《PostgreSQL@K8s 性能优化记》 所揭示的 —— 你需要相当程度的技术水平功力,才能让 K8S 中的数据库性能堪堪持平于裸机。
Latency 的单位是 ms 不是 µs,我差点以为自己眼花了。
另一个关于效率的误区是资源利用率, 不同于离线分析类业务,关键的在线 OLTP 数据库不仅不应当提高资源利用率,反而应当刻意压低资源利用率水位,从而提高系统的可靠性与用户的使用体验。如果有许许多多多零散业务,也可以通过 PDB / 共用共享数据库集群来提高资源利用率。K8S 所主张的弹性效率也并非其独有 —— KVM/EC2 也可以很好地解决这个问题。
在成本上,K8S与各种Operator提供了一个不错的抽象,封装了一部分数据库管理的复杂度,对于没有DBA的团队有一定的吸引力。然而使用它管理数据库所减少的复杂度,比起使用K8S本身引入的复杂度来说就相形见绌了。比如,随机发生的IP地址漂移与Pod自动重启,对于无状态应用来说可能并不是一个大问题,然而对数据库来说这就令人难以忍受了 —— 许多公司不得不尝试魔改 kubelet 以规避这一行为,进而又引入更多的复杂度与维护成本。
正如《从降本增笑到降本增效》“降低复杂度成本” 一节所述:智力功率很难在空间上累加:当数据库出现问题时需要数据库专家来解决;当 Kubernetes 出现问题时需要 K8S 专家看问题;然而当你把数据库放入 Kubernetes 时,复杂度出现排列组合,状态空间开始爆炸,然而单独的数据库专家和 K8S 专家的智力带宽是很难叠加的 —— 你需要一个双料专家才能解决问题,而这样的专家比起单纯的数据库专家无疑要少得多也贵得多。这样的架构杂耍足以让包括头部公有云/大厂在内的绝大多数团队,在遇到故障时出现大翻车。
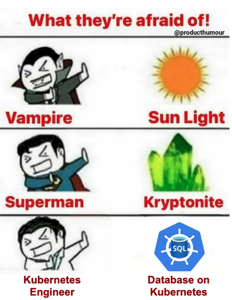
云原生狂热
一个有趣的问题是,既然 K8S 并不适用于有状态的数据库,那么为什么还有这么多厂商 —— 包括 “大厂” 在争先恐后地做这件事呢?恐怕这里的原因并不是技术上的。
Google 照着内部的 Borg 宇宙飞船做了艘 K8S 战舰开源出来,老板们怕踩空被业界淘汰进而互相卷着上线,觉得自己用上 K8S 就跟Google一样牛逼了 —— 有趣的是Google自己不用K8S,开源出来搅屎AWS忽悠业界;然而绝大多数公司并没有 Google 那样的人手去操作战舰。更重要的是他们的问题可能只要一艘舢舨就解决了。裸机上的 MySQL + PHP , PostgreSQL+ Ruby / Python / Go ,已经让无数公司一路干到上市了。
在现代硬件条件下,绝大多数应用,终其生命周期的复杂度都不足以用到 K8S 来解决。然而,以 K8S为代表的“云原生”狂热已经成为了一种畸形的现象:为了k8s而上k8s。一些工程师的目的是去寻找足够“先进”足够酷的,最好是大公司在用的东西来满足自己跳槽,晋升等个人价值的需求,或者趁机堆砌复杂度以提高自己的 Job Security,而压根不是考虑要解决问题是否真的需要这些屠龙术。
云原生领域全景图中充斥着各种花里胡哨的项目,每个新来的开发团队都想引入一套新东西,今天一个 helm 明天一个 kubevela,说起来都是光明前途,效率拉满,实际上成为了 YAML Boy 的架构屎山与复杂度乐园 —— 折腾最新的技术,发明大把的概念,经验值和声望是自己的,复杂度代价反正是用户买单,搞出问题还可以再敲一笔维护费,简直完美!
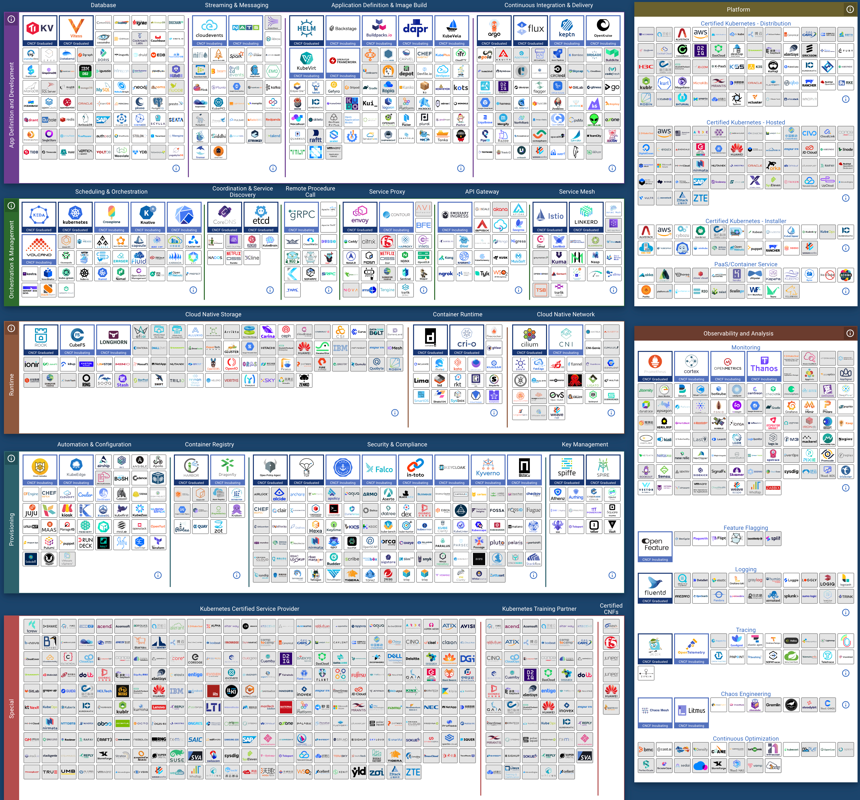
CNCF Landscape
云原生运动的理念是很有感召力的 —— 让本来是公有云专属的弹性调度能力普及到每一个用户身上,K8S 也确实在无状态应用上表现出色。然而过度的狂热已经让 K8S 偏离了原本的初心与方向 —— 简单地做好无状态应用编排调度这件事,被支持有状态应用的妄念拖累的也不再简单了。
明智地决策
几年前刚接触K8S时,我也曾有过这种皈依者狂热 —— 在探探我们也有着两万多核几百套数据库,我迫切地想要尝试将数据库放入 Kubernetes 中,并测遍了各种 Operator。然而在前后长达两三年的方案调研与架构设计中,我最终冷静下来,并放弃了这种疯狂的打算 —— 而是选择基于裸金属/裸操作系统架构我们自己的数据库服务。因为在对我们来说,K8S为数据库带来的收益相比其引入的问题与麻烦,实在是微不足道。
数据库应该放入K8S里吗?这取决于具体场景:对于从资源利用率里用超卖刨食吃的云厂商而言,弹性与资源利用率非常重要,它们直接与收入和利润挂钩;稳定可靠效率都得屈居其次 —— 毕竟可用性低于3个9也不过是按SLA赔偿本月消费25%的代金券而已。但是对于我们自己,以及生态光谱中的大多数用户而言,这些利弊权衡就不成立了:一次性的 Day1 Setup效率,弹性与资源利用率并不是他们最关心的问题;可靠性、性能、Day2 Operation成本,这些数据库的核心属性才是最重要的。
我们将自己的数据库服务架构方案开源出来 —— 即开箱即用的 PostgreSQL 发行版与本地优先的 RDS 替代: Pigsty。我们没有选择 K8S 与 Docker 这种所谓 “一次构建,到处运行 ” 的讨巧办法,而是一个一个地去适配不同的操作系统发行版/不同的大版本,并使用 Ansible 实现类 K8S CRD IaC 的效果封装管理复杂度。这确实是一件非常幸苦的工作,但却是正确的事情 —— 这个世界并不需要又一个在 K8S 中放入PG数据库玩具积木的拙劣尝试,但确实需要一个最大化发挥出硬件性能与可靠性的生产数据库服务架构方案。
Pigsty vs Stackgres
也许有一天,当分布式网络存储的可靠性与性能可以超过本地存储的表现时,以及当主流数据库都对存算分离有一定程度上的支持后,事情会再次发生变化 —— K8S 变得适用于数据库起来。但至少就目前来讲,我认为将严肃的生产 OLTP 数据库放入 K8S ,仍然是不成熟与不合时宜的。希望读者可以擦亮双眼,在这件事上做出明智的选择。
参考阅读
《Docker 的诅咒:曾以为它是终极解法,最后却是“罪大恶极”?》
专用向量数据库凉了吗?
向量存储检索是个真需求,然而专用向量数据库已经凉了。—— 小微需求 OpenAI 亲自下场解决了,标准需求被加装向量扩展的现有成熟数据库抢占。留给专用向量数据库的生态位也许能支持一家专用向量数据库存活,但想靠讲AI故事来整活做成一个产业已经是不可能了。
向量数据库是怎么火起来的?
专用向量数据库早在几年前就出现了,比如 Milvus,主要针对的是非结构化多模态数据的检索。例如以图搜图(拍立淘),以音搜音(Shazam),用视频搜视频这类需求;PostgreSQL 生态的 pgvector,pase 等插件也可以干这些事。总的来说,算是个小众需求,一直不温不火。
但 OpenAI / ChatGPT 的出现改变了这一切:大模型可以理解各种形式的文本/图片/音视频,并统一编码为同一维度的向量,而向量数据库便可以用来存储与检索这些AI大模型的输出 —— Embedding《大模型与向量数据库》。
更具体讲,向量数据库爆火的关键节点是今年3月23日,OpenAI 在其发布的 chatgpt-retrieval-plugin 项目中推荐使用一个向量数据库,在写 ChatGPT 插件时为其添加“长期记忆”能力。然后我们可以看到,无论是 Google Trends 热搜,还是 Github Star 上,所有向量数据库项目的关注度都从那个时间节点开始起飞了。
Google Trends 与 GitHub Star
与此同时,数据库领域在投资领域沉寂了一段时间后,又迎来了一波小阳春 —— Pinecone,Qdrant,Weaviate 诸如此类的“专用向量数据库” 冒了出来,几亿几亿的融钱,生怕错过了这趟 AI 时代的基础设施快车。
向量数据库生态全景图
但是,这些暴烈地狂欢也终将以暴烈的崩塌收场。这一次茶凉的比较快,半年不到的时间,形势就翻天覆地了 —— 现在除了某些二流云厂商赶了个晚集还在发软文叫卖,已经听不到谁还在炒专用向量数据库这个冷饭了。
专用向量数据库神话的破灭还有多远?
向量数据库是一个伪需求吗?
我们不禁要问,向量数据库是一个伪需求吗?答案是:向量的存储与检索是真实需求,而且会随着AI发展水涨船高,前途光明。但这和专用的向量数据库并没有关系 —— 加装向量扩展的经典数据库会成为绝对主流,而专用的向量数据库是一个伪需求。
类似 Pinecone,Weaviate,Qdrant,Chroma 这样的专用向量数据库最初是为了解决 ChatGPT 的记忆能力不足而出现的 Workaround —— 最发布的 ChatGPT 3.5 的上下文窗口只有 4K Token,也就是不到两千个汉字。然而当下 GPT 4 的上下文窗口已经发展到了 128K,扩大了32倍,足够塞进一整篇小说了 —— 而且未来还会更大。这时候,用作临时周转的垫脚石 —— 向量数据库 SaaS 就处在一个尴尬的位置上了。
更致命的是 OpenAI 在今年11月首次开发者大会上发布的新功能 —— GPTs,对于典型的中小知识库场景,OpenAI 已经替你封装好了 “记忆” 与 “知识库” 的功能。你不需要折腾什么向量数据库,只要把知识文件上传上去写好提示词告诉 GPT 怎么用,你就可以开发出一个 Agent 来。尽管目前知识库的大小仅限于几十MB,但这对于很多场景都绰绰有余,而且上限仍有巨大提升空间。
GPTs 将 AI 的易用性提高到一个全新的层次
像 Llama 这样的开源大模型与私有化部署为向量数据库扳回一局 —— 然而,这一部分需求却被加装了向量功能的经典数据库占领了 —— 以 PostgreSQL 上的 PGVector 扩展为先锋代表,其他数据库如 Redis,ElasticSearch, ClickHouse, Cassandra 也紧随其后不甘示弱。说到底,向量与向量检索是一种新的数据类型和查询处理方法,而不是一种全新的基础性数据处理方式。加装一种新的数据类型与索引,对设计良好的现有数据库系统来说并不是什么复杂的事情。
本地私有化部署的 RAG 架构
更大的问题在于,尽管数据库是一件门槛很高的事,但“向量”部分可以说没有任何技术门槛,而且诸如 FAISS 和 SCANN 这样的成熟开源库已经足够完美地解决这个问题了。对于有足够大规模足够复杂的场景的大厂来说,自家工程师可以不费吹灰之力地使用开源库实现这类需求,更犯不上用一个专用向量数据库了。
因此,专用向量数据库陷入了一个死局之中:小需求 OpenAI 亲自下场解决了,标准需求被加装向量扩展的现有成熟数据库抢占,支持超大型需求也几乎没什么门槛,更多可能还要靠模型微调。留给专用向量数据库的生态位也许能足以支持一家专用向量数据库内核厂商活下来,但想做成一个产业是不可能了。
通用数据库 vs 专用数据库
一个合格的向量数据库,首先得是一个合格的数据库。但是数据库是一个相当有门槛的领域,从零开始做到这一点并不容易。我通读了市面上专用向量数据库的文档,能勉强配得上“数据库”称呼的只有一个 Milvus —— 至少它的文档里还有关于备份 / 恢复 / 高可用的部分。其他专用向量数据库的设计,从文档上看基本可以视作对“数据库”这个专业领域的侮辱。
“向量”与“数据库”这两个问题的本质复杂度有着天差地别的区别,以世界上最流行的 PostgreSQL 数据库内核为例,它由上百万行C语言代码编写而成,解决“数据库”这个问题;然而基于 PostgreSQL 的向量数据库扩展 pgvector
只用了不到两千行不到的 C 代码,就解决了“向量”存储与检索的问题。这是对“向量”相对于与“数据库”这件事复杂度门槛的一个粗略量化:万分之一。
如果算上生态扩展,对比就更惊人了。
这也从另一个角度说明了向量数据库的问题 —— “向量”部分的门槛太低。数组数据结构,排序算法,以及两个向量求点积这三个知识点是大一就会讲的通识,稍微机灵点的本科生就拥有足够的知识来实现这样一个所谓的“专用向量数据库”,很难说这种编程大作业,LeetCode 简单题级别的东西有什么技术门槛 。
关系型数据库发展到今天已经相当完善了 —— 它支持各种各样的数据类型,整型,浮点数,字符串,等等等等。如果有人说要重新发明一种新的专用数据库来用,其卖点是支持一种“新的”数据类型 —— 浮点数组,核心功能是计算两个数组的距离并从库中找出最小者,而代价是其他的数据库活计几乎都没法整了,那么稍有经验的用户和工程师都会觉得 —— 这人莫不是得了失心疯?
数据库需求金字塔:性能只是选型考量之一。
在绝大多数情况下,使用专用向量数据库的弊都要远远大于利:数据冗余、 大量不必要的数据搬运工作、分布式组件之间缺乏一致性、额外的专业技能带来的复杂度成本、学习成本、以及人力成本、 额外的软件许可费用、极其有限的查询语言能力、可编程性、可扩展性、有限的工具链、以及与真正数据库相比更差的数据完整性和可用性。用户唯一能够期待的收益通常是性能 —— 响应时间或吞吐量,然而这个仅存的“优点”很快也不再成立了…
案例PvP:pgvector vs pinecone
抽象的理论分析不如实际的案例更有说服力,因此让我们来看一对具体的对比:pgvector 与 pinecone。前者是基于 PostgreSQL 的向量扩展,正在向量数据库生态位中疯狂攻城略地;后者是专用向量数据库 SaaS,列于 OpenAI 首批专用向量库推荐列表首位 —— 两者可以说是通用数据库与专用数据库中最典型的代表。
在 Pinecone 的官方网站上,Pinecone 提出的主要亮点特性是:“高性能,更易用”。首先来看专用向量数据库引以为豪的高性能。Supabase 给出了一个最新的测试案例,以 ANN Benchmark 中的 DBPedia 作为基准,这是由一百万个 OpenAI 1536 维向量组成的数据集。在相同的召回率下,PGVector 都有着更佳的延迟表现与总体吞吐,而且成本上要便宜得多。即使是老版本的 IVFFLAT 索引,都比 Pinecone 表现更好。
来自 Supabase - DBPedia 的测试结果
尽管专用向量数据库 Pinecone 的性能更烂,但说实话:向量数据库的性能其实根本就不重要 —— 以致于生产上 100% 精确的全表暴力扫描 KNN 有时候都是一种切实可行的选项。更何况向量数据库需要与模型搭配使用,当大模型 API 的响应时间在百毫秒 ~ 秒级时,把向量检索的时间从 10ms 优化到 1ms 并不能带来任何用户体验上的收益。在全员HNSW索引的情况下,可伸缩性也几乎不可能成为问题 —— 语义搜索属于读远多于写的场景,如果你需要更高的 QPS 吞吐量,增配/拖从库就可以了。至于节省几倍资源这种事,以常见业务的规模与当下的资源成本来看,相比模型推理的开销只能说连三瓜两枣都算不上了。
在易用性上,专用的 Python API 还是通用的 SQL Interface 更易用,这种事见仁见智 —— 真正的致命问题在于,许多语义检索场景都需要使用一些额外的字段与计算逻辑,来对向量检索召回的结果进行进一步的筛选与处理,即 —— 混合检索。而这些元数据往往保存在一个关系数据库里作为 Source of Truth。Pinecone 确实允许你为每个向量附加不超过 40KB 的元数据,但这件事需要用户自己来维护,基于API的设计会将专用向量数据库变成可扩展性与可维护性的地狱 —— 如果你需要对主数据源进行额外的查询来完成这一点,那为啥不在主关系库上直接以统一的 SQL 一步到位直接实现呢?
作为一个数据库,Pinecone 还缺乏各种数据库应该具备的基础性能力,例如:备份/恢复/高可用、批量更新/查询操作,事务/ACID;此外,除了俭朴的 API Call 之外没有与上游数据源更可靠的数据同步机制。无法实时在召回率与响应速度之间通过参数来进行利弊权衡 —— 除了更改 Pod 类型在三档准确性中选择之外别无他法 —— 你甚至不能通过暴力全表搜索达到 100% 精确度 ,因为 Pinecone 不提供精确 KNN 这个选项!
并不只有 Pinecone 是这样,除了 Milvus 之外的其他几个专用向量数据库也基本类似。当然也有用户会抗辩说一个 SaaS 如何与数据库软件做对比,这并不是一个问题,各家云厂商的 RDS for PostgreSQL 都已经提供了 PGVector 扩展,也有诸如 Neon / Supabase 这样的 Serverless / SaaS 和 Pigsty 这样的自建发行版。如果你可以用低的多的成本,拥有功能更强,性能更好,稳定性安全性更扎实的通用向量数据库,那么又为什么要花大价钱与大把时间去折腾一个没有任何优势的 “专用向量数据库”?想明白这一点的用户已经从 pinecone 向 pgvector 迁移了 —— 《为什么我们用 PGVector 替换了 Pinecone》
小结
向量的存储与检索是一个真实需求,而且会随着AI发展水涨船高,前途光明 —— 向量将成为AI时代的JSON;但这里并没有多少位置留给专用的向量数据库 —— 诸如 PostgreSQL 这样的头部数据库毫不费力的加装了向量功能,并以压倒性的优势从专用向量数据库身上碾过。留给专用向量数据库的生态位也许能支持一家专用向量数据库存活,但想靠讲AI故事来整活,做成一个产业已经是不可能了。
专用向量数据库确实已经凉掉了,希望读者也不要再走弯路,折腾这些没有前途的东西了。
数据库真被卡脖子了吗?
如果说“云数据库”算是成本ROI略欠体面的合格品,那么很多“国产数据库”就是烂泥扶不上墙的残次品。信创操作系统数据库约等于 IT 预制菜进校园。用户捏着鼻子迁移,开发者假装在卖力,陪着不懂也不在乎技术的领导演戏。大量人力财力被挥霍到没有价值的地方去,反而了浪费掉了真正的机会。基础软件行业其实没人卡脖子,真正卡脖子的都是所谓“自己人”。
垄断关系生意
北京欢乐谷门口大喇叭一直在喊:“请不要在门外购买劣质矿泉水”,小贩都被轰的远远儿的。进去后园区就会把一样的东西用五倍的价格卖给你(当然也可能是掺尿内销啤酒这种更烂的东西)。信创数据库与操作系统大体就是这种模式,都是靠垄断保护吃饭的关系生意。这与预制菜进校园有异曲同工之妙:瓦格纳头子靠承包军队/学校伙食发的财都够搞雇佣兵造反了,堪称一本万利。
问题在于,吃预制菜的人不一定有得选,但用数据库和操作系统的用户可以用脚投票,选择更先进还不要钱的开源操作系统/数据库,这可如何是好呢?毕竟国产库很多也是跟在全球开源 OS/DB 社区屁股后面捡面包屑吃。无数国产内核基于开源PG换皮套壳魔改而成。如果说谁在数据库内核上被卡了脖子,那肯定是吃的花样太多给噎着了。
许多公司看 Oracle 大肆收割的眼红的不行不行,羡慕的哈喇子都要流下来了 —— 可如果用户选择直接去用唾手可及的免费开源软件,国产数据库还怎么去割韭菜?这属于国有资产流失啊!土鳖要翻身,得先欺师灭祖:把开源免费的软件包装一下,用 Oracle 的价格卖给你!
首先搞个数据库硬分叉,把 pg
这俩字母先重命名一下;掺点垃圾代码混淆,再换用 C++ 搅一搅 —— 100%代码自主率,自主知识产权都有啦。然后找几个高校教授老院士来站台论证一下,开源数据库 MySQL 和 PostgreSQL 都是渣渣。最后给领导讲一讲:境外势力亡我之心不死,开源都是帝国主义摧毁我们国产软件行业的阳谋,得 “管一管” ,不抵制不行啦!
开源社区主导的项目都已经深度全球化,单一国家想制裁几乎没有办法:ARM 可以制裁,RISC-V 可以制裁吗?Windows 可以制裁,Linux 可以制裁吗?Oracle / MySQL 可以制裁,PostgreSQL 可以制裁吗?但是别人制裁不了你,你可以“制裁”别人,主动把门给关上呀!
这类企业恐怕做梦都盼着国家被技术封锁:门只有从两边一起关,才更容易关严实。门关严实之后,谁掌握了技术输液管,谁就掌握了利润源泉:那些掌握了独占翻墙权的“国产软件”企业只要定期从全球开源生态拾取些面包渣翻译引入进来,饿的嗷嗷叫的国内用户就要感激涕零,高呼遥遥领先了。
谁受到了伤害?
用户是最受伤的:本来的业务系统跑的好好的,突然就被要求 “升级改造” 了。如果是正向改造,那起码还算是有一些价值,但用来替换现有系统的都是些什么牛鬼蛇神。如果是纯粹的开源换皮也就算了,买点服务兜底还算有价值,最离谱的就是那些做一些自以为是“优化”的魔改阉割版本 —— 大把时间本可以用于更有价值的事情,现在却浪费在削足适履,饮掺尿啤酒,当小白鼠踩坑上了。
数据库开发者受了伤,大好的青春年华与技术生涯浪费在没有未来,没有希望的“数据库过家家”游戏上 —— 做出来的东西只能靠销售关系强行填喂给倒霉的用户,听到的都是用户侧同行的怒骂吐槽与冷嘲热讽。就别提技术影响力和出口创汇了,国际同行都不屑于来耻笑一下,“制裁”也不稀得给一个。整个工作毫无技术成就感可言,人也在日复一日的自我怀疑中变得麻木与犬儒。
国家实力受了伤。各行业与全球软件产业链主动脱钩:稳定性,功能性,战斗力受创。自主可控是一个真实需求,但盲目推行某某名录,歪曲自主可控的实质内涵(将运维自主可控扭曲为研发自主可控),用劣币驱逐良币,会导致实质的自主可控能力不升反降。
且不说和开源比,就连 Oracle 好歹还是个 Paper License,也有很多三方服务供应商;而有的国产数据库没 License 就立即死给你看,原厂一完蛋,连带着业务系统跟着遭殃。从被国外领先数据库“卡脖子” 换为国内土鳖供应商卡脖子,并不会提高自主可控能力,还额外损失了功能活性
劣币驱逐良币
在CSDN最近的开发者调研中,在七成受访者对“国产数据库”持负面印象:“技术落后”,“缺乏创新”,这算是是一种比较温和的说法。用户心底真正的评价恐怕更为直白:虚假宣传,大放卫星,落后生产力。为什么国产数据库的风评如此之差,难道是软件工程师不爱国吗?
根据信通院与墨天轮统计,现在已经有了两百六十多款“国产数据库”。其中基于开源 PostgreSQL / MySQL 的占了半壁江山还多。这是相当离谱的数字,实际上,大量数据库厂商并没有能力提供真正意义上的“产品”,只是把开源数据库简单换皮包装提供服务,辅以炒作一些分布式、HTAP之类的伪需求。
真正自研的数据库出现两极分化:极少数真正有创新贡献与使用价值的产品爱惜羽毛,不会刻意标榜“国产”。而剩下的大多数往往多是闭门造车、技术落后的土法数据库,或者开源古早分叉、负向阉割出来的劣质轮子。国产数据库并非没有踏实做事的好公司**,只是****“国产”这个标签被大量钻入数据库领域的平庸低劣产品污染**。
更让人扼腕的是劣币驱逐良币。本已稀缺的数据库研发人力经过这样的挥霍,反而会真正卡死国内数据库产业的脖子。特别是核心的OLTP/关系型数据库领域因为开源的存在,已经不缺足够好用的内核了。能把 PostgreSQL / MySQL 用好并提供服务支持,远比自欺欺人的大炼内核要有价值的多。
出路会在哪里?
中国数据库行业里优秀的工程师并不少,但极其匮乏优秀的领军人物或产品经理。或者说,这种人也有,但根本说不上话。最为重要的是,要找到正确的问题与正确的方向去发力。兵熊熊一个,将熊熊一窝:方向对了,即使只有一个人,也能做出有价值的东西;方向错了,养它一千个内核研发也是白努力。
当下的现状是什么?数据库内核已经卷不动了!作为一项有四五十年历史的技术,能折腾的东西已经被折腾的差不多了。业界已经不缺足够完美的数据库内核了 —— 比如 PostgreSQL,功能完备且开源免费(BSD-Like)。无数”国产数据库“基于PG换皮套壳魔改而成。如果说谁在数据库内核上被卡了脖子,那肯定是吃饱了撑着给噎着的。
那么,真正稀缺的是什么,是把现有的内核用好的能力。要解决这个问题,有两种思路:第一种是开发扩展,以增量功能包的方式为内核加装功能 —— 解决某一个特定领域的问题。第二种是整合生态,将扩展,依赖,底座,基础设施,融合成完整的产品 & 解决方案 —— 数据库发行版。
在这两个方向上发力,可以产生实打实的增量用户价值,站在巨人的肩膀上,并深度参与全球软件供应链,响应号召,打造真正意义上的“人类命运共同体”。相反,去分叉一个现有成熟开源内核是极其愚蠢的做法。像 PostgreSQL 与 Linux 这样的 DB/OS 内核是全世界开发者的集体智慧结晶,并通过全世界用户各种场景的打磨与考验,指望靠某一个公司的力量就能与之抗衡是不切实际的妄想。
中国想要打造自己的世界体系,成为负责任的大国,就应当胸怀天下,扛起开源运动的大旗来:展现社会主义公有制制度在软件信息互联网领域的优越性,积极赞助、参与并引领全球开源软件事业的发展,深度参与全球软件供应链,提高在全球社区中的话语权。关起门在开源社区后面捡面包屑吃,整天搞一些换皮套壳魔改的小动作,做一些没有使用价值的软件分叉,不仅压制了真正的技术创新潜能,更是会贻笑/自绝于全球软件产业链,拉低自己的竞争力。不可不察也。
老冯评论
IT 后发国家如何保证软件系统的自主可控?瑞士政府通过开源立法走在时代前沿,给其他国家打了个样。中说老美政府对开源接受度(相对欧洲)不高是因为美国国内有着无数商业软件、云计算服务公司,是 IT 世界的霸主、创新源泉与先发者。 而后发者如果想要颠覆这种国际秩序,挑战这种软件霸权,真正的王道就是彻底拥抱开源 —— 软件共产主义。这也是 人类命运共同体理念 在软件世界的真正实践,也是一条切实可行,蓬勃发展的康庄大道。
欧洲国家在这件事上一直走在前沿,即使是半欧半亚的俄罗斯,在真正遭受到制裁之后,也是通过开源来满足 IT 软件需求的 —— Postgres Pro 成为了俄罗斯数据库世界的扛把子,迅速填补支撑起了 Oracle / MySQL 离去后的空白 —— 完全没有什么“卡脖子” 问题,也没有什么奇奇怪怪的 “俄罗斯国产数据库/国产操作系统” 行业。
而 “民族主义国产软件” 则是一条会把整个行业带入万劫不复无底深渊的彻底的死路。 有些人精心编制了一个弥天大谎 —— “卡脖子” 来欺骗祖国,将国家对软件 “自主可控” 的真需求歪曲成 “国产化” 的伪需求而谋取私利。 更是有通过无下限的民族主义营销谋取不正当竞争优势,通过低水平重复性建设、恶性硬分叉社区等行为污染开源软件生态,通过制造割裂与脱钩,让软件行业自绝于世界从而垄断技术话语权,这口毒奶将不知道贻害多少年。
总书记在第二十届中央政治局第十一次集体学习会议指出:“发展新质生产力是推动高质量发展的内在要求和重要着力点”。那么什么是新质生产力?在基础软件领域,开源就是新质生产力,而套壳换皮魔改开源的 “国产化软件”,走这条路是走不到世界前列的。
抛开应用 “一行代码不改” 的妄念需求,像 PostgreSQL 这样的开源数据库内核早就可以替代 Oracle 了。许多国产数据库套着PG的皮,打着解决 “Oracle” 卡脖子的幌子,一股脑地去做所谓 “Oracle兼容性” ,却根本看不到数据库领域的前沿发展方向 —— AWS 这样的云厂商拿着开源的 PostgreSQL / MySQL 内核与自己的 RDS 管控 大杀四方,拳打 Oracle,脚踢 SQL Server,已经是数据库市场大哥大了。
高科技行业就是要依靠技术创新驱动。如果你能用开源的PG替代Oracle,那别人也能 —— 最好的结果无非就是甲骨文放弃传统数据库转型做云服务,传统数据库成为低利润的制造业。正如二十年的 PC 行业一样。二十年前 IBM 戴尔惠普都是国际玩家,中国联想说要做到世界一流。今天看联想确实做到了,但是 PC 行业早就不是高科技行业了,只是一个最无聊普通的制造业。
即使是 OB 与 Ti 这样看似最能打的真自研国产分布式数据库,所能期待的最好结局也不过是成为数据库行业的长虹,赚五个点的利润。然后被拿着开源 PostgreSQL 内核提供服务的 云厂商 RDS 和本地优先 RDS 骑脸输出按在地上摩擦,和他们心心念念替代的 Oracle 一起 —— 就像二十年前的 IBM IMS 一样,被冲进历史的马桶中。
参考阅读
EL系操作系统发行版哪家强?
有很多用户都问过我,跑数据库用什么操作系统比较好。特别是考虑到 CentOS 7.9 明年就 EOL了,应该有不少用户需要升级OS了,所以今天分享一些经验之谈。
太长不看
长话短说,在现在这个时间点如果用 EL 系列操作系统发行版,特别是如果要跑 PostgreSQL 相关的服务,我强烈推荐 RockyLinux,有“国产化”要求的也可以选龙蜥 OpenAnolis。AlmaLinux 和 OracleLinux 兼容性有点问题,不建议使用。Euler 属于独一档的 IT 领域预制菜进校园,有 EL 兼容要求的可以直接略过了
兼容水平:RHEL = Rocky ≈ Anolis > Alma > Oracle » Euler 。
在EL大版本上,EL7目前的状态最稳定,但马上 EOL 了,而且很多软件版本都太老了,所以新上的项目不建议使用了;EL 9最新,但偶尔会在仓库源更新后出现软件包依赖错误的问题。,少软件也还没有跟进 EL9 的包,比如 Citus / RedisStack / Greenplum等。
目前综合来看,EL8 是主流的选择:软件版本足够新,也足够稳定。具体的版本上建议使用 RockyLinux 8.9(Green Obsidian) 或 OpenAnolis 8.8 (rhck内核)。 激进的用户可以试试 9.3 ,保守稳妥的用户可以使用 CentOS 7.9 。
测试方法论
我们做开箱即用 PostgreSQL 数据库发行版 Pigsty,不使用容器/编排方案,因此免不了与各种操作系统打交道,基本上 EL 系的 OS 发行版我们都测试过一遍,最近刚刚把 Anolis / Euler 以及 Ubuntu / Debian 的适配做完。关于 OS EL兼容性还是有一些经验心得的。
Pigsty 的场景非常具有代表性 —— 在裸操作系统上运行世界上最先进且最流行的开源关系型数据库 PostgreSQL,以及企业级数据库服务所需要的完整软件组件。包括了 PostgreSQL 生命周期中的5个大版本(12 - 16)以及一百多个扩展插件。还有几十个常用的主机节点软件包,Prometheus / Grafana 可观测性全家桶,以及 ETCD / MinIO / Redis 等辅助组件。
测试方法很简单,这些 EL原生的RPM包,能不能在其他这些“兼容”系统上跑起来 —— 至少安装运行不能出错吧?每次 CI 的时候,我们会拉起三十台安装有不同操作系统的虚拟机进行完整安装,涉及到的软件包如下所示:
repo_packages:
- ansible python3 python3-pip python36-virtualenv python36-requests python36-idna yum-utils createrepo_c sshpass # Distro & Boot
- nginx dnsmasq etcd haproxy vip-manager pg_exporter pgbackrest_exporter # Pigsty Addons
- grafana loki logcli promtail prometheus2 alertmanager pushgateway node_exporter blackbox_exporter nginx_exporter keepalived_exporter # Infra Packages
- lz4 unzip bzip2 zlib yum pv jq git ncdu make patch bash lsof wget uuid tuned nvme-cli numactl grubby sysstat iotop htop rsync tcpdump perf flamegraph # Node Packages 1
- netcat socat ftp lrzsz net-tools ipvsadm bind-utils telnet audit ca-certificates openssl openssh-clients readline vim-minimal keepalived chrony # Node Packages 2
- patroni patroni-etcd pgbouncer pgbadger pgbackrest pgloader pg_activity pg_filedump timescaledb-tools scws pgxnclient pgFormatter # PG Common Tools
- postgresql15* pg_repack_15* wal2json_15* passwordcheck_cracklib_15* pglogical_15* pg_cron_15* postgis33_15* timescaledb-2-postgresql-15* pgvector_15* citus_15* # PGDG 15 Packages
- imgsmlr_15* pg_bigm_15* pg_similarity_15* pgsql-http_15* pgsql-gzip_15* vault_15 pgjwt_15 pg_tle_15* pg_roaringbitmap_15* pointcloud_15* zhparser_15* apache-age_15* hydra_15* pg_sparse_15*
- orafce_15* mysqlcompat_15 mongo_fdw_15* tds_fdw_15* mysql_fdw_15 hdfs_fdw_15 sqlite_fdw_15 pgbouncer_fdw_15 multicorn2_15* powa_15* pg_stat_kcache_15* pg_stat_monitor_15* pg_qualstats_15 pg_track_settings_15 pg_wait_sampling_15 system_stats_15
- plprofiler_15* plproxy_15 plsh_15* pldebugger_15 plpgsql_check_15* pgtt_15 pgq_15* hypopg_15* timestamp9_15* semver_15* prefix_15* periods_15* ip4r_15* tdigest_15* hll_15* pgmp_15 topn_15* geoip_15 extra_window_functions_15 pgsql_tweaks_15 count_distinct_15
- pg_background_15 e-maj_15 pg_catcheck_15 pg_prioritize_15 pgcopydb_15 pgcryptokey_15 logerrors_15 pg_top_15 pg_comparator_15 pg_ivm_15* pgsodium_15* pgfincore_15* ddlx_15 credcheck_15 safeupdate_15 pg_squeeze_15* pg_fkpart_15 pg_jobmon_15 rum_15
- pg_partman_15 pg_permissions_15 pgexportdoc_15 pgimportdoc_15 pg_statement_rollback_15* pg_auth_mon_15 pg_checksums_15 pg_failover_slots_15 pg_readonly_15* postgresql-unit_15* pg_store_plans_15* pg_uuidv7_15* set_user_15* pgaudit17_15
- redis_exporter mysqld_exporter mongodb_exporter docker-ce docker-compose-plugin redis minio mcli ferretdb duckdb sealos # Miscellaneous Packages
测试结果基本可以分为三种情况:100% 兼容,小错误,大麻烦。
-
100% 兼容:RockyLinux,OpenAnolis
-
小错误:AlmaLinux,OracleLinux,CentOS Stream
-
大麻烦:OpenEuler
RockyLinux 属于 100% 兼容,各种软件包安装非常流畅,没有遇到任何问题,OpenAnolis 的使用体验与 Rocky 基本一致。AlmaLinux 和 OracleLinux,以及 CentOS Stream 有少量软件包缺失,有办法补上修复,总的来说有些小错误,但可以克服。Euler 属于独一档的大麻烦,软件包遇到了大量版本依赖错误崩溃,几乎所有包都需要针对性编译,有的包因为系统依赖版本冲突问题连编译都困难了,作为EL系OS发行版的适配成本甚至比 Ubuntu/Debian 还高。
使用体验
RockyLinux 的使用体验最好,它的创始人就是原来 CentOS 的创始人,CentOS 被红帽收购后又另起炉灶搞的新 Fork。目前基本已经占据了原本 CentOS 的生态位。
最重要的是,PostgreSQL 官方源明确声明支持的 EL 系 OS 除了 RHEL 之外就是 RockyLinux 了。PGDG 构建环境就是 Rocky 8.8 与 9.2(6/7用的是CentOS)。可以说是对 PG 支持最好的 OS 发行版了。实际使用体验也非常不错,如果您没有特殊的需求,它应该是 EL 系 OS 的默认选择。
RockyLinux:100% BUG级兼容
龙蜥 / OpenAnolis 是阿里云牵头的国产化操作系统,号称100%兼容EL。本来我并没抱太大期望:只是有用户想用,我就支持一下,但实际效果超出了预期:EL8 的所有 RPM 包都一遍过,适配除了处理下 /etc/os-release 之外没有任何额外工作。适配了 Anolis 一个,就等于适配了十几种 “国产操作系统系统”发行版:阿里云、统信软件、中国移动、麒麟软件、中标软件、凝思软件、浪潮信息、中科方德、新支点、软通动力、博彦科技,可以说是很划算了。
基于 OpenAnolis 的商业操作系统发行版
如果您有“国产化”操作系统方面的需求,选择 OpenAnolis 或衍生的商业发行版,是一个不错的选择。
Oracle Linux / AlmaLinux / CentOS Stream 的兼容性相比 Rocky / Anolis 要拉跨一些,不是所有的 EL RPM 包都能直接安装成功:经常性出现依赖错漏问题。大部分包可以从它们自己的源里面找到补上 —— 有些兼容性问题,但基本上属于可以解决的小麻烦。这几个 OS 整体体验很一般,考虑到 Rocky / Anolis 已经足够好了,如果没有特殊理由我觉得没有必要使用这几种发行版。
OpenEuler 属于最拉跨的独一档,号称 EL兼容,但用起来完全不是这么回事。例如:在 PostgreSQL 内核与核心扩展中, postgresql15* ,patroni ,postgis33_15,pgbadger,pgbouncer 全部都需要重新编译。而且因为使用了不同版本的 LLVM,所有插件的 LLVMJIT 也都必须重新编译才能使用,费了非常多的功夫才完成支持,还不得不阉割掉一些功能,总的来说使用体验非常糟糕。
适配时的一堆额外工作
我们有个大客户不得不用这个 OS,所以我们也不得不去做兼容性适配。适配这种操作系统简直是一种梦魇:工作量比支持 Debian / Ubuntu 系列操作系统还要大,在折腾用户这件事上确实做到了遥遥领先。
BTW,知乎上有篇文章也介绍了这几个OS发行版的坑与对比,可以看看:
一些感想
之前我写过一篇《基础软件到底需要怎样的自主可控》,聊了聊关于国产操作系统/数据库的一些现状。核心的观点是:国家对于基础软件自主可控的核心需求是现有系统在制裁封锁的情况下能否继续运行,即运维自主可控,而不是研发自主可控。
这里我测试适配了两种主流的国产化操作系统发行版,它们体现了两种不同的思路:OpenAnolis 与 EL 完全兼容,站在巨人的肩膀上,为有需要的用户提供服务与支持(运维自主可控),真正满足了用户需求 —— 不要折腾,让现有的软件/系统稳定运行。CentOS 停服,能有国内公司/社区站出来承担责任接手维护工作,这对于广大用户、现有系统与服务来说有着实打实的价值。
反观另外一个 OS Distro,选择了通篇魔改,为华而不实的“自研”虚荣面子去做一些没有使用价值甚至是负优化的垃圾分叉,却导致大量现有的软件不得不重新适配调整甚至弃用,给用户平白添加了不必要的负担,在折腾用户上做到了遥遥领先,堪称是 IT 领域的预制菜进校园,更是污染分裂了软件生态,自绝于全球软件产业链。
OpenEuler 和 OpenGauss 差不多:你说它能不能用?也不是不能用 —— 就是用着感觉跟吃屎一样。但问题是已经有自主可控也免费的饭吃了,那为什么还要吃屎呢?如果说领导就是要按头吃屎,或者给的钱实在太多了,那也没有办法。但如果把屎吃出了肉香味,还自觉遥遥领先,那就有些滑稽了。
我以前也没少嘲讽过阿里云的云服务(特别是EBS和RDS),但是在开源 OS 和 DB 上,谁是做实事谁是吹牛逼还是门清的。至少我认为 OpenAnolis 和 PolarDB 确实是有一些东西,比起 Euler 和 Gauss 这种没有使用价值的魔改分叉来说更配得上给世界另一个选择的说法。高质量有人维护提供服务的开源主干换皮发行版,要远远好于拍脑袋瞎魔改分叉出来的玩意儿。
同样是“自主可控”的EL系国产操作系统,Pigsty 对 OpenAnolis 和 OpenEuler 都提供了支持。前者的支持是开源免费的,因为没有任何适配成本。后者我们会本着客户至上的原则为有需要的客户提供支持:虽然我们已经适配完了,但永远也不会开源免费:必须收取高额的定制服务费用作为精神损失费才行。同理,我们也开源了对 PolarDB 的监控支持,但 OpenGauss 就不好意思了,还是自个儿玩去吧。
技术发展终究要适应先进生产力的发展要求,落后的东西最终总是会被时代所淘汰。用户也应该勇于发出自己的声音,并积极用脚投票,让那些做实事的产品与公司得到奖赏鼓励,让那些吹牛逼的东西早点儿淘汰滚蛋。不要到最后只剩翔吃了才追悔莫及。
基础软件需要什么样的自主可控?
当我们说自主可控时,到底在说什么?
对于一款基础软件(操作系统 / 数据库)来说,自主可控到底是指:由中国公司/中国人开发、发行、控制?还是可以运行在“国产操作系统”/国产芯片上? 名不正则言不顺,言不顺则事不成。当下的“自主可控”乱象正是与定义不清,标准不明有着莫大的关系。但这并不妨碍我们探究一下“信创安可自主可控”这件事,要实现的目标是什么?
国家的需求说起来很简单:打仗吃制裁后,现有系统还能不能继续跑起来。
软件自主可控分为两个部分:运维自主可控 与 研发自主可控 ,国家/用户真正需要的自主可控是前者。如果我们将基础软件“自主可控”的需求用金字塔层次的方式来表达,那么在这个需求金字塔中,国家的需求可以描述为: 对具有实用价值的基础软件:保三争五。至少应当做到 “本地自治运行”,最好能达到 “控制源代码”。
基础软件自主可控需求金字塔
追求研发自主可控必须考虑活性问题。当基础软件领域(操作系统/数据库)已经存在成熟开源内核时,追求所谓 自研 对于国家与用户来说几乎没有实际价值:只有当某个团队功能研发/问题解决的速度超过全球开源社区,内核自研才是有实际意义的选择。大多数号称“自研”的基础软件厂商本质是套壳、换皮、魔改开源内核,自主可控程度属于2~3级甚至更低。低质量的软件分叉不但没有使用价值,更是浪费了稀缺的软件人才与市场机遇空间、并终将导致中国软件行业与全球产业链脱节,产生巨大的负外部性。
当我们从 Oracle/其他国外商业数据库迁移到替代方案时请注意:你的自主可控水平是否有实质意义上的提升?我们需要特别注意与警惕那些打着国产自研旗号的基础软件产品在垄断保护下劣币驱逐良币,抢占真正具有活性的开源基础软件的生态位,这会对自主可控事业造成真正的伤害 —— 所谓:搬石头砸自己的脚,自己卡自己的脖子。
例如,一个所谓“自研”运行时却需要 License 文件不然就立即死给你看的国产数据库(标称L7 ,实际L2),其运维自主可控程度远比不上成熟的开源数据库(L4/L5)。如果该国产数据库公司因为任何原因失能(重组倒闭破产或被一炮轰烂),将导致一系列使用该产品的系统失去长期持续稳定运行的能力。
开源是一种全球协作的软件研发模式,在基础软件内核(操作系统/数据库)中占据压倒性优势地位。开源模式已经很好的解决了基础软件研发的问题,但没有很好地解决软件的运维问题,而这恰好是真正有意义自主可控所应当解决的 —— 软件的最终价值是在其使用过程中,而不是研发过程中实现的。真正有意义的自主可控是帮助国家/用户用好现有成熟开源操作系统/数据库内核 —— 提供基于开源内核的发行版与专业技术服务。在维持好现有/增量系统稳定运行的前提下,响应“人类命运共同体”的倡议,积极参与全球开源软件产业供应链治理,并扩大本国供应商的国际影响力。
综上所述,我们认为,运维自主可控 的重点在于:替代不可控的三方服务与受限制的商业软件,鼓励国内供应商基于流行的开源基础软件提供技术服务与发行版,对于具有重大使用价值的开源基础软件,鼓励学习、探索、研究与贡献。孵化培养国内开源社区,维护公平的竞争环境与健康的商业生态。 而 研发自主可控 的重点在于:积极参与全球开源软件产业供应链治理,提高国内软件公司与团队在全球顶级基础软件开源项目中的话语权,培养具有全球视野与先进研发能力的技术团队。应当停止低水平重复的“国产操作系统/数据库内核分叉“,着力打造具有国际影响力的服务与软件发行版。
附:自主可控的不同等级
对于基础软件来说,可控程度从高到低可细分为以下九个等级:
9:拥有软件发布权(发布权,67%)
8:掌握多数投票权(主导权,51%)
7:掌握少数否决权(否决权,34%)
6:拥有提议话语权(话语权,10%)
5:掌控源代码(跟主干修缺陷)
4:获取源代码(跨平台重分发)
3:掌控二进制(本地自治运行)
2:受限二进制(本地受限使用)
1:租用服务(调用远程服务)
其中,1 - 5 为运维自主可控,5-9 为研发自主可控。精简一下研发自主可控的几个层次,便可得到这张自主可控需求金字塔图:
自主可控第一层,租用服务的自主可控程度最差:硬件、数据都存储在供应商的服务器上。如果提供服务的公司倒闭、停产、消亡,那么软件就无法工作了,而使用这些软件创造的文档与数据就被锁死了。例如 OpenAI 提供的 ChatGPT 便属于此类。
自主可控第二层,受限二进制,意味着软件可以在自己的硬件上运行,但包含有额外的限制条件:例如需要定期更新的授权文件,或必须联网认证方可运行。此类软件的问题与上一层次类似:如果如果提供软件的公司倒闭、停产,那么使用此类软件的应用将在有限时间内死亡。一些需要授权文件才能运行的商业操作系统 / 商业数据库便属于此列。
自主可控第三层,控制二进制,意味着软件可以不受限制地在任意主流硬件上运行,用户可以在没有互联网访问的情况下不受限制地部署软件并使用其完整功能,直到地老天荒。拥有不受限制的二进制,也意味着国内供应商可以基于软件提供自己的服务,进行换皮。绝大多数场景所需要的自主可控程度落在这一层。 自主可控第四层,拥有源代码,意味着软件可以被重新编译与分发,这一层自主可控意味着即使硬件受到制裁,现有开源软件系统也可以运行在国产操作系统/硬件之上。同时也意味着国内供应商可以提供自己的发行版,提供服务,进行套壳与再分发。开源基础软件默认坐落在这一层上,绝大多标称自己“自研”的国产操作系统/数据库实质上属于这一类。
自主可控第五层,掌控源代码,意味着对开源软件有跟进与兜底的能力,这意味着即使在最极端的情况下:全球开源软件社区与中国脱钩,国内供应商也可以自行分叉、跟进主干功能特性、并修复缺陷,长期确保软件的活性与安全性。掌握源代码意味着可以进行实质性魔改,并开始从运维自主可控到研发自主可控过渡。极个别国内厂商拥有此能力,也是国家对于自主可控的期待的合理上限。
从第六层到第九层,就进入了“研发自主可控”的范畴。根据国内供应商的话语权比例可以划分为四个不同的等级(提议权/否决权/主导权/发布权)。这涉及到基础软件开源内核的参与和治理。这意味着国内供应商可以参与到全球开源基础软件供应链中,发出自己的声音与影响力,参与社区治理甚至主导项目的方向。
对于全球范围内有使用价值的开源基础软件来说,对中国有意义的自主可控策略是:去二保三争五。更高的六至九所代表的“研发自主可控” 属于 Nice to have:有当然好,应当尽可能争取,但没有也不影响现有/增量系统的自主可控。切忌为了华而不实的“自研”虚荣面子去做一些没有使用价值甚至是负优化的垃圾分叉,而抛弃功能活性的里子,自绝于全球软件产业链。
正本清源:技术反思录
最近在技术圈有一些热议的话题,云数据库是不是智商税??公有云是不是杀猪盘?分布式数据库是不是伪需求?微服务是不是蠢主意?你还需要运维和DBA吗?中台是不是一场彻头彻尾的自欺欺人?在Twitter与HackerNews上也有大量关于这类话题的讨论与争辩。
在这些议题的背后的脉络是大环境的改变:降本增效压倒其他一切,成为绝对的主旋律。开发者体验,架构可演化性,研发效率这些属性依然重要,但在 ROI 面前都要让路 —— 社会思潮与根本价值观的变化会触发所有技术的重新估值。
有人说,互联网公司砍掉一半人依然可以正常运作,只不过老板不知道是哪一半。现在收购推特的马斯克刷新了这个记录:截止到2023年5月份,推特已经从8000人一路裁员 90% 到现在的不足千人,而依然不影响其平稳运行。这个结果彻底撕下大公司病冗员问题的遮羞布,其余互联网大厂早晚会跟进,掀起新一轮大规模裁员的血雨腥风。
在经济繁荣期,大家可以有余闲冗员去自由探索,也可以使劲儿吹牛造害铺张浪费炒作。但在经济萧条下行阶段,所有务实的企业与组织都会开始重新审视过往的利弊权衡。同样的事情不仅会发生在人上,也会发生在技术上,这是实体世界的危机传导到技术界的表现:泡沫总会在某个时刻需要出清,而这件事已正在发生中。
公有云,Kubernetes,微服务,云数据库,分布式数据库,大数据全家桶,Serverless,HTAP,Microservice,等等等等,所有这些技术与理念都将面临拷问:有些事不上秤没有四两,上了秤一千斤也打不住。这个过程必然伴随着怀疑、痛苦,伤害与毁灭,但也孕育着希望,喜悦,发展与新生。花里胡哨华而不实的东西会消失在历史长河里,大浪淘沙能存留下来的才是真正的好技术。
在这场技术界的惊涛骇浪中,需要有人透过现象看本质,脚踏实地的把各项技术的好与坏,适用场景与利弊权衡讲清楚。而我本人愿意作为一个亲历者,见证者,评叙者,参与者躬身入局,加入其中。这里拟定了一个议题列表集,名为《正本清源:技术反思录》,将依次撰文讨论评论业界关心的热点与技术:
- 国产数据库是大炼钢铁吗?
- 中国对PostgreSQL的贡献约等于零吗?
- MySQL的正确性为何如此拉垮?
- 没错,数据库确实应该放入 K8s 里!(转载SealOS)
- 数据库应该放入K8S里吗?
- 把数据库放入Docker是一个好主意吗?
- 向量数据库凉了吗?
- 阿里云的羊毛抓紧薅,五千的云服务器三百拿
- 数据库真被卡脖子了吗?
- EL系操作系统发行版哪家强?
- 基础软件到底需要什么样的自主可控?
- 如何看待 MySQL vs PGSQL 直播闹剧
- 驳《MySQL:这个星球最成功的数据库》
- 向量是新的JSON 【译评】
- 【译】微服务是不是个蠢主意?
- 分布式数据库是伪需求吗?
- 数据库需求层次金字塔
- StackOverflow 2022数据库年度调查
- DBA还是一份好工作吗?
- PostgreSQL会修改开源许可证吗?
- Redis不开源是“开源”之耻,更是公有云之耻
- PostgreSQL正在吞噬数据库世界
- RDS阉掉了PostgreSQL的灵魂
- 技术极简主义:一切皆用Postgres
写作计划
《分布式数据库是不是伪需求?》
《国产数据库是不是大跃进?》
《TPC-C打榜是不是放卫星?》
《信创数据库是不是恰烂钱?》
《谁卡住了中国数据库的脖子?》
《微服务是不是蠢主意?》
《Serverless是不是榨钱术?》
《RCU/WCU计费是不是阳谋杀猪?》
《数据库到底要不要放入K8S?》
《HTAP是不是纸上谈兵?》
《单机分布式一体化是不是脱裤放屁?》
《你真的需要专用向量数据库吗?》
《你真的需要专用时序数据库吗?》
《你真的需要专用地理数据库吗?》
《APM时序数据库选型姿势指北》
《202x数据库选型指南白皮书》
《开源崛起:商业数据库还能走多远?》
《云厂商的 SLA 到底靠不靠得住?》
《大厂技术管理思想真的先进吗?》
《卷数据库内核还有没有出路?》
《用户到底需要什么样的数据库?》
《再搞 MySQL 还有没有前途?》
如果您有任何认为值得讨论的话题,也欢迎在评论区中留言提出,我将视情况加入列表中。
数据库需求层次金字塔
与马斯洛需求金字塔类似,用户对于数据库的需求也有着一个递进的层次。用户对于数据库的需求从下往上可以分为八个层次,分别与人的八个需求层次相对应:
- 生理需求,功能:内核/正确性/ACID
- 安全需求,安全:备份/保密/完整/可用
- 归属需求,可靠:高可用/监控/告警
- 尊重需求,ROI:性能/成本/复杂度
- 认知需求,洞察:可观测性/数字化/可视化
- 审美需求,掌控:可控制性/易用性/IaC
- 自我实现,智能:标准化/产品化/智能化
- 超越需求,变革:真·自治数据库
安全需求与生理需求同属基础需求,一个用于生产环境的严肃数据库系统至少应当满足这两类需求,才足以称得上是合格。归属需求与尊重需求同属进阶需求,满足这两类需求,可以称得上是体面。认知需求与审美需求属于高级需求,满足这两类需求,方能配得上 品味 二字。
在自我实现与超越需求上,不同种类的用户可能会有不同的需求,比如普通工程师的超越需求可能是升职加薪,搞出成绩赚大钱;而头部用户关注的可能是意义、创新与行业变革。
但是在基础需求与进阶需求上,所有类型的用户几乎是高度一致的。
生理需求
生理需求是级别最低、最急迫的需求,如:食物、水、空气、睡眠。
对于数据库用户来说,生理需求指的是功能:
- **内核特性:**数据库内核的特性是否满足需求?
- 正确性:功能是否正确实现,没有显著缺陷?
- ACID:是否支持确保正确性的核心功能— 事务?
对于数据库来说,功能需求就是最基础的生理需求。正确性与 ACID 是数据库最基本的要求:诚然一些不甚重要的数据与边缘系统,可以使用更灵活的数据模型,NoSQL数据库,KV存储。但对于关键核心数据来说经典 ACID 关系型数据库的地位仍然是无可取代的。此外,如果用户需要的就是 PostGIS 处理地理空间数据的能力,或者TimescaleDB 处理时序数据的能力,那么没有这些特性的数据库内核就会被一票否决。
安全需求
安全需求同样属于基础层面的需求,其中包括对人身安全、生活稳定以及免遭痛苦、威胁或疾病、身体健康以及有自己的财产等与自身安全感有关的事情。
对于数据库来说,安全需求包括:
- 机密:避免未授权的访问,数据不泄漏,不被拖库
- 完整:数据不丢不错不漏,即使误删了也有办法找回来。
- 可用:可以稳定提供服务,出了故障也有办法及时恢复回来。
安全需求无论对于数据库还是人类都是至关重要的。数据库如果丢了,被拖库了,或者数据错乱了,一些企业可能就直接破产了。满足安全需求意味着数据库有了兜底,有了灾难生存能力。冷备份,WAL归档,异地备份仓库,访问控制,流量加密,身份认证,这些技术用于满足安全需求。
安全需求与生理需求同属基础需求,一个用于生产环境的严肃数据库系统至少应当满足这两类需求,才足以称得上是合格。
归属需求
爱和归属的需求(常称为“社交需求”)属于进阶需求,如:对友谊,爱情以及隶属关系的需求。
对于数据库来说,社交需求意味着:
- 监控:有人会关注着数据库健康,监控诸如心率、血氧等核心生理指标。
- 告警:而当数据库出现问题时,指标异常时,会有人接到通知来及时处理。
- 高可用:主库不再单打独斗,拥有了自己的追随者分担工作并在故障时能接管工作。
对数据库可靠性的需求,可以与人类对于爱和归属的需求相类比。归属意味着数据库是有人关心,有人照看着,有人支持着的。监控负责感知环境收集数据库指标,而告警组件将异常现象问题及时上抛给人类处理。多物理从库副本+自动故障切换实现高可用架构的数据库,甚至软件自身便足以检测判定应对很多常见的故障。
归属需求属于进阶需求,当基础需求(功能/安全)得到满足后,用户会开始对监控告警高可用产生需求。一个体面的数据库服务,监控告警高可用是必不可少的。
尊重需求
尊重需求是指人们对自己的尊重和自信,以及希望获得他人的尊重的需求,属于进阶层面的需求。对于数据库来说,尊重需求主要包括:
- 性能:能够支撑高并发、大规模数据处理等高性能场景。
- 成本:具有合理的价格和成本控制。
- 复杂度:易于使用和管理,不会带来过多的复杂度。
对于数据库来说,安全可靠是本分,物美价廉才能出彩。数据库产品的 ROI 对应于人的尊重需求。正所谓:性价比是第一产品力,更强力、更便宜、更好用是三个核心诉求:更高的 ROI 意味着数据库以更低的财务代价与复杂度代价实现更优秀的性能表现。任何开创性的特色功能与设计,说到底也是通过提高 ROI 来赢得真正的赞誉与尊重的。
归属需求与尊重需求同属进阶需求,一个数据库系统只有满足这两类需求,才足以称得上是体面。满足了基础需求与进阶需求的用户,则会开始产生更高层次的需求:认知与审美。
认知需求
认知需求是指人们对于知识、理解和掌握新技能的需求,属于进阶层面的需求。对于数据库来说,认知需求主要包括:
- 可观测性:能够对数据库与相关系统内部运行状态进行观测,做到全知。
- 可视化:将数据通过图表等方式进行可视化展示,揭示内在联系,提供洞察。
- 数字化:使用数据作为决策依据,使用标准化决策过程而非老师傅拍脑袋。
人要进步发展须进行自省,而认知需求对于数据库也一样重要:归属需求中的“监控“只关注数据库的基本生存状态,而认知需求关注的是对数据库与环境的理解与洞察。现代可观测性技术栈将收集丰富的监控指标并进行可视化呈现,而 DBA / 研发 / 运维 / 数据分析 人员则会从数据与可视化中提取洞察,形成对系统的理解与认知。
没有观测就谈不上控制,可观测是为了可控制,全知即全能。只有对数据库有了深入的认知,才可以真正做到收放自如,随心所欲不逾矩。
审美需求
审美需求是指人们对于美的需求,包括审美体验、审美评价和审美创造。对于数据库来说,审美需求主要包括:
- 可控制性:用户的意志可以被数据库系统贯彻执行。
- 易用性:友好的界面接口工具,最小化人工操作。
- IaC:基础设施即代码,使用声明式配置描述环境。
对于数据库来说,审美需求意味着更高级的掌控能力:简单易用的接口,高度自动化的实现,精细的定制选项,以及声明式的管理哲学。
高度可控,简单易用的数据库,才是有品味的数据库。可控制性是可观测性的对偶概念,指:是否可以通过一些允许的程序让系统调整到其状态空间内的任何一个状态。传统的运维方式关注过程,要创建/销毁/扩缩容数据库集群,用户需要按照手册依次执行各种命令;而现代管理方式关注状态,用户声明式的表达自己想要什么,而系统自动调整至用户所描述的状态。
洞察与掌控求属于高级需求,满足这两类需求的数据库系统,才足以称得上 品味。而这两者,也是满足更高超越层面需求的基石。
自我实现
自我实现(Self-actualization)是指人们追求最高水平的自我实现和个人成长的需求,属于超越层面的需求。对于数据库来说,自我实现需求主要包括:
- 标准化:将各类操作沉淀为 SOP ,沉淀故障文档应急预案与制度最佳实践,量产DBA。
- 产品化:将用好/管好数据库的经验沉淀转化为可批量复制的工具、产品与服务。
- 智能化:范式转变,沉淀出领域特定的模型,完成人到软件的转变与飞跃。
数据库的自我实现与人类似:繁衍与进化。为了持续存在,数据库需要“繁衍”扩大存在规模,这需要的是标准化与产品化,而不是一个接一个的项目。关系数据库内核功能标准化已经有SQL作为标准,然而使用数据库的方法与管理数据库的人还差的很远:更多依赖的是老师傅的直觉与经验。以 GPT4 为代表的大模型,揭示了由 AI 替代专家(领域模型)的可能性,Soon or Later,一定会演进出现模型化的DBA,实现感知-决策-执行三个层面的彻底自动化。
超越需求
超越需求(Self-transcendence)是指人们追求更高层次的价值和意义的需求,属于最高层面的需求。对于数据库来说,这也许意味着 一个几乎不需要人参与的真·自治数据库系统
前面的所有需求都得到满足时,超越需求会出现。想要做到真正的数据库自治,前提就是感知、思考、执行三个部分的自动化与智能化。认知层次的需求解决“信息系统”,负责感知职能;审美层次的需求解决“行动系统”,负责实施控制;而自我实现层次解决“模型系统”,负责做出决策。
这也可也说是数据库领域的圣杯与终极目标之一了。
然后呢?
理论模型可以帮助我们对数据系统/发行版/管控软件/云服务进行更深刻的评价与对比。比如:大部分土法自建的数据库可能还在生理需求和安全需求上挣扎,属于不合格残次品。云数据库基本属于能满足下三层功能安全可靠需求的合格品,但是在 ROI / 价格上就不太体面(参阅《云数据库是不是杀猪盘》)。顶级资深数据库专家自建,可以满足更高层次的需求,但实在是太过金贵,供不应求。最后还得是广告时间:
虽然我是 Pigsty 的作者,但我更是一个资深的甲方用户。我做这个东西的原因正是因为市面上没有足够好的能满足 L4 L5 感知/管控需求的数据库产品或服务,所以才自己动手撸了一个。开源 RDS 替代 Pigsty + IDC/云服务器自建,在满足上述需求的前提下,还可以覆盖认知、审美与少部分自我实现的需求。让你的数据库坚如磐石,辅助自动驾驶,更离谱的是开源免费,ROI 上吊打一切云数据库,如果你会用到 PGSQL, (以及REDIS, ETCD, MINIO, 或者 Prometheus/Grafana全家桶), 那还不赶紧试试?http://demo.pigsty.cc
最近发布了 Pigsty 2.0.1 版本,使用以下命令一键安装。
curl -fsSL https://repo.pigsty.cc/get | bash
分布式数据库是不是伪需求?
随着硬件技术的进步,单机数据库的容量和性能已达到了前所未有的高度。而分布式(TP)数据库在这种变革面前极为无力,和“数据中台”一样穿着皇帝的新衣,处于自欺欺人的状态里。
太长不看
分布式数据库的核心权衡是:“以质换量”,牺牲功能、性能、复杂度、可靠性,换取更大的数据容量与请求吞吐量。但分久必合,硬件变革让集中式数据库的容量与吞吐达到一个全新高度,使分布式(TP)数据库失去了存在意义。
以 NVMe SSD 为代表的硬件遵循摩尔定律以指数速度演进,十年间性能翻了几十倍,价格降了几十倍,性价比提高了三个数量级。单卡 32TB+, 4K随机读写 IOPS 可达 1600K/600K,延时 70µs/10µs,价格不到 200 ¥/TB·年。跑集中式数据库单机能有一两百万的点写/点查 QPS。
真正需要分布式数据库的场景屈指可数,典型的中型互联网公司/银行请求数量级在几万到几十万QPS,不重复TP数据在百TB上下量级。真实世界中 99% 以上的场景用不上分布式数据库,剩下1%也大概率可以通过经典的水平/垂直拆分等工程手段解决。
头部互联网公司可能有极少数真正的适用场景,然而此类公司没有任何付费意愿。市场根本无法养活如此之多的分布式数据库内核,能够成活的产品靠的也不见得是分布式这个卖点。HATP 、分布式单机一体化是迷茫分布式TP数据库厂商寻求转型的挣扎,但离 PMF 仍有不小距离。
互联网的牵引
“分布式数据库” 并不是一个严格定义的术语。狭义上它与 NewSQL:cockroachdb / yugabytesdb / tidb / oceanbase / TDSQL 等数据库高度重合;广义上 Oracle / PostgreSQL / MySQL / SQL Server / PolarDB / Aurora 这种跨多个物理节点,使用主从复制或者共享存储的经典数据库也能归入其中。在本文语境中,分布式数据库指前者,且只涉及核心定位为事务处理型(OLTP)的分布式关系型数据库。
分布式数据库的兴起源于互联网应用的快速发展和数据量的爆炸式增长。在那个时代,传统的关系型数据库在面对海量数据和高并发访问时,往往会出现性能瓶颈和可伸缩性问题。即使用 Oracle 与 Exadata,在面对海量 CRUD 时也有些无力,更别提每年以百千万计的高昂软硬件费用。
互联网公司走上了另一条路,用诸如 MySQL 这样免费的开源数据库自建。老研发/DBA可能还会记得那条 MySQL 经验规约:单表记录不要超过 2100万,否则性能会迅速劣化;与之对应的是,数据库分库分表开始成为大厂显学。
这里的基本想法是“三个臭皮匠,顶个诸葛亮”,用一堆便宜的 x86 服务器 + 大量分库分表开源数据库实例弄出一个海量 CRUD 简单数据存储。故而,分布式数据库往往诞生于互联网公司的场景,并沿着手工分库分表 → 分库分表中间件 → 分布式数据库这条路径发展进步。
作为一个行业解决方案,分布式数据库成功满足了互联网公司的场景需求。但是如果想把它抽象沉淀成一个产品对外输出,还需要想清楚几个问题:
十年前的利弊权衡,在今天是否依然成立?
互联网公司的场景,对其他行业是否适用?
分布式事务数据库,会不会是一个伪需求?
分布式的权衡
“分布式” 同 “HTAP”、 “存算分离”、“Serverless”、“湖仓一体” 这样的Buzzword一样,对企业用户来说没有意义。务实的甲方关注的是实打实的属性与能力:功能性能、安全可靠、投入产出、成本效益。真正重要的是利弊权衡:分布式数据库相比经典集中式数据库,牺牲了什么换取了什么?
数据库需求层次金字塔[1]
分布式数据库的核心Trade Off 可以概括为:“以质换量”:牺牲功能、性能、复杂度、可靠性,换取更大的数据容量与请求吞吐量。
NewSQL 通常主打“分布式”的概念,通过“分布式”解决水平伸缩性问题。在架构上通常拥有多个对等数据节点以及协调者,使用分布式共识协议 Paxos/Raft 进行复制,可以通过添加数据节点的方式进行水平伸缩。
首先,分布式数据库因其内在局限性,会牺牲许多功能,只能提供较为简单有限的 CRUD 查询支持。其次,分布式数据库因为需要通过多次网络 RPC 完成请求,所以性能相比集中式数据库通常有70%以上的折损。再者,分布式数据库通常由DN/CN以及TSO等多个组件构成,运维管理复杂,引入大量非本质复杂度。最后,分布式数据库在高可用容灾方面相较于经典集中式主从并没有质变,反而因为复数组件引入大量额外失效点。
在以前,分布式数据库的利弊权衡是成立的:互联网需要更大的数据存储容量与更高的访问吞吐量:这个问题是必须解决的,而这些缺点是可以克服的。但今日,硬件的发展废问了 量 的问题,那么分布式数据库的存在意义就连同着它想解决的问题本身被一并抹除了。
时代变了,大人
新硬件的冲击
摩尔定律指出,每18~24个月,处理器性能翻倍,成本减半。这个规律也基本适用于存储。从2013年开始到2023年是5~6个周期,性能和成本和10年前比应该有几十倍的差距,是不是这样呢?
让我们看一下 2013 年典型 SSD 的性能指标,并与 2022 年 PCI-e Gen4 NVMe SSD 的典型产品进行对比。不难发现:硬盘4K随机读写 IOPS从 60K/40K 到了 1600K/600K,价格从 2220$/TB 到 40$/TB 。性能翻了15 ~ 26倍,价格便宜了56 倍[3,4,5],作为经验法则在数量级上肯定是成立了。
2013 年 HDD/SSD 性能指标
2022 年NVMe Gen4 SSD 性能指标
十年前,机械硬盘还是绝对主流。1TB 的硬盘价格大概七八百元,64GB 的SSD 还要再贵点。十年后,主流 3.2TB 的企业级 NVMe SSD 也不过三千块钱。按五年质保折算,1TB每月成本只要 16块钱,每年成本不到 200块。作为参考,云厂商号称物美价廉的 S3对象存储都要 1800¥/TB·年。
2013 - 2030 SSD/HDD 单位价格与预测
典型的第四代本地 NVMe 磁盘单卡最大容量可达 32TB~ 64TB,提供 70µs/10µs 4K随机读/写延迟,1600K/600K 的读写IOPS,第五代更是有着单卡十几GB/s 的惊人带宽。
这样的卡配上一台经典 Dell 64C / 512G 服务器,IDC代维5年折旧,总共十万块不到。而这样一台服务器跑 PostgreSQL sysbench 单机点写入可以接近百万QPS,点查询干到两百万 QPS 不成问题。
这是什么概念呢?对于一个典型的中型互联网公司/银行,数据库请求数量级通常在几万/几十万 QPS这个范围;不重复的TP数据量级在百TB上下浮动。考虑到使用硬件存储压缩卡还能有个几倍压缩比,这类场景在现代硬件条件下,有可能集中式数据库单机单卡就直接搞定了[6]。
在以前,用户可能需要先砸个几百万搞 exadata 高端存储,再花天价购买 Oracle 商业数据库授权与原厂服务。而现在做到这些,硬件上只需一块几千块的企业级 SSD 卡即可起步;像 PostgreSQL 这样的开源 Oracle 替代,最大单表32TB照样跑得飞快,不再有当年MySQL非要分表不可的桎梏。原本高性能的数据库服务从情报/银行领域的奢侈品,变成各行各业都能轻松负担得起的平价服务[7]。
性价比是第一产品力,高性能大容量的存储在十年间性价比提高了三个数量级,分布式数据库曾经的价值亮点,在这种大力出奇迹的硬件变革下显得软弱无力。
伪需求的困境
在当下,牺牲功能性能复杂度换取伸缩性有极大概率是伪需求。
在现代硬件的加持下,真实世界中 99%+ 的场景超不出单机集中式数据库的支持范围,剩下1%也大概率可以通过经典的水平/垂直拆分等工程手段解决。这一点对于互联网公司也能成立:即使是全球头部大厂,不可拆分的TP单表超过几十TB的场景依然罕见。
NewSQL的祖师爷 Google Spanner 是为了解决海量数据伸缩性的问题,但又有多少企业能有Google的业务数据量?从数据量上来讲,绝大多数企业终其生命周期的TP数据量,都超不过集中式数据库的单机瓶颈,而且这个瓶颈仍然在以摩尔定律的速度指数增长中。从请求吞吐量上来讲,很多企业的数据库性能余量足够让他们把业务逻辑全部用存储过程实现并丝滑地跑在数据库中。
“过早优化是万恶之源”,为了不需要的规模去设计是白费功夫。如果量不再成为问题,那么为了不需要的量去牺牲其他属性就成了一件毫无意义的事情。
“过早优化是万恶之源”
在数据库的许多细分领域中,分布式并不是伪需求:如果你需要一个高度可靠容灾的简单低频 KV 存储元数据,那么分布式的 etcd 就是合适的选择;如果你需要一张全球地理分布的表可以在各地任意读写,并愿意承受巨大的性能衰减作为代价,那么分布式的 YugabyteDB 也许是一个不错的选择。如果你需要进行信息公示并防止篡改与抵赖,区块链在本质上也是一种 Leaderless 的分布式账本数据库;
对于大规模数据分析OLAP来说,分布式可以说是必不可少(不过这种一般称为数据仓库,MPP);但是在事务处理OLTP领域,分布式可以说是大可不必:OTLP数据库属于工作性记忆,而工作记忆的特点就是小、快、功能丰富。即使是非常庞大的业务系统,同一时刻活跃的工作集也不会特别大。OLTP 系统设计的一个基本经验法则就是:如果你的问题规模可以在单机内解决,就不要去折腾分布式数据库。
OLTP 数据库已经有几十年的历史,现有内核已经发展到了相当成熟的地步。TP 领域标准正在逐渐收敛至 PostgreSQL,MySQL,Oracle 三种 Wire Protocol 。如果只是折腾数据库自动分库分表再加个全局事务这种“分布式”,那一定是没有出路的。如果真能有“分布式”数据库杀出一条血路,那大概率也不是因为“分布式”这个“伪需求”,而应当归功于新功能、开源生态、兼容性、易用性、国产信创、自主可控这些因素。
迷茫下的挣扎
分布式数据库最大的挑战来自于市场结构:最有可能会使用分布式TP数据库的互联网公司,反而是最不可能为此付费的一个群体。互联网公司可以作为很好的高质量用户甚至贡献者,提供案例、反馈与PR,但唯独在为软件掏钱买单这件事上与其模因本能相抵触。即使头部分布式数据库厂商,也面临着叫好不叫座的难题。
近日与某分布式数据库厂工程师闲聊时获悉,在客户那儿做 POC 时,Oracle 10秒跑完的查询,他们的分布式数据库用上各种资源和 Dirty Hack 都有一个数量级上的差距。即使是从10年前 PostgreSQL 9.2 分叉出来的 openGauss,都能在一些场景下干翻不少分布式数据库,更别提10年后的 PostgreSQL 15 与 Oracle 23c 了。这种差距甚至会让原厂都感到迷茫,分布式数据库的出路在哪里?
所以一些分布式数据库开始自救转型, HTAP 是一个典型例子:分布式搞事务鸡肋,但是做分析很好呀。那么为什么不能捏在一起凑一凑?一套系统,同时可以做事务处理与分析哟!但真实世界的工程师都明白:AP系统和TP系统各有各的模式,强行把两个需求南辕北辙的系统硬捏合在一块,只会让两件事都难以成功。不论是使用经典 ETL/CDC 推拉到专用 ClickHouse/Greenplum/Doris 去处理,还是逻辑复制到In-Mem列存的专用从库,哪一种都要比用一个奇美拉杂交HTAP数据库要更靠谱。
另一种思路是 单机分布式一体化:打不过就加入 :添加一个单机模式以规避代价高昂的网络RPC开销,起码在那些用不上分布式的99%场景中,不至于在硬指标上被集中式数据库碾压得一塌糊涂 —— 用不上分布式没关系,先拽上车别被其他人截胡! 但这里的问题本质与 HTAP 是一样的:强行整合异质数据系统没有意义,如果这样做有价值,那么为什么没人去把所有异构数据库整合一个什么都能做的巨无霸二进制 —— 数据库全能王? 因为这样违背了KISS原则:Keep It Simple, Stupid!
分布式数据库和数据中台的处境类似[8]:起源于互联网大厂内部的场景,也解决过领域特定的问题。曾几何时乘着互联网行业的东风,数据库言必谈分布式,火热风光好不得意。却因为过度的包装吹捧,承诺了太多不切实际的东西,又无法达到用户预期 —— 最终一地鸡毛,成为皇帝的新衣。
TP数据库领域还有很多地方值得投入精力:Leveraging new hardwares,积极拥抱 CXL,RDMA,NVMe 等底层体系结构变革;或者提供简单易用的声明式接口,让数据库的使用与管理更加便利;提供更为智能的自动驾驶监控管控,尽可能消除运维性的杂活儿;开发类似 Babelfish 的 MySQL / Oracle 兼容插件,实现关系数据库 WireProtocol 统一。哪怕砸钱堆人提供更好的支持服务,都比一个 “分布式” 的伪需求噱头要更有意义。
因时而动,君子不器。愿分布式数据库厂商们找到自己的 PMF,做一些用户真正需要的东西。
References
[1] 数据库需求层次金字塔 : https://mp.weixin.qq.com/s/1xR92Z67kvvj2_NpUMie1Q
[2] PostgreSQL到底有多强? : https://mp.weixin.qq.com/s/651zXDKGwFy8i0Owrmm-Xg
[3] 2013年SSD性能 : https://www.snia.org/sites/default/files/SNIASSSI.SSDPerformance-APrimer2013.pdf
[4] 2022年镁光9400 NVMe SSD 规格说明 : https://media-www.micron.com/-/media/client/global/documents/products/product-flyer/9400_nvme_ssd_product_brief.pdf
[5] 2013-2030 SSD价格走势与预测 : https://blocksandfiles.com/2021/01/25/wikibon-ssds-vs-hard-drives-wrights-law/
[6] 单实例100TB使用压缩卡到20TB: https://mp.weixin.qq.com/s/JSQPzep09rDYbM-x5ptsZA
[7] 公有云是不是杀猪盘?: https://mp.weixin.qq.com/s/UxjiUBTpb1pRUfGtR9V3ag
[8] 中台:一场彻头彻尾的自欺欺人: https://mp.weixin.qq.com/s/VgTU7NcOwmrX-nbrBBeH_w
微服务是不是个蠢主意?
亚马逊的Prime Video团队发表了一篇非常引人注目的案例研究[2] ,讲述了他们为什么放弃了微服务与Serverless架构而改用单体架构。这一举措让他们在运营成本上节省了惊人的 90%,还简化了系统复杂度,堪称一个巨大的胜利。
但除了赞扬他们的明智之举之外,我认为这里还有一个重要洞察适用于我们整个行业:
“我们最初设计的解决方案是:使用Serverless组件的分布式系统架构… 理论上这个架构可以让我们独立伸缩扩展每个服务组件。然而,我们使用某些组件的方式导致我们在大约5%的预期负载时,就遇到了硬性的伸缩限制。”
“理论上的” —— 这是对近年来在科技行业肆虐的微服务狂热做的精辟概括。现在纸上谈兵的理论终于有了真实世界的结论:在实践中,微服务的理念就像塞壬歌声一样诱惑着你,为系统添加毫无必要的复杂度,而 Serverless 只会让事情更糟糕。
这个故事最搞笑的地方是:亚马逊自己就是面向服务架构 / SOA 的最初典范与原始代言人。在微服务流行之前,这种组织模式还是很合理的:在一个疯狂的规模下,公司内部通信使用 API 调用的模式,是能吊打协调跨团队会议的模式的。
SOA 在亚马逊的规模下很有意义,没有任何一个团队能够知道或理解 驾驶这么一艘巨无霸邮轮所需的方方面面,而让团队之间通过公开发布的 API 进行协作简直是神来之笔。
但正如很多“好主意”一样,这种模式在脱离了原本的场景用在其他地方后,就开始变得极为有害了:特别是塞进单一应用架构内部时 —— 而人们就是这么搞微服务的。
从很多层面上来说,微服务是一种僵尸架构,是一种顽强的思想病毒:从 J2EE 的黑暗时代(Remote Server Beans,有人听说过吗),一直到 WS-Deathstar[3] 死星式的胡言乱语,再到现在微服务与 Serverless 的形式,它一直在吞噬大脑,消磨人们的智力。
不过这第三波浪潮总算是到顶了,我在 2016 年就写过一首关于 “宏伟的单体应用[4]” 的赞歌。Kubernetes 背后的意见领袖高塔先生也在 2020年 单体才是未来[5] 一文中表达过这一点:
“我们要打破单体应用,找到先前从未有过的工程纪律… 现在人们从编写垃圾代码变成打造垃圾平台与基础设施。
人们沉迷于这些与时髦术语绑定的热钱与炒作,因为微服务带来了大量的新开销,招聘机会与工作岗位,唯独对于解决他们的问题来说实际上并没有必要。“
没错,当你拥有一个连贯的单一团队与单体应用程序时,用网络调用和服务拆分取代方法调用与模块切分,在几乎所有情况下都是一个无比疯狂的想法。
我很高兴在记忆中已经是第三次击退这种僵尸狂潮一样的蠢主意了。但我们必须保持警惕,因为我们早晚还得继续这么干:有些山炮想法无论弄死多少次都会卷土重来。你能做的就是当它们借尸还魂的时候及时认出来,用文章霰弹枪给他喷个稀巴烂。
有效的复杂系统总是从简单的系统演化而来。反之亦然:从零设计的复杂系统没一个能有效工作的。
—— 约翰・加尔,Systemantics(1975)
本文作者 DHH, Ruby on Rails 作者,37signals CTO,译者冯若航。
原题为 Even Amazon can’t make sense of serverless or microservices[1] 。即《亚马逊自个都觉得微服务和Serverless扯淡了》
References
[1]
Even Amazon can’t make sense of serverless or microservices: https://world.hey.com/dhh/even-amazon-can-t-make-sense-of-serverless-or-microservices-59625580
[2]
引人注目的案例研究: https://www.primevideotech.com/video-streaming/scaling-up-the-prime-video-audio-video-monitoring-service-and-reducing-costs-by-90
[3]
WS-Deathstar: https://www.flickr.com/photos/psd/1428661128/
[4]
宏伟的单体应用: https://m.signalvnoise.com/the-majestic-monolith/
[5]
单体才是未来: https://changelog.com/posts/monoliths-are-the-future
是时候和GPL说再见了
原文由 Martin Kleppmann 于2021年4月14日发表,译者:冯若航。
Martin Kleppmann是《设计数据密集型应用》(a.k.a DDIA)的作者,译者冯若航为该书中文译者。
本文的导火索是Richard Stallman恢复原职,对于自由软件基金会(FSF)的董事会而言,这是一位充满争议的人物。我对此感到震惊,并与其他人一起呼吁将他撤职。这次事件让我重新评估了自由软件基金会在计算机领域的地位 —— 它是GNU项目(宽泛地说它属于Linux发行版的一部分)和以GNU通用公共许可证(GPL)为中心的软件许可证系列的管理者。这些努力不幸被Stallman的行为所玷污。然而这并不是我今天真正想谈的内容。
在本文中,我认为我们应该远离GPL和相关的许可证(LGPL、AGPL),原因与Stallman无关,只是因为,我认为它们未能实现其目的,而且它们造成的麻烦比它们产生的价值要更大。
首先简单介绍一下背景:GPL系列许可证的定义性特征是 copyleft 的概念,它指出,如果你用了一些GPL许可的代码并对其进行修改或构建,你也必须在同一许可证下免费提供你的修改/扩展(被称为"衍生作品")(大致意思)。这样一来,GPL的源代码就不能被纳入闭源软件中。乍看之下,这似乎是个好主意。那么问题在哪里?
敌人变了
在上世纪80年代和90年代,当GPL被创造出来时,自由软件运动的敌人是微软和其他销售闭源(“专有”)软件的公司。GPL打算破坏这种商业模式,主要出于两个原因:
- 闭源软件不容易被用户所修改;你可以用,也可以不用,但你不能根据自己的需求对它进行修改定制。为了抵制这种情况,GPL设计的宗旨即是,迫使公司发布其软件的源代码,这样软件的用户就可以研究、修改、编译和使用他们自己的修改定制版本,从而获得按需定制自己计算设备的自由。
- 此外,GPL的动机也包括对公平的渴望:如果你在业余时间写了一些软件并免费发布,但是别人用它获利,又不向社区回馈任何东西,你肯定也不希望这样的事情发生。强制衍生作品开源,至少可以确保一些兜底的"回报"。
这些原因在1990年有意义,但我认为,世界已经变了,闭源软件已经不是主要问题所在。在2020年,计算自由的敌人是云计算软件(又称:软件即服务/SaaS,又称网络应用/Web Apps)—— 即主要在供应商的服务器上运行的软件,而你的所有数据也存储在这些服务器上。典型的例子包括:Google Docs、Trello、Slack、Figma、Notion和其他许多软件。
这些“云软件”也许有一个客户端组件(手机App,网页App,跑在你浏览器中的JavaScript),但它们只能与供应商的服务端共同工作。而云软件存在很多问题:
- 如果提供云软件的公司倒闭,或决定停产,软件就没法工作了,而你用这些软件创造的文档与数据就被锁死了。对于初创公司编写的软件来说,这是一个很常见的问题:这些公司可能会被大公司收购,而大公司没有兴趣继续维护这些初创公司的产品。
- 谷歌和其他云服务可能在没有任何警告和追索手段的情况下,突然暂停你的账户。例如,您可能在完全无辜的情况下,被自动化系统判定为违反服务条款:其他人可能入侵了你的账户,并在你不知情的情况下使用它来发送恶意软件或钓鱼邮件,触发违背服务条款。因而,你可能会突然发现自己用Google Docs或其它App创建的文档全部都被永久锁死,无法访问了。
- 而那些运行在你自己的电脑上的软件,即使软件供应商破产了,它也可以继续运行,直到永远。(如果软件不再与你的操作系统兼容,你也可以在虚拟机和模拟器中运行它,当然前提是它不需要联络服务器来检查许可证)。例如,互联网档案馆有一个超过10万个历史软件的软件集锦,你可以在浏览器中的模拟器里运行!相比之下,如果云软件被关闭,你没有办法保存它,因为你从来就没有服务端软件的副本,无论是源代码还是编译后的形式。
- 20世纪90年代,无法定制或扩展你所使用的软件的问题,在云软件中进一步加剧。对于在你自己的电脑上运行的闭源软件,至少有人可以对它的数据文件格式进行逆向工程,这样你还可以把它加载到其他的替代软件里(例如OOXML之前的微软Office文件格式,或者规范发布前的Photoshop文件)。有了云软件,甚至连这个都做不到了,因为数据只存储在云端,而不是你自己电脑上的文件。
如果所有的软件都是免费和开源的,这些问题就都解决了。然而,开源实际上并不是解决云软件问题的必要条件;即使是闭源软件也可以避免上述问题,只要它运行在你自己的电脑上,而不是供应商的云服务器上。请注意,互联网档案馆能够在没有源代码的情况下维持历史软件的正常运行:如果只是出于存档的目的,在模拟器中运行编译后的机器代码就够了。也许拥有源码会让事情更容易一些,但这并不是不关键,最重要的事情,还是要有一份软件的副本。
本地优先的软件
我和我的合作者们以前曾主张过本地优先软件的概念,这是对云软件的这些问题的一种回应。本地优先的软件在你自己的电脑上运行,将其数据存储在你的本地硬盘上,同时也保留了云计算软件的便利性,比如,实时协作,和在你所有的设备上同步数据。开源的本地优先的软件当然非常好,但这并不是必须的,本地优先软件90%的优点同样适用于闭源的软件。
云软件,而不是闭源软件,才是对软件自由的真正威胁,原因在于:云厂商能够突然心血来潮随心所欲地锁定你的所有数据,其危害要比无法查看和修改你的软件源码的危害大得多。因此,普及本地优先的软件显得更为重要和紧迫。如果在这一过程中,我们也能让更多的软件开放源代码,那也很不错,但这并没有那么关键。我们要聚焦在最重要与最紧迫的挑战上。
促进软件自由的法律工具
Copyleft软件许可证是一种法律工具,它试图迫使更多的软件供应商公开其源码。尤其是AGPL,它尝试迫使云厂商发布其服务器端软件的源代码。然而这并没有什么用:大多数云厂商只是简单拒绝使用AGPL许可的软件:要么使用一个采用更宽松许可的替代实现版本,要么自己重新实现必要的功能,或者直接购买一个没有版权限制的商业许可。有些代码无论如何都不会开放,我不认为这个许可证真的有让任何本来没开源的软件变开源。
作为一种促进软件自由的法律工具,我认为 copyleft 在很大程度上是失败的,因为它们在阻止云软件兴起上毫无建树,而且可能在促进开源软件份额增长上也没什么用。开源软件已经很成功了,但这种成功大部分都属于 non-copyleft 的项目(如Apache、MIT或BSD许可证),即使在GPL许可证的项目中(如Linux),我也怀疑版权方面是否真的是项目成功的重要因素。
对于促进软件自由而言,我相信更有前景的法律工具是政府监管。例如,GDPR提出了数据可移植权,这意味着用户必须可以能将他们的数据从一个服务转移到其它的服务中。现有的可移植性的实现,例如谷歌Takeout,是相当初级的(你真的能用一堆JSON压缩档案做点什么吗?),但我们可以游说监管机构推动更好的可移植性/互操作性,例如,要求相互竞争的两个供应商在它们的两个应用程序之间,实时双向同步你的数据。
另一条有希望的途径是,推动[公共部门的采购倾向于开源、本地优先的软件](https://joinup.ec.europa.eu/sites/default/files/document/2011-12/OSS-procurement-guideline -final.pdf),而不是闭源的云软件。这为企业开发和维护高质量的开源软件创造了积极的激励机制,而版权条款却没有这样做。
你可能会争论说,软件许可证是开发者个人可以控制的东西,而政府监管和公共政策是一个更大的问题,不在任何一个个体权力范围之内。是的,但你选择一个软件许可证能产生多大的影响?任何不喜欢你的许可证的人可以简单地选择不使用你的软件,在这种情况下,你的力量是零。有效的改变来自于对大问题的集体行动,而不是来自于一个人的小开源项目选择一种许可证而不是另一种。
GPL-家族许可证的其他问题
你可以强迫一家公司提供他们的GPL衍生软件项目的源码,但你不能强迫他们成为开源社区的好公民(例如,持续维护它们添加的功能特性、修复错误、帮助其他贡献者、提供良好的文档、参与项目管理)。如果它们没有真正参与开源项目,那么这些 “扔到你面前 “的源代码又有什么用?最好情况下,它没有价值;最坏的情况下,它还是有害的,因为它把维护的负担转嫁给了项目的其他贡献者。
我们需要人们成为优秀的开源社区贡献者,而这是通过保持开放欢迎的态度,建立正确的激励机制来实现的,而不是通过软件许可证。
最后,GPL许可证家族在实际使用中的一个问题是,它们与其他广泛使用的许可证不兼容,这使得在同一个项目中使用某些库的组合变得更为困难,且不必要地分裂了开源生态。如果GPL许可证有其他强大的优势,也许这个问题还值得忍受。但正如上面所述,我不认为这些优势存在。
结论
GPL和其他 copyleft 许可证并不坏,我只是认为它们毫无意义。它们有实际问题,而且被FSF的行为所玷污;但最重要的是,我不认为它们对软件自由做出了有效贡献。现在唯一真正在用 copyleft 的商业软件厂商(MongoDB, Elastic) —— 它们想阻止亚马逊将其软件作为服务提供,这当然很好,但这纯粹是出于商业上的考虑,而不是软件自由。
开源软件已经取得了巨大的成功,自由软件运动源于1990年代的反微软情绪,它已经走过了很长的路。我承认自由软件基金会对这一切的开始起到了重要作用。然而30年过去了,生态已经发生了变化,而自由软件基金会却没有跟上,而且变得越来越不合群。它没能对云软件和其他最近对软件自由的威胁做出清晰的回应,只是继续重复着几十年前的老论调。现在,通过恢复Stallman的地位和驳回对他的关注,FSF正在积极地伤害自由软件的事业。我们必须与FSF和他们的世界观保持距离。
基于所有这些原因,我认为抓着GPL和 copyleft 已经没有意义了,放手吧。相反,我会鼓励你为你的项目采用一种宽容的许可协议(例如MIT, BSD, Apache 2.0),然后把你的精力放在真正能对软件自由产生影响的事情上。抵制云软件的垄断效应,发展可持续的商业模式,让开源软件茁壮成长,并推动监管,将软件用户的利益置于供应商的利益之上。
- 感谢Rob McQueen对本帖草稿的反馈。
参考文献
- RMS官复原职:(https://www.fsf.org/news/statement-of-fsf-board-on-election-of-richard-stallman
- 自由软件基金会主页:https://www.fsf.org/
- 弹劾RMS的公开信:https://rms-open-letter.github.io/
- GNU项目声明:https://www.gnu.org/gnu/incorrect-quotation.en.html
- GNU通用公共许可证 https://en.wikipedia.org/wiki/GNU_General_Public_License
- copyleft: https://en.wikipedia.org/wiki/Copyleft
- 衍生作品的定义:https://en.wikipedia.org/wiki/Derivative_work
- x.ai被Bizzabo收购:https://ourincrediblejourney.tumblr.com/
- Google Account Suspended No Reason Given:https://www.paullimitless.com/google-account-suspended-no-reason-given/
- Google暂停用户账户:https://twitter.com/Demilogic/status/1358661840402845696
- 互联网历史软件归档:https://archive.org/details/softwarelibrary
- Office Open XML:https://en.wikipedia.org/wiki/Office_Open_XML
- Photoshop File Formats Specification:https://www.adobe.com/devnet-apps/photoshop/fileformatashtml/
- 本地优先软件:https://www.inkandswitch.com/local-first.html
- AGPL协议:https://en.wikipedia.org/wiki/Affero_General_Public_License
- Elastic商业许可证:https://www.elastic.co/cn/pricing/faq/licensing
- 数据可移植权:https://ico.org.uk/for-organisations/guide-to-data-protection/guide-to-the-general-data-protection-regulation-gdpr/individual-rights/right-to-data-portability/
- 谷歌Takeout(带走你的数据):https://en.wikipedia.org/wiki/Google_Takeout
- 互操作性新闻:https://interoperability.news/
- 欧盟开源软件采购指南:https://joinup.ec.europa.eu/sites/default/files/document/2011-12/OSS-procurement-guideline%20-final.pdf
- 许可证兼容性:https://gplv3.fsf.org/wiki/index.php/Compatible_licenses
- MongoDB SSPL协议FAQ:https://gplv3.fsf.org/wiki/index.php/Compatible_licenses
- Elastic许可变更问题汇总:https://gplv3.fsf.org/wiki/index.php/Compatible_licenses
- “自由软件”:一个过时的想法:https://r0ml.medium.com/free-software-an-idea-whose-time-has-passed-6570c1d8218a
- 一条FSF未曾设想的路:https://lu.is/blog/2021/04/07/values-centered-npos-with-kmaher/
容器化数据库是个好主意吗?
前言:这篇文章是19年1月写的,四年过去了,涉及到数据库与容器的利弊权衡依然成立。这里进行细微调整后重新发出。明天我会发布一篇《数据库是否应当放入K8S中?》,那么今天就先用这篇老文来预热一下吧。
对于无状态的应用服务而言,容器是一个相当完美的开发运维解决方案。然而对于带持久状态的服务 —— 数据库来说,事情就没有那么简单了。生产环境的数据库是否应当放入容器中,仍然是一个充满争议的问题。
站在开发者的角度上,我非常喜欢Docker,并相信容器也许是未来软件开发部署运维的标准方式。但站在DBA的立场上,我认为就目前而言,将生产环境数据库放入Docker / K8S 中仍然是一个馊主意。
Docker解决什么问题?
让我们先来看一看Docker对自己的描述。
Docker用于形容自己的词汇包括:轻量,标准化,可移植,节约成本,提高效率,自动,集成,高效运维。这些说法并没有问题,Docker在整体意义上确实让开发和运维都变得更容易了。因而可以看到很多公司都热切地希望将自己的软件与服务容器化。但有时候这种热情会走向另一个极端:将一切软件服务都容器化,甚至是生产环境的数据库。
容器最初是针对无状态的应用而设计的,在逻辑上,容器内应用产生的临时数据也属于该容器的一部分。用容器创建起一个服务,用完之后销毁它。这些应用本身没有状态,状态通常保存在容器外部的数据库里,这是经典的架构与用法,也是容器的设计哲学。
但当用户想把数据库本身也放到容器中时,事情就变得不一样了:数据库是有状态的,为了维持这个状态不随容器停止而销毁,数据库容器需要在容器上打一个洞,与底层操作系统上的数据卷相联通。这样的容器,不再是一个能够随意创建,销毁,搬运,转移的对象,而是与底层环境相绑定的对象。因此,传统应用使用容器的诸多优势,对于数据库容器来说都不复存在。
可靠性
让软件跑起来,和让软件可靠地运行是两回事。数据库是信息系统的核心,在绝大多数场景下属于**关键(Critical)**应用,Critical Application可按字面解释,就是出了问题会要命的应用。这与我们的日常经验相符:Word/Excel/PPT这些办公软件如果崩了强制重启即可,没什么大不了的;但正在编辑的文档如果丢了、脏了、乱了,那才是真的灾难。数据库亦然,对于不少公司,特别是互联网公司来说,如果数据库被删了又没有可用备份,基本上可以宣告关门大吉了。
可靠性(Reliability)是数据库最重要的属性。可靠性是系统在困境(adversity)(硬件故障、软件故障、人为错误)中仍可正常工作(正确完成功能,并能达到期望的性能水准)的能力。可靠性意味着容错(fault-tolerant)与韧性(resilient),它是一种安全属性,并不像性能与可维护性那样的活性属性直观可衡量。它只能通过长时间的正常运行来证明,或者某一次故障来否证。很多人往往会在平时忽视安全属性,而在生病后,车祸后,被抢劫后才追悔莫及。安全生产重于泰山,数据库被删,被搅乱,被脱库后再捶胸顿足是没有意义的。
回头再看一看Docker对自己的特性描述中,并没有包含“可靠”这个对于数据库至关重要的属性。
可靠性证明与社区知识
如前所述,可靠性并没有一个很好的衡量方式。只有通过长时间的正确运行,我们才能对一个系统的可靠性逐渐建立信心。在裸机上部署数据库可谓自古以来的实践,通过几十年的持续工作,它很好的证明了自己的可靠性。Docker虽为DevOps带来一场革命,但仅仅五年的历史对于可靠性证明而言仍然是图样图森破。对关乎身家性命的生产数据库而言还远远不够:因为还没有足够的小白鼠去趟雷。
想要提高可靠性,最重要的就是从故障中吸取经验。故障是宝贵的经验财富:它将未知问题变为已知问题,是运维知识的表现形式。社区的故障经验绝大多都基于裸机部署的假设,各式各样的故障在几十年里都已经被人们踩了个遍。如果你遇到一些问题,大概率是别人已经踩过的坑,可以比较方便地处理与解决。同样的故障如果加上一个“Docker”关键字,能找到的有用信息就要少的多。这也意味着当疑难杂症出现时,成功抢救恢复数据的概率要更低,处理紧急故障所需的时间会更长。
微妙的现实是,如果没有特殊理由,企业与个人通常并不愿意分享故障方面的经验。故障有损企业的声誉:可能暴露一些敏感信息,或者是企业与团队的垃圾程度。另一方面,故障经验几乎都是真金白银的损失与学费换来的,是运维人员的核心价值所在,因此有关故障方面的公开资料并不多。
额外失效点
开发关心Feature,而运维关注Bug。相比裸机部署而言,将数据库放入Docker中并不能降低硬件故障、软件错误、人为失误的发生概率。用裸机会有的硬件故障,用Docker一个也不会少。软件缺陷主要是应用Bug,也不会因为采用容器与否而降低,人为失误同理。相反,引入Docker会因为引入了额外的组件,额外的复杂度,额外的失效点,导致系统整体可靠性下降。
举个最简单的例子,dockerd守护进程崩了怎么办,数据库进程就直接歇菜了。尽管这种事情发生的概率并不高,但它们在裸机上 —— 压根不会发生。
此外,一个额外组件引入的失效点可能并不止一个:Docker产生的问题并不仅仅是Docker本身的问题。当故障发生时,可能是单纯Docker的问题,或者是Docker与数据库相互作用产生的问题,还可能是Docker与操作系统,编排系统,虚拟机,网络,磁盘相互作用产生的问题。可以参见官方PostgreSQL Docker镜像的Issue列表:https://github.com/docker-library/postgres/issues?q=。
正如《从降本增笑到降本增效》中所说,智力功率很难在空间上累加 —— 团队的智力功率往往取决于最资深几个灵魂人物的水平以及他们的沟通成本。当数据库出现问题时需要数据库专家来解决;当容器出现问题时需要容器专家来看问题;然而当你把数据库放入 Kubernetes 时,单独的数据库专家和 K8S 专家的智力带宽是很难叠加的 —— 你需要一个双料专家才能解决问题。而同时精通这两者的软件肯定要比单独的数据库专家少得多。
此外,彼之蜜糖,吾之砒霜。某些Docker的Feature,在特定的环境下也可能会变为Bug。
隔离性
Docker提供了进程级别的隔离性,通常来说隔离性对应用来说是个好属性。应用看不见别的进程,自然也不会有很多相互作用导致的问题,进而提高了系统的可靠性。但隔离性对于数据库而言不一定完全是好事。
一个微妙的真实案例是在同一个数据目录上启动两个PostgreSQL实例,或者在宿主机和容器内同时启动了两个数据库实例。在裸机上第二次启动尝试会失败,因为PostgreSQL能意识到另一个实例的存在而拒绝启动;但在使用Docker的情况下因其隔离性,第二个实例无法意识到宿主机或其他数据库容器中的另一个实例。如果没有配置合理的Fencing机制(例如通过宿主机端口互斥,pid文件互斥),两个运行在同一数据目录上的数据库进程能把数据文件搅成一团浆糊。
数据库需不需要隔离性?当然需要, 但不是这种隔离性。数据库的性能很重要,因此往往是独占物理机部署。除了数据库进程和必要的工具,不会有其他应用。即使放在容器中,也往往采用独占绑定物理机的模式运行。因此Docker提供的隔离性对于这种数据库部署方案而言并没有什么意义;不过对云数据库厂商来说,这倒真是一个实用的Feature,用来搞多租户超卖妙用无穷。
工具
数据库需要工具来维护,包括各式各样的运维脚本,部署,备份,归档,故障切换,大小版本升级,插件安装,连接池,性能分析,监控,调优,巡检,修复。这些工具,也大多针对裸机部署而设计。这些工具与数据库一样,都需要精心而充分的测试。让一个东西跑起来,与确信这个东西能持久稳定正确的运行,是完全不同的可靠性水准。
一个简单的例子是插件与包管理,PostgreSQL提供了很多实用的插件,譬如PostGIS。假如想为数据库安装该插件,在裸机上只要yum install
然后create extension postgis
两条命令就可以。但如果是在Docker里,按照Docker的实践原则,用户需要在镜像层次进行这个变更,否则下次容器重启时这个扩展就没了。因而需要修改Dockerfile,重新构建新镜像并推送到服务器上,最后重启数据库容器,毫无疑问,要麻烦的多。
包管理是操作系统发行版的核心问题。然而 Docker 搅乱了这一切,例如,许多 PostgreSQL 不再以 RPM/DEB 包的形式发布二进制,而是以加装扩展的 Postgres Docker 镜像分发。这就会立即产生一个显著的问题,如果我想同时使用两种,三种,或者PG生态的一百多种扩展,那么应该如何把这些散碎的镜像整合到一起呢?相比可靠的操作系统包管理,构建Docker镜像总是需要耗费更多时间与精力才能正常起效。
再比如说监控,在传统的裸机部署模式下,机器的各项指标是数据库指标的重要组成部分。容器中的监控与裸机上的监控有很多微妙的区别。不注意可能会掉到坑里。例如,CPU各种模式的时长之和,在裸机上始终会是100%,但这样的假设在容器中就不一定总是成立了。再比方说依赖/proc
文件系统的监控程序可能在容器中获得与裸机上涵义完全不同的指标。虽然这类问题最终都是可解的(例如把Proc文件系统挂载到容器内),但相比简洁明了的方案,没人喜欢复杂丑陋的work around。
类似的问题包括一些故障检测工具与系统常用命令,虽然理论上可以直接在宿主机上执行,但谁能保证容器里的结果和裸机上的结果有着相同的涵义?更为棘手的是紧急故障处理时,一些需要临时安装使用的工具在容器里没有,外网不通,如果再走Dockerfile→Image→重启这种路径毫无疑问会让人抓狂。
把Docker当成虚拟机来用的话,很多工具大抵上还是可以正常工作的,不过这样就丧失了使用的Docker的大部分意义,不过是把它当成了另一个包管理器用而已。有人觉得Docker通过标准化的部署方式增加了系统的可靠性,因为环境更为标准化更为可控。这一点不能否认。私以为,标准化的部署方式虽然很不错,但如果运维管理数据库的人本身了解如何配置数据库环境,将环境初始化命令写在Shell脚本里和写在Dockerfile里并没有本质上的区别。
可维护性
软件的大部分开销并不在最初的开发阶段,而是在持续的维护阶段,包括修复漏洞、保持系统正常运行、处理故障、版本升级,偿还技术债、添加新的功能等等。可维护性对于运维人员的工作生活质量非常重要。应该说可维护性是Docker最讨喜的地方:Infrastructure as code。可以认为Docker的最大价值就在于它能够把软件的运维经验沉淀成可复用的代码,以一种简便的方式积累起来,而不再是散落在各个角落的install/setup文档。在这一点上Docker做的相当出色,尤其是对于逻辑经常变化的无状态应用而言。Docker和K8s能让用户轻松部署,完成扩容,缩容,发布,滚动升级等工作,让Dev也能干Ops的活,让Ops也能干DBA的活(迫真)。
环境配置
如果说Docker最大的优点是什么,那也许就是环境配置的标准化了。标准化的环境有助于交付变更,交流问题,复现Bug。使用二进制镜像(本质是物化了的Dockerfile安装脚本)相比执行安装脚本而言更为快捷,管理更方便。一些编译复杂,依赖如山的扩展也不用每次都重新构建了,这些都是很不错的特性。
不幸的是,数据库并不像通常的业务应用一样来来去去更新频繁,创建新实例或者交付环境本身是一个极低频的操作。同时DBA们通常都会积累下各种安装配置维护脚本,一键配置环境也并不会比Docker慢多少。因此在环境配置上Docker的优势就没有那么显著了,只能说是 Nice to have。当然,在没有专职DBA时,使用Docker镜像可能还是要比自己瞎折腾要好一些,因为起码镜像中多少沉淀了一些运维经验。
通常来说,数据库初始化之后连续运行几个月几年也并不稀奇。占据数据库管理工作主要内容的并不是创建新实例与交付环境,主要还是日常运维的部分 —— Day2 Operation。不幸的是,在这一点上Docker并没有什么优势,反而会产生不少的额外麻烦。
Day2 Operation
Docker确实能极大地简化来无状态应用的日常维护工作,诸如创建销毁,版本升级,扩容等,但同样的结论能延伸到数据库上吗?
数据库容器不可能像应用容器一样随意销毁创建,重启迁移。因而Docker并不能对数据库的日常运维的体验有什么提升,真正有帮助的倒是诸如 ansible 之类的工具。而对于日常运维而言,很多操作都需要通过docker exec
的方式将脚本透传至容器内执行。底下跑的还是一样的脚本,只不过用docker-exec
来执行又额外多了一层包装,这就有点脱裤子放屁的意味了。
此外,很多命令行工具在和Docker配合使用时都相当尴尬。譬如docker exec
会将stderr
和stdout
混在一起,让很多依赖管道的命令无法正常工作。以PostgreSQL为例,在裸机部署模式下,某些日常ETL任务可以用一行bash轻松搞定:
psql <src-url> -c 'COPY tbl TO STDOUT' |\
psql <dst-url> -c 'COPY tdb FROM STDIN'
但如果宿主机上没有合适的客户端二进制程序,那就只能这样用Docker容器中的二进制:
docker exec -it srcpg gosu postgres bash -c "psql -c \"COPY tbl TO STDOUT\" 2>/dev/null" |\ docker exec -i dstpg gosu postgres psql -c 'COPY tbl FROM STDIN;'
当用户想为容器里的数据库做一个物理备份时,原本很简单的一条命令现在需要很多额外的包装:docker
套gosu
套bash
套pg_basebackup
:
docker exec -i postgres_pg_1 gosu postgres bash -c 'pg_basebackup -Xf -Ft -c fast -D - 2>/dev/null' | tar -xC /tmp/backup/basebackup
如果说客户端应用psql|pg_basebackup|pg_dump
还可以通过在宿主机上安装对应版本的客户端工具来绕开这个问题,那么服务端的应用就真的无解了。总不能在不断升级容器内数据库软件的版本时每次都一并把宿主机上的服务器端二进制版本升级了吧?
另一个Docker喜欢讲的例子是软件版本升级:例如用Docker升级数据库小版本,只要简单地修改Dockerfile里的版本号,重新构建镜像然后重启数据库容器就可以了。没错,至少对于无状态的应用来说这是成立的。但当需要进行数据库原地大版本升级时问题就来了,用户还需要同时修改数据库状态。在裸机上一行bash命令就可以解决的问题,在Docker下可能就会变成这样的东西:https://github.com/tianon/docker-postgres-upgrade。
如果数据库容器不能像AppServer一样随意地调度,快速地扩展,也无法在初始配置,日常运维,以及紧急故障处理时相比普通脚本的方式带来更多便利性,我们又为什么要把生产环境的数据库塞进容器里呢?
Docker和K8s一个很讨喜的地方是很容易进行扩容,至少对于无状态的应用而言是这样:一键拉起起几个新容器,随意调度到哪个节点都无所谓。但数据库不一样,作为一个有状态的应用,数据库并不能像普通AppServer一样随意创建,销毁,水平扩展。譬如,用户创建一个新从库,即使使用容器,也得从主库上重新拉取基础备份。生产环境中动辄几TB的数据库,创建副本也需要个把钟头才能完成,也需要人工介入与检查,并逐渐放量预热缓存才能上线承载流量。相比之下,在同样的操作系统初始环境下,运行现成的拉从库脚本与跑docker run
在本质上又能有什么区别 —— 时间都花在拖从库上了。
使用Docker承放生产数据库的一个尴尬之处就在于,数据库是有状态的,而且为了建立这个状态需要额外的工序。通常来说设置一个新PostgreSQL从库的流程是,先通过pg_basebackup
建立本地的数据目录副本,然后再在本地数据目录上启动postmaster
进程。然而容器是和进程绑定的,一旦进程退出容器也随之停止。因此为了在Docker中扩容一个新从库:要么需要先后启动pg_basebackup
容器拉取数据目录,再在同一个数据卷上启动postgres
两个容器;要么需要在创建容器的过程中就指定好复制目标并等待几个小时的复制完成;要么在postgres
容器中再使用pg_basebackup
偷天换日替换数据目录。无论哪一种方案都是既不优雅也不简洁。因为容器的这种进程隔离抽象,对于数据库这种充满状态的多进程,多任务,多实例协作的应用存在抽象泄漏,它很难优雅地覆盖这些场景。当然有很多折衷的办法可以打补丁来解决这类问题,然而其代价就是大量非本征复杂度,最终受伤的还是系统的可维护性。
总的来说,Docker 在某些层面上可以提高系统的可维护性,比如简化创建新实例的操作,但它引入的新麻烦让这样的优势显得苍白无力。
性能
性能也是人们经常关注的一个维度。从性能的角度来看,数据库的基本部署原则当然是离硬件越近越好,额外的隔离与抽象不利于数据库的性能:越多的隔离意味着越多的开销,即使只是内核栈中的额外拷贝。对于追求性能的场景,一些数据库选择绕开操作系统的页面管理机制直接操作磁盘,而一些数据库甚至会使用FPGA甚至GPU加速查询处理。
实事求是地讲,Docker作为一种轻量化的容器,性能上的折损并不大,通常不会超过 10% 。但毫无疑问的是,将数据库放入Docker只会让性能变得更差而不是更好。
总结
容器技术与编排技术对于运维而言是非常有价值的东西,它实际上弥补了从软件到服务之间的空白,其愿景是将运维的经验与能力代码化模块化。容器技术将成为未来的包管理方式,而编排技术将进一步发展为“数据中心分布式集群操作系统”,成为一切软件的底层基础设施Runtime。当越来越多的坑被踩完后,人们可以放心大胆的把一切应用,有状态的还是无状态的都放到容器中去运行。但现在起码对于数据库而言,还只是一个美好的愿景与鸡肋的选项。
需要再次强调的是,以上讨论仅限于生产环境数据库。对于开发测试而言,尽管有基于Vagrant的虚拟机沙箱,但我也支持使用Docker —— 毕竟不是所有的开发人员都知道怎么配置本地测试数据库环境,使用Docker交付环境显然要比一堆手册简单明了的多。对于生产环境的无状态应用,甚至一些带有衍生状态的不甚重要衍生数据系统(譬如Redis缓存),Docker也是一个不错的选择。但对于生产环境的核心关系型数据库而言,如果里面的数据真的很重要,使用Docker前还是需要三思:这样做的价值到底在哪里?出了疑难杂症能Hold住吗?搞砸了这锅背的动吗?
任何技术决策都是一个利弊权衡的过程,譬如这里使用Docker的核心权衡可能就是牺牲可靠性换取可维护性。确实有一些场景,数据可靠性并不是那么重要,或者说有其他的考量:譬如对于云计算厂商来说,把数据库放到容器里混部超卖就是一件很好的事情:容器的隔离性,高资源利用率,以及管理上的便利性都与该场景十分契合。这种情况下将数据库放入Docker中,也许对他们而言就是利大于弊的。但对于更多的场景来说,可靠性往往都是优先级最高的的属性,牺牲可靠性换取可维护性通常并不是一个可取的选择。更何况也很难说运维管理数据库的工作,会因为用了Docker而轻松多少:为了安装部署一次性的便利而牺牲长久的日常运维可维护性并不是一个好主意。
综上所述,将生产环境的数据库放入容器中恐怕并不是一个明智的选择。
理解时间:闰年闰秒,时间与时区
前几天出现了四年一遇的闰年 2月29号,每到这一天,总会有一些土鳖软件出现大翻车。这种问题如果运气不好,可能要等上四年才会暴露出来。 比如今天新鲜出炉的:禾赛科技激光雷达和新西兰加油站都因为闰年Bug无法使用。
聊一聊闰年,闰秒,时间与时区的原理,以及在数据库与编程语言中的注意事项。
0x01 秒与计时
时间的单位是秒,但秒的定义并不是一成不变的。它有一个天文学定义,也有一个物理学定义。
世界时(UT1)
在最开始,秒的定义来源于日。秒被定义为平均太阳日的1/86400。而太阳日,则是由天文学现象定义的:两次连续正午时分的间隔被定义为一个太阳日;一天有86400秒,一秒等于86400分之一天,Perfect!以这一标准形成的时间标准,就称为世界时(Univeral Time, UT1),或不严谨的说,格林威治标准时(Greenwich Mean Time, GMT),下面就用GMT来指代它了。
这个定义很直观,但有一个问题:它是基于天文学现象的,即地球与太阳的周期性运动。不论是用地球的公转还是自转来定义秒,都有一个很尴尬的地方:虽然地球自转与公转的变化速度很慢,但并不是恒常的,譬如:地球的自转越来越慢,而地月位置也导致了每天的时长其实都不完全相同。这意味着作为物理基本单位的秒,其时长竟然是变化的。在衡量时间段的长短上就比较尴尬,几十年的一秒可能和今天的一秒长度已经不是一回事了。
原子时(TAI)
为了解决这个问题,在1967年之后,秒的定义变成了:铯133原子基态的两个超精细能级间跃迁对应辐射的9,192,631,770个周期的持续时间。秒的定义从天文学定义升级成为了物理学定义,其描述由相对易变的天文现象升级到了更稳定的宇宙中的基本物理事实。现在我们有了真正精准的秒啦:一亿年的偏差也不超过一秒。
当然,这么精确的秒除了用来衡量时间间隔,也可以用来计时。从1958-01-01 00:00:00
开始作为公共时间原点,国际原子钟开始了计数,每计数9,192,631,770这么多个原子能级跃迁周期就+1s,这个钟走的非常准,每一秒都很均匀。使用这定义的时间称为国际原子时(International Atomic Time, TAI),下文简称TAI。
冲突
在最开始,这两种秒是等价的:一天是 86400 天文秒,也等于 86400 物理秒,毕竟物理学这个定义就是特意去凑天文学的定义嘛。所以相应的,GMT也与国际原子时TAI也保持着同步。然而正如前面所说,天文学现象影响因素太多了,并不是真正的“天行有常”。随着地球自转公转速度变化,天文定义的秒要比物理定义的秒稍微长了那么一点点,这也就意味着GMT要比TAI稍微落后一点点。
那么哪种定义说了算,世界时还是原子时?如果理论与生活实践经验相违背,绝大多数人都不会选择反直觉的方案:假设一种极端场景,两个钟之间的差异日积月累,到最后出现了几分钟甚至几小时的差值:明明日当午,按GMT应当是12:00:00
,但GMT走慢了,TAI显示的时间已经是晚上六点了,这就违背了直觉。在表示时刻这一点上,还是由天文定义说了算,即以GMT为准。
当然,就算是天文定义说了算,也要尊重物理规律,毕竟原子钟走的这么准不是?实际上世界时与原子时之间的差值也就在几秒的量级。那么我们会自然而然地想到,使用国际原子时TAI作为基准,但加上一些闰秒(leap second)修正到GMT不就行了?既有高精度,又符合常识。于是就有了新的协调世界时(Coordinated Universal Time, UTC)。
协调世界时(UTC)
UTC是调和GMT与TAI的产物:
-
UTC使用精确的国际原子时TAI作为计时基础
-
UTC使用国际时GMT作为修正目标
-
UTC使用闰秒作为修正手段,
我们通常所说的时间,通常就是指世界协调时间UTC,它与世界时GMT的差值在0.9秒内,在要求不严格的实践中,可以近似认为UTC时间与GMT时间是相同的,很多人也把它与GMT混为一谈。
但问题紧接着就来了,按照传统,一天24小时,一小时60分钟,一分钟60秒,日和秒之间有86400的换算关系。以前用日来定义秒,现在秒成了基本单位,就要用秒去定义日。但现在一天不等于86400秒了。无论用哪头定义哪头,都会顾此失彼。唯一的办法,就是打破这种传统:一分钟不一定只有60秒了,它在需要的时候可以有61秒!
这就是闰秒机制,UTC以TAI为基准,因此走的也比GMT快。假设UTC和GMT的差异不断变大,在即将超过一秒时,让UTC中的某一分钟变为61秒,续的这一秒就像UTC在等GMT一样,然后误差就追回来了。每次续一秒时,UTC时间都会落后TAI多一秒,截止至今,UTC已经落后TAI三十多秒了。最近的一次闰秒调整是在2016年跨年:
国际标准时间UTC将在格林尼治时间2016年12月31日23时59分59秒(北京时间2017年1月1日7时59分59秒)之后,在原子时钟实施一个正闰秒,即增加1秒,然后才会跨入新的一年。
所以说,GMT和UTC还是有区别的,UTC里你能看到2016-12-31 23:59:60
的时间,但GMT里就不会。
0x02 本地时间与时区
刚才讨论的时间都默认了一个前提:位于本初子午线(0度经线)上的时间。我们还需要考虑地球上的其他地方:毕竟美帝艳阳高照时,中国还在午夜呢。
本地时间,顾名思义就是以当地的太阳来计算的时间:正午就是12:00。太阳东升西落,东经120度上的本地时间比起本初子午线上就早了120° / (360°/24) = 8
个小时。这意味着在北京当地时间12点整时,UTC时间其实是12-8=4
,早晨4:00。
大家统一用UTC时间好不好呢?可以当然可以,毕竟中国横跨三个时区,也只用了一个北京时间。只要大家习惯就行。但大家都已经习惯了本地正午算12点了,强迫全世界人民用统一的时间其实违背了历史习惯。时区的设置使得长途旅行者能够简单地知道当地人的作息时间:反正差不多都是朝九晚五上班。这就降低了沟通成本。于是就有了时区的概念。当然像新疆这种硬要用北京时间的结果就是,游客乍一看当地人11点12点才上班可能会有些懵。
但在大一统的国家内部,使用统一的时间也有助于降低沟通成本。假如一个新疆人和一个黑龙江人打电话,一个用的乌鲁木齐时间,一个用的北京时间,那就会鸡同鸭讲。都约着12点,结果实际差了两个小时。时区的选用并不完全是按照地理经度而来的,也有很多的其他因素考量(例如行政区划)。
这就引出了时区的概念:时区是地球上使用同一个本地时间定义的区域。时区实际上可以视作从地理区域到时间偏移量的单射。
但其实有没有那个地理区域都不重要,关键在于时间偏移量的概念。UTC/GMT时间本身的偏移量为0,时区的偏移量都是相对于UTC时间而言的。这里,本地时间,UTC时间与时区的关系是:
本地时间 = UTC时间 + 本地时区偏移量。
比如UTC、GMT的时区都是+0
,意味着没有偏移量。中国所处的东八区偏移量就是+8
。意味着计算当地时间时,要在UTC时间的基础上增加8个小时。
夏令时(Daylight Saving Time, DST),可以视为一种特殊的时区偏移修正。指的是在夏天天亮的较早的时候把时间调快一个小时(实际上不一定是一个小时),从而节省能源(灯火)。我国在86年到92年之间曾短暂使用过夏令时。欧盟从1996年开始使用夏令时,不过欧盟最近的民调显示,84%的民众希望取消夏令时。对程序员而言,夏令时也是一个额外的麻烦事,希望它能尽快被扫入历史的垃圾桶。
0x03 时间的表示
那么,时间又如何表示呢?使用TAI的秒数来表示时间当然不会有歧义,但使用不便。习惯上我们将时间分为三个部分:日期,时间,时区,而每个部分都有多种表示方法。对于时间的表示,世界诸国人民各有各的习惯,例如,2006年1月2日,美国人就可能喜欢使用诸如January 2, 1999
,1/2/1999
这样的日期表示形式,而中国人也许会用诸如“2006年1月2日”,“2006/01/02”这样的表示形式。发送邮件时,首部中的时间则采用RFC2822中规定的Sat, 24 Nov 2035 11:45:15 −0500
格式。此外,还有一系列的RFC与标准,用于指定日期与时间的表示格式。
ANSIC = "Mon Jan _2 15:04:05 2006"
UnixDate = "Mon Jan _2 15:04:05 MST 2006"
RubyDate = "Mon Jan 02 15:04:05 -0700 2006"
RFC822 = "02 Jan 06 15:04 MST"
RFC822Z = "02 Jan 06 15:04 -0700" // RFC822 with numeric zone
RFC850 = "Monday, 02-Jan-06 15:04:05 MST"
RFC1123 = "Mon, 02 Jan 2006 15:04:05 MST"
RFC1123Z = "Mon, 02 Jan 2006 15:04:05 -0700" // RFC1123 with numeric zone
RFC3339 = "2006-01-02T15:04:05Z07:00"
RFC3339Nano = "2006-01-02T15:04:05.999999999Z07:00"
不过在这里,我们只关注计算机中的日期表示形式与存储方式。而计算机中,时间最经典的表示形式,就是Unix时间戳。
Unix时间戳
比起UTC/GMT,对于程序员来说,更为熟悉的可能是另一种时间:Unix时间戳。UNIX时间戳是从1970年1月1日(UTC/GMT的午夜,在1972年之前没有闰秒)开始所经过的秒数,注意这里的秒其实是GMT中的秒,也就是不计闰秒,毕竟一天等于86400秒已经写死到无数程序的逻辑里去了,想改是不可能改的。
使用GMT秒数的好处是,计算日期的时候根本不用考虑闰秒的问题。毕竟闰年已经很讨厌了,再来一个没有规律的闰秒,绝对会让程序员抓狂。当然这不代表就不需要考虑闰秒的问题了,诸如ntp等时间服务还是需要考虑闰秒的问题的,应用程序有可能会受到影响:比如遇到‘时光倒流’拿到两次59
秒,或者获取到秒数为60
的时间值,一些实现简陋的程序可能就直接崩了。当然,也有一种将闰秒均摊到某一天全天的顺滑手段。
Unix时间戳背后的思想很简单,建立一条时间轴,以某一个纪元点(Epoch)作为原点,将时间表示为距离原点的秒数。Unix时间戳的纪元为GMT时间的1970-01-01 00:00:00
,32位系统上的时间戳实际上是一个有符号四字节整型,以秒为单位。这意味它能表示的时间范围为:2^32 / 86400 / 365 = 68
年,差不多从1901年到2038年。
当然,时间戳并不是只有这一种表示方法,但通常这是最为传统稳妥可靠的做法。毕竟不是所有的程序员都能处理好许多和时区、闰秒相关的微妙错误。使用Unix时间戳的好处就是时区已经固定死了是GMT了,存储空间与某些计算处理(比如排序)也相对容易。
在*nix命令行中使用date +%s
可以获取Unix时间戳。而date -r @1500000000
则可以反向将Unix时间戳转换为其他时间格式,例如转换为2017-07-14 10:40:00
可以使用:
date -d @1500000000 '+%Y-%m-%d %H:%M:%S' # Linux
date -r 1500000000 '+%Y-%m-%d %H:%M:%S' # MacOS, BSD
在很久以前,当主板上的电池没电之后,系统的时钟就会自动重置成0;还有很多软件的Bug也会导致导致时间戳为0,也就是1970-01-01
;以至于这个纪元时间很多非程序员都知道了。
当然,4字节 Unix 时间戳的上限 2038 年离今天 (2024) 年已经不是遥不可及了,还没及时改成 8 字节时间戳的软件到时候就要面临比闰天加油站罢工严峻得多的千年虫问题 —— 直接罢工了,比如直到今天还没有改过来的二傻子 MySQL 。
PostgreSQL中的时间存储
通常情况下,Unix时间戳是传递/存储时间的最佳方式,它通常在计算机内部以整型的形式存在,内容为距离某个特定纪元的秒数。它极为简单,无歧义,存储占用更紧实,便于比较大小,且在程序员之间存在广泛共识。不过,Epoch+整数偏移量的方式适合在机器上进行存储与交换,但它并不是一种人类可读的格式(也许有些程序员可读)。
PostgreSQL 提供了丰富的日期时间数据类型与相关函数,它能以高度灵活的方式自动适配各种格式的时间输入输出,并在内部以高效的整型表示进行存储与计算。在PostgreSQL中,变量CURRENT_TIMESTAMP
或函数now()
会返回当前事务开始时的本地时间戳,返回的类型是TIMESTAMP WITH TIME ZONE
,这是一个PostgreSQL扩展,会在时间戳上带有额外的时区信息。SQL标准所规定的类型为TIMESTAMP
,在PostgreSQL中使用8字节的长整型实现。可以使用SQL语法AT TIME ZONE zone
或内置函数timezone(zone,ts)
将带有时区的TIMESTAMP
转换为不带时区的标准版本。
通常最佳实践是,只要应用稍具规模或涉及到任何国际化的功能,要么按照PostgreSQL Wiki中推荐的最佳实践 使用PostgreSQL 自己提供的 TimestampTZ
扩展类型,要么使用 TIMESTAMP
类型并固定存储 GMT / UTC 时间。
PostgreSQL 的时间戳实现用的是 8 字节,表示的时间范围从公元前 4713 年到 29万年后,精度为1微秒,完全不用担心 2038 千年虫问题。
-- 获取本地事务开始时的时间戳
vonng=# SELECT now(), CURRENT_TIMESTAMP;
now | current_timestamp
-------------------------------+-------------------------------
2018-12-11 21:50:15.317141+08 | 2018-12-11 21:50:15.317141+08
-- now()/CURRENT_TIMESTAMP返回的是带有时区信息的时间戳
vonng=# SELECT pg_typeof(now()),pg_typeof(CURRENT_TIMESTAMP);
pg_typeof | pg_typeof
--------------------------+--------------------------
timestamp with time zone | timestamp with time zone
-- 将本地时区+8时间转换为UTC时间,转化得到的是TIMESTAMP
-- 注意不要使用从TIMESTAMPTZ到TIMESTAMP的强制类型转换,会直接截断时区信息。
vonng=# SELECT now() AT TIME ZONE 'UTC';
timezone
----------------------------
2018-12-11 13:50:25.790108
-- 再将UTC时间转换为太平洋时间
vonng=# SELECT (now() AT TIME ZONE 'UTC') AT TIME ZONE 'PST';
timezone
-------------------------------
2018-12-12 05:50:37.770066+08
-- 查看PG自带的时区数据表
vonng=# TABLE pg_timezone_names LIMIT 4;
name | abbrev | utc_offset | is_dst
------------------+--------+------------+--------
Indian/Mauritius | +04 | 04:00:00 | f
Indian/Chagos | +06 | 06:00:00 | f
Indian/Mayotte | EAT | 03:00:00 | f
Indian/Christmas | +07 | 07:00:00 | f
...
-- 查看PG自带的时区缩写
vonng=# TABLE pg_timezone_abbrevs LIMIT 4;
abbrev | utc_offset | is_dst
--------+------------+--------
ACDT | 10:30:00 | t
ACSST | 10:30:00 | t
ACST | 09:30:00 | f
ACT | -05:00:00 | f
...
常见困惑:闰天
关于闰天,PostgreSQL处理的很好,但是需要特别注意的是对于闰年加减时间范围的运算规律。 例如,如果你在 ‘2024-02-29’ 号往前减“一年”,结果是 “2023-02-28”,但如果你减去 365 天,则是“2023-03-01”。 反过来,如果你都加一年,12个月或者加365天,结果都是明年的2月28号。 这样处理肯定是比那些直接用年份+1来计算的二傻子软件靠谱多了。
postgres=# SELECT '2023-02-29'::DATE; --# 2023 年不是闰年
ERROR: date/time field value out of range: "2023-02-29"
LINE 1: SELECT '2023-02-29'::DATE;
^
postgres=# SELECT '2024-02-29'::DATE;
today
------------
2024-02-29
postgres=# SELECT '2024-02-29'::DATE + '1year'::INTERVAL;
next_year
---------------------
2025-02-28 00:00:00
postgres=# SELECT '2024-02-29'::DATE + '365day'::INTERVAL;
next_365d
---------------------
2025-02-28 00:00:00
postgres=# SELECT '2024-02-29'::DATE - '1year'::INTERVAL;
prev_year
---------------------
2023-02-28 00:00:00
postgres=# SELECT '2024-02-29'::DATE - '365day'::INTERVAL;
prev_365d
---------------------
2023-03-01 00:00:00
常见困惑:时间戳互转
PostgreSQL 中一个经常让人困惑的问题就是TIMESTAMP
与TIMESTAMPTZ
之间的相互转化问题。
-- 使用 `::TIMESTAMP` 将 `TIMESTAMPTZ` 强制转换为 `TIMESTAMP`,会直接截断时区部分内容
-- 时间的其余"内容"保持不变
vonng=# SELECT now(), now()::TIMESTAMP;
now | now
-------------------------------+--------------------------
2018-12-12 05:50:37.770066+08 | 2018-12-12 05:50:37.770066+08
-- 对有时区版TIMESTAMPTZ使用AT TIME ZONE语法
-- 会将其转换为无时区版的TIMESTAMP,返回给定时区下的时间
vonng=# SELECT now(), now() AT TIME ZONE 'UTC';
now | timezone
-------------------------------+----------------------------
2019-05-23 16:58:47.071135+08 | 2019-05-23 08:58:47.071135
-- 对无时区版TIMESTAMP使用AT TIME ZONE语法
-- 会将其转换为带时区版的TIMESTAMPTZ,即在给定时区下解释该无时区时间戳。
vonng=# SELECT now()::TIMESTAMP, now()::TIMESTAMP AT TIME ZONE 'UTC';
now | timezone
----------------------------+-------------------------------
2019-05-23 17:03:00.872533 | 2019-05-24 01:03:00.872533+08
-- 这里的意思是,UTC时间的 2019-05-23 17:03:00
常见困惑:时区偏移量
当然 PostgreSQL 中的时间戳也有一个与时区相关的设计比较违反直觉,就是在使用 AT TIME ZONE
的时候,应该尽可能避免使用 +8
, -6
这样的数字时区,而应该使用时区名。
这是因为当你在时区部分使用数值时,PostgreSQL 会将其视作 Interval 类型进行处理,被解释为 “Fixed” Offsets from UTC,不常用,文档也不推荐使用。
举个例子,今天东八区中午 (SELECT ‘2024-01-15 12:00:00+08’::TIMESTAMPTZ) 转换为 UTC 时间戳,为 ‘2024-01-15 04:00:00’ (东八区的12点 = UTC0时区的4点)这没有问题:
2024-01-15 04:00:00
现在,我们使用 ‘+1’ 作为 zone,直观的想法应该是,+1 代表东一时区当前的时间,应该是 “2024-01-15 05:00:00+1” 但结果让人惊讶:反而是提前了一个小时
> SELECT '2024-01-15 12:00:00+08'::TIMESTAMPTZ AT TIME ZONE '+1';
2024-01-15 03:00:00
而如果我们反过来如果想当然的使用 -1
作为西一区的时区名,结果也是错误的:
> SELECT '2024-01-15 04:00:00+00'::TIMESTAMPTZ AT TIME ZONE '-1';
timezone
---------------------
2024-01-15 05:00:00
这里面的原因是,使用 Interval 而非时区名,处理逻辑是不一样的:
首先东八区 TIMESTAMPTZ '2024-01-15 12:00:00+08'
被转换为 UTC 时间的 TIMESTAMPTZ '2024-01-15 04:00:00+00'
。
然后 UTC时间的 TIMESTAMPTZ '2024-01-15 04:00:00+00'
被截断时区部分为 '2024-01-15 04:00:00'
并拼接新时区 +1
成为一个新的 TIMESTAMPTZ '2024-01-15 04:00:00+1'
,然后这个新的时间戳被重新转换为不带时间戳的 UTC 时间 '2024-01-15 03:00:00'
理解字符编码原理
程序员,是与Code(代码/编码)打交道的,而字符编码又是最为基础的编码。 如何使用二进制数来表示字符,这个字符编码问题并没有看上去那么简单,实际上它的复杂程度远超一般人的想象:输入、比较排序与搜索、反转、换行与分词、大小写、区域设置,控制字符,组合字符与规范化,排序规则,处理不同语言中的特异需求,变长编码,字节序与BOM,Surrogate,历史兼容性,正则表达式兼容性,微妙与严重的安全问题等等等等。
如果不了解字符编码的基本原理,即使只是简单常规的字符串比较、排序、随机访问操作,都可能会一不小心栽进大坑中。但根据我的观察,很多工程师与程序员却对字符编码本身几近一无所知,只是对诸如ASCII,Unicode,UTF这些名词有一些模糊的感性认识。因此尝试写这一篇科普文,希望能讲清楚这个问题。
0x01 基本概念
万物皆数 —— 毕达哥拉斯
为了解释字符编码,我们首先需要理解什么是编码,什么又是字符?
编码
从程序员的视角来看,我们有着许许多多的基础数据类型:整数,浮点数,字符串,指针。程序员将它们视作理所当然的东西,但从数字计算机的物理本质来看,只有一种类型才是真正的基础类型:二进制数。
而编码(Code)就是这些高级类型与底层二进制表示之间映射转换的桥梁。编码分为两个部分:编码(encode)与解码(decode),以无处不在的自然数为例。数字42,这个纯粹抽象的数学概念,在计算机中可能就会表示为00101010
的二进制位串(假设使用8位整型)。从抽象数字42到二进制数表示00101010
的这个过程就是编码。相应的,当计算机读取到00101010
这个二进制位串时,它会根据上下文将其解释为抽象的数字42,这个过程就是解码(decode)。
任何‘高级’数据类型与底层二进制表示之间都有着编码与解码的过程,比如单精度浮点数,这种看上去这么基础的类型,也存在着一套相当复杂的编码过程。例如在float32
中,1.0和-2.0就表示为如下的二进制串:
0 01111111 00000000000000000000000 = 1
1 10000000 00000000000000000000000 = −2
字符串当然也不例外。字符串是如此的重要与基础,以至于几乎所有语言都将其作为内置类型而实现。字符串,它首先是一个串(String),所谓串,就是由同类事物依序构成的序列。对于字符串而言,就是由**字符(Character)**构成的序列。字符串或字符的编码,实际上就是将抽象的字符序列映射为其二进制表示的规则。
不过,在讨论字符编码问题之前,我们先来看一看,什么是字符?
字符
字符是指字母、数字、标点、表意文字(如汉字)、符号、或者其他文本形式的书写“原子”。它是书面语中最小语义单元的抽象实体。这里说的字符都是抽象字符(abstract character),其确切定义是:用于组织、控制、显示文本数据的信息单元。
抽象字符是一种抽象的符号,与具体的形式无关:区分字符(character)与字形(Glyph)是非常重要的,我们在屏幕上看到的有形的东西是字形(Glyph),它是抽象字符的视觉表示形式。抽象字符通过渲染(Render)呈现为字形,用户界面呈现的字形通过人眼被感知,通过人脑被认知,最终又在人的大脑中还原为抽象的实体概念。字形在这个过程中起到了媒介的作用,但决不能将其等价为抽象字符本身。
要注意的是,虽然多数时候字形与字符是一一对应的,但仍然存在一些多对多的情况:一个字形可能由多个字符组合而成,例如抽象字符à
(拼音中的第四声a),我们将其视作单个‘字符’,但它既可以真的是一个单独的字符,也可以由字符a
与去声撇号字符 ̀
组合而成。另一方面,一个字符也可能由多个字形组成,例如很多阿拉伯语印地语中的文字,由很多图元(字形)组成的符号,复杂地像一幅画,实际上却是单个字符。
>>> print u'\u00e9', u'e\u0301',u'e\u0301\u0301\u0301'
é é é́́
字形的集合构成了字体(font),不过那些都属于渲染的内容:渲染是将字符序列映射为字形序列的过程。 那是另一个堪比字符编码的复杂主题,本文不会涉及渲染的部分,而专注于另一侧:将抽象字符转变为二进制字节序列的过程,即,字符编码(Character Encoding)。
思路
我们会想,如果有一张表,能将所有的字符一一映射到字节byte(s),问题不就解决了吗?实际上对于英文和一些西欧文字而言,这么做是很直观的想法,ASCII就是这样做的:它通过ASCII编码表,使用一个字节中的7位,将128个字符编码为相应的二进制值,一个字符正好对应一个字节(单射而非满射,一半字节没有对应字符)。一步到位,简洁、清晰、高效。
计算机科学发源于欧美,因而文本处理的问题,一开始指的就是英文处理的问题。不过计算机是个好东西,世界各族人民都想用。但语言文字是一个极其复杂的问题:学一门语言文字已经相当令人头大,更别提设计一套能够处理世界各国语言文字的编码标准了。从简单的ASCII发展到当代的大一统Unicode标准,人们遇到了各种问题,也走过一些弯路。
好在计算机科学中,有句俗语:“计算机科学领域的任何问题都可以通过增加一个间接的中间层来解决”。字符编码的模型与架构也是随着历史而不断演进的,下面我们就先来概览一下现代编码模型中的体系结构。
0x02 模型概览
- 现代编码模型自底向上分为五个层次:
- 抽象字符表(Abstract Character Repertoire, ACR)
- 编码字符集(Coded Character Set, CCS)
- 字符编码表(Character Encoding Form, CEF)
- 字符编码方案(Character Encoding Schema, CES)
- 传输编码语法(Transfer Encoding Syntax, TES)
我们所熟悉的诸多名词,都可以归类到这个模型的相应层次之中。例如,Unicode字符集(UCS),ASCII字符集,GBK字符集,这些都属于编码字符集CCS;而常见的UTF8,UTF16,UTF32这些概念,都属于字符编码表CEF,不过也有同名的字符编码方案CES。而我们熟悉的base64
,URLEncode
这些就属于传输编码语法TES。
这些概念之间的关系可以用下图表示:
可以看到,为了将一个抽象字符转换为二进制,中间其实经过了几次概念的转换。在抽象字符序列与字节序列间还有两种中间形态:码位序列与码元序列。简单来说:
-
所有待编码的抽象字符构成的集合,称为抽象字符集。
-
因为我们需要指称集合中的某个具体字符,故为每一个抽象字符指定一个唯一的自然数作为标识,这个被指定的自然数,就称作字符的码位(Code Point)。
-
码位与字符集中的抽象字符是一一对应的。抽象字符集中的字符经过编码就形成了编码字符集。
-
码位是正整数,但计算机的整数表示范围是有限的,因此需要调和无限的码位与有限的整型之间的矛盾。字符编码表将码位映射为码元序列(Code Unit Sequence),将整数转变为计算机中的整型。
-
计算机中多字节整型存在大小端字节序的问题,字符编码方案指明了字节序问题的解决方案。
Unicode标准为什么不像ASCII那样一步到位,直接将抽象字符映射为二进制表示呢?实际上如果只有一种字符编码方案,譬如UTF-8,那么确实是一步到位的。可惜因为一些历史原因(比如觉得65536个字符绝对够用了…),我们有好几种编码方案。但不论如何,比起各国自己搞的百花齐放的编码方案,Unicode的编码方案已经是非常简洁了。可以说,每一层都是为了解决一些问题而不得已引入的:
- 抽象字符集到编码字符集解决了唯一标识字符的问题(字形无法唯一标识字符);
- 编码字符集到字符编码表解决了无限的自然数到有限的计算机整型的映射问题(调和无限与有限);
- 字符编码方案则解决了字节序的问题(解决传输歧义)。
下面让我们来看一下每个层次之间的细节。
0x03 字符集
字符集,顾名思义就是字符的集合。字符是什么,在第一节中已经解释过了。在现代编码模型中, 有两种层次不同的字符集:抽象字符集 ACR与编码字符集 CCS。
抽象字符集 ACR
抽象字符集顾名思义,指的是抽象字符的集合。已经有了很多标准的字符集定义,US-ASCII, UCS(Unicode),GBK这些我们耳熟能详的名字,都是(或至少是)抽象字符集
US-ASCII定义了128个抽象字符的集合。GBK挑选了两万多个中日韩汉字和其他一些字符组成字符集,而UCS则尝试去容纳一切的抽象字符。它们都是抽象字符集。
- 抽象字符 英文字母
A
同时属于US-ASCII, UCS, GBK这三个字符集。 - 抽象字符 中文文字
蛤
不属于US-ASCII,属于GBK字符集,也属于UCS字符集。 - 抽象文字 Emoji
😂
不属于US-ASCII与GBK字符集,但属于UCS字符集。
抽象字符集可以使用类似set
的数据结构来表示:
# ACR
{"a","啊","あ","Д","α","å","😯"}
编码字符集 CCS
集合的一个重要特性,就是无序性。集合中的元素都是无序的,所以抽象字符集中的字符都是无序的。
这就带来一个问题,如何指称字符集中的某个特定字符呢?我们不能抽象字符的字形来指代其实体,原因如前面所说,看上去一样的字形,实际上可能由不同的字符组合而成(如字形à
就有两种字符组合方式)。对于抽象字符,我们有必要给它们分配唯一对应的ID,用关系型数据库的话来说,字符数据表需要一个主键。这个码位分配(Code Point Allocation)的操作就称为编码(Encode)。它将抽象字符与一个正整数关联起来。
如果抽象字符集中的所有字符都有了对应的码位(Code Point/Code Position),这个集合就升级成了映射:类似于从set
数据结构变成了dict
。我们称这个映射为编码字符集 CCS。
# CCS
{
"a": 97,
"啊": 21834,
"あ": 12354,
"Д": 1044,
"α": 945,
"å": 229,
"😯": 128559
}
注意这里的映射是单射,每个抽象字符都有唯一的正整数码位,但并不是所有的正整数都有对应的抽象字符。码位被分为七大类:图形,格式,控制,代理,非字符,保留。像代理(Surrogate, D800-DFFF)区中的码位,单独使用时就不对应任何字符。
抽象字符集与编码字符集之间的区别通常是Trivial的,毕竟指定字符的同时通常也会指定一个顺序,为每个字符分配一个数字ID。所以我们通常就将它们统称为字符集。字符集解决的问题是,将抽象字符单向映射为自然数。那么既然计算机已经解决了整数编码的问题,是不是直接用字符码位的整型二进制表示就可以了呢?
不幸的是,还有另外一个问题。字符集有开放与封闭之分,譬如ASCII字符集定义了128个抽象字符,再也不会增加。它就是一个封闭字符集。而Unicode尝试收纳所有的字符,一直在不断地扩张之中。截止至2016.06,Unicode 9.0.0已经收纳了128,237个字符,并且未来仍然会继续增长,它是一个开放的字符集。开放意味着字符的数量是没有上限的,随时可以添加新的字符,例如Emoji,几乎每年都会有新的表情字符被引入到Unicode字符集中。这就产生了一对内在的矛盾:无限的自然数与有限的整型值之间的矛盾。
而字符编码表,就是为了解决这个问题的。
0x04 字符编码表
字符集解决了抽象字符到自然数的映射问题,将自然数表示为二进制就是字符编码的另一个核心问题了。字符编码表(CEF)会将一个自然数,转换为一个或多个计算机内部的整型数值。这些整型数值称为码元。码元是能用于处理或交换编码文本的最小比特组合。
码元与数据的表示关系紧密,通常计算机处理字符的码元为一字节的整数倍:1字节,2字节,4字节。对应着几种基础的整型:uint8
, uint16
, uint32
,单字节、双字节、四字节整型。整形的计算往往以计算机的字长作为一个基础单元,通常来讲,也就是4字节或8字节。
曾经,人们以为使用16位短整型来表示字符就足够了,16位短整型可以表示2的十六次方个状态,也就是65536个字符,看上去已经足够多了。但是程序员们很少从这种事情上吸取教训:光是中国的汉字可能就有十万个,一个旨在兼容全世界字符的编码不能不考虑这一点。因此如果使用一个整型来表示一个码位,双字节的短整型int16
并不足以表示所有字符。另一方面,四字节的int32能表示约41亿个状态,在进入星辰大海宇宙文明的阶段之前,恐怕是不太可能有这么多的字符需要表示的。(实际上到现在也就分配了不到14万个字符)。
根据使用码元单位的不同,我们有了三种字符编码表:UTF8,UTF-16,UTF-32。
属性\编码 | UTF8 | UTF16 | UTF32 |
---|---|---|---|
使用码元 | uint8 |
uint16 |
uint32 |
码元长度 | 1byte = 8bit | 2byte = 16bit | 4byte = 32bit |
编码长度 | 1码位 = 1~4码元 | 1码位 = 1或2码元 | 1码位 = 1码元 |
独门特性 | 兼容ASCII | 针对BMP优化 | 定长编码 |
定长编码与变长编码
双字节的整数只能表示65536个状态,对于目前已有的十四万个字符显得捉襟见肘。但另一方面,四字节整数可以表示约42亿个状态。恐怕直到人类进入宇宙深空时都遇不到这么多字符。因此对于码元而言,如果采用四字节,我们可以确保编码是定长的:一个(表示字符的)自然数码位始终能用一个uint32
表示。但如果使用uint8
或uint16
作为码元,超出单个码元表示范围的字符就需要使用多个码元来表示了。因此是为变长编码。因此,UTF-32是定长编码,而UTF-8和UTF-16是变长编码。
设计编码时,容错是最为重要的考量之一:计算机并不是绝对可靠的,诸如比特反转,数据坏块等问题是很有可能遇到的。字符编码的一个基本要求就是自同步(self-synchronization )。对于变长编码而言,这个问题尤为重要。应用程序必须能够从二进制数据中解析出字符的边界,才可以正确解码字符。如果如果文本数据中出现了一些细微的错漏,导致边界解析错误,我们希望错误的影响仅仅局限于那个字符,而不是后续所有的文本边界都失去了同步,变成乱码无法解析。
为了保证能够从编码的二进制中自然而然的凸显出字符边界,所有的变长编码方案都应当确保编码之间不会出现重叠(Overlap):譬如一个双码元的字符,其第二个码元本身不应当是另一个字符的表示,否则在出现错误时,程序无法分辨出它到底是一个单独的字符,还是某个双码元字符的一部分,就达不到自同步的要求。我们在UTF-8和UTF-16中可以看到,它们的编码表都是针对这一于要求而设计的。
下面让我们来看一下三种具体的编码表:UTF-32, UTF-16, UTF-8。
UTF32
最为简单的编码方案,就是使用一个四字节标准整型int32
表示一个字符,也就是采用四字节32位无符号整数作为码元,即,UTF-32。很多时候计算机内部处理字符时,确实是这么做的。例如在C语言和Go语言中,很多API都是使用int
来接收单个字符的。
UTF-32最突出的特性是定长编码,一个码位始终编码为一个码元,因此具有随机访问与实现简单的优势:第n个字符,就是数组中的第n个码元,使用简单,实现更简单。当然这样的编码方式有个缺陷:特别浪费存储。虽然总共有十几万个字符,但即使是中文,最常用的字符通常码位也落在65535以内,可以使用两个字节来表示。而对于纯英文文本而言,只要一个字节来表示一个字符就足够了。因此使用UTF32可能导致二至四倍的存储消耗,都是真金白银啊。当然在内存与磁盘容量没有限制的时候,用UTF32可能是最为省心的做法。
UTF16
UTF16是一种变长编码,使用双字节16位无符号整型作为码元。位于U+0000-U+FFFF之间的码位使用单个16位码元表示,而在U+10000-U+10FFFF之间的码位则使用两个16位的码元表示。这种由两个码元组成的码元对儿,称为代理对(Surrogate Paris)。
UTF16是针对**基本多语言平面(Basic Multilingual Plane, BMP)**优化的,也就是码位位于U+FFFF以内可以用单个16位码元表示的部分。Anyway,对于落在BMP内的高频常用字符而言,UTF-16可以视作定长编码,也就有着与UTF32一样随机访问的好处,但节省了一倍的存储空间。
UTF-16源于早期的Unicode标准,那时候人们认为65536个码位足以表达所有字符了。结果汉字一种文字就足够打爆它了……。**代理(Surrogate)**就是针对此打的补丁。它通过预留一部分码位作为特殊标记,将UTF-16改造成了变长编码。很多诞生于那一时期的编程语言与操作系统都受此影响(Java,Windows等)
对于需要权衡性能与存储的应用,UTF-16是一种选择。尤其是当所处理的字符集仅限于BMP时,完全可以假装它是一种定长编码。需要注意的是UTF-16本质上是变长的,因此当出现超出BMP的字符时,如果以定长编码的方式来计算处理,很可能会出现错误,甚至崩溃。这也是为什么很多应用无法正确处理Emoji的原因。
UTF8
UTF8是一种完完全全的变长编码,它使用单字节8位无符号整数作为码元。0xFF以内的码位使用单字节编码,且与ASCII保持完全一致;U+0100-U+07FF之间的码位使用两个字节;U+0800到U+FFFF之间的码位使用三字节,超出U+FFFF的码位使用四字节,后续还可以继续扩展到最多用7个字节来表示一个字符。
UTF8最大的优点,一是面向字节编码,二是兼容ASCII,三是能够自我同步。众所周知,只有多字节的类型才会存在大小端字节序的问题,如果码元本身就是单个字节,就压根不存在字节序的问题了。而兼容性,或者说ASCII透明性,使得历史上海量使用ASCII编码的程序与文件无需任何变动就能继续在UTF-8编码下继续工作(ASCII范围内)。最后,自我同步机制使得UTF-8具有良好的容错性。
这些特性这使得UTF-8非常适合用于信息的传输与交换。互联网上大多数文本文件的编码都是UTF-8。而Go、Python3也采用了UTF-8作为其默认编码。
当然,UTF-8也是有代价的。对于中文而言,UTF-8通常使用三个字节进行编码。比起双字节编码而言带来了50%的额外存储开销。与此同时,变长编码无法进行随机访问字符,也使得处理相比“定长编码”更为复杂,也会有更高的计算开销。对于正确性不甚在乎,但对性能有严苛要求的中文文字处理应用可能不会喜欢UTF-8。
UTF-8的一个巨大优势就在于,它没有字节序的问题。而UTF-16与UTF-32就不得不操心大端字节在前还是小端字节在前的问题了。这个问题通常在**字符编码方案(Character Encoding Schema)**中通过BOM来解决。
字符编码方案
字符编码表 CEF解决了如何将自然数码位编码为码元序列的问题,无论使用哪种码元,计算机中都有相应的整型。但我们可以说编码问题就解决了吗?还不行,假设一个字符按照UTF16拆成了若干个码元组成的码元序列,因为每个码元都是一个uint16
,实际上各由两个字节组成。因此将码元序列化为字节序列的时候,就会遇到一些问题:每个码元究竟是高位字节在前还是低位字节在前呢?这就是大小端字节序问题。
对于网络交换和本地处理,大小端序各有优劣,因此不同的系统往往也会采用不同的大小端序。为了标明二进制文件的大小端序,人们引入了**字节序标记(Byte Order Mark, BOM)**的概念。BOM是放置于编码字节序列开始处的一段特殊字节序列,用于表示文本序列的大小端序。
字符编码方案,实质上就是带有字节序列化方案的字符编码表。即:CES = 解决端序问题的CEF。对于大小端序标识方法的不同选择,产生了几种不同的字符编码方案:
- UTF-8:没有端序问题。
- UTF-16LE:小端序UTF-16,不带BOM
- UTF-16BE:大端序UTF-16,不带BOM
- UTF-16:通过BOM指定端序
- UTF-32LE:小端序UTF-32,不带BOM
- UTF-32BE:大端序UTF-32,不带BOM
- UTF-32:通过BOM指定端序
UTF-8因为已经采用字节作为码元了,所以实际上不存在字节序的问题。其他两种UTF,都有三个相应地字符编码方案:一个大端版本,一个小端版本,还有一个随机应变大小端带 BOM的版本。
当然要注意,在当前上下文中的UTF-8,UTF-16,UTF-32其实是CES层次的概念,即带有字节序列化方案的CEF,这会与CEF层次的同名概念产生混淆。因此,当我们在说UTF-8,UTF-16,UTF-32时,一定要注意区分它是CEF还是CES。例如,作为一种编码方案的UTF-16产生的字节序列是会带有BOM的,而作为一种编码表的UTF-16产生的码元序列则是没有BOM这个概念的。
0x05 UTF-8
介绍完了现代编码模型,让我们深入看一下一个具体的编码方案:UTF-8。 UTF-8将Unicode码位映射成1~4个字节,满足如下规则:
标量值 | 字节1 | 字节2 | 字节3 | 字节4 |
---|---|---|---|---|
00000000 0xxxxxxx |
0xxxxxxx |
|||
00000yyy yyxxxxxx |
110yyyyy |
10xxxxxx |
||
zzzzyyyy yyxxxxxx |
1110zzzz |
10yyyyyy |
10xxxxxx |
|
000uuuuu zzzzyyyy yyxxxxxx |
11110uuu |
10uuzzzz |
10yyyyyy |
10xxxxxx |
其实比起死记硬背,UTF-8的编码规则可以通过几个约束自然而然地推断出来:
- 与ASCII编码保持兼容,因此有第一行的规则。
- 需要有自我同步机制,因此需要在首字节中保有当前字符的长度信息。
- 需要容错机制,码元之间不允许发生重叠,这意味着字节2,3,4,…不能出现字节1可能出现的码元。
0, 10, 110, 1110, 11110, …
这些是不会发生冲突的字节前缀,0
前缀被ASCII兼容规则对应的码元用掉了。次优的10
前缀就分配给后缀字节作为前缀,表示自己是某个字符的外挂部分。相应地,110,1110,11110
这几个前缀就用于首字节中的长度标记,例如110
前缀的首字节就表示当前字符还有一个额外的外挂字节,而1110
前缀的首字节就表示还有两个额外的外挂字节。因此,UTF-8的编码规则其实非常简单。下面是使用Go语言编写的函数,展示了将一个码位编码为UTF-8字节序列的逻辑:
func UTF8Encode(i uint32) (b []byte) {
switch {
case i <= 0xFF: /* 1 byte */
b = append(b, byte(i))
case i <= 0x7FF: /* 2 byte */
b = append(b, 0xC0|byte(i>>6))
b = append(b, 0x80|byte(i)&0x3F)
case i <= 0xFFFF: /* 3 byte*/
b = append(b, 0xE0|byte(i>>12))
b = append(b, 0x80|byte(i>>6)&0x3F)
b = append(b, 0x80|byte(i)&0x3F)
default: /* 4 byte*/
b = append(b, 0xF0|byte(i>>18))
b = append(b, 0x80|byte(i>>12)&0x3F)
b = append(b, 0x80|byte(i>>6)&0x3F)
b = append(b, 0x80|byte(i)&0x3F)
}
return
}
0x06 编程语言中的字符编码
讲完了现代编码模型,让我们来看两个现实编程语言中的例子:Go和Python2。这两者都是非常简单实用的语言。但在字符编码的模型设计上却是两个典型:一个正例一个反例。
Go
Go语言的缔造者之一,Ken Thompson,同时也是UTF-8的发明人(同时也是C语言,Go语言,Unix的缔造者),因此Go对于字符编码的实现堪称典范。Go的语法与C和Python类似,非常简单。它也是一门比较新的语言,抛开了一些历史包袱,直接使用了UTF-8作为默认编码。
UTF-8编码在Go语言中有着特殊的位置,无论是源代码的文本编码,还是字符串的内部编码都是UTF-8。Go绕开前辈语言们踩过的坑,使用了UTF8作为默认编码是一个非常明智的选择。相比之下,Java,Javascript都使用 UCS-2/UTF16作为内部编码,早期还有随机访问的优势,可当Unicode增长超出BMP之后,这一优势也荡然无存了。相比之下,字节序,Surrogate , 空间冗余带来的麻烦却仍让人头大无比。
Go语言中有三种重要的基本文本类型: byte
, rune
,string
,分别是字节,字符,与字符串。其中:
- 字节
byte
实际上是uint8
的别名,[]byte
表示字节序列。 - 字符
rune
实质上是int32
的别名,表示一个Unicode的码位。[]rune
表示码位序列 - 字符串
string
实质上是UTF-8编码的二进制字节数组(底层是字节数组),加上一个长度字段。
而相应的编码与解码操作为:
- 编码:使用
string(rune_array)
将字符数组转换为UTF-8编码的字符串。 - 解码:使用
for i,r := range str
语法迭代字符串中的字符,实际上是依次将二进制UTF-8字节序列还原为码位序列。
更详细的内容可以参阅文档,我也写过一篇博文详细解释了Go语言中的文本类型。
Python2
如果说Go可以作为字符编码处理实现的典范,那么Python2则可以当做一个最典型的反例了。Python2使用ASCII作为默认编码以及默认源文件编码,因此如果不理解字符编码的相关知识,以及Python2的一些设计,在处理非ASCII编码很容易出现一些错误。实际上只要看到Python3与Python2在字符编码处理上的差异有多大就大概有数了。Python2用的人还是不少,所以这里的坑其实很多,但其实最严重的问题是:
- Python2的默认编码方案的非常不合理。
- Python2的字符串类型与字符串字面值很容易让人混淆。
第一个问题是,Python2的默认编码方案的非常不合理:
- Python2使用
'xxx'
作为字节串字面值,其类型为<str>
,但<str>
本质上是字节串而不是字符串。 - Python2使用
u'xxx'
作为字符串字面值的语法,其类型为<unicode>
,<unicode>
是真正意义上的字符串,每一个字符都属于UCS。
与此同时,Python2解释器的默认编码方案(CES)是US-ASCII 。作为对照,Java,C#,Javascript等语言内部的默认编码方案都是UTF-16,Go语言的内部默认编码方案使用UTF-8。默认使用US-ASCII的python2简直是骨骼清奇,当然,这也有一部分历史原因在里头。Python3就乖乖地改成UTF-8了。
第二个问题:python的默认’字符串类型<str>
与其叫字符串,不如叫字节串,用下标去访问的每一个元素都是一个字节。而<unicode>
类型才是真正意义上的字符串,用下标去访问的每一个元素都是一个字符(虽然底下可能每个字符长度不同)。字符串<unicode>
与 字节串<str>
的关系为:
- 字符串
<unicode>
通过 字符编码方案编码得到字节串<str>
- 字节串
<str>
通过 字符编码方案解码得到字符串<unicode>
字节串就字节串,为啥要起个类型名叫<str>
呢?另外,字面值语法用一对什么前缀都没有的引号表示str,这样的设计非常反直觉。因此让很多人掉进了坑里。当然,<str>
与<unicode>
这样的类型设计以及两者的关系设计本身是无可厚非的。该黑的应该是这两个类型起的名字和字面值表示方法。至于怎么改进是好的,Python3已经给出答案。在理解了字符编码模型之后,什么样的操作才是正确的操作,读者应该已经心里有数了。
并发异常那些事
并发程序很难写对,更难写好。很多程序员也没有真正弄清楚这些问题,不过是一股脑地把这些问题丢给数据库而已。并发异常并不仅仅是一个理论问题:这些异常曾经造成过很多资金损失,耗费过大量财务审计人员的心血。但即使是最流行、最强大的关系型数据库(通常被认为是“ACID”数据库),也会使用弱隔离级别,所以它们也不一定能防止这些并发异常的发生。
比起盲目地依赖工具,我们应该对存在的并发问题的种类,以及如何防止这些问题有深入的理解。 本文将阐述SQL92标准中定义的隔离级别及其缺陷,现代模型中的隔离级别与定义这些级别的异常现象。
0x01 引子
大多数数据库都会同时被多个客户端访问。如果它们各自读写数据库的不同部分,这是没有问题的,但是如果它们访问相同的数据库记录,则可能会遇到并发异常。
下图是一个简单的并发异常案例:两个客户端同时在数据库中增长一个计数器。(假设数据库中没有自增操作)每个客户端需要读取计数器的当前值,加1再回写新值。因为有两次增长操作,计数器应该从42增至44;但由于并发异常,实际上只增长至43。
图 两个客户之间的竞争状态同时递增计数器
事务ACID特性中的I,即隔离性(Isolation)就是为了解决这种问题。隔离性意味着,同时执行的事务是相互隔离的:它们不能相互踩踏。传统的数据库教科书将隔离性形式化为可串行化(Serializability),这意味着每个事务可以假装它是唯一在整个数据库上运行的事务。数据库确保当事务已经提交时,结果与它们按顺序运行(一个接一个)是一样的,尽管实际上它们可能是并发运行的。
如果两个事务不触及相同的数据,它们可以安全地并行(parallel)运行,因为两者都不依赖于另一个。当一个事务读取由另一个事务同时进行修改的数据时,或者当两个事务试图同时修改相同的数据时,并发问题(竞争条件)才会出现。只读事务之间不会有问题,但只要至少一个事务涉及到写操作,就有可能出现冲突,或曰:并发异常。
并发异常很难通过测试找出来,因为这样的错误只有在特殊时机下才会触发。这样的时机可能很少,通常很难重现。也很难对并发问题进行推理研究,特别是在大型应用中,你不一定知道有没有其他的应用代码正在访问数据库。在一次只有一个用户时,应用开发已经很麻烦了,有许多并发用户使其更加困难,因为任何数据都可能随时改变。
出于这个原因,数据库一直尝试通过提供**事务隔离(transaction isolation)**来隐藏应用开发中的并发问题。从理论上讲,隔离可以通过假装没有并发发生,让程序员的生活更加轻松:可串行化的隔离等级意味着数据库保证事务的效果与真的串行执行(即一次一个事务,没有任何并发)是等价的。
实际上不幸的是:隔离并没有那么简单。可串行化会有性能损失,许多数据库与应用不愿意支付这个代价。因此,系统通常使用较弱的隔离级别来防止一部分,而不是全部的并发问题。这些弱隔离等级难以理解,并且会导致微妙的错误,但是它们仍然在实践中被使用。一些流行的数据库如Oracle 11g,甚至没有实现可串行化。在Oracle中有一个名为“可串行化”的隔离级别,但实际上它实现了一种叫做**快照隔离(snapshot isolation)**的功能,这是一种比可串行化更弱的保证。
在研究现实世界中的并发异常前,让我们先来复习一下SQL92标准定义的事务隔离等级。
0x02 SQL92标准
按照ANSI SQL92的标准,三种**现象(phenomena)**区分出了四种隔离等级,如下表所示:
隔离等级 | 脏写P0 | 脏读 P1 | 不可重复读 P2 | 幻读 P3 |
---|---|---|---|---|
读未提交RU | ✅ | ⚠️ | ⚠️ | ⚠️ |
读已提交RC | ✅ | ✅ | ⚠️ | ⚠️ |
可重复读RR | ✅ | ✅ | ✅ | ⚠️ |
可串行化SR | ✅ | ✅ | ✅ | ✅ |
- 四种现象分别缩写为P0,P1,P2,P3,P是**现象(Phenonmena)**的首字母。
- 脏写没有在标准中指明,但却是任何隔离等级都需要必须避免的异常
这四种异常可以概述如下:
P0 脏写(Dirty Write)
事务T1修改了数据项,而另一个事务T2在T1提交或回滚之前就修改了T1修改的数据项。
无论如何,事务必须避免这种情况。
P1 脏读(Dirty Read)
事务T1修改了数据项,另一个事务T2在T1提交或回滚前就读到了这个数据项。
如果T1选择了回滚,那么T2实际上读到了一个事实上不存在(未提交)的数据项。
P2 不可重复读( Non-repeatable or Fuzzy Read)
事务T1读取了一个数据项,然后另一个事务T2修改或删除了该数据项并提交。
如果T1尝试重新读取该数据项,它就会看到修改过后的值,或发现值已经被删除。
P3 幻读(Phantom)
事务T1读取了满足某一搜索条件的数据项集合,事务T2创建了新的满足该搜索条件的数据项并提交。
如果T1再次使用同样的搜索条件查询,它会获得与第一次查询不同的结果。
标准的问题
SQL92标准对于隔离级别的定义是有缺陷的 —— 模糊,不精确,并不像标准应有的样子独立于实现。标准其实针对的是基于锁调度的实现来讲的,而基于多版本的实现就很难对号入座。有几个数据库实现了“可重复读”,但它们实际提供的保证存在很大的差异,尽管表面上是标准化的,但没有人真正知道可重复读的意思。
标准还有其他的问题,例如在P3中只提到了创建/插入的情况,但实际上任何写入都可能导致异常现象。 此外,标准对于可串行化也语焉不详,只是说“SERIALIZABLE
隔离级别必须保证通常所知的完全序列化执行”。
现象与异常
现象(phenomena)与异常(anomalies)并不相同。现象不一定是异常,但异常肯定是现象。例如在脏读的例子中,如果T1回滚而T2提交,那么这肯定算一种异常:看到了不存在的东西。但无论T1和T2各自选择回滚还是提交,这都是一种可能导致脏读的现象。通常而言,异常是一种严格解释,而现象是一种宽泛解释。
0x03 现代模型
相比之下,现代的隔离等级与一致性等级对于这个问题有更清晰的阐述,如图所示:
图:隔离等级偏序关系图
图:一致性与隔离等级偏序关系
右子树主要讨论的是多副本情况下的一致性等级,略过不提。为了讨论便利起见,本图中刨除了MAV、CS、I-CI、P-CI等隔离等级,主要需要关注的是快照隔离SI。
表:各个隔离等级及其可能出现的异常现象
等级\现象 | P0 | P1 | P4C | P4 | P2 | P3 | A5A | A5B |
---|---|---|---|---|---|---|---|---|
读未提交 RU | ✅ | ⚠️ | ⚠️ | ⚠️ | ⚠️ | ⚠️ | ⚠️ | ⚠️ |
读已提交 RC | ✅ | ✅ | ⚠️ | ⚠️ | ⚠️ | ⚠️ | ⚠️ | ⚠️ |
游标稳定性 CS | ✅ | ✅ | ✅ | ⚠️? | ⚠️? | ⚠️ | ⚠️ | ⚠️? |
可重复读 RR | ✅ | ✅ | ✅ | ✅ | ✅ | ⚠️ | ✅ | ✅ |
快照隔离 SI | ✅ | ✅ | ✅ | ✅ | ✅ | ✅? | ✅ | ⚠️ |
可序列化 SR | ✅ | ✅ | ✅ | ✅ | ✅ | ✅ | ✅ | ✅ |
带有?标记的表示可能出现异常,依具体实现而异。
主流关系型数据库的实际隔离等级
相应地,将主流关系型数据库为了“兼容标准”而标称的隔离等级映射到现代隔离等级模型中,如下表所示:
表:主流关系型数据库标称隔离等级与实际隔离之间的对照关系
实际\标称 | PostgreSQL/9.2+ | MySQL/InnoDB | Oracle(11g) | SQL Server |
---|---|---|---|---|
读未提交 RU | RU | RU | ||
读已提交 RC | RC | RC, RR | RC | RC |
可重复读 RR | RR | |||
快照隔离 SI | RR | SR | SI | |
可序列化 SR | SR | SR | SR |
以PostgreSQL为例
如果按照ANSI SQL92标准来看,PostgreSQL实际上只有两个隔离等级:RC与SR。
隔离等级 | 脏读 P1 | 不可重复读 P2 | 幻读 P3 |
---|---|---|---|
RU,RC | ✅ | ⚠️ | ⚠️ |
RR,SR | ✅ | ✅ | ✅ |
其中,RU和RC隔离等级中可能出现P2与P3两种异常情况。而RR与SR则能避免P1,P2,P3所有的异常。
当然实际上如果按照现代隔离等级模型,PostgreSQL的RR隔离等级实际上是快照隔离SI,无法解决A5B写偏差的问题。直到9.2引入可串行化快照隔离SSI之后才有真正意义上的SR,如下表所示:
标称 | 实际 | P2 | P3 | A5A | P4 | A5B |
---|---|---|---|---|---|---|
RC | RC | ⚠️ | ⚠️ | ⚠️ | ⚠️ | ⚠️ |
RR | SI | ✅ | ✅ | ✅ | ✅ | ⚠️ |
SR | SR | ✅ | ✅ | ✅ | ✅ | ✅ |
作为一种粗略的理解,可以将RC等级视作语句级快照,而将RR等级视作事务级快照。
以MySQL为例
MySQL的RR隔离等级因为无法阻止丢失更新问题,被认为没有提供真正意义上的快照隔离/可重复读。
标称 | 实际 | P2 | P3 | A5A | P4 | A5B |
---|---|---|---|---|---|---|
RC | RC | ⚠️ | ⚠️ | ⚠️ | ⚠️ | ⚠️ |
RR | RC | ✅ | ✅? | ✅ | ⚠️ | ⚠️ |
SR | SR | ✅ | ✅ | ✅ | ✅ | ✅ |
参考测试用例:ept/hermitage/mysql
0x04 并发异常
回到这张图来,各个异常等级恰好就是通过可能出现的异常来定义的。如果在某个隔离等级A中会出现的所有异常都不会在隔离等级B中出现,我们就认为隔离等级A弱于隔离等级B。但如果某些异常在等级A中出现,在等级B中避免,同时另一些异常在等级B中出现,却在A中避免,这两个隔离等级就无法比较强弱了。
例如在这幅图中:RR与SI是明显强于RC的。但RR与SI之间的相对强弱却难以比较。SI能够避免RR中可能出现的幻读P3,但会出现写偏差A5B的问题;RR不会出现写偏差A5B,但有可能出现P3幻读。
防止脏写与脏读可以简单地通过数据项上的读锁与写锁来阻止,其形式化表示为:
P0: w1[x]...w2[x]...((c1 or a1) and (c2 or a2)) in any order)
P1: w1[x]...r2[x]...((c1 or a1) and (c2 or a2)) in any order)
A1: w1[x]...r2[x]...(a1 and c2 in any order)
因为大多数数据库使用RC作为默认隔离等级,因此脏写P0,脏读P1等异常通常很难遇到,就不再细说了。
下面以PostgreSQL为例,介绍这几种通常情况下可能出现的并发异常现象:
- P2:不可重复读
- P3:幻读
- A5A:读偏差
- P4:丢失跟新
- A5B:写偏差
这五种异常有两种分类方式,第一可以按照隔离等级来区分。
- P2,P3,A5A,P4是RC中会出现,RR不会出现的异常;A5B是RR中会出现,SR中不会出现的异常。
第二种分类方式是按照冲突类型来分类:只读事务与读写事务之间的冲突,以及读写事务之间的冲突。
- P2,P3,A5A是读事务与写事务之间的并发异常,而P4与A5B则是读写事务之间的并发异常。
读-写异常
让我们先来考虑一种比较简单的情况:一个只读事务与一个读写事务之间的冲突。例如:
- P2:不可重复读。
- A5A:读偏差(一种常见的不可重复读问题)
- P3:幻读
在PostgreSQL中,这三种异常都会在RC隔离等级中出现,但使用RR(实际为SI)隔离等级就不会有这些问题。
不可重复读 P2
假设我们有一张账户表,存储了用户的银行账户余额,id是用户标识,balance是账户余额,其定义如下
CREATE TABLE account(
id INTEGER PRIMARY KEY,
balance INTEGER
);
譬如,在事务1中前后进行两次相同的查询,但两次查询间,事务2写入并提交,结果查询得到的结果不同。
START TRANSACTION ISOLATION LEVEL READ COMMITTED; -- T1, RC, 只读
START TRANSACTION ISOLATION LEVEL READ COMMITTED; -- T2, RC, 读写
SELECT * FROM account WHERE k = 'a'; -- T1, 查询账户a,看不到任何结果
INSERT INTO account VALUES('a', 500); -- T2, 插入记录(a,500)
COMMIT; -- T2, 提交
SELECT * FROM account WHERE id = 'a'; -- T1, 重复查询,得到结果(a,500)
COMMIT; -- T1陷入迷惑,为什么同样的查询结果不同?
对于事务1而言,在同一个事务中执行相同的查询,竟然会出现不一样的结果,也就是说读取的结果不可重复。这就是不可重复读的一个例子,即现象P2。在PostgreSQL的RC级别中是会出现的,但如果将事务T1的隔离等级设置为RR,就不会出现这种问题了:
START TRANSACTION ISOLATION LEVEL REPEATABLE READ; -- T1, RR, 只读
START TRANSACTION ISOLATION LEVEL READ COMMITTED; -- T2, RC, 读写
SELECT * FROM counter WHERE k = 'x'; -- T1, 查询不到任何结果
INSERT INTO counter VALUES('x', 10); -- T2, 插入记录(x,10) @ RR
COMMIT; -- T2, 提交
SELECT * FROM counter WHERE k = 'x'; -- T1, 还是查询不到任何结果
COMMIT; -- T1, 在RR下,两次查询的结果保持一致。
不可重复读的形式化表示:
P2: r1[x]...w2[x]...((c1 or a1) and (c2 or a2) in any order)
A2: r1[x]...w2[x]...c2...r1[x]...c1
读偏差 A5A
另一类读-写异常是读偏差(A5A):考虑一个直观的例子,假设用户有两个账户:a和b,各有500元。
-- 假设有一张账户表,用户有两个账户a,b,各有500元。
CREATE TABLE account(
id INTEGER PRIMARY KEY,
balance INTEGER
);
INSERT INTO account VALUES('a', 500), ('b', 500);
现在用户向系统提交从账户b向账户a转账100元的请求,并从网页上并查看自己的账户余额。在RC隔离级别下,下列操作历史的结果可能会让用户感到困惑:
START TRANSACTION ISOLATION LEVEL READ COMMITTED; -- T1, RC, 只读,用户观察
START TRANSACTION ISOLATION LEVEL READ COMMITTED; -- T2, RC, 读写,系统转账
SELECT * FROM account WHERE id = 'a'; -- T1, 用户查询账户a, 500元
UPDATE account SET balance -= 100 WHERE id = 'b'; -- T2, 系统扣减账户b 100元
UPDATE account SET balance += 100 WHERE id = 'a'; -- T2, 系统添加账户a 100元
COMMIT; -- T2, 系统转账事务提交提交
SELECT * FROM account WHERE id = 'a'; -- T1, 用户查询账户b, 400元
COMMIT; -- T1, 用户陷入迷惑,为什么我总余额(400+500)少了100元?
这个例子中,只读事务读取到了系统的一个不一致的快照。这种现象称为读偏差(read skew),记作A5A。但其实说到底,读偏差的根本原因是不可重复读。只要避免了P2,自然能避免A5A。
但读偏差是很常见的一类问题,在一些场景中,我们希望获取一致的状态快照,读偏差是不能接受的。一个典型的场景就是备份。通常对于大型数据库,备份需要花费若干个小时。备份进程运行时,数据库仍然会接受写入操作。因此如果存在读偏差,备份可能会包含一些旧的部分和一些新的部分。如果从这样的备份中恢复,那么不一致(比如消失的钱)就会变成永久的。此外,一些长时间运行的分析查询通常也希望能在一个一致的快照上进行。如果一个查询在不同时间看见不同的东西,那么返回的结果可能毫无意义。
快照隔离是这个问题最常见的解决方案。PostgreSQL的RR隔离等级实际上就是快照隔离,提供了事务级一致性快照的功能。例如,如果我们将T1的隔离等级设置为可重复读,就不会有这个问题了。
START TRANSACTION ISOLATION LEVEL REPEATABLE READ; -- T1, RR, 只读,用户观察
START TRANSACTION ISOLATION LEVEL READ COMMITTED; -- T2, RC, 读写,系统转账
SELECT * FROM account WHERE id = 'a'; -- T1 用户查询账户a, 500元
UPDATE account SET balance -= 100 WHERE id = 'b'; -- T2 系统扣减账户b 100元
UPDATE account SET balance += 100 WHERE id = 'a'; -- T2 系统添加账户a 100元
COMMIT; -- T2, 系统转账事务提交提交
SELECT * FROM account WHERE id = 'a'; -- T1 用户查询账户b, 500元
COMMIT; -- T1没有观察到T2的写入结果{a:600,b:400},但它观察到的是一致性的快照。
读偏差的形式化表示:
A5A: r1[x]...w2[x]...w2[y]...c2...r1[y]...(c1 or a1)
幻读 P3
在ANSI SQL92中,幻读是用于区分RR和SR的现象,实际上它经常与不可重复读P2混为一谈。唯一的区别在于读取列时是否使用了谓词(predicate),也就是Where条件。 将上一个例子中查询是否存在账户,变为满足特定条件账户的数目,就成了一个所谓的“幻读”问题。
START TRANSACTION ISOLATION LEVEL READ COMMITTED; -- T1, RC, 只读
START TRANSACTION ISOLATION LEVEL READ COMMITTED; -- T2, RC, 读写
SELECT count(*) FROM account WHERE balance > 0; -- T1, 查询有存款的账户数目。0
INSERT INTO account VALUES('a', 500); -- T2, 插入记录(a,500)
COMMIT; -- T2, 提交
SELECT count(*) FROM account WHERE balance > 0; -- T1, 查询有存款的账户数目。1
COMMIT; -- T1陷入迷惑,为什么冒出来一个人?
同理,事务1在使用PostgreSQL的RR隔离级别之后,事务1就不会看到满足谓词P的结果发生变化了。
START TRANSACTION ISOLATION LEVEL REPEATABLE READ; -- T1, RR, 只读
START TRANSACTION ISOLATION LEVEL READ COMMITTED; -- T2, RC, 读写
SELECT count(*) FROM account WHERE balance > 0; -- T1, 查询有存款的账户数目。0
INSERT INTO account VALUES('a', 500); -- T2, 插入记录(a,500)
COMMIT; -- T2, 提交
SELECT count(*) FROM account WHERE balance > 0; -- T1, 查询有存款的账户数目。0
COMMIT; -- T1, 读取到了一致的快照(虽然不是最新鲜的)
之所以有这种相当Trivial的区分,因为基于锁的隔离等级实现往往需要额外的谓词锁机制来解决这类特殊的读-写冲突问题。但是基于MVCC的实现,以PostgreSQL的SI为例,就天然地一步到位解决了所有这些问题。
幻读的形式化表示:
P3: r1[P]...w2[y in P]...((c1 or a1) and (c2 or a2) any order)
A3: r1[P]...w2[y in P]...c2...r1[P]...c1
幻读会出现在MySQL的RC,RR隔离等级中,但不会出现在PostgreSQL的RR隔离等级(实际为SI)中。
写-写异常
上面几节讨论了只读事务在并发写入时可能发生的异常。通常这种读取异常可能只要稍后重试就会消失,但如果涉及到写入,问题就比较严重了,因为这种读取到的暂时不一致状态很可能经由写入变成永久性的不一致…。到目前为止我们只讨论了在并发写入发生时,只读事务可以看见什么。如果两个事务并发执行写入,还可能会有一种更有趣的写-写异常:
- P4: 丢失更新:PostgreSQL的RC级别存在,RR级别不存在(MySQL的RR会存在)。
- A5B:写入偏差:PostgreSQL的RR隔离级别会存在。
其中,写偏差(A5B)可以视作丢失更新(P4)的泛化情况。快照隔离能够解决丢失更新的问题,却无法解决写入偏差的问题。解决写入偏差需要真正的可串行化隔离等级。
丢失更新-P4-例1
仍然以上文中的账户表为例,假设有一个账户x,余额500元。
CREATE TABLE account(
id TEXT PRIMARY KEY,
balance INTEGER
);
INSERT INTO account VALUES('x', 500);
有两个事务T1,T2,分别希望向该账户打入两笔钱,比如一笔100,一笔200。从顺序执行的角度来看,无论两个事务谁先执行,最后的结果都应当是余额 = 500 + 200 + 100 = 800。
START TRANSACTION ISOLATION LEVEL READ COMMITTED; -- T1
START TRANSACTION ISOLATION LEVEL READ COMMITTED; -- T2
SELECT balance FROM account WHERE id = 'x'; -- T1, 查询当前余额=500
SELECT balance FROM account WHERE id = 'x'; -- T2, 查询当前余额也=500
UPDATE account SET balance = 500 + 100; -- T1, 在原余额基础上增加100元
UPDATE account SET balance = 500 + 200; -- T2, 在原余额基础上增加200元,被T1阻塞。
COMMIT; -- T1, 提交前可以看到余额为600。T1提交后解除对T2的阻塞,T2进行了更新。
COMMIT; -- T2, T2提交,提交前可以看到余额为700
-- 最终结果为700
但奇妙的时机导致了意想不到的结果,最后账户的余额为700元,事务1的转账更新丢失了!
但令人意外的是,两个事务都看到了UPDATE 1的更新结果,都检查了自己更新的结果无误,都收到了事务成功提交的确认。结果事务1的更新丢失了,这就很尴尬了。最起码事务应当知道这里可能出现问题,而不是当成什么事都没有就混过去了。
如果使用RR隔离等级(主要是T2,T1可以是RC,但出于对称性尽量都用RR),后执行更新的语句就会报错中止事务。这就允许应用知耻而后勇,进行重试。
START TRANSACTION ISOLATION LEVEL REPEATABLE READ; -- T1, 这里RC也可以
START TRANSACTION ISOLATION LEVEL REPEATABLE READ; -- T2, 关键是T2必须为RR
SELECT balance FROM account WHERE id = 'x'; -- T1, 查询当前余额=500
SELECT balance FROM account WHERE id = 'x'; -- T2, 查询当前余额也=500
UPDATE account SET balance = 500 + 100; -- T1, 在原余额基础上增加100元
UPDATE account SET balance = 500 + 200; -- T2, 在原余额基础上增加200元,被T1阻塞。
COMMIT; -- T1, 提交前可以看到余额为600。T1提交后解除对T2的阻塞
-- T2 Update报错:ERROR: could not serialize access due to concurrent update
ROLLBACK; -- T2, T2只能回滚
-- 最终结果为600,但T2知道了错误可以重试,并在无竞争的环境中最终达成正确的结果800。
当然我们可以看到,在RC隔离等级的情况中,T1提交,解除对T2的阻塞时,Update操作已经能够看到T1的变更了(balance=600)。但事务2还是用自己先前计算出的增量值覆盖了T1的写入。对于这种特殊情况,可以使用原子操作解决,例如:UPDATE account SET balance = balance + 100;
。这样的语句在RC隔离级别中也能正确地并发更新账户。但并不是所有的问题都能简单到能用原子操作来解决的,让我们来看另一个例子。
丢失更新-P4-例2
让我们来看一个更微妙的例子:UPDATE
与DELETE
之间的冲突。
假设业务上每人最多有两个账户,用户最多能选择一个账号作为有效账号,管理员会定期删除无效账号。
账户表有一个字段valid表示该账户是否有效,定义如下所示:
CREATE TABLE account(
id TEXT PRIMARY KEY,
valid BOOLEAN
);
INSERT INTO account VALUES('a', TRUE), ('b', FALSE);
现在考虑这样一种情况,用户要切换自己的有效账号,与此同时管理员要清理无效账号。
从顺序执行的角度来看,无论是用户先切换还是管理员先清理,最后结果的共同点是:总会有一个账号被删掉。
START TRANSACTION ISOLATION LEVEL READ COMMITTED; -- T1, 用户更换有效账户
START TRANSACTION ISOLATION LEVEL READ COMMITTED; -- T2, 管理员删除账户
UPDATE account SET valid = NOT valid; -- T1, 原子操作,将有效账户无效账户状态反转
DELETE FROM account WHERE NOT valid; -- T2, 管理员删除无效账户。
COMMIT; -- T1, 提交,T1提交后解除对T2的阻塞
-- T2 DELETE执行完毕,返回DELETE 0
COMMIT; -- T2, T2能正常提交,但检查的话会发现自己没有删掉任何记录。
-- 无论T2选择提交还是回滚,最后的结果都是(a,f),(b,t)
从下图中可以看到,事务2的DELETE原本锁定了行(b,f)
准备删除,但因为事务1的并发更新而阻塞。当T1提交解除T2的阻塞时,事务2看见了事务1的提交结果:自己锁定的那一行已经不满足删除条件了,因此只好放弃删除。
相应的,改用RR隔离等级,至少给了T2知道错误的机会,在合适的时机重试就可以达到序列执行的效果。
START TRANSACTION ISOLATION LEVEL REPEATABLE READ; -- T1, 用户更换有效账户
START TRANSACTION ISOLATION LEVEL REPEATABLE READ; -- T2, 管理员删除账户
UPDATE account SET valid = NOT valid; -- T1, 原子操作,将有效账户无效账户状态反转
DELETE FROM account WHERE NOT valid; -- T2, 管理员删除无效账户。
COMMIT; -- T1, 提交,T1提交后解除对T2的阻塞
-- T2 DELETE报错:ERROR: could not serialize access due to concurrent update
ROLLBACK; -- T2, T2只能回滚
SI隔离级别小结
上面提到的异常,包括P2,P3,A5A,P4,都会在RC中出现,但却不会在SI中出现。特别需要注意的是,P3幻读问题会在RR中出现,却不会在SI中出现。从ANSI标准的意义上,SI可以算是可串行化了。SI解决的问题一言以蔽之,就是提供了真正的事务级别的快照。因而各种读-写异常(P2,P3,A5A)都不会再出现了。而且,SI还可以解决**丢失更新(P4)**的问题(MySQL的RR解决不了)。
丢失更新是一种非常常见的问题,因此也有不少应对的方法。典型的方式有三种:原子操作,显式锁定,冲突检测。原子操作通常是最好的解决方案,前提是你的逻辑可以用原子操作来表达。如果数据库的内置原子操作没有提供必要的功能,防止丢失更新的另一个选择是让应用显式地锁定将要更新的对象。然后应用程序可以执行读取-修改-写入序列,如果任何其他事务尝试同时读取同一个对象,则强制等待,直到第一个读取-修改-写入序列完成。(例如MySQL和PostgreSQL的SELECT FOR UPDATE
子句)
另一种应对丢失更新的方法是自动冲突检测。如果事务管理器检测到丢失更新,则中止事务并强制它们重试其读取-修改-写入序列。这种方法的一个优点是,数据库可以结合快照隔离高效地执行此检查。事实上,PostgreSQL的可重复读,Oracle的可串行化和SQL Server的快照隔离级别,都会自动检测到丢失更新,并中止惹麻烦的事务。但是,MySQL/InnoDB的可重复读并不会检测丢失更新。一些专家认为,数据库必须能防止丢失更新才称得上是提供了快照隔离,所以在这个定义下,MySQL下没有提供快照隔离。
但正所谓成也快照败也快照,每个事务都能看到一致的快照,却带来了一些额外的问题。在SI等级中,一种称为写偏差(A5B)的问题仍然可能会发生:例如两个事务基于过时的快照更新了对方读取的数据,提交后才发现违反了约束。丢失更新其实是写偏差的一种特例:两个写入事务竞争写入同一条记录。竞争写入同一条数据能够被数据库的丢失更新检测机制发现,但如果两个事务基于各自的写入了不同的数据项,又怎么办呢?
写偏差 A5B
考虑一个运维值班的例子:互联网公司通常会要求几位运维同时值班,但底线是至少有一位运维在值班。运维可以翘班,只要至少有一个同事在值班就行:
CREATE TABLE duty (
name TEXT PRIMARY KEY,
oncall BOOLEAN
);
-- Alice和Bob都在值班
INSERT INTO duty VALUES ('Alice', TRUE), ('Bob', True);
假如应用逻辑约束是:不允许无人值班。即:SELECT count(*) FROM duty WHERE oncall
值必须大于0。现在假设A和B两位运维正在值班,两人都感觉不舒服决定请假。不幸的是两人同时按下了翘班按钮。则下列执行时序会导致异常的结果:
START TRANSACTION ISOLATION LEVEL REPEATABLE READ; -- T1, Alice
START TRANSACTION ISOLATION LEVEL REPEATABLE READ; -- T2, Bob
SELECT count(*) FROM duty WHERE oncall; -- T1, 查询当前值班人数, 2
SELECT count(*) FROM duty WHERE oncall; -- T2, 查询当前值班人数, 2
UPDATE duty SET oncall = FALSE WHERE name = 'Alice'; -- T1, 认为其他人在值班,Alice翘班
UPDATE duty SET oncall = FALSE WHERE name = 'Bob'; -- T2, 也认为其他人在值班,Bob翘班
COMMIT; -- T1
COMMIT; -- T2
SELECT count(*) FROM duty; -- 观察者, 结果为0, 没有人在值班了!
两个事务看到了同一个一致性快照,首先检查翘班条件,发现有两名运维在值班,那么自己翘班是ok的,于是更新自己的值班状态并提交。两个事务都提交之后,没有运维在值班了,违背了应用定义的一致性。
但如果两个事务并不是**同时(Concurrently)**执行的,而是分了先后次序,那么后一个事务在执行检查时就会发现不满足翘班条件而终止。因此,事务之间的并发导致了异常现象。
对事务而言,明明在执行翘班操作之前看到值班人数为2,执行翘班操作之后看到值班人数为1,但为啥提交之后看到的就是0了呢?这就好像看见幻象一样,但这与SQL92标准定义的幻读并不一样,标准定义的幻读是因为不可重复读的屁股没擦干净,读到了不该读的东西(对于谓词查询不可重复读取),而这里则是因为快照的存在,事务无法意识到自己读取的记录已经被改变。
问题的关键在于不同读写事务之间的读写依赖。如果某个事务读取了一些数据作为行动的前提,那么如果当该事务执行后续写入操作时,这些被读取的行已经被其他事务修改,这就意味着事务依赖的前提可能已经改变。
写偏差的形式化表示:
A5B: r1[x]...r2[y]...w1[y]...w2[x]...(c1 and c2 occur)
此类问题的共性
事务基于一个前提采取行动(事务开始时候的事实,例如:“目前有两名运维正在值班”)。之后当事务要提交时,原始数据可能已经改变——前提可能不再成立。
-
一个
SELECT
查询找出符合条件的行,并检查是否满足一些约束(至少有两个运维在值班)。 -
根据第一个查询的结果,应用代码决定是否继续。(可能会继续操作,也可能中止并报错)
-
如果应用决定继续操作,就执行写入(插入、更新或删除),并提交事务。
**这个写入的效果改变了步骤2 中的先决条件。**换句话说,如果在提交写入后,重复执行一次步骤1 的SELECT查询,将会得到不同的结果。因为写入改变符合搜索条件的行集(只有一个运维在值班)。
在SI中,每个事务都拥有自己的一致性快照。但SI是不提供**线性一致性(强一致性)**保证的。事务看到的快照副本可能因为其他事务的写入而变得陈旧,但事务中的写入无法意识到这一点。
与丢失更新的联系
作为一个特例,如果不同读写事务是对同一数据对象进行写入,这就成了丢失更新问题。通常会在RC中出现,在RR/SI隔离级别中避免。对于相同对象的并发写入可以被数据库检测出来,但如果是向不同数据对象写入,违背应用逻辑定义的约束,那RR/SI隔离等级下的数据库就无能为力了。
解决方案
有很多种方案能应对这些问题,可串行化当然是ok的,但也存在一些其他手段,例如锁
显式锁定
START TRANSACTION ISOLATION LEVEL REPEATABLE READ; -- T1, 用户更换有效账户
START TRANSACTION ISOLATION LEVEL REPEATABLE READ; -- T2, 管理员删除账户
SELECT count(*) FROM duty WHERE oncall FOR UPDATE; -- T1, 查询当前值班人数, 2
SELECT count(*) FROM duty WHERE oncall FOR UPDATE; -- T2, 查询当前值班人数, 2
WITH candidate AS (SELECT name FROM duty WHERE oncall FOR UPDATE)
SELECT count(*) FROM candidate; -- T1
WITH candidate AS (SELECT name FROM duty WHERE oncall FOR UPDATE)
SELECT count(*) FROM candidate; -- T2, 被T1阻塞
UPDATE duty SET oncall = FALSE WHERE name = 'Alice'; -- T1, 执行更新
COMMIT; -- T1, 解除T2的阻塞
-- T2报错:ERROR: could not serialize access due to concurrent update
ROLLBACK; -- T2 只能回滚
使用SELECT FOR UPDATE
语句,可以显式锁定待更新的行,这样,当后续事务想要获取相同的锁时就会被阻塞。这种方法在MySQL中称为悲观锁。这种方法本质上属于一种物化冲突,将写偏差的问题转换成了丢失更新的问题,因此允许在RR级别解决原本SR级别才能解决的问题。
在最极端的情况下(比如表没有唯一索引),显式锁定可能蜕化为表锁。无论如何,这种方式都有相对严重的性能问题,而且可能更频繁地导致死锁。因此也存一些基于谓词锁和索引范围锁的优化。
显式约束
如果应用逻辑定义的约束可以使用数据库约束表达,那是最方便的。因为事务会在提交时(或语句执行时)检查约束,违背了约束的事务会被中止。不幸的是,很多应用约束都难以表述为数据库约束,或者难以承受这种数据库约束表示的性能负担。
可串行化
使用可串行化隔离等级可以避免这一问题,这也是可串行化的定义:避免一切序列化异常。这可能是最简单的方法了,只需要使用SERIALIZABLE
的事务隔离等级就可以了。
START TRANSACTION ISOLATION LEVEL SERIALIZABLE; -- T1, Alice
START TRANSACTION ISOLATION LEVEL SERIALIZABLE; -- T2, Bob
SELECT count(*) FROM duty WHERE oncall; -- T1, 查询当前值班人数, 2
SELECT count(*) FROM duty WHERE oncall; -- T2, 查询当前值班人数, 2
UPDATE duty SET oncall = FALSE WHERE name = 'Alice'; -- T1, 认为其他人在值班,Alice翘班
UPDATE duty SET oncall = FALSE WHERE name = 'Bob'; -- T2, 也认为其他人在值班,Bob翘班
COMMIT; -- T1
COMMIT; -- T2, 报错中止
-- ERROR: could not serialize access due to read/write dependencies among transactions
-- DETAIL: Reason code: Canceled on identification as a pivot, during commit attempt.
-- HINT: The transaction might succeed if retried.
在事务2提交的时候,会发现自己读取的行已经被T1改变了,因此中止了事务。稍后重试很可能就不会有问题了。
PostgreSQL使用SSI实现可串行化隔离等级,这是一种乐观并发控制机制:如果有足够的备用容量,并且事务之间的争用不是太高,乐观并发控制技术往往表现比悲观的要好不少。
数据库约束,物化冲突在某些场景下是很方便的。如果应用约束能用数据库约束表示,那么事务在写入或提交时就会意识到冲突并中止冲突事务。但并不是所有的问题都能用这种方式解决的,可串行化的隔离等级是一种更为通用的方案。
0x06 并发控制技术
本文简要介绍了并发异常,这也是事务ACID中的“隔离性”所要解决的问题。本文简单讲述了ANSI SQL92标准定义的隔离等级以及其缺陷,并简单介绍了现代模型中的隔离等级(简化)。最后详细介绍了区分隔离等级的几种异常现象。当然,这篇文章只讲异常问题,不讲解决方案与实现原理,关于这些隔离等级背后的实现原理,将留到下一篇文章来陈述。但这里可以大概提一下:
从宽泛的意义来说,有两大类并发控制技术:多版本并发控制(MVCC),严格两阶段锁定(S2PL),每种技术都有多种变体。
在MVCC中,每个写操作都会创建数据项的一个新版本,同时保留旧版本。当事务读取数据对象时,系统会选择其中的一个版本,来确保各个事务间相互隔离。 MVCC的主要优势在于“读不会阻塞写,而写也不会阻塞读”。相反,基于S2PL的系统在写操作发生时必须阻塞读操作,因为因为写入者获取了对象的排他锁。
PostgreSQL、SQL Server、Oracle使用一种MVCC的变体,称为快照隔离(Snapshot Isolation,SI)。为了实现SI,一些RDBMS(例如Oracle)使用回滚段。当写入新的数据对象时,旧版本的对象先被写入回滚段,随后用新对象覆写至数据区域。 PostgreSQL使用更简单的方法:一个新数据对象被直接插入到相关的表页中。读取对象时,PostgreSQL通过可见性检查规则,为每个事物选择合适的对象版本作为响应。
但数据库技术发展至今,这两种技术已经不是那样泾渭分明,进入了一个你中有我我中有你的状态:例如在PostgreSQL中,DML操作使用SI/SSI,而DDL操作仍然会使用2PL。但具体的细节,就留到下一篇吧。
Reference
【1】Designing Data-Intensive Application,ch7
【2】Highly Available Transactions: Virtues and Limitations
【3】A Critique of ANSI SQL Isolation Levels
【4】Granularity of Locks and Degrees of Consistency in a Shared Data Base
【5】Hermitage: Testing the ‘I’ in ACID
区块链与分布式数据库
区块链的本质,想提供的功能,及其演化方向,就是分布式数据库。
确切的讲,是拜占庭容错(抗恶意节点攻击)的分布式(无领导者复制)数据库。
如果这种分布式数据库用来存储各种币的交易记录,这个系统就叫做所谓的“XX币”。例如以太坊就是这样一个分布式数据库,上面除了记载着各种山寨币的交易记录,还可以记载各种奇奇怪怪的内容。花一点以太币,就可以在这个分布式数据库里留下一条记录(一封信)。而所谓智能合约就是这个分布式数据库上的存储过程。
从形式上看,区块链 与 预写式日志(Write-Ahead-Log, WAL, Binlog, Redolog) 在设计原理上是高度一致的。
WAL是数据库的核心数据结构,记录了从数据库创建之初到当前时刻的所有变更,用于实现主从复制、备份回滚、故障恢复等功能。如果保留了全量的WAL日志,就可以从起点回放WAL,时间旅行到任意时刻的状态,如PostgreSQL的PITR。
区块链其实就是这样一份日志,它记录了从创世以来的每笔Transaction。回放日志就可以还原数据库任意时刻的状态(反之则不成立)。所以区块链当然可以算作某种意义上的数据库。
区块链的两大特性:去中心化与防篡改,用数据库的概念也很好理解:
- 去中心化的实质就是无领导者复制(leaderless replication),核心在于分布式共识。
- 防篡改的实质就是拜占庭容错,即,使得 篡改WAL的计算代价在概率上不可行 。
正如WAL分为日志段,区块链也被划分为一个一个 区块 ,且每一段带有先前日志段的哈希指纹。
所谓挖矿就是一个公开的猜数字比快游戏(满足条件的数字才会被共识承认),先猜中者能获取下一个日志段的初夜权:向日志段里写一笔向自己转账的记录(就是挖矿的奖励),并广播出去(如果别人也猜中了,以先广播至多数为准)。所有节点通过共识算法,保证当前最长的链为权威日志版本。区块链通过共识算法实现日志段的无主复制。
而如果想要修改某个WAL日志段中的一比交易记录,比如,转给自己一万个比特币,需要把这个区块以及其后所有区块的指纹给凑出来(连猜几次数字),并让多数节点相信这个伪造版本才行(拼一个更长的伪造版本,意味着猜更多次数字)。比特币中六个区块确认一个交易就是这个意思,篡改六个日志段之前的记录的算例代价,通常在概率上是不可行的。区块链通过这种机制(如Merkle树)实现拜占庭容错。
区块链涉及到的相关技术中,除了分布式共识外都很简单,但这种应用方式与机制设计确实是相当惊艳的。区块链可以算是一次数据库的演化尝试,长期来看前景广阔。但搞链能立竿见影起作用的领域,好像都是老大哥的地盘。而且不管怎么吹嘘,现在的区块链离真正意义上的分布式数据库还差的太远,所以现在入场搞应用的大概率都是先烈。
一致性:过载的术语
一致性这个词重载的很厉害,在不同的语境和上下文中,它其实代表着不同的东西:
- 在事务的上下文中,比如ACID里的C,指的就是通常的一致性(Consistency)
- 在分布式系统的上下文中,例如CAP里的C,实际指的是线性一致性(Linearizability)
- 此外,“一致性哈希”,“最终一致性”这些名词里的“一致性”也有不同的涵义。
这些一致性彼此不同却又有着千丝万缕的联系,所以经常会把人绕晕。
-
在事务的上下文中,一致性(Consistency) 的概念是:对数据的一组特定陈述必须始终成立。即不变量(invariants)。具体到分布式事务的上下文中这个不变量是:所有参与事务的节点状态保持一致:要么全部成功提交,要么全部失败回滚,不会出现一些节点成功一些节点失败的情况。
-
在分布式系统的上下文中,线性一致性(Linearizability) 的概念是:多副本的系统能够对外表现地像只有单个副本一样(系统保证从任何副本读取到的值都是最新的),且所有操作都以原子的方式生效(一旦某个新值被任一客户端读取到,后续任意读取不会再返回旧值)。
-
线性一致性这个词可能有些陌生,但说起它的另一个名字大家就清楚了:强一致性(strong consistency) ,当然还有一些诨名:原子一致性(atomic consistency),立即一致性(immediate consistency) 或 外部一致性(external consistency ) 说的都是它。
这两个“一致性”完全不是一回事儿,但之间其实有着微妙的联系,它们之间的桥梁就是共识(Consensus)
简单来说
- 分布式事务一致性会因为协调者单点引入可用性问题
- 为了解决可用性问题,分布式事务的节点需要在协调者故障时就新协调者选取达成共识
- 解决共识问题等价于实现一个线性一致的存储
- 解决共识问题等价于实现全序广播(total order boardcast)
- Paxos/Raft 实现了全序广播
具体来讲
-
为了保证分布式事务的一致性,分布式事务通常需要一个协调者(Coordinator)/事务管理器(Transaction Manager)来决定事务的最终提交状态。但无论2PC还是3PC,都无法应对协调者失效的问题,而且具有扩大故障的趋势。这就牺牲了可靠性、可维护性与可扩展性。为了让分布式事务真正可用,就需要在协调者挂点的时候能赶快选举出一个新的协调者来解决分歧,这就需要所有节点对谁是Boss达成共识(Consensus)。
-
共识意味着让几个节点就某事达成一致,可以用来确定一些互不相容的操作中,哪一个才是赢家。共识问题通常形式化如下:一个或多个节点可以提议(propose)某些值,而共识算法决定采用其中的某个值。在保证分布式事务一致性的场景中,每个节点可以投票提议,并对谁是新的协调者达成共识。
-
共识问题与许多问题等价,两个最典型的问题就是:
- 实现一个具有线性一致性的存储系统
- 实现全序广播(保证消息不丢失,且消息以相同的顺序传递给每个节点。)
Raft算法解决了全序广播问题。维护多副本日志间的一致性,其实就是让所有节点对同全局操作顺序达成一致,也其实就是让日志系统具有线性一致性。 因而解决了共识问题。(当然正因为共识问题与实现强一致存储问题等价,Raft的具体实现etcd
其实就是一个线性一致的分布式数据库。)
总结一下
-
分布式事务中的一致性则与事务ACID中的C一脉相承,并不是一个严格的术语。(因为什么叫一致,什么叫不一致其实是应用说了算。在分布式事务的场景下可以认为是:所有节点的事务状态始终保持相同)
-
分布式事务本身的一致性是通过协调者内部的原子操作与多阶段提交协议保证的,不需要共识;但解决分布式事务一致性带来的可用性问题需要用到共识。
参考阅读
[1] 一致性与共识
为什么要学习数据库原理
我们学校开了数据库系统原理课程。但是我还是很迷茫,这几节课老师一上来就讲一堆令人头大的名词概念,我以为我们知道“如何设计构建表”,“如何mysql增删改查”就行了……那为什么还要了解关系模式的表示方法,计算,规范化……概念模型……各种模型的相互转换,为什么还要了解什么关系代数,什么笛卡尔积……这些的理论知识。我十分困惑,通过这些理论概念,该课的目的或者说该书的目的究竟是想让学生学会什么呢?
只会写代码的是码农;学好数据库,基本能混口饭吃;在此基础上再学好操作系统和计算机网络,就能当一个不错的程序员。如果能再把离散数学、数字电路、体系结构、数据结构/算法、编译原理学通透,再加上丰富的实践经验与领域特定知识,就能算是一个优秀的工程师了。(前端算IO密集型应用就别抬杠了)
计算机其实就是存储/IO/CPU三大件; 而计算说穿了就是两个东西:数据与算法(状态与转移函数)。常见的软件应用,除了各种模拟仿真、模型训练、视频游戏这些属于计算密集型应用外,绝大多数都属于数据密集型应用。从最抽象的意义上讲,这些应用干的事儿就是把数据拿进来,存进数据库,需要的时候再拿出来。
抽象是应对复杂度的最强武器。操作系统提供了对存储的基本抽象:内存寻址空间与磁盘逻辑块号。文件系统在此基础上提供了文件名到地址空间的KV存储抽象。而数据库则在其基础上提供了对应用通用存储需求的高级抽象。
在真实世界中,除非准备从基础组件的轮子造起,不然根本没那么多机会去摆弄花哨的数据结构和算法(对数据密集型应用而言)。甚至写代码的本事可能也没那么重要:可能只会有那么一两个Ad Hoc算法需要在应用层实现,大部分需求都有现成的轮子可以使用,主要的创造性工作往往是在数据模型设计上。实际生产中,数据表就是数据结构,索引与查询就是算法。而应用代码往往扮演的是胶水的角色,处理IO与业务逻辑,其他大部分的工作都是在数据系统之间搬运数据。
在最宽泛的意义上,有状态的地方就有数据库。它无所不在,网站的背后、应用的内部,单机软件,区块链里,甚至在离数据库最远的Web浏览器中,也逐渐出现了其雏形:各类状态管理框架与本地存储。“数据库”可以简单地只是内存中的哈希表/磁盘上的日志,也可以复杂到由多种数据系统集成而来。关系型数据库只是数据系统的冰山一角(或者说冰山之巅),实际上存在着各种各样的数据系统组件:
- 数据库:存储数据,以便自己或其他应用程序之后能再次找到(PostgreSQL,MySQL,Oracle)
- 缓存:记住开销昂贵操作的结果,加快读取速度(Redis,Memcached)
- 搜索索引:允许用户按关键字搜索数据,或以各种方式对数据进行过滤(ElasticSearch)
- 流处理:向其他进程发送消息,进行异步处理(Kafka,Flink)
- 批处理:定期处理累积的大批量数据(Hadoop)
架构师最重要的能力之一,就是了解这些组件的性能特点与应用场景,能够灵活地权衡取舍、集成拼接这些数据系统。绝大多数工程师都不会去从零开始编写存储引擎,因为在开发应用时,数据库已经是足够完美的工具了。关系型数据库则是目前所有数据系统中使用最广泛的组件,可以说是程序员吃饭的主要家伙,重要性不言而喻。
了解意义(WHY)比了解方法(HOW)更重要。但一个很遗憾的现实是,以大多数学生,甚至相当一部分公司能够接触到的现实问题而言,拿几个文件甚至在内存里放着估计都能应付大多数场景了(需求简单到低级抽象就可以Handle)。没什么机会接触到数据库真正要解决的问题,也就难有真正使用与学习数据库的驱动力,更别提数据库原理了。当软硬件故障把数据搞成一团浆糊(可靠性);当单表超出了内存大小,并发访问的用户增多(可扩展性),当代码的复杂度发生爆炸,开发陷入泥潭(可维护性),人们才会真正意识到数据库的重要性。所以我也理解当前这种填鸭教学现状的苦衷:工作之后很难有这么大把的完整时间来学习原理了,所以老师只好先使劲灌输,多少让学生对这些知识有个印象。等学生参加工作后真正遇到这些问题,也许会想起大学好像还学了个叫数据库的东西,这些知识就会开始反刍。
数据库,尤其是关系型数据库,非常重要。那为什么要学习其原理呢?
对优秀的工程师来说,只会用数据库是远远不够的。学习原理对于当CRUD BOY搬砖收益并不大,但当通用组件真的无解需要自己撸起袖子上时,没有金坷垃怎么种庄稼?设计系统时,理解原理能让你以最少的复杂度代价写出更可靠高效的代码;遇到疑难杂症需要排查时,理解原理能带来精准的直觉与深刻的洞察。
数据库是一个博大精深的领域,存储I/O计算无所不包。其主要原理也可以粗略分为几个部分:数据模型设计原理(应用)、存储引擎原理(基础)、索引与查询优化器的原理(性能)、事务与并发控制的原理(正确性)、故障恢复与复制系统的原理(可靠性)。所有的原理都有其存在意义:为了解决实际问题。
例如数据模型设计中的范式理论,就是为了解决数据冗余这一问题而提出的,它是为了把事情做漂亮(可维护)。它是模型设计中一个很重要的设计权衡:通常而言,冗余少则复杂度小/可维护性强,冗余高则性能好。比如用了冗余字段,那更新时原本一条SQL就搞定的事情,现在现在就要用两条SQL更新两个地方,需要考虑多对象事务,以及并发执行时可能的竞态条件。这就需要仔细权衡利弊,选择合适的规范化等级。数据模型设计,就是生产中的数据结构设计。不了解这些原理,就难以提取良好的抽象,其他工作也就无从谈起。
而关系代数与索引的原理,则在查询优化中扮演重要的角色,它是为了把事情做得快(性能,可扩展) 。当数据量越来越大,SQL写的越来越复杂时,它的意义就会体现出来:怎样写出等价但是更高效的查询? 当查询优化器没那么智能时,就需要人来干这件事。这种优化往往成本极小而收益巨大,比如一个需要几秒的KNN查询,如果知道R树索引的原理,就可以通过改写查询,创建GIST索引优化到1毫秒内,千倍的性能提升。不了解索引与查询设计原理,就难以充分发挥数据库的性能。
事务与并发控制的原理,是为了把事情做正确(可靠性) 。事务是数据处理领域最伟大的抽象之一,它提供了很多有用的保证(ACID),但这些保证到底意味着什么?事务的原子性让你在提交前能随时中止事务并丢弃所有写入,相应地,事务的 持久性 则承诺一旦事务成功提交,即使发生硬件故障或数据库崩溃,写入的任何数据也不会丢失。这让错误处理变得无比简单:要么成功完事,要么失败重试。有了后悔药,程序员不用再担心半路翻车会留下惨不忍睹的车祸现场了。
另一方面,事务的隔离性则保证同时执行的事务无法相互影响(Serializable), 数据库提供了不同的隔离等级保证,以供程序员在性能与正确性之间进行权衡。编写并发程序并不容易,在几万TPS的负载下,各种极低概率,匪夷所思的问题都会出现:事务之间相互踩踏,丢失更新,幻读与写入偏差,慢查询拖慢快查询导致连接堆积,单表数据库并发增大后的性能急剧恶化,甚至快慢查询都减少但因比例变化导致的灵异抽风。这些问题,在低负载的情况下会潜伏着,随着规模量级增长突然跳出来,给你一个大大的惊喜。现实中真正可能出现的各类异常,也绝非SQL标准中简单的几种异常能说清的。不理解事务的原理,意味着应用的正确性与数据的完整性可能遭受不必要的损失。
故障恢复与复制的原理,可能对于程序员没有那么重要,但架构师与DBA必须清楚。高可用是很多应用的追求目标,但什么是高可用,高可用怎么保证?读写分离?快慢分离?异地多活?x地x中心?说穿了底下的核心技术其实就是复制(Replication)(或再加上自动故障切换(Failover))。这里有无穷无尽的坑:复制延迟带来的各种灵异现象,网络分区与脑裂,存疑事务blahblah。不理解复制的原理,高可用就无从谈起。
对于一些程序员而言,可能数据库就是“增删改查”,包一包接口,原理似乎属于“屠龙之技”。如果止步于此,那原理确实没什么好学的,但有志者应当打破砂锅问到底的精神。私认为只了解自己本领域知识是不够的,只有把当前领域赖以建立的上层领域摸清楚,才能称为专家。在数据库面前,后端也是前端;对于程序员知识栈而言,数据库是一个合适的栈底。
上面讲了WHY,下面就说一下 HOW
数据库教学的一个矛盾是:如果连数据库都不会用,那学数据库原理有个卵用呢?
学数据库的原则是学以致用。只有实践,才能带来对问题的深刻理解;只有先知其然,才有条件去知其所以然。教材可以先草草的过一遍,然后直接去看数据库文档,上手去把数据库用起来,做个东西出来。通过实践掌握数据库的使用,再去学习原理就会事半功倍(以及充满动力)。对于学习而言,有条件去实习当然最好,没有条件那最好的办法就是自己创造场景,自己挖掘需求。
比如,从解决个人需求开始:管理个人密码,体重跟踪,记账,做个小网站、在线聊天小程序。当它演化的越来越复杂,开始有多个用户,出现各种蛋疼问题之后,你就会开始意识到事务的意义。
再比如,结合爬虫,抓一些房价、股价、地理、社交网络的数据存在数据库里,做一些挖掘与分析。当你积累的数据越来越多,分析查询越来越复杂;SQL长得没法读,跑起来慢出猪叫,这时候关系代数的理论就能指导你进一步进行优化。
当你意识到这些设计都是为了解决现实生产中的问题,并亲自遇到过这些问题之后,再去学习原理,才能相互印证,并知其所以然。当你发现查询时间随数据增长而指数增长时;当你遇到成千上万的用户同时读写为并发控制焦头烂额时;当你碰上软硬件故障把数据搅得稀巴烂时;当你发现数据冗余让代码复杂度快速爆炸时;你就会发现这些设计存在的意义。
教材、书籍、文档、视频、邮件组、博客都是很好的学习资源。教材的话华章的黑皮系列教材都还不错,《数据库系统概念》这本就挺好的。但我推荐先看看这本书:《设计数据密集型应用》 ,写的非常好,我觉得不错就义务翻译了一下。纸上得来终觉浅,绝知此事要躬行。实践方能出真知,新手上路选哪家?个人推荐世界上最先进的开源关系型数据库PostgreSQL,设计优雅,功能强大。传教就有请德哥出场了:https://github.com/digoal/blog 。有时间的话可以再看看Redis,源码简单易读,实践中也很常用,非关系型数据库也应当多了解一下。
最后,关系型数据库虽然强大,却绝非数据处理的终章,尽可能多地去尝试各种各样的数据库吧。
知乎原题:计算机系为什么要学数据库原理和设计?